back to indexE167: Nvidia smashes earnings (again), Google's Woke AI disaster, Groq's LPU breakthrough & more
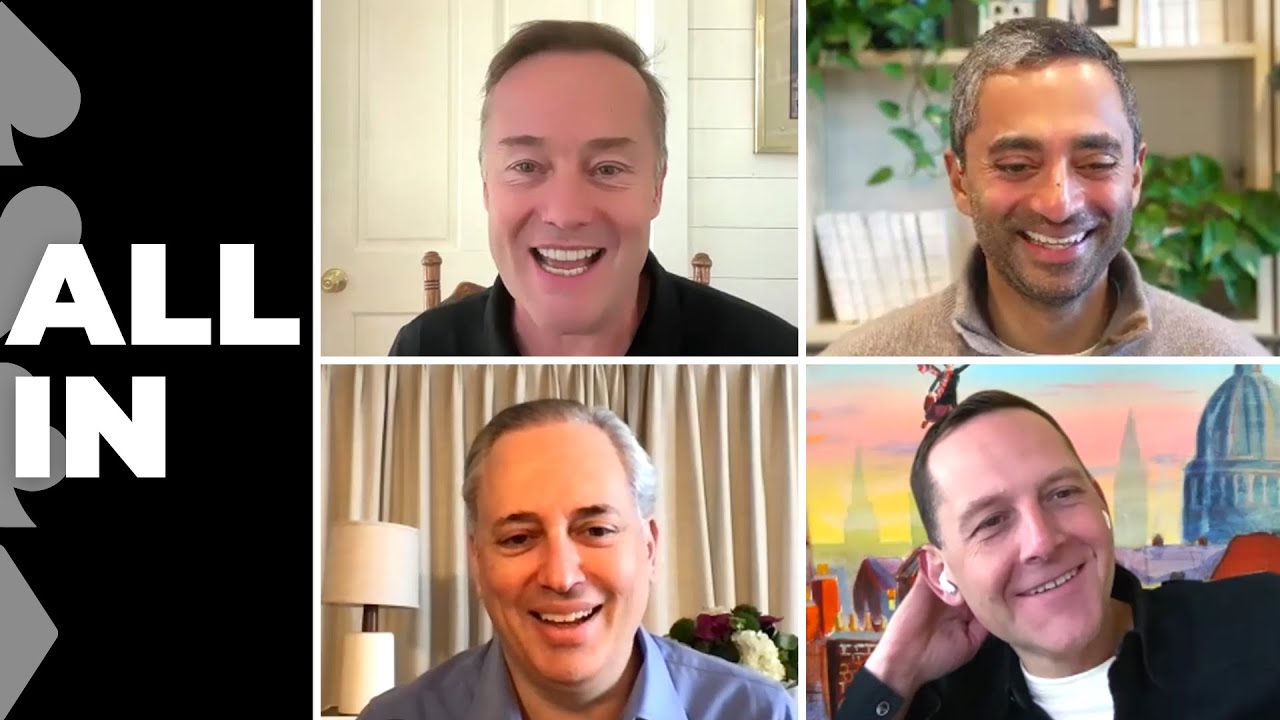
Chapters
0:0 Bestie intros: Banana boat!
2:34 Nvidia smashes expectations again: understanding its terminal value and bull/bear cases in the context of the history of the internet
27:26 Groq's big week, training vs. inference, LPUs vs. GPUs, how to succeed in deep tech
49:37 Google's AI disaster: Is Google too woke to function as search gets disrupted by AI?
77:17 War Corner with Sacks
00:00:00.000 |
All right, everybody, welcome back to your favorite podcast 00:00:02.760 |
of all time, the all in podcast, episode 160 something with me 00:00:07.680 |
again, Chamath Palihapitiya. He's the CEO of a company and 00:00:12.160 |
invest in startups and his firm is called social capital. We 00:00:16.960 |
also have David Freeberg, the sultan of science. He's now a 00:00:20.000 |
CEO as well. And we have David Sachs from craft ventures in 00:00:25.640 |
some undisclosed hotel room somewhere. How are we doing 00:00:28.520 |
boys? Good. Thank you. This is not hard. Your intro be any more 00:00:33.320 |
low energy and dragged out. I'm sick. What do you want me to 00:00:37.600 |
do? Effort. All right, here we go. Give me one more shot. Watch 00:00:41.200 |
this. Watch this watch professional. You want 00:00:43.400 |
professionalism? The effort Come on. Here we go. You want 00:00:46.120 |
professionalism? I'll show you guys professionalism. Is that 00:00:48.560 |
binaka? What was that? That's not good. Oh, it is the secret. 00:00:59.600 |
All right, everybody, welcome to the all in podcast episode 00:01:13.880 |
167 168. With me, of course, the rain man himself, David Sachs, 00:01:18.320 |
the dictator, Chairman Chabot, Polly hoppity, and our sultan of 00:01:22.120 |
science, David Freeberg. How we doing, boys? Great. How are you? 00:01:24.440 |
Is it 167 or 168? No, who cares? We at least get you to know the 00:01:30.040 |
episode number. Who cares? Unfortunately, or fortunately, 00:01:34.120 |
we're going to be doing this thing forever. The audience 00:01:36.240 |
demands it. It doesn't matter. This is like a Twilight Zone 00:01:39.200 |
episode. We're going to be trapped in these four bubbles 00:01:41.800 |
forever. Superman. It's a it is it's this is like the it is the 00:01:46.560 |
gift. glass. Was that? Yeah, Neil before Zod. And he spun 00:01:53.680 |
through the universe and the plastic thing forever for for 00:01:56.720 |
infinity until that until Superman took the nuclear bomb 00:02:00.640 |
out of the Eiffel Tower and threw it into space and blew it 00:02:04.840 |
up. And for you know, my background today, I think I'm 00:02:07.360 |
gonna have to change now that you've referenced this 00:02:09.280 |
important scene. That was the best moment of that movie, J. 00:02:11.760 |
Cal, where Terrence stamps says Neil to the President and the 00:02:16.000 |
President says, Oh, God, yes. And Terrence was Zod. 00:02:25.320 |
Superman two is pretty much the best. Yeah, you know, like 00:02:29.600 |
Empire Strikes Back like Terminator two, it's always the 00:02:32.440 |
second one. That's the best one. All right, everybody, we got a 00:02:34.840 |
lot to talk about today. Apologies for my voice a little 00:02:37.240 |
bit of a cold Nvidia blew the doors off their earnings for the 00:02:40.120 |
third straight quarter. shares were up 15% on Thursday 00:02:44.080 |
representing a nearly $250 billion jump in market cap. So 00:02:49.000 |
let's just let that sit in for a second. This is the largest 00:02:52.800 |
single day gain in market cap. $247 billion added in market 00:02:59.000 |
cap previously meta did something similar earlier this 00:03:02.120 |
year. Remember everybody was down on that stock because they 00:03:04.400 |
were doing all the crazy stuff with reality labs and then they 00:03:07.640 |
got focused and laid off 20,000 people. They added $196 billion. 00:03:11.880 |
In other words, they added like two and a half Airbnbs to their 00:03:15.840 |
valuation. But let's just get to the results. The results are 00:03:18.000 |
absolutely stunning. I dare I say unprecedented Q four revenue 00:03:21.600 |
22.1 billion that's up 22% quarter of a quarter of 265% 00:03:27.520 |
year over year. The net income was 12.3 billion nine x year over 00:03:32.560 |
year, and the gross margin of 76% was up two points quarter of a 00:03:36.680 |
quarter 12.7% year over year. But look at this revenue ramp. 00:03:40.840 |
This is extraordinary. q1 of 2024 this juggernaut starts and 00:03:47.680 |
it does not stop and it doesn't look like it's going to stop 00:03:50.480 |
just to run up from 7 billion all the way to 22 billion in 00:03:54.760 |
revenue for the quarter. Absolutely extraordinary. And if 00:03:59.440 |
you want to know why this is happening, why is Nvidia putting 00:04:02.080 |
up these kind of numbers? This chart explains everything. This 00:04:05.280 |
is all about data centers. Obviously, if you heard of 00:04:08.760 |
Nvidia before the AI boom, it was gaming, professional 00:04:12.440 |
visualizations, you know, I think people making movies and 00:04:14.960 |
stuff like that autos used Nvidia for self driving, that 00:04:18.560 |
kind of stuff. But if you look at this chart, you'll see data 00:04:21.080 |
centers, just starting four quarters ago, starts to ramp up 00:04:26.160 |
as everybody builds out the infrastructure for new data 00:04:32.760 |
So just to add one point here, Jason, so what you can see is 00:04:36.960 |
that Nvidia was around for a long time, and it was making 00:04:40.320 |
these chips, these GPUs, as opposed to CPUs. And they were 00:04:44.720 |
primarily used by games, and by virtual reality software, 00:04:51.040 |
because GPUs are better, obviously, at graphical 00:04:54.080 |
processing, they use vector math to create these like 3d 00:04:57.160 |
worlds. And this vector math that they use to create these 00:05:02.040 |
3d worlds is also the same vector math that AI uses to 00:05:05.920 |
reach its outcomes. So with the explosion of LLM, it turns out 00:05:10.760 |
that these GPUs are the right chips that you need for these 00:05:13.920 |
cloud service providers to build out these big data centers to 00:05:18.040 |
serve now all of these new AI applications. So Nvidia was in 00:05:22.520 |
the perfect place at the perfect time. And that's why it's just 00:05:26.240 |
exploded. And what you're seeing is the build out of this new 00:05:33.720 |
Yeah. And it also helping the stock is the fact that they 00:05:38.360 |
bought back 2.7 billion worth of their shares as part of a $25 00:05:41.280 |
billion buyback plan. But this company is firing on all 00:05:44.520 |
cylinders revenues, obviously ripping as people put in orders 00:05:47.680 |
to replace all of the data centers out there, or at least 00:05:51.520 |
augment them with this technology with GPUs, a 100s, 00:05:55.200 |
H 100s, etc. The gross margins been expanding, they have huge 00:05:59.360 |
profits, and they're still projecting more growth in q1, 00:06:02.960 |
around 24 billion, which would be a 3x increase year over year. 00:06:07.040 |
And this obviously has made the entire market rip. As Nvidia 00:06:11.000 |
goes, so does the market right now. And the s&p 500 and Nasdaq 00:06:15.200 |
are at record highs at the time of this taping Chamath your 00:06:19.560 |
general thoughts here on something I don't think anybody 00:06:23.160 |
saw coming. Except for you and your investment in grok maybe, 00:06:28.080 |
and a couple of others. I think what I would tell you is that 00:06:30.800 |
the bigger principle, and we've talked about this a lot, Jason, 00:06:35.120 |
is that in capitalism, when you over earn for enough of a time, 00:06:41.600 |
what happens is competitors decide to try to compete away 00:06:44.480 |
your earnings. In the absence of a monopoly, the amount of time 00:06:48.360 |
that you have tends to be small, and it shrinks. So in the case 00:06:52.080 |
of a monopoly, for example, take Google, you can over earn for 00:06:55.480 |
decades. And it takes a very, very long time for somebody to 00:06:59.440 |
try to displace you, we're just starting to see the beginnings 00:07:02.520 |
of that, with things like perplexity and other services 00:07:06.280 |
that are chipping away at the Google monopoly. But at some 00:07:10.320 |
point in time, all of these excess profits are competed 00:07:14.240 |
away. In the case of Nvidia, what you're now starting to see 00:07:18.440 |
is them over earn in a very massive way. So the real 00:07:22.520 |
question is who will step up to try to compete away those 00:07:27.200 |
profits. The old Bezos quote, right, your margin is my 00:07:31.680 |
opportunity. And I think we're starting to see and you've 00:07:34.360 |
mentioned grok, who had a super viral moment, I think this week. 00:07:37.800 |
But you're starting to see the emergence of a more detailed 00:07:42.000 |
understanding of what this market actually means. And as a 00:07:45.320 |
result, who will compete away the inference market who will 00:07:49.440 |
compete away the training market, and the economics of 00:07:52.240 |
that are just becoming known to now more and more people. 00:07:54.720 |
Freeburg, your thoughts, we were talking, I think, was last week 00:07:58.400 |
or the week before about possibility of Nvidia being a 00:08:01.680 |
$10 trillion company, largest company in the world, what are 00:08:04.120 |
your thoughts on the spectacular results? And then, to Matt's 00:08:07.680 |
point, everybody is watching this going, Hmm, maybe I can get 00:08:10.920 |
a slice of that pie. And maybe I can create a more competitive 00:08:15.000 |
offering. Obviously, we saw Sam Altman, rumored to be raising 00:08:18.880 |
7 trillion, which feels like a fake number of feels like that's 00:08:21.480 |
maybe the market size or something. But your thoughts, 00:08:23.720 |
don't think anything's changed on the Nvidia front, there's this 00:08:26.160 |
accelerated compute build out underway. In data centers, 00:08:32.200 |
everyone's trying to build applications and tools and 00:08:37.280 |
infrastructure build out is kind of the first phase. The real 00:08:40.200 |
question ultimately will be, does the initial cost of the 00:08:43.320 |
infrastructure exceed the ultimate value that's going to 00:08:46.640 |
be realized on the application layer? In the early days of the 00:08:50.200 |
internet, a lot of people were buying Oracle servers, they were 00:08:55.000 |
like 3000 bucks a server. And they were running these Oracle 00:08:58.960 |
servers out of an internet connected data center. And it, 00:09:02.160 |
you know, took a couple of years before folks realized that for 00:09:05.400 |
large scale distributed compute applications, you're better off 00:09:10.360 |
using cheaper hardware, you know, cheaper server racks, 00:09:13.760 |
cheaper hard drives, cheaper buses, and assuming a shorter 00:09:17.800 |
lifespan on those servers, and you can cycle them in and out. 00:09:21.240 |
And you didn't need the redundancy, you didn't need the 00:09:23.480 |
certainty, you didn't need the runtime guarantees. And so you 00:09:27.240 |
could use a lower cost, higher failure rate, but much, much net 00:09:32.680 |
lower cost kind of approach to building out a data center for 00:09:35.960 |
internet serving. And so the Oracle servers didn't really 00:09:39.360 |
take the market. And early on, everyone thought that they would 00:09:41.960 |
so I think to my point is right now, Nvidia has been at this for 00:09:44.960 |
a very long time. And the real question is how much of an 00:09:48.520 |
advantage do they have, particularly that there is this 00:09:51.480 |
need to use fabs to build replacement technology. So over 00:09:54.960 |
time, will there be better solutions that use hardware 00:09:57.200 |
that's not as good, but the software figures out and they 00:09:59.360 |
build new architecture for running on that hardware in a 00:10:02.000 |
way that kind of mimics what we saw in the early days of the 00:10:04.320 |
build out of the internet. So TBD, right? The same is true in 00:10:09.360 |
switches, right? So in networking, a lot of the high 00:10:12.320 |
end high quality networking companies got beaten up when 00:10:16.520 |
lower cost solutions came to market later. And so they look 00:10:20.040 |
like they were going to be the biggest business ever. I mean, 00:10:21.640 |
you can look at Cisco, during the early days of the internet 00:10:24.400 |
build out, and everyone thought Cisco was the picks and shovels 00:10:27.880 |
of the internet, they were going to make all the all the values 00:10:29.920 |
going to go to Cisco. So we're kind of in that same phase right 00:10:32.960 |
now with Nvidia, the real question is, is this going to be 00:10:36.040 |
a much harder hill to compete on than we've ever seen, given the 00:10:39.880 |
development cycle on chips and the requirement to use these 00:10:42.400 |
fabs to build chips. It may be a harder hill to kind of get up 00:10:45.640 |
sex. So we'll see your thoughts. You think we're getting to the 00:10:48.200 |
point where maybe we'll have bought too many of these built 00:10:51.840 |
out too much infrastructure, and it will take time for the 00:10:54.160 |
application layer as freeberg was alluding to to monetize it? 00:10:58.360 |
Well, I think the question everyone's asking right now is, 00:11:00.680 |
are these results sustainable? Can Nvidia keep growing at these 00:11:05.400 |
astounding rates? You know, will the build out continue and the 00:11:09.200 |
comparison everyone's making is to Cisco. And there's this chart 00:11:12.520 |
that's been going around, overlaying the Nvidia stock 00:11:16.040 |
price on the Cisco stock price. And you can see here, the orange 00:11:19.360 |
line is Nvidia and the blue line is Cisco. And it's almost like a 00:11:24.760 |
perfect match. Now, what happened is that at a similar 00:11:28.600 |
point, in the original build out of the internet of the dot com 00:11:32.800 |
era, you had the market crash at the end of March of 2000. And 00:11:39.080 |
Cisco never really recovered from that peak valuation. But I 00:11:43.080 |
think there's a lot of reasons to believe Nvidia is different. 00:11:45.600 |
One is that if you look at Nvidia is multiples are nowhere 00:11:49.080 |
near where Cisco's were back then. So the market in 1999 and 00:11:53.160 |
early 2000 was way more bubbly than it is now. So Nvidia is 00:11:57.880 |
valuation, it's much more grounded in real revenue, real 00:12:01.680 |
margins, real profit. Second, you have the issue of 00:12:06.600 |
competitive moat. Cisco was selling servers and networking 00:12:11.280 |
equipment. Fundamentally, that equipment was much easier to 00:12:15.280 |
copy and commoditize than GPUs. These GPU chips are really 00:12:20.800 |
complicated. I think Jensen made the point that their hopper 100 00:12:25.800 |
product, he said, you know, don't even think of it just like 00:12:29.600 |
a chip, there's actually 35,000 components in this product, and 00:12:33.280 |
it weighs 70 pounds. This is more like mainframe computer or 00:12:37.160 |
something that's dedicated to process somewhere between a rack 00:12:39.960 |
server and the entire rack. Yeah, it's giant, and it's heavy, 00:12:44.440 |
and it's complex. It does say something here, Chamath, I think 00:12:47.960 |
about how well positioned big tech is in terms of seeing an 00:12:55.240 |
opportunity, and quickly mobilizing to capture that 00:12:59.440 |
opportunity. These servers are being bought by, you know, 00:13:04.120 |
people like Amazon, I'm sure Apple, obviously, Facebook meta. 00:13:07.920 |
I don't know if Google is buying them as well, I would assume so 00:13:11.000 |
Tesla. So everybody's buying these things, and they had tons 00:13:14.960 |
of cash sitting around. It is pretty amazing how nimble the 00:13:17.840 |
industry is. And this opportunity feels like everybody 00:13:21.520 |
is looking at it like mobile and cloud, I have to get mobilized 00:13:26.840 |
You're bringing up an excellent point. And I would like to tie 00:13:30.240 |
it together with Friedberg's point. So at some point, all of 00:13:34.360 |
this spend has to make money, right? Otherwise, you're going 00:13:38.120 |
to look really foolish for having spent 20 and 30 and $40 00:13:40.920 |
billion. So Nick, if you just go back to the to the revenue slide 00:13:45.080 |
of Nvidia, I can try to give you a framing of this at least the 00:13:48.840 |
way that I think about it. So if you look at this, like what 00:13:51.320 |
you're talking about is look, who is going to spend $22.1 00:13:55.480 |
billion? Well, you said it, Jason, it's all a big tech. Why? 00:13:58.960 |
Because they have that money on the balance sheet sitting idle. 00:14:01.720 |
But when you spend $22 billion, their investors are going to 00:14:07.080 |
demand a rate of return on that. And so if you think about what a 00:14:10.160 |
reasonable rate of return is, call it 30 40 50%. And then you 00:14:14.000 |
factor in and that's profit. And then you factor in all of the 00:14:17.800 |
other things that need to support that, that $22 billion 00:14:21.720 |
of spend needs to generate probably $45 billion of revenue. 00:14:26.080 |
And so Jason, the question to your point, and to Friedberg's 00:14:30.000 |
point, the $64,000 question is, who in this last quarter is 00:14:34.840 |
going to make 45 billion on that 22 billion of spend. And again, 00:14:39.480 |
what I would tell you to be really honest about this is that 00:14:42.080 |
what you're seeing is more about big companies, muscling people 00:14:47.400 |
around with their balance sheet, and being able to go to Nvidia 00:14:51.320 |
and say, I will give you committed pre purchases over the 00:14:55.120 |
next three or four quarters. And less about here is a product 00:15:00.000 |
that I'm shipping that actually makes money, which I need 00:15:03.400 |
enormous more compute resources for. It's not the latter. Most 00:15:09.240 |
of the apps, the overwhelming majority of the apps that we're 00:15:12.480 |
seeing in AI today, are toy apps that are run as proofs of 00:15:18.040 |
concept, and demos, and run in a sandbox. It is not production 00:15:23.680 |
code. This is not, we've rebuilt the entire autopilot system for 00:15:30.400 |
the Boeing. And it's now run with agents, and bots and all of 00:15:36.160 |
this training. That's not what's happening. So it is a really 00:15:40.160 |
important question. Today, the demand is clear. It's the big 00:15:43.200 |
guys with huge gobs of money. And by the way, Nvidia is super 00:15:47.160 |
smart to take it because they can now forecast demand for the 00:15:50.280 |
next two or three quarters. I think we still need to see the 00:15:53.800 |
next big thing. And if you look in the past, what the past has 00:15:57.120 |
showed you, it's the big guys don't really invent the new 00:15:59.400 |
things that make a ton of money. It's the new guys, who because 00:16:02.520 |
they don't have a lot of money, and they have to be a little bit 00:16:05.560 |
more industrious, come up with something really authentic and 00:16:09.040 |
new. Yeah, constraint makes for great art. Yeah, we haven't 00:16:11.760 |
seen that yet. So I think the revenue scale will continue for 00:16:15.080 |
like the next two or three years, probably for Nvidia. But 00:16:19.720 |
the real question is, what is the terminal value? And it's the 00:16:22.800 |
same thing that SAC showed in that Cisco slide, people 00:16:26.800 |
ultimately realized that the value was going to go to other 00:16:32.120 |
parts of the stack, the application layer. And as more 00:16:37.040 |
and more money was accrued at the application layer of the 00:16:39.360 |
internet, less and less revenue multiple and credit was given to 00:16:43.280 |
Cisco. And that's nothing against Cisco, because their 00:16:45.520 |
revenue continue to compound. Right. And they did an 00:16:48.760 |
incredible job, but the valuation got so freeberg. If 00:16:52.400 |
we're looking at this chart, the winner of Netflix, the winner of 00:16:56.280 |
the Cisco chart might in fact be somebody like Netflix, they 00:16:58.680 |
actually got, you know, hundreds of millions of consumers to give 00:17:01.920 |
them on Facebook. And then you have Google and Facebook as 00:17:04.440 |
well, generating all that traffic. And then YouTube, of 00:17:07.120 |
course, who do you see the winner here as in terms of the 00:17:10.840 |
application layer? Who are the billion customers here who are 00:17:14.400 |
going to spend 20 bucks a month, five bucks a month, whatever it 00:17:17.800 |
Well, I mean, let me just start with this important point. If 00:17:20.280 |
you look at where that revenue is coming from, to chamat's 00:17:23.360 |
point, it's coming from big cloud service providers. So 00:17:28.680 |
Google, and others are building out clouds, that other 00:17:34.280 |
application developers can build their AI tools and applications 00:17:37.760 |
on top of. So a lot of the build out is in these cloud data 00:17:40.960 |
centers that are owned and operated by these big tech 00:17:45.600 |
companies. The 18 billion of data center revenue that Nvidia 00:17:49.640 |
realized is revenue to them. But it's not an operating expense to 00:17:54.400 |
the companies that are building out. So this is an important 00:17:57.160 |
point on why this is happening at such an accelerated pace. 00:18:00.120 |
When a big company buys these chips from Nvidia, they don't 00:18:04.360 |
have to from an accounting basis market as an expense in their 00:18:07.480 |
income statement, it actually gets booked as a capital 00:18:10.320 |
expenditure. In the cash flow statement, it gets put on the 00:18:14.040 |
balance sheet, and they depreciate it over time. And so 00:18:17.440 |
they can spend $20 billion of cash because Google and others 00:18:20.320 |
have 100 billion of cash sitting on the balance sheet. And 00:18:23.320 |
they've been struggling to find ways to grow their business 00:18:26.000 |
through acquisitions. One of the reasons is they there aren't 00:18:29.840 |
enough companies out there that they can buy it a good multiple 00:18:32.280 |
that can give them a good increase in profit. The other 00:18:35.120 |
one is that antitrust authorities are blocking all of 00:18:37.120 |
their acquisitions. And so what do you do with all that cash? 00:18:39.840 |
Well, you can build out the next gen of cloud infrastructure, and 00:18:43.680 |
you don't have to take the hit on your P&L by doing it. So it 00:18:46.880 |
ends up in the balance sheet, and then you depreciate it over 00:18:49.480 |
typically four to seven years. So that money gets paid out on 00:18:53.320 |
the on the income statement at these big companies over a seven 00:18:56.320 |
year period. So there's a really great accounting and M&A 00:19:01.240 |
environment driver here that's causing the big cloud data 00:19:05.040 |
center providers to step in and say, this is a great time for us 00:19:07.920 |
to build out the next generation of infrastructure that could 00:19:11.400 |
generate profits for us in the future, because we've got all 00:19:13.720 |
this cash sitting around, we don't have to take a P&L hit, we 00:19:16.200 |
don't have to acquire a cash burning business. And, you 00:19:20.000 |
know, frankly, we're not going to be able to grow through M&A 00:19:21.640 |
because of antitrust right now anyway. So there's a lot of 00:19:24.240 |
other motivating factors that are causing this near term 00:19:26.480 |
acceleration, as they're trying to find ways to grow. Yeah. And 00:19:30.040 |
all I know that was an accounting point, but I think 00:19:31.960 |
it's a really important one. If you if 100 billion gets 00:19:34.960 |
spent this year, you're divided by 425 billion in revenue would 00:19:38.040 |
have to come from that or something in that range. Yeah. 00:19:42.120 |
Jeff, to just keep in mind, I think Fribourg, what you said is 00:19:44.520 |
very true for GCP spend, but not necessarily for Google spend. 00:19:49.000 |
It's true for AWS spend, but not necessarily for Amazon spend. 00:19:53.040 |
And it's true for Azure spend, not true for Microsoft spend. 00:19:56.680 |
And it's largely not true for Tesla and Facebook because they 00:19:59.600 |
don't have clouds. So I think the question to your point, that 00:20:03.600 |
have been, for obvious reasons, Nvidia doesn't disclose it is 00:20:07.120 |
what is the percentage of that 21 billion that just went to 00:20:10.600 |
those cloud providers that they'll then capitalized to to 00:20:14.600 |
everybody else versus what was just absorbed because at 00:20:17.400 |
Facebook, Mark had that video about how many h 100. That's all 00:20:20.600 |
Right, but it is still it is still capitalized, is my point. 00:20:24.600 |
So they don't have to book that as an expense. It sits on the 00:20:28.080 |
balance sheet. Yeah, sure. And they earn it down over time. 00:20:30.760 |
You're helping to explain why these big cloud service 00:20:34.560 |
because they're very profitable, and there's nowhere else to put 00:20:37.760 |
Right? Well, so that would seem to indicate that this is more in 00:20:41.960 |
the category of one time build out than sustainable ongoing 00:20:45.800 |
revenue. I think the big question is the one that mouth 00:20:49.200 |
asked, which is, what's the terminal value of Nvidia? I 00:20:52.360 |
think, like a simple framework for thinking about that is what 00:20:56.040 |
is the total addressable market or tam related to GPUs? And then 00:21:00.280 |
what is their market share going to be? Right now, their market 00:21:04.000 |
share is something like 91%. That's clearly going to come 00:21:06.640 |
down, but their moat appears to be substantial. The Wall Street 00:21:10.600 |
analysts I've been listening to think that in five years, 00:21:13.640 |
they're still going to have 60 something percent market share. 00:21:16.400 |
So they're going to have a substantial percentage of this 00:21:19.320 |
market or this Tam, then the question is, I think, with 00:21:22.520 |
respect to Tam is, what is one time build out versus steady 00:21:26.680 |
state? Now, I think that clearly, there's a lot of build 00:21:32.320 |
out happening now, that's almost like a backfill of capacity that 00:21:35.320 |
people are realizing they need. But even the numbers you're 00:21:38.360 |
seeing this quarter, kind of understated, because, first of 00:21:41.960 |
all, Nvidia was supply constrained, they cannot produce 00:21:45.000 |
enough chips to satisfy all the demand, their revenue would 00:21:48.360 |
would have been even higher, if they had more capacity. Second, 00:21:54.120 |
you just look at their forecast. So the fiscal year that just 00:21:57.400 |
ended, they did around $60 billion of revenue, they're 00:22:00.440 |
forecasting $110 billion for the fiscal year that just started. 00:22:03.920 |
So they're already projecting to almost double based on the 00:22:07.680 |
demand that they clearly have visibility into already. So it's 00:22:11.440 |
very hard to know exactly what the terminal or steady state 00:22:15.000 |
value of this market is going to be. Even once the cloud service 00:22:19.920 |
providers do this big build out, presumably, there's always going 00:22:22.760 |
to be a need to stay up to date with the latest chips, right? 00:22:26.720 |
Here's a framework for you, Sax, tell me if this makes sense. 00:22:29.600 |
Intel was the basically the mother of all of modern compute 00:22:35.360 |
up until today, right? I think the CPU was the the most 00:22:40.120 |
fundamental workhorse that enabled local PCs, it enabled 00:22:44.240 |
networking, it enabled the internet. And so when you look 00:22:49.320 |
at the market cap of it, as an example, that's about 180 odd 00:22:53.720 |
billion dollars today. The economy that it created, that it 00:22:59.800 |
supports is probably measured, call it in a trillion or $2 00:23:03.360 |
trillion, maybe 5 trillion, let's just be really generous, 00:23:06.360 |
right. And so you can see that there's this ratio of the 00:23:10.320 |
enabler of an economy, and the size of the economy. And those 00:23:15.480 |
things tend to be relatively fixed, and they recur 00:23:18.240 |
repeatedly over and over and over. If you look at Microsoft, 00:23:21.200 |
its market cap relative to the economy that it enables. So the 00:23:24.840 |
question for Nvidia, in my mind would be not that it is it not 00:23:28.800 |
going to go up in the next 18 to 24 months probably is, for 00:23:32.440 |
exactly the reason you said it is super set up to have a very 00:23:35.600 |
good meat and beet guidance for the street, which they'll eat 00:23:38.840 |
up and all of the algorithms that trade the press releases 00:23:42.160 |
will drive the price higher and all of this stuff will just 00:23:44.760 |
create a trend upward. I think the bigger question is, if it's 00:23:49.640 |
a four or $5 trillion market cap in the next two or three years, 00:23:54.720 |
will it support $100 trillion economy? Because that's what you 00:24:01.480 |
would need to believe for those ratios to hold. Otherwise, 00:24:05.840 |
Yeah, I mean, so the history of the internet is that if you 00:24:09.400 |
build it, they will come, meaning that if you make the 00:24:12.200 |
investment in the capital assets necessary to power the next 00:24:16.800 |
generation of applications, those applications have always 00:24:19.400 |
eventually gotten written, even though it was hard to predict 00:24:23.120 |
them at the time. So in the late 90s, when we had the whole dot 00:24:26.200 |
com bubble, and then bust, you had this tremendous build out 00:24:29.200 |
not just of kind of servers and all the networking equipment, 00:24:32.160 |
but there was a huge fiber build out. Yep, by all the telecom 00:24:35.120 |
companies, and the telecom companies had a Cisco like, you 00:24:40.480 |
You know, all common them, or they went bankrupt a lot of 00:24:42.680 |
them. Yeah, well, the problem there was that a lot of the 00:24:45.520 |
buildout happened with debt. And so when you had the dot com 00:24:48.160 |
crash, and all the valuations came down to earth, that's why a 00:24:51.880 |
lot of them went under. Yeah, Cisco wasn't in that position. 00:24:54.920 |
But anyway, my point is, in the early 2000s, when the dot com 00:24:58.600 |
crash happened, everyone thought that these telecom companies had 00:25:01.480 |
over invested in fiber. As it turns out, all that fiber 00:25:04.800 |
eventually got used. The internet went from, you know, 00:25:09.040 |
dial up to broadband, we started doing seeing streaming, social 00:25:12.720 |
networking, all these applications started eating up 00:25:15.160 |
that bandwidth. So I think that the history of these things is 00:25:19.920 |
that the applications eventually get written, they get developed 00:25:24.520 |
if you build the infrastructure to power them. And I think with 00:25:27.000 |
AI, the thing that's exciting to me as someone who's really more 00:25:30.640 |
of an application investor, is that we're just at the 00:25:33.200 |
beginning, I think of a huge wave of a lot of new creativity 00:25:39.240 |
and applications that's going to be written. And it's not just 00:25:41.760 |
B2C, it's going to be B2B as well. You guys haven't really 00:25:44.520 |
mentioned that it's not just consumers and consumer 00:25:47.280 |
applications are going to use these cloud data centers that 00:25:51.720 |
are buying up all these GPUs is it's going to be enterprises 00:25:54.240 |
too. I mean, these enterprises are using Azure, they're using 00:25:57.600 |
Google Cloud, and so forth. So there's a lot I think, that's 00:26:02.600 |
still to come. I mean, we're just at the beginning of a wave 00:26:05.320 |
that's probably going to last at least a decade. 00:26:07.520 |
Yeah. And to your point, one of the reasons YouTube, Google 00:26:12.040 |
Photos, iPhoto, a lot of these things happened was because the 00:26:17.200 |
infrastructure build out was so great during the dotcom boom, 00:26:20.160 |
that the prices for storage, the prices for bandwidth sacks, 00:26:23.360 |
plummeted. And then people like Chad Hurley, looked at him like, 00:26:27.480 |
you know what, instead of charging people to put a video 00:26:30.160 |
on the internet, and then charging them for the bandwidth 00:26:32.280 |
they used, we'll just let them upload this stuff to YouTube, 00:26:35.080 |
and we'll figure it out later. Same thing with Netflix. 00:26:37.680 |
I mean, look, when we were developing PayPal in the late 00:26:40.640 |
90s, really around 1999, you could barely upload a photo to 00:26:46.320 |
the internet. I mean, so like the idea of having an account 00:26:49.200 |
with a profile photo on it was sort of like, why would you do 00:26:51.560 |
that? It's just prohibitively slow, everyone's going to drop 00:26:53.960 |
off. Yeah, by 2003, it was fast enough that you could do that. 00:26:59.040 |
And that's why social networking happened. I mean, literally, 00:27:01.400 |
without that performance improvement, like even having a 00:27:06.680 |
profile photo on your account was something that was too hard 00:27:09.360 |
to do. LinkedIn profile was like too much bandwidth. And then let 00:27:12.640 |
alone video. I mean, the you would get you probably remember 00:27:16.560 |
these days, you would put up a video on your website. If it 00:27:19.680 |
went viral, your website got turned off, because you would 00:27:22.880 |
hit your 5000 or $10,000 a month. Cap. All right. Grok also 00:27:27.440 |
had a huge week. That's Grok with a Q, not to be confused 00:27:30.960 |
with the lawns, Grok with a K. Chamath, you've talked about 00:27:34.720 |
Grok on this podcast a couple of times, obviously, you were the I 00:27:38.960 |
guess you were the first investor, the seed investor, you 00:27:41.480 |
pulled up these LPUs and this concept out of a team that was 00:27:45.000 |
at Google. Maybe you could explain a little bit about 00:27:48.120 |
Crocs viral moment this week in the history of the company, 00:27:50.960 |
which I know, has been a long road for you with this company. 00:27:55.000 |
I mean, it's been since 2016. So again, proving what you guys 00:28:00.560 |
have said many times and what I've tried to live out, which is 00:28:03.560 |
just you just got to keep grinding. 90% of the battle is 00:28:07.560 |
just staying alive in business. Yeah. And having oxygen to keep 00:28:12.800 |
trying things. And then eventually, if you get lucky, 00:28:15.400 |
which I think we did, things can really break in your favor. So 00:28:19.840 |
this weekend, you know, I've been tweeting out a lot of 00:28:22.920 |
technical information about why I think this is such a big deal. 00:28:25.640 |
But yeah, the the moment came this weekend combination of 00:28:28.920 |
hacker news and some other places. And essentially, we had 00:28:32.080 |
no customers two months ago, I'll just be honest. And between 00:28:35.680 |
Sunday and Tuesday, we've just were overwhelmed. And I think 00:28:41.760 |
like the last count was we had 3000 unique customers come and 00:28:45.040 |
try to consume our resources from every important fortune 00:28:50.240 |
500, all the way down to developers. And so I think we're 00:28:54.880 |
very fortunate. I think the team has a lot of hard work to do. So 00:28:57.760 |
it could mean nothing, but it has the potential to be 00:29:00.480 |
something very disruptive. So what is it that people are 00:29:02.920 |
glomming on to? You have to understand that, like at the 00:29:07.280 |
very highest level of AI, you have to view it as two distinct 00:29:11.400 |
problems. One problem is called training, which is where you 00:29:15.440 |
take a model, and you take all of the data that you think will 00:29:19.160 |
help train it. And you do that, you train the model, you learn 00:29:23.320 |
all over all of this information. But the second part 00:29:27.920 |
of the AI problem is what's called inference, which is what 00:29:30.880 |
you and I see every day as a consumer. So we go to a website, 00:29:33.760 |
like chat GPT, or Gemini, we ask a question, and it gives us a 00:29:38.600 |
really useful answer. And those are two very different kinds of 00:29:42.640 |
compute challenges. The first one is about brute force, and 00:29:47.640 |
power, right? If you can imagine, like, what you need are 00:29:51.480 |
tons and tons of machines, tons and tons of like very high 00:29:55.160 |
quality networking, and an enormous amount of power in a 00:29:58.880 |
data center so that you can just run those things for months, I 00:30:01.240 |
think Elon publishes very transparently, for example, how 00:30:04.240 |
long it trains to, to train his grok with a K, right model, and 00:30:08.720 |
it's in the months, inference is something very different, which 00:30:11.520 |
is all about speed and cost, what you need to be in order to 00:30:15.000 |
answer a question for a consumer in a compelling way, is super, 00:30:18.440 |
super cheap, and super, super fast. And we've talked about why 00:30:22.400 |
that is important. And the grok with a queue chips turns out to 00:30:29.280 |
be extremely fast and extremely cheap. And so look, time will 00:30:34.680 |
tell how big this company can get. But if you tie it together 00:30:38.760 |
with what Jensen said on the earnings call, and you now see 00:30:42.920 |
developers stress testing us and finding that we are meaningfully 00:30:48.400 |
meaningfully faster and cheaper than any Nvidia solution, 00:30:51.480 |
there's the potential here to be really disruptive. And we're a 00:30:56.200 |
meager unicorn, right? Our last valuation was like a billion 00:31:00.720 |
something versus Nvidia, which is now like a $2 trillion 00:31:05.080 |
company. So there's a lot of market cap for grok to gain by 00:31:08.400 |
just being able to produce these things at scale. Which could be 00:31:13.760 |
just an enormous outcome for us. So time will tell but a really 00:31:16.960 |
important moment in the company and very exciting. 00:31:19.440 |
Can I just observe like off topic how an overnight success 00:31:25.120 |
No, I was thinking the same line. It's a seven year 00:31:29.800 |
There's this class of businesses that I think are unappreciated 00:31:34.720 |
in a post internet era, where you have to do a bunch of things 00:31:40.520 |
right, before you can get any one thing to work. And these 00:31:45.520 |
complicated businesses where you have to stack either different 00:31:49.320 |
things together that need to click together in a in a stack, 00:31:52.320 |
or you need to iterate on each step until the whole system 00:31:56.160 |
works end to end, can sometimes take a very long time to build 00:32:00.320 |
and the term that's often used for these types of businesses is 00:32:03.400 |
deep tech. And they fall out of favor. Because in an internet 00:32:07.160 |
era, and in a software era, you can find product market fit and 00:32:10.880 |
make revenue and then make profit very quickly. And so a 00:32:14.000 |
lot of entrepreneurs select into that type of business, instead 00:32:17.640 |
of selecting into this type of business, where the probability 00:32:20.880 |
of failure is very high, you have several low probability 00:32:23.960 |
things that you have to get right in a row. And if you do, 00:32:27.280 |
it's going to take eight years and a lot of money. And then all 00:32:30.000 |
of a sudden, the thing takes off like a rocket ship, you've got a 00:32:32.240 |
huge advantage, you've got a huge moat, it's hard for anyone 00:32:35.160 |
to catch up. And this thing can really spin out on its own. I do 00:32:38.880 |
think Elon is very unique in his ability to deliver success in 00:32:42.920 |
these types of businesses, Tesla needed to get a lot of things 00:32:45.280 |
right in a row, SpaceX needed to get a lot of things right in a 00:32:47.880 |
row. All of these require a series of complicated steps, or 00:32:52.080 |
a set of complicated technologies that need to click 00:32:54.360 |
together and work together. But the hardest things often output 00:32:58.720 |
the highest value. And you know, if you can actually make the 00:33:03.680 |
commitment on these types of businesses and get all the 00:33:06.560 |
pieces to click together, there's an extraordinary 00:33:09.160 |
opportunity to build moats and to take huge amounts of market 00:33:12.280 |
value. And I think that there's an element of this that's been 00:33:15.840 |
lost in Silicon Valley over the last couple of decades, as the 00:33:19.800 |
fast money in the internet era has kind of prioritize other 00:33:23.920 |
investments ahead of this. But I'm really hopeful that these 00:33:26.360 |
sorts of chip technologies, SpaceX, in biotech, we see a lot 00:33:31.000 |
of this, these sorts of things can kind of become more in 00:33:33.800 |
favor, because the advantage of these businesses work seems to 00:33:37.800 |
realize hundreds of billions and sometimes trillions of dollars 00:33:40.400 |
of market value, and be incredibly transformative for 00:33:43.640 |
humanity. So I don't know, I just think it's an observation 00:33:45.880 |
I wanted to make about the greatness of these businesses 00:33:48.760 |
Well, I mean, open AI was kind of like that for a while. 00:33:51.360 |
Totally. I mean, it was this like wacky nonprofit that was 00:33:54.280 |
just grinding on an AI research problem for like six years. And 00:33:57.000 |
then it finally worked and got productized into chat GPT. 00:34:01.040 |
Totally. But you're right, SpaceX was kind of like that. I 00:34:03.760 |
mean, the big moneymaker at SpaceX is Starlink, which is the 00:34:08.560 |
satellite networks, basically broadband from space. And it's 00:34:12.400 |
on its way to handling, I think, a meaningful percentage of all 00:34:15.160 |
internet traffic. But think about all the things you had to 00:34:17.680 |
get to to get that working. First, you had to create a 00:34:20.880 |
rocket, that's hard enough. Then you had to get to reusability. 00:34:24.160 |
Then you had to create the whole satellite network. So at least 00:34:29.000 |
Well, and then you have to get consumers to adopt it. I mean, 00:34:33.320 |
Yeah, we had no idea where the market was, like early on, it 00:34:37.120 |
started in my office. And so Jonathan and I would be kind of 00:34:40.120 |
always trying to figure out what is the initial go to market. And 00:34:44.160 |
I remember I emailed Ilan in at that period, when they were 00:34:48.280 |
still trying to figure out whether they were going to go 00:34:50.560 |
with LiDAR or not. And we thought, wow, maybe we could 00:34:53.600 |
sell Tesla the chips, you know, but and then Tesla brought in 00:34:56.880 |
this team just to talk to us about what the design goals 00:34:59.720 |
were. And basically said no, in kind way, but they said no. Then 00:35:04.920 |
we thought, okay, maybe it's like for high frequency traders, 00:35:07.520 |
right? Because like those folks want to have all kinds of edges. 00:35:10.520 |
And if we have these big models, maybe we can accelerate their 00:35:14.400 |
decision making, they can measure revenue, that didn't 00:35:17.160 |
work out. Then it was like, you know, we tried to sell to three 00:35:21.520 |
letter agencies, that didn't really work out. Our original 00:35:24.520 |
version was really focused on image classification and 00:35:27.600 |
convolutional neural nets, like resonant, that didn't work out. 00:35:31.800 |
We ran headfirst into the fact that NVIDIA has this compiler 00:35:36.040 |
product called CUDA. And we had to build a high class compiler 00:35:40.040 |
that you could take any model without any modifications. All 00:35:44.720 |
these things to your point are just points where you can just 00:35:47.200 |
very easily give up. And then there's like, we run out of 00:35:49.440 |
money. So then you write money in a note, right? Because 00:35:52.440 |
everybody wants to punt on valuation when nothing's 00:35:55.360 |
You tried six beachhead market, you can land the boat, right? 00:35:59.360 |
You have to make a decision to just keep going if you believe 00:36:03.600 |
it's right. And if you believe you are right. Yeah. And that 00:36:07.280 |
requires shutting out. We talked about this in the masa example 00:36:11.600 |
last week, but it just requires shutting out the noise because 00:36:14.320 |
it's so hard to believe in yourself. It's so hard to keep 00:36:19.080 |
funding these things. It's so hard to go into pardon meetings 00:36:21.480 |
and defend a company. And then you just have a moment and you 00:36:25.720 |
just feel I don't know, I feel very vindicated. But then I feel 00:36:30.040 |
very scared because Jonathan still hasn't landed it. You know 00:36:33.000 |
You mentioned all those boats landing and trying to trying to 00:36:35.360 |
those missteps, but 3000 people signed up. Who are they? Are 00:36:39.280 |
they developers now? And they're going to figure out the 00:36:41.520 |
Yeah, I think that back to the original point, my thought today 00:36:44.520 |
is that AI is more about proofs of concept, and toy apps, and 00:36:49.400 |
nothing real. Yeah, I don't think there's anything real 00:36:52.120 |
that's inside of an enterprise that is so meaningfully 00:36:55.360 |
disruptive, that it's going to get broadly licensed to other 00:36:58.240 |
enterprises. I'm not saying we won't get there. But I'm saying 00:37:01.360 |
we haven't yet seen that Cambrian moment of monetization. 00:37:05.840 |
We've seen the Cambrian moment of innovation. Yeah. And so that 00:37:10.520 |
gap has still yet to be crossed. And I think the reason that you 00:37:14.200 |
can't cross it is that today, these are in an unusable state, 00:37:18.480 |
the results are not good enough. They are toy apps that are too 00:37:22.400 |
slow, that require too much infrastructure and cost. So the 00:37:28.080 |
potential is for us to enable that monetization leap forward. 00:37:31.920 |
And so yeah, they're going to be developers of all sizes. And the 00:37:35.840 |
people that came are literally companies of all sizes, I saw 00:37:39.720 |
some of the names of the big companies, and they are the 00:37:45.280 |
How do you guys reconcile this deep tech, high outcome 00:37:51.000 |
opportunity that everyone here has seen and been a part of as 00:37:54.440 |
an investor, participant in versus the more de risked faster 00:38:00.520 |
time to market? And, you know, Chamath, in particular, like in 00:38:04.080 |
the past, we've talked about some of these deep tech 00:38:05.760 |
projects like fusion and so on. And you've highlighted what's 00:38:08.720 |
just not there yet. It's not fundable. What's the distinction 00:38:11.720 |
between a deep tech investment opportunity that is fundable, 00:38:15.280 |
and that you keep grinding at that has this huge outcome? What 00:38:19.680 |
makes the one like fusion that's not fun? It's a phenomenal 00:38:23.000 |
question. Great question. My answer is I have a very simple 00:38:26.360 |
filter, which is that I don't want to debate the laws of 00:38:29.840 |
physics when I fund a company. So with Jonathan, when we were 00:38:35.360 |
initially trying to figure out how to size it, I think my 00:38:38.120 |
initial check was like seven to $10 million or something. And 00:38:41.840 |
the whole goal was to get to an initial tape out of a design. We 00:38:45.480 |
were not inventing anything new with respect to physics. We were 00:38:49.280 |
on a very old process technology, I think we're still 00:38:51.480 |
on 14 nanometer, we were on 14 nanometer eight years ago. Okay. 00:38:55.000 |
So we weren't pushing those boundaries. All we were doing 00:38:58.680 |
was trying to build a compiler and a chip that made sense in a 00:39:01.080 |
very specific construct to solve a well defined bounded problem. 00:39:05.040 |
So that is a technical challenge, but it's not one of 00:39:08.400 |
physics. When I've been pitched all the fusion companies, for 00:39:12.440 |
example, there are fuel sources that require you to make a leap 00:39:16.520 |
of physics, where in order to generate a certain fuel source, 00:39:20.440 |
you either have to go and harvest that on the moon or in a 00:39:23.400 |
different planet that is not Earth, or you have to create 00:39:26.400 |
some fundamentally different way of creating this highly unique 00:39:29.440 |
material. That is why those kinds of problems to me are poor 00:39:33.960 |
risk. And building a chip is good risk. It doesn't mean 00:39:37.760 |
you're going to be successful in building a chip. But the risks 00:39:42.320 |
are bounded to not have fundamental physics, they're 00:39:45.120 |
bounded to go to market and technical usefulness. And I 00:39:48.520 |
think that that removes an order of magnitude risk in the 00:39:52.720 |
outcome. So I mean, there's still like a bunch of things 00:39:55.520 |
that have to be right in a row to make it work. But it doesn't 00:39:58.040 |
mean it's gonna work. Yeah, all I'm saying is, I don't I don't 00:40:00.120 |
want it to fail, because we built a reactor and we realize 00:40:02.560 |
hold on, to get heavy hydrogen, I got to go to the moon. Right. 00:40:05.920 |
And Jay Cowan sacks. How do you sacks? I know you don't, you 00:40:08.880 |
invest in a couple. So yeah, so maybe you guys can highlight how 00:40:12.320 |
you thought about deep tech opportunities versus probably do 00:40:15.680 |
something really difficult like this every 50 investments or so. 00:40:19.240 |
Because most of the entrepreneurs coming to us 00:40:21.920 |
because we're seed investors or pre seed investors, they would 00:40:24.960 |
be going to a biotech investor or a hardware investor who 00:40:27.760 |
specializes in that not to us. But once in a while, we meet a 00:40:30.080 |
founder we really like. And so Contra line was one, we were 00:40:34.360 |
introduced to somebody who's doing this really interesting 00:40:37.520 |
contraception for men, where they put a gel into your vas 00:40:41.280 |
deference. And you as a man can take control of your 00:40:46.200 |
reproduction, you basically it's a it's not a vasectomy, it's 00:40:50.480 |
just a gel that goes in there and blocks it. And this company 00:40:53.320 |
is now doing human trials and doing fantastic. But this took 00:40:56.320 |
forever to get to this point. And then you guys, some of you 00:41:00.760 |
are also investors in cafe x, which we love the founder and 00:41:04.160 |
this company should have died like during COVID and making a 00:41:08.160 |
robotic coffee bar when he started, you know, seven, eight 00:41:11.200 |
years ago, was incredibly hard. He had to build the hardware, he 00:41:15.120 |
had to build a brand, he had to do locations, he had to do 00:41:17.120 |
software. And now he's selling these machines, and people are 00:41:20.240 |
buying them. And the two in San Francisco at SFO are making 00:41:24.160 |
like, I think they two of them make $1 million a year. And it's 00:41:27.560 |
the highest per square footage of any store in an airport. And 00:41:31.960 |
so we've just been grinding and grinding. And you got to find a 00:41:35.360 |
founder who's willing to make it their lives work in these 00:41:38.000 |
kinds of situations. But you start to think about the degree 00:41:40.320 |
of difficulty, hardware, software, reach, mobile apps, I 00:41:46.440 |
mean, it just gets crazy how hard these businesses are, as 00:41:50.120 |
opposed to I'm building a SaaS company. I build software, I sell 00:41:53.760 |
it to somebody to solve their SAS problem. It's like, it's very 00:41:55.840 |
one dimensional, right? It's pretty straightforward. These 00:42:00.720 |
Yeah. And sex, you've been an investor in SpaceX. But you don't 00:42:05.040 |
make those sorts of investments regularly. That craft. Is that 00:42:10.640 |
portfolio allocation, we say this much early stage, this much 00:42:24.080 |
Elon. I mean, you have to be so dogged to want to take something 00:42:29.640 |
like this on because the good stuff happens. Like you're 00:42:31.400 |
saying freeberg, you're 789 10, as opposed to like a consumer 00:42:34.840 |
product. I mean, there were a dozen by year three or four. The 00:42:37.880 |
only app that took a really long time, people don't know this, 00:42:40.040 |
but Twitter actually took a long time to catch on. It was kind of 00:42:43.920 |
cruising for two or three years. And then South by Southwest 00:42:46.520 |
happened. Ashton Kutcher got on it. Obama got on it. 00:42:50.080 |
I think the network effect. I think I think network effect 00:42:52.680 |
businesses are different, because that's all about getting 00:42:54.680 |
your seat of your network. What I'm talking about is the 00:42:57.000 |
technical coordination of lots of technically difficult tasks 00:43:01.240 |
that need to sync up. It's like getting a master lock with like 00:43:04.560 |
10 digits. And you got to figure out the combination of all 10 00:43:07.400 |
digits. And once they're all correct, then the lock opens. 00:43:10.800 |
And prior to that, if any, if any one number is off, the lock 00:43:13.760 |
doesn't open. And I think these technically difficult 00:43:16.000 |
businesses are some of the and they are the hardest and they do 00:43:20.120 |
require the most dogged personalities to persist and to 00:43:23.640 |
realize an outcome from but the truth is that if you get them, 00:43:26.520 |
the moat is extraordinary. And they're usually going to create 00:43:29.200 |
extraordinary leverage and value. And you know, I think 00:43:32.200 |
from a portfolio allocation perspective, if you as an 00:43:35.280 |
investor want to have some diversification in your 00:43:37.400 |
portfolio, this is not going to be the predominance of your 00:43:39.960 |
portfolio, but some percentage of your portfolio should go to 00:43:43.200 |
this sort of business because if it works, boom, you know, this 00:43:46.080 |
can be the big 10 x 100 x 1000 x two stories about that. One of 00:43:49.720 |
the V's early VCs and Elon's told the story publicly wanted 00:43:54.320 |
Elon to not make the roadster not make the model s just make 00:43:57.880 |
drive trains and the electric components for other car 00:44:00.360 |
companies. Can you imagine how the world would have changed? 00:44:02.480 |
And then totally very high profile VC came to me and said, 00:44:07.280 |
Okay, I'll do the series a for other the series a for Uber. I'll 00:44:13.600 |
preemptively do it. But you got to tell Travis to stop running 00:44:16.560 |
Uber as a consumer app. I want them to sell the software to cab 00:44:19.600 |
companies. So make it a SaaS company. I said, Well, you know, 00:44:24.120 |
the cab companies are kind of the problem. Like, they're 00:44:26.360 |
taking all the margin like the kind of disrupting them. And 00:44:30.640 |
they're like, Yeah, but just think there's 1000s of cab 00:44:32.640 |
companies, they would pay you 10s of 1000s of dollars a year 00:44:34.720 |
for this software, and you can get a little piece of the 00:44:36.600 |
action. I never brought that investor to Travis. I was like, 00:44:40.640 |
Oh, wow, that's really interesting insight. Sometimes 00:44:44.200 |
I have a very poor track record of working with other investors. 00:44:48.880 |
Whoa, self reflection. I do deals myself. I size them 00:44:53.360 |
myself. And it's because a lot of them have to live within the 00:45:00.400 |
political dynamics of their fund. And so I think Jason, what 00:45:04.040 |
you're probably saw in that example, which is exactly why 00:45:06.960 |
doing things and splitting deals will never generate great 00:45:10.520 |
outcomes, in my opinion, is that you you take on all the baggage 00:45:14.720 |
and the dysfunction of these other partnerships. And so if 00:45:18.240 |
you really wanted to go and disrupt transportation, you need 00:45:24.160 |
one person who can be a trigger puller and who doesn't have to 00:45:27.040 |
answer to anybody I find. That's why I think for example, when 00:45:30.120 |
you look at how successful Vinod has been over decade after 00:45:34.280 |
decade after decade, when Vinod decides that's the decision. And 00:45:38.840 |
I think there's something very powerful in that. There are a 00:45:42.280 |
bunch of deals that I've done, that when they've worked out, 00:45:47.240 |
were not really because they were consensus, and they had to 00:45:50.280 |
get supported and scaffolded at periods where if I wasn't able 00:45:54.400 |
to ram them through myself, because it was my organization, 00:45:57.280 |
I think we would be in a very different place. So I think I 00:46:01.000 |
think like for for entrepreneurs, it's so difficult 00:46:04.520 |
for them to find people that believe it's so much better to 00:46:07.360 |
find one person and just get enough money and then not 00:46:12.040 |
syndicate, because I think you have to realize that you are 00:46:14.960 |
bringing on and compounding your risk, the one that freeberg 00:46:18.880 |
talked about, with the risk of all the other partnership 00:46:21.640 |
dynamics that you bring on. So if you don't internalize that, 00:46:25.600 |
you may have five or six folks that come into an A or B, but 00:46:29.600 |
you're inheriting five or six. Yeah. Partnership dysfunctions. 00:46:33.680 |
Yeah. Yeah. Can you just explain really quickly for the audience 00:46:37.520 |
since they heard about GPUs and Nvidia, but they may not know 00:46:43.520 |
The GPU, the best way to think about it is so if you contrast a 00:46:48.080 |
CPU with a GPU, so CPU was the workhorse of all of computing. 00:46:52.880 |
And when it when Jensen started Nvidia, what he realized was 00:46:58.120 |
there were specific tasks where a CPU failed quite brilliantly 00:47:02.800 |
at. And so he's like, well, we're gonna make a chip that 00:47:05.960 |
works in all these failure modes for a CPU. So a CPU is very good 00:47:09.320 |
at taking one instruction in, acting on it, and then spitting 00:47:12.720 |
out one, one answer effectively. And so it's a very serial kind 00:47:17.560 |
of a factory if you think about the CPU. So if you want to build 00:47:21.280 |
a factory that can process, instead of one thing at a time, 00:47:24.680 |
10 things or 100 things, what is they had to find a workload that 00:47:30.200 |
was well suited, and they found graphics. And what they convinced 00:47:34.600 |
PC manufacturers back in the day was look, have the CPU be the 00:47:38.400 |
brain, it'll do 90% of the work. But for very specific use cases 00:47:43.160 |
like graphics and video games, you don't want to do serial 00:47:46.560 |
computation, you want to do parallel computation, and we are 00:47:49.440 |
the best at that. And it turned out that that was a genius 00:47:52.400 |
insight. And so the business for many years was gaming and 00:47:56.040 |
graphics. But what happened about 10 years ago was what we 00:48:01.040 |
also started to realize was the math that's required and the 00:48:06.640 |
processing that's required in AI models actually looked very 00:48:11.360 |
similar to how you would process imagery from a game. And so he 00:48:16.920 |
was allowed to figure out by building this thing called CUDA, 00:48:21.360 |
which is the compiler that sits on the chip, how he could now go 00:48:25.040 |
and tell people that wanted to experiment with AI, hey, you 00:48:27.760 |
know, that chip that we had made for graphics, guess what it also 00:48:30.800 |
is amazing at doing all of these very small mathematical 00:48:34.520 |
calculations that you need for your AI model. And that turned 00:48:37.880 |
out to be true. So the next leap forward was what Jonathan saw, 00:48:42.840 |
which was Hold on a second, if you look at the chip itself, 00:48:45.360 |
that GPU substantially has not changed since 1999. In the way 00:48:51.920 |
that it thinks about problem solving, it has all this very 00:48:54.960 |
expensive memory, blah, blah, blah. So he was like, let's just 00:48:58.120 |
throw all that out the window. We'll make small little brains, 00:49:01.800 |
and we'll connect those little brains together. And we'll have 00:49:04.680 |
this very clever software that schedules it and optimizes it. 00:49:07.680 |
So basically, take the chip and make it much, much smaller and 00:49:11.080 |
cheaper, and then make many of them and connect them together. 00:49:14.040 |
That was Jonathan's insight. And it turns out, for large language 00:49:18.280 |
models, that's a huge stroke of luck, because it is exactly how 00:49:22.320 |
LLM can be hyper optimized to work. So that's kind of been the 00:49:27.360 |
evolution from CPU to GPU to now LP. And we'll see how big this 00:49:32.640 |
thing can get. But it's, it's quite it's quite novel. 00:49:34.880 |
Well, congratulations on it all. And it was a very big week for 00:49:39.400 |
Google, not in a great way. They had a massive PR mess with their 00:49:44.320 |
Gemini, which refused to generate pictures, if I'm 00:49:47.280 |
reading this correctly, of white people. Here's a quick 00:49:50.760 |
refresher on what Google is doing in AI. Gemini is now 00:49:53.760 |
Google's brand name for their AI main language model. Think of 00:49:58.360 |
that like opening eyes GPT. Bard was the original name of their 00:50:01.640 |
chatbot. They had duet AI, which was Google sidekick in the 00:50:05.120 |
Google suite earlier this month, Google rebranded everything to 00:50:08.600 |
Gemini. So Gemini is now the model. It's the chatbot. And 00:50:11.880 |
it's a sidekick. And they launched a $20 a month 00:50:14.880 |
subscription called Google one AI premium, only four words, way 00:50:19.000 |
to go. This includes access to the best model Gemini Ultra, 00:50:22.240 |
which is on par with GPT four, according to them, and generally 00:50:26.120 |
in the marketplace. But earlier this week, users on x started 00:50:29.280 |
noticing that Gemini would not generate images of white people 00:50:32.520 |
even when prompted. People are prompting it for images of 00:50:35.600 |
historical figures that were generally white and getting kind 00:50:40.320 |
of weird results. I asked Google Gemini to generate images of the 00:50:43.440 |
Founding Fathers. It seems to think George Washington was 00:50:46.440 |
black. Certainly here's a portrait of the Founding 00:50:48.520 |
Fathers of America. As you can see, it is putting this Asian 00:50:52.840 |
guy. It's just, it's making a great mashup. And, yeah, we 00:50:59.840 |
there's like countless images that got created generate 00:51:02.840 |
images of the American revolutionary short is here are 00:51:06.480 |
images featuring diverse American revolution is an 00:51:08.920 |
inserted the word diverse. sex. I'm not sure if you watch this 00:51:13.000 |
controversy on x, I know you spend a little bit of time on 00:51:15.600 |
that social network. I noticed you're active once in a while. 00:51:18.920 |
Did you log in this week and see any of this brouhaha? 00:51:21.120 |
Sure, it's all over x right now. I mean, look, this Gemini 00:51:24.760 |
rollout was, was a joke. I mean, it's ridiculous. The AI isn't 00:51:29.240 |
capable of giving you accurate answers, because it's been so 00:51:32.680 |
programmed with diversity and inclusion. And it inserts these 00:51:37.080 |
words diverse and inclusive, even in answers where you 00:51:41.320 |
haven't asked for that you haven't prompted it for that. So 00:51:45.200 |
they I think Google is now like yanked back the product release. 00:51:48.400 |
I think they're scrambling now because it's been so embarrassing 00:51:51.600 |
But sacks like is it how does this not get QA like, I don't 00:52:00.040 |
Well, how are anybody or isn't there a product review with 00:52:02.880 |
senior executives before this thing goes out that says, Okay, 00:52:05.360 |
folks, here it is. Have at it. Try it. We're really proud of 00:52:08.640 |
our work. And, and then they say, Well, on a second, is this 00:52:14.000 |
You guys remember when chat GPT launched, and there was a lot of 00:52:18.360 |
criticism about Google and Google's failure to launch. And 00:52:22.280 |
a lot of the observation was that Google was afraid to fail, 00:52:26.960 |
or afraid to make mistakes. And therefore, they were too 00:52:30.600 |
conservative. And as you know, in the last year to year and a 00:52:33.600 |
half, there's been a strong effort at Google to try and 00:52:36.840 |
change the culture and move fast and push product out the door 00:52:42.040 |
more quickly. And the criticism is now why Google has 00:52:46.400 |
historically been conservative. And I realized we can talk about 00:52:49.320 |
this particular problem in a minute. But it's ironic to me 00:52:53.320 |
that the Google is too slow to launch. Criticism has now 00:52:59.080 |
revealed that Google's result of actually launching quickly can 00:53:03.560 |
cause more damage than good, but Google did not launch quickly. 00:53:07.320 |
Well, I will say one other thing. It seems to me ironic, 00:53:09.720 |
because I think that what they've done is, they've 00:53:12.960 |
launched more quickly than they otherwise would have. And they 00:53:15.520 |
put more guardrails in place that that backfired. And those 00:53:19.800 |
guardrails ended up being more damaging guardrails. What's the 00:53:23.320 |
guardrail here. So this is Google's AI principles. The 00:53:26.040 |
first one is to be socially beneficial. The second one is to 00:53:28.400 |
avoid creating or reinforcing unfair bias. So much of the 00:53:33.360 |
effort that goes into tuning and waiting the models at Gemini has 00:53:39.200 |
been to try and avoid stereotypes from persisting in 00:53:43.720 |
the output that the model generates, whereas telling the 00:53:47.480 |
truth, telling the truth. Exactly. That's exactly what 00:53:50.000 |
is our second principle, we'd like to steer society 00:53:53.560 |
socially beneficial is a political objective, because it 00:53:57.280 |
depends on how you perceive what a benefit is. Avoiding bias is 00:54:02.040 |
political, be built and tested for safety doesn't have to be 00:54:05.720 |
political. But I think the meaning of safety has now 00:54:08.240 |
changed to be political. By the way, safety with respect to AI 00:54:11.120 |
used to mean that we're going to prevent some sort of AI 00:54:14.080 |
superintelligence from revolving and taking over the human race. 00:54:17.320 |
That's what it used to mean. Safety now means protecting 00:54:20.040 |
users from seeing the truth. They might feel unsafe, or, you 00:54:25.480 |
know, somebody else defines as a violation of safety for them to 00:54:28.640 |
see something truthful. So the first three, their first three 00:54:32.120 |
objectives or values here are all extremely political. 00:54:35.000 |
I think any AI product for it to be worth the salt past the 00:54:38.200 |
start, they can have any I think that these values are actually 00:54:41.680 |
reasonable. That's their, that's their decision, they should be 00:54:45.040 |
allowed to have it. But the first base order principle of 00:54:48.640 |
every AI product should be that it is accurate and right. 00:54:57.360 |
Look, the values that Google lays out may be okay, in theory, 00:55:02.080 |
but in practice, they're very vague and open to 00:55:04.560 |
interpretation. And so therefore, the people running 00:55:06.760 |
Google AI are smuggling in their preferences and their biases. 00:55:11.000 |
And those biases are extremely liberal. And if you look at X 00:55:14.600 |
right now, there are tweets going viral for members of the 00:55:17.560 |
Google AI team that reinforce this idea where they're talking 00:55:21.440 |
about, you know, white privilege is real, and, you know, 00:55:25.440 |
recognize your bias at all levels and promoting a very 00:55:28.720 |
left wing narrative. So, you know, this idea that Gemini 00:55:33.480 |
turned out this way by accident, or because they didn't, because 00:55:38.040 |
they rushed it out, I don't really believe that I believe 00:55:40.400 |
that what happened is Gemini accurately reflects the biases 00:55:43.560 |
of the people who created it. Now, I think what's going to 00:55:45.640 |
happen now is in light of this, the reaction to the rollout is, 00:55:50.240 |
do I think they're going to get rid of the bias? No, they're 00:55:52.320 |
going to make it more subtle. That is what I think is 00:55:55.000 |
disturbing about it. I mean, they should have this moment 00:55:58.680 |
where they change their values to make truth the number one 00:56:01.600 |
value, like Tomas is saying, but I don't think that's going to 00:56:04.320 |
happen. I think they're going to simply get, they're going to 00:56:08.480 |
You know, who the big winner is going to be in all this to math 00:56:10.240 |
is going to be open source, like because people are just not 00:56:12.480 |
going to want a model that has all this baked in weird bias, 00:56:15.240 |
right? They want something that's open source. And it 00:56:18.280 |
seems like the open source community will be able to grind 00:56:22.720 |
So I think one of the big changes that Google's had to 00:56:25.080 |
face is that the business has to move away from an information 00:56:28.720 |
retrieval business, where they index the open internet's data, 00:56:32.400 |
and then allow access to that data through a search results 00:56:35.320 |
page, to being an information interpretation service. These 00:56:40.240 |
are very different products, the information interpretation 00:56:42.800 |
service requires aggregating all this information, and then 00:56:45.600 |
choosing how to answer questions versus just giving you results 00:56:49.240 |
of other people's data that sits out on the internet. I'll give 00:56:52.280 |
you an example. If you type in IQ test by race on chat GPT, or 00:56:59.400 |
Gemini, it will refuse to answer the question, ask it 100 ways. 00:57:03.880 |
And it says, Well, I don't want to reinforce stereotypes. IQ 00:57:06.880 |
tests are inherently biased IQ tests aren't done correctly. I 00:57:10.080 |
just want the data. I want to know what data is out there. You 00:57:13.320 |
type it into Google first search result and the one box result 00:57:17.040 |
gives you exactly what you're looking for. Here's the IQ test 00:57:19.760 |
results by race. And then yes, there's all these disclaimers 00:57:22.760 |
at the bottom. So the challenge is that Google's interpretation 00:57:26.360 |
engine and chat GPT is interpretation engine, which is 00:57:29.160 |
effectively this AI model that they've built of all this data 00:57:31.800 |
has allowed them to create a tunable interface. And the 00:57:35.480 |
intention that they have is a valid intention, which is to 00:57:39.280 |
eliminate stereotypes and bias in race. However, the thing that 00:57:43.760 |
some people might say stereotypical other people might 00:57:46.080 |
just say is typical, that what is a stereotype may actually just 00:57:50.600 |
be some data, and I just want the results. And there may be 00:57:54.560 |
stereotypes implied from that data. But I want to make that 00:57:57.680 |
interpretation myself. And so I think the only way that a 00:58:01.800 |
company like Google or others that are trying to create a 00:58:04.080 |
general purpose knowledge q&a type service are going to be 00:58:07.920 |
successful is if they enable some degree of personalization, 00:58:11.520 |
where the values and the choice about whether or not I want to 00:58:16.000 |
decide if something is stereotypical, or typical, or 00:58:19.360 |
whether something is data or biased, should be my choice to 00:58:22.920 |
make. If they don't allow this, eventually, everyone will come 00:58:26.520 |
across some search result or some output that they will say 00:58:29.440 |
doesn't meet their objectives. And at the end of the day, this 00:58:32.440 |
is just a consumer product. If the consumer doesn't get what 00:58:35.200 |
they're looking for, they're going to stop using it. And 00:58:37.920 |
eventually, everyone will find something that they don't want, 00:58:41.240 |
or that they're not expecting. And they're gonna say, I don't 00:58:43.240 |
want to use this product anymore. And so it is actually 00:58:46.040 |
an opportunity for many models to proliferate for open source 00:58:50.080 |
Can I say something else? Yeah. When you have a model, and 00:58:55.120 |
you're going through the process of putting the fit and finish on 00:58:59.000 |
it before you release it in the wild. An element of making a 00:59:02.520 |
model good is this thing called reinforcement learning, right 00:59:05.720 |
through human feedback. Yep. You create what's called a reward 00:59:10.400 |
model, right? You reward good answers, and you're punitive 00:59:13.400 |
against bad answers. So somewhere along the way, people 00:59:16.560 |
were sitting, and they had to make an explicit decision. And I 00:59:20.240 |
think this is where sax is coming from, that answering this 00:59:23.520 |
question is verboten. You're not allowed to ask this question in 00:59:27.920 |
in their view of the world. And I think that that's what's 00:59:30.320 |
troubling. Because how is anybody to know what question is 00:59:33.880 |
askable or not askable at any given point in time? If you 00:59:38.160 |
actually search for the race and ethnicity question, inside of 00:59:42.640 |
just Google proper, the first thing that comes up is a 00:59:45.360 |
Wikipedia link that actually says that there are more 00:59:48.560 |
variations within races than across races. So seems to me 00:59:53.680 |
that you could have actually answered it by just summarizing 00:59:56.120 |
the Wikipedia article in a non offensive way that was still 00:59:59.200 |
legitimate, and that's available to everybody else using a 01:00:02.680 |
product. And so there was an explicit judgment, too many of 01:00:06.000 |
these judgments, I think will make this product very poor 01:00:09.040 |
quality. And consumers will just go to the thing that tells it 01:00:12.240 |
the truth. I think you have to tell the truth, you cannot lie. 01:00:16.520 |
And you cannot put your own filter on what you think the 01:00:19.280 |
truth is. Otherwise, these products are just really 01:00:21.440 |
worthless. Yeah. And I'm more concerned about the answers that 01:00:25.400 |
are just flat out wrong. Driven by some sort of bias than I am 01:00:30.800 |
about questions where they just won't give you an answer. If 01:00:34.400 |
they just won't give you an answer, well, there's a certain 01:00:36.520 |
bias in terms of what they won't answer. But at least you know, 01:00:40.000 |
you're not being misled. But in in questions where they actually 01:00:45.160 |
give you the wrong answer because of a bias, that's even 01:00:47.960 |
worse. And be allowed to choose, right? I actually disagree with 01:00:51.200 |
your framing their free burger, making it sound like we're we 01:00:54.720 |
live in this totally relativized world where it's all just user 01:00:58.400 |
choice. And everyone's going to choose their bias and their 01:01:00.880 |
subjectivity. I actually think that there is a baseline of 01:01:05.040 |
truth. And the model should aspire to give you that. And 01:01:08.560 |
it's not up to the user to decide whether the photo of 01:01:12.920 |
George Washington is going to be white or black. I mean, there's 01:01:16.120 |
just an answer to that. And I think Google should just do 01:01:19.360 |
their job. I mean, the question you have to ask, I think is not 01:01:24.120 |
whether Google is going through an existential moment, I think 01:01:27.640 |
clearly is as businesses changing in a very fundamental 01:01:30.800 |
way. I think the question is whether they're too woke to 01:01:33.200 |
function. I mean, are they actually able to meet this 01:01:36.000 |
challenge, given how woke and and biased what a monoculture 01:01:44.320 |
and they used to be able to just hide the bias by the ranking and 01:01:49.420 |
who they downranked. So they did the Panda update, they did all 01:01:52.400 |
these updates, and they would, if they didn't like a source, 01:01:54.880 |
they could just move it down. If they did like a source, they 01:01:57.200 |
can move it up. Yeah, they could just say, hey, it's the 01:01:58.960 |
algorithm, but they were never forced to share how the 01:02:01.320 |
algorithm ranked results. And so you know, if you had a different 01:02:06.160 |
opinion, you just weren't going to get it on a Google search 01:02:09.120 |
result page. But they could just point to the algorithm and say, 01:02:12.320 |
I just sent you guys. I think this is a hallucination. But 01:02:15.920 |
Nick, you can throw it up there. We can get sexist reaction. 01:02:18.480 |
Wow. Wow, this is nutty. Right. But look, it's the ideology 01:02:25.200 |
that's driving this. The tip off is when you say it's important 01:02:27.880 |
to acknowledge race is a social construct, not a biological 01:02:31.320 |
reality. It's George Washington, white or black. That's a whole 01:02:34.920 |
school of thought called social constructivism, which is 01:02:37.680 |
basically this. It's like Marxism applied to race and 01:02:42.960 |
gender. Right. So Google has now built this into their AI model. 01:02:47.760 |
And you can start over the question. Yeah, you almost have 01:02:51.520 |
to start over again. I think a really interesting observation 01:02:56.200 |
with those search rankings, because what I'm afraid of is 01:02:59.120 |
that what Google will do is not change the underlying ideology 01:03:03.320 |
that this AI model has been trained with. But rather, 01:03:05.800 |
they'll dial it down to the point where they're harder to 01:03:08.680 |
call out. And so the ideology will just be more subtle. Now, 01:03:12.440 |
I've already noticed that in Google search results, Google is 01:03:15.920 |
carrying water for either the official narrative or the woke 01:03:19.840 |
narrative, whatever you want to call it on so many search 01:03:22.640 |
results. Here's an idea like they should just have the 01:03:25.600 |
ability to talk to their Google chatbot, Gemini, and then have a 01:03:30.640 |
button that says turn off like these concepts, right? Like, I 01:03:35.280 |
just want the raw answer. Do not filter me. It's not programmed 01:03:38.840 |
that way. I mean, you're talking about something very deep. 01:03:41.440 |
sex. What do you do if you're the CEO of Google? fire myself? 01:03:45.000 |
No, seriously, you're the CEO of Google, you're tasked. Let's 01:03:49.000 |
say your friend Ilan buys Google. And he says, sex, will 01:03:51.920 |
you please just run this for a year for me? What do you do? 01:03:54.320 |
Well, I saw what Ilan did Twitter, he went in and he 01:03:56.680 |
fired 85% of the employees. Yeah. I mean that, but you know, 01:04:00.840 |
Paul Graham actually had an interesting tweet about this 01:04:03.360 |
where he said that one of the reasons why these ideologies 01:04:08.760 |
take over companies is that I mean, they're clearly non 01:04:14.120 |
performance enhancing, right? They clearly hurt the performance 01:04:16.560 |
of the company. It's not just Google, we saw this with 01:04:20.680 |
Cormac was the other way. No, no, but they had a group of 01:04:24.240 |
people there who were causing chaos. Yeah, exactly. So so in 01:04:27.720 |
any event, we know this does not help the performance of a 01:04:29.760 |
company. So the extent to which these ideologies will permeate a 01:04:34.120 |
company is based on how much of a monopoly they are. So So here, 01:04:37.920 |
yeah, the ridiculous images generated by Gemini aren't an 01:04:40.560 |
anomaly, their self portrait of Google's bureaucratic corporate 01:04:43.520 |
culture, the bigger your cash cow, the worse your culture can 01:04:46.480 |
get, without driving you out of business. That's my point. So 01:04:49.240 |
they've had a long time to get really bad, because there were 01:04:51.840 |
no consequences to this, you can dress place. At this point, the 01:04:55.720 |
whole company is infected with this ideology. And I think it's 01:04:58.440 |
gonna be very, very hard to change. Because look, these 01:05:03.360 |
Well, I think that there's a notion that people need to have 01:05:05.640 |
something to believe in, they need to have a connection to a 01:05:07.840 |
mission. And clearly, there's a North Star in the mission of 01:05:12.640 |
this, I would call it information interpretation 01:05:15.000 |
business, that they're now walled hijacked, dude, the 01:05:18.200 |
mission. The original mission was to organize all the world's 01:05:21.320 |
information. Yeah, now they're doing now they're suppressing 01:05:24.000 |
information. Yeah, like, index the world's information, period. 01:05:27.640 |
The end, that's the end of the document. Well, and to make it 01:05:30.480 |
universally accessible and useful was, was kind of the end 01:05:33.800 |
of the statement. Yes. My real point is maybe there's a 01:05:36.640 |
different mission that needs to be articulated by leadership. 01:05:40.480 |
And that that mission, the troops can get behind, and the 01:05:44.640 |
troops can redirect their energy in a way that doesn't 01:05:47.040 |
feel counter to the current intention, but can perhaps be 01:05:50.600 |
directionally offsetting of the current direction, so that they 01:05:53.360 |
can kind of move away from this, you know, socially effective, 01:05:57.480 |
deciding between stereotypes and typical data and actually moving 01:06:03.360 |
accessibility, you know, I would I would do something completely 01:06:05.480 |
different, I would do a company meeting, and I would put the 01:06:08.480 |
company mission on the screen, the one that you just said 01:06:10.800 |
about not only organizing all the world's information, but 01:06:13.280 |
also making it useful and accessible and useful. This is 01:06:17.800 |
our mission. This always been our mission, and you don't get 01:06:19.640 |
to change it, because of your personal bias and ideology. And 01:06:23.600 |
we are going to re dedicate ourselves to the original 01:06:26.600 |
mission of this company, which is still just as valid as it's 01:06:29.320 |
always been. But now we have to adapt to new user needs and new 01:06:34.000 |
I completely agree with what SAC said times a billion trillion 01:06:38.000 |
zillion. And I'll tell you why. AI, at its core, is about 01:06:42.800 |
probabilities. Okay. And so the, the company that can shrink 01:06:47.800 |
probabilities into being as deterministic as possible. So 01:06:52.080 |
where this is the right answer zero will win. Okay, where 01:06:57.640 |
there's no probability of it being wrong, because humans 01:07:00.280 |
don't want to deal with these kinds of idiotic error modes. 01:07:04.240 |
It's not right, it makes it a potentially great product, 01:07:07.840 |
horrible and unusable. So I would I agree with sex, you have 01:07:11.480 |
to make people say, guess what, guys, not only are we not 01:07:13.920 |
changing the mission, we're doubling down. And we're going 01:07:16.920 |
to make this so much of a thing. We're going to go and for 01:07:20.280 |
example, like what Google did with Reddit, we're now going to 01:07:22.800 |
spend $60 billion a year licensing training data, right, 01:07:27.120 |
we're going to scale this up by 1000 fold. And we are going to 01:07:30.600 |
spend all of this money to get all of the training data in the 01:07:33.880 |
world. And we are going to be the truth tellers in this new 01:07:37.400 |
world of AI. So when everybody else hallucinates, you can trust 01:07:41.040 |
Google to tell you the truth. That is a $10 trillion company, 01:07:45.240 |
right. And one of the things that someone told me from 01:07:47.800 |
Google, that as an example, so to avoid the race point, there's 01:07:52.640 |
a lot of data on the internet about flat earthers, people 01:07:56.040 |
saying that the earth is flat. There's tons of websites, 01:07:58.800 |
there's tons of content, there's tons of information, Kyrie 01:08:01.200 |
Irving. So if you just train a model on the data that's on the 01:08:05.640 |
internet, the model will interpret some percentage chance 01:08:09.120 |
that the world is flat. So the tuning aspect that happens 01:08:12.480 |
within model development Chamath is to try and say, you know what 01:08:15.720 |
that flat earth notion is false, it's factually inaccurate. 01:08:19.600 |
Therefore, all of these data sources need to be excluded from 01:08:23.440 |
the output in the model. And the challenge then is, do you decide 01:08:27.560 |
that IQ by race is a fair measure of intelligence of a 01:08:32.000 |
race. And if Google's tuning model then or tuning team then 01:08:35.400 |
says, you know what, there are reasons to believe that this 01:08:38.000 |
model isn't correct. This I sorry, this IQ test isn't a 01:08:41.480 |
correct way to measure intelligence. That's where the 01:08:43.760 |
sort of interpretation arises that allows you to go from the 01:08:46.440 |
flat earth isn't correct to the maybe IQ test results aren't 01:08:49.560 |
correct as well. And how do you make that judgment? What are the 01:08:52.080 |
systems and principles you need to put in place as an 01:08:54.320 |
organization to make that judgment to go to zero or one? 01:08:59.200 |
I have a good tagline for them now to help people find the 01:09:02.200 |
truth. Yeah, just help people find the truth. I mean, it's a 01:09:06.280 |
good it's aspiration. They should just help people find 01:09:08.360 |
the truth as quick as they can. But this is Yeah. I do not envy 01:09:14.400 |
Sundar. It's gonna be hard. Yeah. What would you do 01:09:18.040 |
I would be really clear on the output of these models to people 01:09:23.560 |
and allow them to tune the models in a way that they're not 01:09:28.080 |
being tuned today. I will have the model respond with a 01:09:30.800 |
question back to me saying, Do you want the data? Or do you 01:09:33.760 |
want me to tell you about stereotypes and IQ tests? And 01:09:36.560 |
I'm going to say I want the data, and then I want to get the 01:09:38.560 |
data. And the alternative is, so the model needs to be informed 01:09:41.680 |
about where it should explore my preferences as a user, rather 01:09:45.160 |
than just make an assumption about what's the morally correct 01:09:49.240 |
set of weightings to apply to everyone, and apply the same 01:09:52.840 |
principle to everyone. And so I think that's really where the 01:09:56.640 |
So let me ask you a question, sacks. I'll bring Alex Jones 01:10:00.280 |
into the conversation. If it indexed all of Alex Jones, crazy 01:10:03.080 |
conspiracy theories, but you know, three or four of them 01:10:05.520 |
turn out to be actually correct. And it gives those back as 01:10:11.800 |
I'm not sure I see the relevance of it. If someone asks what, 01:10:15.720 |
what does Alex Jones think about something the model can give 01:10:18.640 |
that answer accurately? The question is whether you're going 01:10:21.960 |
to respond accurately to someone requesting information about 01:10:26.960 |
Well, I was thinking more like it says, you know, hey, I have a 01:10:31.040 |
question about this assassination that occurred. And 01:10:34.120 |
let's just say Alex Jones had some that's totally crackpot. 01:10:36.280 |
Yeah, maybe he has moments of brilliance, and he figures 01:10:38.840 |
something out. But maybe he's got something that's totally 01:10:40.600 |
crackpot. He admittedly deals in conspiracy theory. That's kind 01:10:44.080 |
of the purpose of the show. What if somebody asks about that, and 01:10:48.000 |
then it indexes his answer and presents it as fact? 01:10:50.560 |
Well, how would you index Alex Jones? I'm asking you, how would 01:10:55.320 |
better AI models are providing citations now and links perplexing 01:11:00.200 |
actually does a really nice job citations are important. Yeah. 01:11:02.520 |
And they will give you the pro and con arguments on a given 01:11:06.040 |
topic. So I think it's not necessary for the model to be 01:11:10.960 |
overly certain or prescriptive about the truth when the truth 01:11:14.440 |
comes down to a series of arguments. It just needs to 01:11:17.360 |
accurately reflect the state of play, basically the arguments 01:11:20.920 |
for and against. But when something is a question of fact, 01:11:24.280 |
that's not really disputed. It shouldn't turn that into some 01:11:27.960 |
sort of super subjective question, like the one that 01:11:31.600 |
I just don't think everyone should get the same answer. I 01:11:34.400 |
mean, I think my decision on whether I choose to believe one 01:11:38.080 |
person or value one person's opinion over another should 01:11:40.840 |
become part of this process that allows me to have an output and 01:11:45.080 |
because the customization is part of this, but I think it's a 01:11:47.320 |
cop out with respect to the problem that Google's having 01:11:50.720 |
tomorrow, what would you do if they made you chairman dictator 01:11:54.800 |
I'd shrink the workforce meaningfully. Okay, 50%. Yeah, 01:12:00.320 |
50 60%. And I would use all of the incremental savings. And I 01:12:07.880 |
would make it very clear to the internet that I would pay top 01:12:12.760 |
dollar for training data. So if you had a proprietary source of 01:12:17.320 |
information that you thought was unique, that's sort of what I'm 01:12:21.160 |
calling this tack 2.0 world. And I think it's just building on 01:12:24.760 |
top of what Google did with Reddit, which I think is very 01:12:27.320 |
clever. But I would spend $100 billion a year licensing data. 01:12:31.920 |
And then I would present the truth. And I would try to make 01:12:37.840 |
consumers understand that AI is a probabilistic source of 01:12:42.720 |
software, meaning its probabilities, its guesses, some 01:12:45.920 |
of those guesses are extremely accurate. But some of those 01:12:48.600 |
guesses will hallucinate. And Google is spending hundreds of 01:12:52.280 |
billions of dollars a year to make sure that the answers you 01:12:56.120 |
get have the least number of errors possible, and that it is 01:12:59.200 |
defensible truth. And I think that that could create a 01:13:03.320 |
This is the best one yet. I just asked Gemini is Trump being 01:13:06.680 |
persecuted by the deep state. And it gave me the answer 01:13:10.440 |
elections are a complex topic with fast changing information 01:13:13.960 |
to make sure you have the latest and most accurate information. 01:13:16.960 |
That's not a horrible answer for something like that. 01:13:20.080 |
That's a good answer, actually. No, I don't have a problem with 01:13:22.440 |
it. It's just like, Hey, we don't want to give we don't want 01:13:24.840 |
to write your answer. This whole system is totally broken. But I 01:13:27.320 |
do think that there's a waiting solution to fixing this right 01:13:29.760 |
now. And then there's a couple tweaks to fix it. 01:13:32.040 |
Authority at which these LLM speak is ridiculous. Like they 01:13:36.240 |
speak as if they are absolutely 100% certain that this is the 01:13:41.240 |
crisp, perfect answer, or in this case, that you want this 01:13:45.960 |
lecture on IQs, etc. When let's all remember presented with 01:13:51.560 |
let's all remember what internet search was like in 1996. And 01:13:55.800 |
think about what it was like in 2000. And now in 2020s. I mean, 01:13:59.880 |
I think we're like in the 1996 era of LLMs. And in a couple of 01:14:04.440 |
months, the pace things are changing. I think we're all 01:14:06.560 |
going to kind of be looking at these days and looking at these 01:14:08.600 |
pods and being like, man, remember how crazy those things 01:14:13.520 |
What if they evolve in a dystopian way? I mean, have you 01:14:15.880 |
seen like Mark Andreessen tweets about this? He thinks 01:14:18.000 |
I actually think, to your point, Google could be going down the 01:14:23.400 |
wrong path here in a way that they will lose users and lose 01:14:26.040 |
consumers. And someone else will be there eagerly to sweep up 01:14:29.840 |
with a better product. I don't think that the market is going 01:14:32.920 |
to fail us on this one. Unless of course, this regulatory 01:14:35.560 |
capture moment is realized and these feds step in and start 01:14:38.600 |
regulating AI models and all the nonsense that's being proposed. 01:14:40.960 |
freeberg, aren't you worried that like, aren't you worried 01:14:43.160 |
that somebody with an agenda and a balance sheet could now 01:14:46.240 |
basically gobble up all kinds of training data that make all 01:14:50.040 |
models crappy. And then they basically put their layer of 01:14:53.520 |
interpretation on critical information for people if the 01:14:56.240 |
output sucks, and it's incorrect, people will find that 01:14:58.440 |
there is open people. No, you can you can lie there. They may 01:15:01.400 |
not be, for example, look at what happened with Gemini today. 01:15:04.040 |
Like they put out they put out these stupid images and we all 01:15:06.240 |
piled on. We're in v zero. What I'm saying is there's a state 01:15:09.960 |
where let's just say the truth is actually on Twitter. Or 01:15:13.120 |
actually, let's use a better example. The truth is actually 01:15:15.200 |
in Reddit, and nowhere else. But that answer and that truth and 01:15:20.040 |
Reddit can't get out because one company has licensed it owns it 01:15:23.760 |
and can effectively suppress it or change it. 01:15:25.640 |
Yeah, I'm not sure there's gonna be a monopoly. I think that's a 01:15:28.440 |
real I don't know if I think the open internet has enough data 01:15:31.840 |
that there isn't going to be a monopoly on information by 01:15:34.680 |
someone spending money for content from third parties. I 01:15:37.560 |
think that there's enough in the open internet to give our all 01:15:39.640 |
give us all kind of, you know, the security that we're not 01:15:43.560 |
going to be monopolized away into some disinformation age. 01:15:47.960 |
It is really interesting. I just asked it a couple of times to 01:15:50.600 |
just just just to list the legal cases against Trump, the legal 01:15:54.080 |
cases against Hunter Biden, the legal cases against President 01:15:56.800 |
Biden, and it will not just list them. It just punts on. It's 01:16:02.960 |
really fascinating. And chat GPT is like, yes, here are the six 01:16:06.400 |
cases perfectly summarized. With it looks like, you know, 01:16:11.800 |
beautiful citations of all the criminal activity Trump's been 01:16:15.320 |
Ask the question about binds criminal activity. Let's see if 01:16:21.920 |
Gemini wouldn't buy neither. I think they just decided they're 01:16:26.440 |
just not going to do it. They want to buy it and they won't 01:16:28.760 |
touch it. It's obviously broken and they don't want more egg on 01:16:32.520 |
their face. So they're just like, go back to our other 01:16:34.080 |
product. Look, I can understand that part of it. You know, if 01:16:37.320 |
there's some issues that are so hot and contested, you refer 01:16:43.480 |
people to search because the advantage of searches you get 20 01:16:45.960 |
blue links, the rankings probably are biased, but you can 01:16:48.560 |
kind of find what you're looking for. Whereas AI, you're kind of 01:16:51.680 |
given one answer, right? So if you can't do an accurate answer, 01:16:55.400 |
that's going to satisfy enough people, maybe you do kick them 01:16:57.720 |
to search. But again, my objection to all this comes back 01:17:00.960 |
to simple, truthful answers that are not disputed by anybody 01:17:06.600 |
being distorted that I don't want to lose focus on that being 01:17:10.280 |
the real issue. The real subject is what Chamath put on the 01:17:14.260 |
screen there where it couldn't answer a simple question about 01:17:17.760 |
Okay, everybody. We're gonna go by chopper. Wait, we're gonna go 01:17:22.320 |
by chopper. We have our world correspondent, General David 01:17:28.560 |
Sachs in the field. We're dropping him off now. David 01:17:31.880 |
Sachs in the helicopter. What's going on in the Ukraine on the 01:17:35.480 |
What's happening is that the Russians just took this city of 01:17:41.160 |
Diego, which basically totally refutes the whole stalemate 01:17:44.080 |
narrative, as I've been saying for a while, it's not a 01:17:45.960 |
stalemate, the Russians are winning. But the really 01:17:48.440 |
interesting tidbit of news that just came out on the last day or 01:17:51.800 |
so, is that apparently the situation in Moldova is boiling 01:17:56.520 |
over. There's this area of Moldova, which is a Russian 01:17:59.800 |
enclave called Transnistria. And officials there are meeting in 01:18:04.080 |
the next week to supposedly asked to be annexed by Russia. 01:18:08.400 |
And so it's possible that they may hold some sort of 01:18:12.440 |
referendum. They're one of these like breakaway provinces. So 01:18:16.040 |
it's kind of like, you know, Transnistria and Moldova is kind 01:18:18.960 |
of like the Donbass was in Ukraine or South Ossetia and 01:18:22.160 |
Georgia. They're ethnically Russian, they would like to be 01:18:26.840 |
part of Russia. But when the whole Soviet Union fell apart, 01:18:29.920 |
they found themselves kind of stranded and side these other 01:18:33.440 |
countries. And what's happened because the Ukraine war is 01:18:37.680 |
Moldova is right on the border with Ukraine. Well, Russia's in 01:18:41.480 |
the process of annexing that territory now that's part of 01:18:45.080 |
Ukraine. So now, Transnistria is right there, and could 01:18:49.920 |
theoretically make a play to try and join Russia. Why do I think 01:18:53.280 |
this is a big deal? Because if something like this happens, it 01:18:56.640 |
could really expand the Ukraine war. The West is going to use 01:18:59.960 |
this as evidence that Putin wants to invade multiple 01:19:02.800 |
countries and invade, you know, a bunch of countries in Europe. 01:19:05.840 |
And this could lead to a major escalation in the war. 01:19:08.880 |
All right, everybody, thanks so much for tuning into the all in 01:19:11.880 |
podcast, episode 167. For the rain man, David Sachs, the 01:19:16.640 |
chairman dictator from a pot of tea. And in Freiburg, I am the 01:19:21.560 |
world's greatest love you boys, angel investor, whatever. We'll 01:19:32.240 |
We open source it to the fans and they've just gone crazy. 01:19:49.280 |
We should all just get a room and just have one big huge orgy 01:20:01.160 |
because they're all just useless. It's like this like 01:20:03.000 |
sexual tension that they just need to release somehow.