back to indexGary Marcus: Toward a Hybrid of Deep Learning and Symbolic AI | Lex Fridman Podcast #43
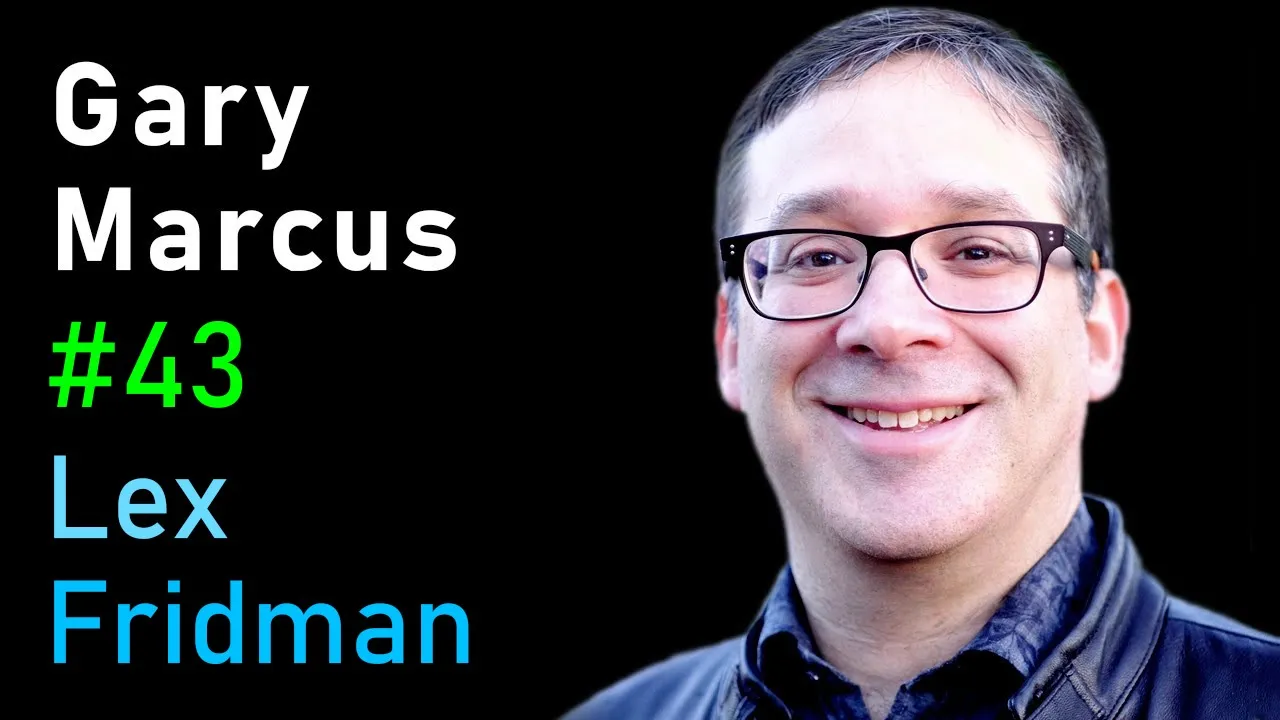
00:00:00.000 |
The following is a conversation with Gary Marcus. 00:00:05.000 |
founder of Robust AI and Geometric Intelligence. 00:00:25.520 |
highlighting the limits of deep learning and AI in general, 00:00:28.840 |
and discussing the challenges before our AI community 00:00:40.120 |
I try to find paths toward insight, towards new ideas. 00:00:47.640 |
I'll often continuously try on several hats, several roles. 00:00:52.280 |
One, for example, is the role of a three-year-old 00:01:00.360 |
The other might be a role of a devil's advocate 00:01:02.920 |
who presents counter ideas with a goal of arriving 00:01:23.120 |
give it five stars on iTunes, support it on Patreon, 00:01:32.520 |
And now, here's my conversation with Gary Marcus. 00:01:36.340 |
Do you think human civilization will one day have 00:01:40.400 |
to face an AI-driven technological singularity 00:01:42.960 |
that will, in a societal way, modify our place 00:01:46.520 |
in the food chain of intelligent living beings 00:01:50.120 |
- I think our place in the food chain is already changed. 00:01:54.860 |
So there are lots of things people used to do by hand 00:01:59.200 |
If you think of a singularity as like one single moment, 00:02:04.600 |
But I think that there's a lot of gradual change, 00:02:09.280 |
I mean, I'm here to tell you why I think it's not nearly 00:02:11.460 |
as good as people think, but the overall trend is clear. 00:02:14.440 |
Maybe Ray Kurzweil thinks it's an exponential, 00:02:22.440 |
We are gonna get to human-level intelligence, 00:02:27.440 |
artificial general intelligence at some point, 00:02:31.840 |
in the food chain, 'cause a lot of the tedious things 00:02:34.280 |
that we do now, we're gonna have machines do, 00:02:36.280 |
and a lot of the dangerous things that we do now, 00:02:41.700 |
from people finding their meaning through their work, 00:02:53.940 |
in fact, removing the meaning of the word singularity, 00:02:56.620 |
it'll be a very gradual transformation, in your view? 00:03:00.540 |
- I think that it'll be somewhere in between, 00:03:03.460 |
and I guess it depends what you mean by gradual and sudden. 00:03:08.860 |
that intelligence is a multidimensional variable. 00:03:14.420 |
as if IQ was one number, and the day that you hit 262 00:03:22.700 |
And really, there's lots of facets to intelligence. 00:03:28.560 |
and there's mathematical intelligence, and so forth. 00:03:32.060 |
Machines, in their mathematical intelligence, 00:03:46.860 |
that machines have grasped, and some that they haven't, 00:03:51.780 |
to get them to, say, understand natural language, 00:04:03.020 |
And I don't know that all of these things will come at once. 00:04:05.620 |
I think there are certain vital prerequisites 00:04:09.320 |
So, for example, machines don't really have common sense now. 00:04:12.500 |
So they don't understand that bottles contain water, 00:04:15.540 |
and that people drink water to quench their thirst, 00:04:19.380 |
They don't know these basic facts about human beings, 00:04:22.100 |
and I think that that's a rate-limiting step for many things. 00:04:25.300 |
It's a rate-limiting step for reading, for example, 00:04:29.740 |
oh my God, that person's running out of water, 00:04:38.500 |
and your knowledge about how things work matter. 00:04:41.220 |
And so a computer can't understand that movie 00:04:44.320 |
if it doesn't have that background knowledge. 00:04:47.900 |
And so there are lots of places where if we had a good 00:04:53.740 |
many things would accelerate relatively quickly, 00:04:56.540 |
but I don't think even that is a single point. 00:05:02.500 |
And we might, for example, find that we make a lot of progress 00:05:05.660 |
on physical reasoning, getting machines to understand, 00:05:11.940 |
or how this gadget here works, and so forth and so on. 00:05:24.380 |
or to do direct experimentation on a microphone stand 00:05:28.700 |
than it is to do direct experimentation on human beings 00:05:34.860 |
- That's a really interesting point, actually. 00:05:36.860 |
Whether it's easier to gain common sense knowledge 00:05:43.300 |
includes both physical knowledge and psychological knowledge. 00:05:51.060 |
And the argument I was making is physical knowledge 00:05:55.980 |
lift a bottle, try putting a bottle cap on it, 00:06:01.980 |
and so the robot could do some experimentation. 00:06:09.180 |
So I can sort of guess how you might react to something 00:06:18.460 |
in the same way, or we'll probably shut them down. 00:06:24.260 |
how I respond to pain by pinching me in different ways, 00:06:31.020 |
and companies are gonna get sued or whatever. 00:06:32.900 |
So there's certain kinds of practical experience 00:06:41.060 |
What is more difficult to gain a grounding in? 00:06:49.980 |
I would say that human behavior is easier expressed 00:07:01.140 |
So you get to study and manipulate even a human behavior 00:07:09.580 |
So it's true why you said pain is like physical pain, 00:07:16.060 |
Emotional pain might be much easier to experiment with, 00:07:38.460 |
I wasn't there, but the conference has been renamed NIRPS, 00:07:41.340 |
but it used to be called NIPS when he gave the talk. 00:07:53.940 |
They understand what draws people back to things. 00:07:59.260 |
But even so, I think that there are only some slices 00:08:07.260 |
And of course, they're doing all kinds of VR stuff, 00:08:08.980 |
and maybe that'll change, and they'll expand their data. 00:08:25.140 |
some of the deepest things about human nature 00:08:27.860 |
and the human mind could be explored through digital form. 00:08:32.220 |
just now that brought up, I wonder what is more difficult? 00:08:41.820 |
but the people who are thinking beyond deep learning 00:08:52.300 |
which requires an understanding of the physical world 00:09:03.620 |
And it's interesting whether that's hard or easy. 00:09:06.820 |
- I think some parts of it are and some aren't. 00:09:08.540 |
So my company that I recently founded with Rod Brooks, 00:09:18.580 |
and psychological reasoning among many other things. 00:09:21.500 |
And there are pieces of each of these that are accessible. 00:09:29.720 |
that's a relatively accessible piece of physical reasoning. 00:09:34.760 |
and you know the height of the robot, it's not that hard. 00:09:37.000 |
If you wanted to do physical reasoning about Jenga, 00:09:45.240 |
With psychological reasoning, it's not that hard to know, 00:09:51.700 |
but it's really hard to know exactly what those goals are. 00:09:56.800 |
I mean, you could argue it's extremely difficult 00:09:58.800 |
to understand the sources of human frustration 00:10:07.960 |
- There's some things that are gonna be obvious 00:10:10.540 |
So I don't think anybody really can do this well yet, 00:10:14.200 |
but I think it's not inconceivable to imagine machines 00:10:18.200 |
in the not so distant future being able to understand 00:10:22.600 |
that if people lose in a game, that they don't like that. 00:10:31.520 |
and so that makes it relatively easy to code. 00:10:34.600 |
On the other hand, if you wanted to capture everything 00:10:36.820 |
about frustration, well, people can get frustrated 00:10:48.560 |
the harder it is for anything like the existing techniques 00:11:02.600 |
I had two excuses, I'll give you my excuses up front, 00:11:07.000 |
I was jet lagged and I hadn't played in 25 or 30 years, 00:11:10.920 |
but the outcome is he completely destroyed me 00:11:14.360 |
- Have you ever been beaten in any board game by a machine? 00:11:19.360 |
- I have, I actually played the predecessor to Deep Blue, 00:11:29.960 |
- And that was, and after that you realize it's over for us. 00:11:35.280 |
- Well, there's no point in my playing Deep Blue, 00:11:36.800 |
I mean, it's a waste of Deep Blue's computation. 00:11:41.480 |
'cause we both gave lectures at this same event 00:11:46.000 |
I forgot to mention that not only did he crush me, 00:11:47.920 |
but he crushed 29 other people at the same time. 00:11:53.800 |
and emotional experience of being beaten by a machine, 00:11:57.280 |
I imagine, to you who thinks about these things, 00:12:03.520 |
Or no, it was a simple mathematical experience? 00:12:09.720 |
particularly where you have perfect information, 00:12:14.760 |
and there's more computation for the computer, 00:12:18.840 |
I mean, I'm not sad when a computer calculates 00:12:25.240 |
Like, I know I can't win that game, I'm not gonna try. 00:12:28.920 |
- Well, with a system like AlphaGo or AlphaZero, 00:12:32.120 |
do you see a little bit more magic in a system like that, 00:12:35.120 |
even though it's simply playing a board game, 00:12:37.280 |
but because there's a strong learning component? 00:12:42.640 |
'cause Kasparov and I are working on an article 00:12:52.000 |
is that AI is actually a grab bag of different techniques 00:12:56.080 |
or they each have their own unique strengths and weaknesses. 00:13:06.600 |
Well, no, some problems are really accessible 00:13:09.520 |
like chess and Go and other problems like reading 00:13:12.040 |
are completely outside the current technology. 00:13:26.180 |
you know, I wrote a piece recently that they lost 00:13:30.540 |
but they spent $530 million more than they made last year. 00:13:34.900 |
So, you know, they're making huge investments, 00:13:37.860 |
and they have applied the same kinds of techniques 00:13:45.540 |
'cause it's a fundamentally different kind of problem. 00:13:47.900 |
Chess and Go and so forth are closed end problems, 00:13:58.200 |
but fundamentally, you know, the Go board has 361 squares, 00:14:09.120 |
the next sentence could be anything, you know, 00:14:14.440 |
- That's fascinating that you think this way. 00:14:19.680 |
but so I'll play the role of a person who says-- 00:14:22.320 |
- You can put clothes on the emperor, good luck with it. 00:14:24.280 |
- Romanticizes the notion of the emperor, period, 00:14:46.040 |
what's being written and then maybe even more complicated 00:14:53.600 |
I would argue that language is much closer to Go 00:15:01.440 |
When you say the possibility of the number of sentences 00:15:06.440 |
but it nevertheless is much more constrained. 00:15:09.240 |
It feels maybe I'm wrong than the possibilities 00:15:23.240 |
This bottle, I don't know if it would be in the field of view 00:15:28.680 |
and I can use the word on here, maybe not here, 00:15:32.880 |
but that one word encompasses in analog space 00:15:39.280 |
So there is a way in which language filters down 00:15:49.840 |
you have to follow the rules of that grammar. 00:15:52.680 |
but by and large, we follow the rules of grammar. 00:15:57.000 |
So there are ways in which language is a constrained system. 00:16:02.280 |
Let's say there's an infinite number of possible sentences, 00:16:04.880 |
and you can establish that by just stacking them up. 00:16:09.480 |
You think that I think that there's water on the table. 00:16:11.720 |
Your mother thinks that you think that I think 00:16:14.560 |
Your brother thinks that maybe your mom is wrong 00:16:19.320 |
You know, we can make sentences of infinite length, 00:16:23.560 |
This is a very silly example, a very, very silly example, 00:16:26.400 |
a very, very, very, very, very, very silly example, 00:16:32.440 |
In any case, it's vast by any reasonable measure. 00:16:35.800 |
And for example, almost anything in the physical world 00:16:40.480 |
And interestingly, many of the sentences that we understand, 00:16:43.800 |
we can only understand if we have a very rich model 00:16:47.840 |
So I don't ultimately want to adjudicate the debate 00:16:50.640 |
that I think you just set up, but I find it interesting. 00:16:53.440 |
Maybe the physical world is even more complicated 00:17:06.120 |
for linguists, people trying to understand it. 00:17:09.680 |
and that's part of what's driven my whole career. 00:17:15.360 |
why kids couldn't learn language when machines couldn't. 00:17:20.560 |
We're gonna get into communication intelligence 00:17:32.520 |
So you've written in your book, in your new book, 00:17:37.320 |
it would be arrogant to suppose that we could forecast 00:17:47.080 |
What do AI systems with or without physical bodies 00:17:56.800 |
but if you were to philosophize and imagine, do-- 00:18:06.760 |
Like, people figured out how electricity worked. 00:18:09.680 |
They had no idea that that was gonna lead to cell phones. 00:18:19.440 |
they weren't really thinking that cell phones 00:18:23.360 |
- There are, nevertheless, predictions of the future, 00:18:25.720 |
which are statistically unlikely to come to be, 00:18:37.520 |
even though it's most very likely to be wrong. 00:18:42.760 |
We can predict that AI will be faster than it is now. 00:18:49.520 |
It will be better in the sense of being more general 00:18:58.340 |
You know, I mean, these are easy predictions. 00:19:09.840 |
But I can predict that people will never wanna pay 00:19:13.280 |
They're never gonna want it to take longer to get there. 00:19:15.280 |
And you know, so like you can't predict everything, 00:19:18.920 |
Sure, of course it's gonna be faster and better. 00:19:20.960 |
And what we can't really predict is the full scope 00:19:31.960 |
although I'm very skeptical about current AI, 00:19:45.000 |
I mean, I've heard people make those kind of arguments. 00:19:50.480 |
And probably 500 years is plenty to get there. 00:19:55.560 |
And then once it's here, it really will change everything. 00:20:01.100 |
are you talking about human level intelligence? 00:20:16.600 |
like reason flexibly and understand language and so forth. 00:20:21.200 |
But that doesn't mean they have to be identical to humans. 00:20:29.960 |
So they like arguments that seem to support them 00:20:32.480 |
and they dismiss arguments that they don't like. 00:20:35.480 |
There's no reason that a machine should ever do that. 00:20:38.720 |
- So you see that those limitations of memory 00:20:58.960 |
but as AI programmers, but eventually AI will exceed it. 00:21:09.440 |
and do it without some of the flaws that human beings have. 00:21:12.240 |
The other thing I'll say is I wrote a whole book, 00:21:21.440 |
which was about the limits of the human mind. 00:21:24.080 |
Current book is kind of about those few things 00:21:33.320 |
our mortality, our bias is a strength, not a weakness? 00:21:43.540 |
from which motivation springs and meaning springs? 00:21:50.940 |
I think that there's a lot of making lemonade out of lemons. 00:21:55.180 |
So we, for example, do a lot of free association 00:22:02.620 |
And we enjoy that and we make poetry out of it 00:22:04.580 |
and we make kind of movies with free associations 00:22:08.200 |
I don't think that's really a virtue of the system. 00:22:12.360 |
I think that the limitations in human reasoning 00:22:16.640 |
Like, for example, politically, we can't see eye to eye 00:22:30.000 |
'cause we can't interpret the data in shared ways. 00:22:36.520 |
So my free associations are different from yours 00:22:38.960 |
and you're kind of amused by them and that's great. 00:22:42.700 |
So there are lots of ways in which we take a lousy situation 00:22:47.600 |
Another example would be our memories are terrible. 00:22:52.400 |
where you flip over two cards, try to find a pair. 00:22:56.560 |
Computers, like, this is the dullest game in the world. 00:23:02.600 |
Can we make a fun game out of having this terrible memory? 00:23:10.600 |
and optimizing some kind of utility function. 00:23:13.580 |
But you think in general there is a utility function. 00:23:16.320 |
There's an objective function that's better than others. 00:23:24.440 |
- I think you could design a better memory system. 00:23:36.520 |
To get rid of memories that are no longer useful. 00:23:48.880 |
with where you parked the day before and so forth. 00:23:55.400 |
I mean, I've heard all kinds of wacky arguments. 00:24:17.800 |
- Do you think it's possible to build a system, 00:24:20.400 |
so you said human level intelligence is a weird concept. 00:24:23.820 |
- Well, I'm saying I prefer general intelligence. 00:24:32.000 |
I'm saying that per se shouldn't be the objective, 00:24:37.240 |
the things they do well and incorporate that into our AI, 00:24:39.680 |
just as we incorporate the things that machines do well 00:24:45.800 |
can do all this brute force computation that people can't. 00:24:50.840 |
is because I would like to see machines solve problems 00:24:59.480 |
the strengths of machines to do all this computation 00:25:02.240 |
with the ability, let's say, of people to read. 00:25:11.760 |
There's no way for any doctor or whatever to read them all. 00:25:15.440 |
A machine that could read would be a brilliant thing. 00:25:18.000 |
And that would be strengths of brute force computation 00:25:21.100 |
combined with kind of subtlety and understanding medicine 00:25:29.680 |
So, Jan Lekun believes that human intelligence 00:25:38.160 |
We have lots of narrow intelligences for specific problems. 00:25:42.160 |
But the fact is, like, anybody can walk into, 00:25:51.720 |
So, you can reason about what happens in a bank robbery 00:25:58.640 |
and wants to go to IVF to try to have a child. 00:26:02.800 |
Or you can, you know, the list is essentially endless. 00:26:05.960 |
And not everybody understands every scene in a movie. 00:26:11.760 |
that pretty much any ordinary adult can understand. 00:26:24.360 |
the kind of possibilities of experiences that are possible. 00:26:27.360 |
But in fact, the amount of experience that are possible 00:26:35.120 |
that humans are constrained in what they can understand, 00:26:49.840 |
And then I say, can it play on a rectangular board 00:26:53.680 |
And you say, well, if I retrain it from scratch 00:27:01.120 |
We don't have even a system that could play Go 00:27:21.120 |
that you can do all kinds of Go board shapes flexibly. 00:27:25.760 |
- Well, I mean, that would be like a first step 00:27:29.040 |
Obviously, that's not what it really meaning. 00:27:31.360 |
What I mean by general is that you could transfer 00:27:36.120 |
the knowledge you learn in one domain to another. 00:27:38.960 |
So if you learn about bank robberies in movies 00:27:44.780 |
then you can understand that amazing scene in "Breaking Bad" 00:27:52.600 |
And you can reflect on how that car chase scene 00:27:55.520 |
is like all the other car chase scenes you've ever seen 00:28:05.760 |
So the idea of general is you could just do it 00:28:07.320 |
on a lot of, transfer it across a lot of domains. 00:28:10.720 |
are infinitely general or that humans are perfect. 00:28:17.400 |
But right now, the bar is here and we're there 00:28:46.320 |
integrating prior knowledge, causal reasoning, 00:29:07.480 |
in your view has the biggest impact on the AI community? 00:29:18.000 |
So some of them might be solved independently of others, 00:29:28.480 |
So right now we have an approach that's dominant 00:29:31.360 |
where you take statistical approximations of things, 00:29:40.360 |
but you don't understand that there's a thread 00:29:42.280 |
on the bottle cap that fits with the thread on the bottle, 00:29:45.320 |
and that that tightens, and if I tighten enough 00:29:47.800 |
that there's a seal and the water will come out. 00:29:49.640 |
Like there's no machine that understands that. 00:29:57.800 |
then a lot of these other things start to fall 00:30:02.840 |
Right now, you're like learning correlations between pixels 00:30:05.640 |
when you play a video game or something like that. 00:30:11.800 |
and then you alter the video game in small ways, 00:30:13.640 |
like you move the paddle and break out a few pixels, 00:30:19.000 |
it doesn't have a representation of a paddle, 00:30:20.920 |
a ball, a wall, a set of bricks, and so forth. 00:30:31.060 |
but it's nevertheless full of mystery, full of promise. 00:30:38.000 |
So the way you've been discussing it now is very intuitive. 00:30:40.960 |
It makes a lot of sense that that is something 00:30:45.600 |
But the argument could be that we're oversimplifying it 00:30:49.720 |
because we're oversimplifying the notion of common sense 00:30:53.180 |
because that's how we, it feels like we as humans 00:31:05.200 |
one of the things that might come as a surprise to them 00:31:14.080 |
that I like common sense, but that chapter actually starts 00:31:25.480 |
that believe in at least some of what good old-fashioned AI 00:31:28.080 |
try to do, so we believe in symbols and logic 00:31:30.720 |
and programming, things like that are important. 00:31:37.040 |
that we hold fairly dear aren't really enough. 00:31:39.560 |
So we talk about why common sense is actually many things. 00:31:46.560 |
So things like taxonomy, so I know that a bottle 00:31:54.480 |
and objects are material things in the physical world. 00:32:00.480 |
If I know that vessels need to not have holes in them, 00:32:05.480 |
then I can infer that in order to carry their contents 00:32:09.540 |
then I can infer that a bottle shouldn't have a hole 00:32:12.840 |
So you can do hierarchical inference and so forth. 00:32:15.600 |
And we say that's great, but it's only a tiny piece 00:32:21.120 |
We give lots of examples that don't fit into that. 00:32:23.440 |
So another one that we talk about is a cheese grater. 00:32:29.520 |
You can build a model in the game engine sense of a model 00:32:33.400 |
so that you could have a little cartoon character 00:32:35.760 |
flying around through the holes of the grater, 00:32:41.620 |
that really understands why the handle is on top 00:32:47.620 |
or how you'd hold the cheese with respect to the grater 00:32:52.120 |
- Do you think these ideas are just abstractions 00:32:59.920 |
- I'm a skeptic that that kind of emergence per se can work. 00:33:03.120 |
So I think that deep learning might play a role 00:33:05.840 |
in the systems that do what I want systems to do, 00:33:29.640 |
which Jan Lekun is famous for, which is an abstraction. 00:33:34.960 |
So the abstraction is an object looks the same 00:33:41.960 |
essentially why he was a co-winner of the Turing Award 00:33:47.620 |
then your system would be a whole lot more efficient. 00:33:53.200 |
but people don't have systems that kind of reify things 00:34:00.400 |
if you don't program that in advance as a system, 00:34:02.720 |
it kind of realizes that this is the same thing as this, 00:34:26.080 |
with that brilliant idea, can get you a Turing Award, 00:34:34.780 |
and something we'll talk about, the expert system, 00:34:40.020 |
So it feels like one, there's a huge amount of limitations 00:34:43.480 |
which you clearly outline with deep learning, 00:34:49.580 |
it does it, it does a lot of stuff automatically 00:34:54.900 |
- Well, and that's part of why people love it, right? 00:34:57.100 |
But I always think of this quote from Bertrand Russell, 00:35:08.140 |
a notion of causality, or even how a bottle works, 00:35:14.260 |
45-page academic paper trying just to understand 00:35:21.100 |
but it's a very detailed analysis of all the things, 00:35:33.180 |
but Ernie did the hard work for that particular paper. 00:35:42.820 |
It's a way to do it, but on that way of doing it, 00:35:55.580 |
Everybody would rather just feed their system in 00:35:58.340 |
with a bunch of videos with a bunch of containers, 00:36:00.300 |
and have the systems infer how containers work. 00:36:14.580 |
that in a robust way can actually watch videos 00:36:18.700 |
and predict exactly which containers would leak 00:36:21.300 |
and which ones wouldn't or something like that. 00:36:23.540 |
And I know someone's gonna go out and do that 00:36:25.060 |
since I said it, and I look forward to seeing it. 00:36:38.820 |
should go into defining an unsupervised learning algorithm 00:36:43.180 |
that will watch videos, use the next frame, basically, 00:36:57.820 |
My intuition, based on years of watching this stuff 00:37:01.740 |
and making predictions 20 years ago that still hold, 00:37:03.940 |
even though there's a lot more computation and so forth, 00:37:06.500 |
is that we actually have to do a different kind of hard work, 00:37:08.520 |
which is more like building a design specification 00:37:15.060 |
how we do things like what Jan did for convolution 00:37:22.580 |
The current systems don't have that much knowledge 00:37:30.540 |
and having the same perception, I guess I'll say, 00:37:38.260 |
They don't see how to naturally fit one with the other. 00:37:45.540 |
there's a temptation to go too far the other way, 00:37:47.620 |
so it's just having an expert sort of sit down 00:37:56.540 |
From my view, one really exciting possibility 00:37:59.220 |
is of active learning where it's continuous interaction 00:38:04.080 |
As the machine, there's kind of deep learning type 00:38:07.060 |
extraction of information from data, patterns, and so on, 00:38:10.120 |
but humans also guiding the learning procedures, 00:38:19.940 |
of how the machine learns, whatever the task is. 00:38:22.100 |
- I was with you with almost everything you said 00:38:30.500 |
So let's remember, deep learning is a particular way 00:38:38.820 |
There are other things you can do with deep learning, 00:38:44.620 |
is I have a lot of examples and I have labels for them. 00:38:47.600 |
So here are pictures, this one's the Eiffel Tower, 00:38:53.320 |
this one's a cat, this one's a pig, and so forth. 00:38:55.180 |
You just get millions of examples, millions of labels. 00:39:04.440 |
but it is not good at representing abstract knowledge. 00:39:09.380 |
like bottles contain liquid and have tops to them 00:39:17.840 |
It is an example of having a machine learn something, 00:39:21.300 |
but it's a machine that learns a particular kind of thing, 00:39:27.760 |
for learning about the abstractions that govern our world. 00:39:34.300 |
is maybe people should be working on devising such things. 00:39:40.580 |
is deep neural networks do form abstractions, 00:39:56.500 |
which are as powerful as our human abstractions 00:40:10.640 |
but I think the answer is at least partly no. 00:40:13.100 |
One of the kinds of classical neural network architectures 00:40:35.880 |
It's like binary one, one, and a bunch of zeros. 00:40:41.640 |
with the precursors of contemporary deep learning. 00:40:48.600 |
you could train these networks on all the even numbers, 00:40:52.200 |
and they would never generalize to the odd number. 00:40:54.800 |
A lot of people thought that I was, I don't know, 00:41:00.240 |
But it is true that with this class of networks 00:41:05.000 |
that they would never, ever make this generalization. 00:41:14.840 |
what is the probability that the rightmost output node 00:41:21.320 |
in everything they'd ever been trained on, it was a zero. 00:41:27.120 |
And so they figured, well, I turned it on now. 00:41:29.060 |
Whereas a person would look at the same problem and say, 00:41:45.460 |
I can tell you if y is three, then x is five. 00:41:49.200 |
And now I can do it with some totally different number, 00:41:51.880 |
Then you can say, well, obviously it's a million and two, 00:41:57.520 |
And deep learning systems kind of emulate that, 00:42:04.200 |
you can fudge a solution to that particular problem. 00:42:20.320 |
They're not structured over these operations over variables. 00:42:23.120 |
Now, someday people may do a new form of deep learning 00:42:34.320 |
But the sort of classic stuff like people use for ImageNet 00:42:38.840 |
And you have people like Hinton going around saying, 00:42:52.040 |
'cause we really do need to have the gasoline engine stuff 00:42:55.960 |
that represents, I mean, I don't think it's a good analogy, 00:43:06.240 |
that we do need to throw out everything and start over. 00:43:25.400 |
You can't be like, I don't know what to throw out, 00:43:32.120 |
but the variables and the operations over variables. 00:43:37.760 |
and which John McCarthy did when he founded AI, 00:43:41.520 |
that stuff is the stuff that we build most computers out of. 00:43:45.400 |
"We don't need computer programmers anymore." 00:43:54.440 |
And most of them, they do a little bit of machine learning, 00:44:04.520 |
the conditionals and comparing operations over variables. 00:44:08.080 |
Like there's this fantasy you can machine learn anything. 00:44:10.200 |
There's some things you would never wanna machine learn. 00:44:17.760 |
and you recorded which packets were transmitted 00:44:22.480 |
Or to build a web browser by taking logs of keystrokes 00:44:29.480 |
and then trying to learn the relation between them. 00:44:37.440 |
the stuff that I think AI needs to avail itself of 00:44:53.880 |
- Sure, so I mean, first I just wanna clarify, 00:45:06.480 |
like medical knowledge with a large set of rules. 00:45:09.440 |
So if the patient has this symptom and this other symptom, 00:45:12.800 |
then it is likely that they have this disease. 00:45:16.820 |
and they were symbol manipulating rules of just the sort 00:45:33.920 |
but the difference is those guys did in the 80s 00:45:39.980 |
almost entirely handwritten with no machine learning. 00:45:44.300 |
is almost entirely one species of machine learning 00:45:48.240 |
And what I'm counseling is actually a hybrid. 00:45:50.320 |
I'm saying that both of these things have their advantage. 00:45:52.880 |
So if you're talking about perceptual classification, 00:45:57.080 |
Deep learning is the best tool we've got right now. 00:46:04.120 |
is probably still the best available alternative. 00:46:18.600 |
of both the expert systems and the deep learning, 00:46:21.040 |
but are gonna find new ways to synthesize them. 00:46:23.240 |
- How hard do you think it is to add knowledge 00:46:30.460 |
to add extra information to symbol manipulating systems? 00:46:40.120 |
Partly because a lot of the things that are important, 00:46:46.100 |
So if you pay someone on Amazon Mechanical Turk 00:47:02.160 |
You know, they're gonna tell you more exotic things, 00:47:08.940 |
but they're not getting to the root of the problem. 00:47:12.460 |
So untutored humans aren't very good at knowing, 00:47:19.680 |
the computer system developers actually need. 00:47:23.460 |
I don't think that that's an irremediable problem. 00:47:32.060 |
There's one at MIT, we're recording this at MIT, 00:47:50.100 |
So they're like, go to the room with the television 00:48:06.860 |
which is like, I wanna fit a certain number of exercises 00:48:12.240 |
I mean, you want some kind of abstract description. 00:48:15.060 |
The fact that you happen to press the remote control 00:48:20.020 |
isn't really the essence of the exercise routine. 00:48:23.060 |
But if you just ask people like, what did they do? 00:48:31.900 |
in order to craft the right kind of knowledge. 00:48:39.340 |
or at least we're not able to communicate it effectively. 00:48:43.300 |
- Yeah, most of it we would recognize if somebody said it, 00:48:47.440 |
But we wouldn't think to say that it's true or not. 00:48:49.660 |
- It's a really interesting mathematical property. 00:48:59.940 |
but we're unlikely to retrieve it in the reverse. 00:49:07.220 |
I would say there's a huge ocean of that knowledge. 00:49:18.780 |
I'll give you an asterisk on this in a second, 00:49:28.640 |
One is like, could you build it into a machine? 00:49:34.480 |
that we could go download and stick into our machine? 00:49:45.040 |
You know, the closest, and this is the asterisk, 00:50:00.200 |
tried to hand code it, there are various issues, 00:50:10.640 |
- Why do you think there's not more excitement/money 00:50:16.400 |
- There was, people view that project as a failure. 00:50:22.060 |
of a specific instance that was conceived 30 years ago 00:50:28.140 |
So, you know, in 2010, people had the same attitude 00:50:32.760 |
They're like, this stuff doesn't really work. 00:50:35.500 |
And, you know, all these other algorithms work better 00:50:38.620 |
and so forth, and then certain key technical advances 00:50:43.780 |
of graphics processing units that changed that. 00:50:46.400 |
It wasn't even anything foundational in the techniques. 00:50:51.240 |
but mostly it was just more compute and more data, 00:50:55.280 |
things like ImageNet that didn't exist before, 00:50:59.040 |
And it could be, to work, it could be that, you know, 00:51:02.200 |
Psyche just needs a few more things or something like Psyche 00:51:05.480 |
but the widespread view is that that just doesn't work. 00:51:08.840 |
And people are reasoning from a single example. 00:51:16.580 |
and there were many, many efforts in deep learning 00:51:26.660 |
that has any commercial value whatsoever at this point. 00:51:35.980 |
and he said, I had a company too, I was talking about, 00:51:45.640 |
And the problem was he did it in 1986 or something like that. 00:51:51.060 |
We didn't have the tools then, not the algorithms. 00:51:53.840 |
You know, his algorithms weren't that different 00:51:55.400 |
from modern algorithms, but he didn't have the GPUs 00:52:01.320 |
It could be that, you know, symbol manipulation per se 00:52:14.440 |
My perspective on it is not that we want to resuscitate 00:52:19.320 |
that stuff per se, but we want to borrow lessons from it, 00:52:21.480 |
bring together with other things that we've learned. 00:52:28.200 |
and there'll be an explosion of symbol manipulation efforts. 00:52:33.640 |
Paul Allen's AI Institute, are trying to do that. 00:52:39.360 |
they're not doing it for quite the reason that you said, 00:52:43.240 |
that at least spark interest in common sense reasoning. 00:52:53.280 |
Rich Sutton wrote a blog post titled "Bitter Lesson." 00:52:57.240 |
but he said that the biggest lesson that can be read 00:52:59.920 |
from 70 years of AI research is that general methods 00:53:03.040 |
that leverage computation are ultimately the most effective. 00:53:06.320 |
Do you think that-- - The most effective of what? 00:53:14.520 |
and for some reinforcement learning problems. 00:53:22.840 |
but I would also say they have been most effective 00:53:26.400 |
generally because everything we've done up to-- 00:53:47.140 |
but has there been something truly successful 00:54:11.400 |
So I don't think classical AI was wildly successful, 00:54:19.240 |
Nobody even notices them 'cause they're so pervasive. 00:54:21.920 |
So there are some successes for classical AI. 00:54:26.640 |
I think deep learning has been more successful, 00:54:33.160 |
is just because you can build a better ladder 00:54:34.880 |
doesn't mean you can build a ladder to the moon. 00:54:39.440 |
if you have a perceptual classification problem, 00:54:42.320 |
throwing a lot of data at it is better than anything else. 00:54:45.840 |
But that has not given us any material progress 00:54:56.320 |
Problems like that, there's no actual progress there. 00:54:59.520 |
- So flip side of that, if we remove data from the picture, 00:55:05.880 |
a very simple algorithm and you wait for compute to scale. 00:55:19.160 |
do you think compute can unlock some of the things 00:55:21.760 |
with either deep learning or symbol manipulation that-- 00:55:37.480 |
- Exactly, there's diminishing returns on more money, 00:55:42.640 |
Except maybe the people who signed the giving pledge, 00:55:49.720 |
But the rest of us, if you wanna give me more money, fine. 00:55:52.560 |
- You say more money, more problems, but okay. 00:55:55.600 |
What I would say to you is your brain uses like 20 watts, 00:56:00.160 |
and it does a lot of things that deep learning doesn't do, 00:56:07.080 |
So it's an existence proof that you don't need 00:56:29.160 |
how, what have you learned about AI from having-- 00:56:38.240 |
I've learned a lot by watching my two intelligent agents. 00:56:41.240 |
I think that what's fundamentally interesting, 00:56:48.720 |
is the way that they set their own problems to solve. 00:56:58.240 |
and they're constantly creating new challenges. 00:57:03.800 |
They're like, well, what if this, or what if that, 00:57:10.360 |
So they're doing these what if scenarios all the time. 00:57:14.080 |
And that's how they learn something about the world 00:57:17.560 |
and grow their minds, and machines don't really do that. 00:57:23.680 |
And you've talked about this, you've written about it, 00:57:25.280 |
you've thought about it, nature versus nurture. 00:57:28.600 |
So what innate knowledge do you think we're born with, 00:57:38.340 |
- Can I just say how much I like that question? 00:57:40.680 |
You phrased it just right, and almost nobody ever does, 00:57:53.480 |
when it is obviously has to be nature and nurture. 00:58:05.920 |
And so many people get that wrong, including in the field. 00:58:12.320 |
the learning side, I must not be allowed to work 00:58:15.360 |
on the innate side, or that will be cheating. 00:58:25.240 |
I've talked to folks who studied the development 00:58:27.200 |
of the brain, and I mean, the growth of the brain 00:58:30.720 |
in the first few days, in the first few months, 00:58:39.560 |
So that process of development from a stem cell 00:58:42.360 |
to the growth of the central nervous system and so on, 00:58:52.320 |
so all of that comes into play, and it's unclear. 00:58:55.360 |
It's not just whether it's a dichotomy or not, 00:58:57.360 |
it's where most, or where the knowledge is encoded. 00:59:02.100 |
So what's your intuition about the innate knowledge, 00:59:11.380 |
- One of my earlier books was actually trying 00:59:15.880 |
Like, how is it the genes even build innate knowledge? 00:59:21.480 |
we're having today, there's actually two questions. 00:59:43.040 |
and 3D geometry and all of this kind of stuff. 00:59:57.360 |
And then there's a question of what AI should have. 01:00:01.640 |
But I would say that it's a pretty interesting 01:00:08.760 |
that allows us to do a lot of interesting things. 01:00:10.600 |
So I would argue or guess, based on my reading 01:00:18.080 |
that children are born with a notion of space, 01:00:30.260 |
No certain causation, if I didn't just say that. 01:00:35.640 |
They're like frameworks for learning the other things. 01:00:58.180 |
So I think it's an interesting open question, 01:01:06.360 |
in the way that the interfaces of a software package 01:01:18.320 |
because a lot of the things that we reason about 01:01:20.720 |
are the relations between space and time and cause. 01:01:26.440 |
about what's gonna happen with the bottle cap 01:01:32.560 |
If the cap is over here, I get a different outcome. 01:01:35.720 |
If the timing is different, if I put this here, 01:01:38.560 |
after I move that, then I get a different outcome. 01:01:43.040 |
So obviously these mechanisms, whatever they are, 01:01:49.920 |
- So I think evolution had a significant role 01:01:53.160 |
to play in the development of this whole colluge, right? 01:01:59.200 |
- Oh, it's terribly inefficient, except that-- 01:02:05.720 |
It's inefficient except that once it gets a good idea, 01:02:28.440 |
So fish have it and dogs have it and we have it. 01:02:31.660 |
We have adaptations of it and specializations of it. 01:02:34.080 |
But, and the same thing with a primate brain plan. 01:02:37.120 |
So monkeys have it and apes have it and we have it. 01:02:41.080 |
So there are additional innovations like color vision 01:02:45.840 |
So it takes evolution a long time to get a good idea, 01:02:48.840 |
but, and I'm being anthropomorphic and not literal here, 01:02:55.560 |
which caches out into one set of genes or in the genome, 01:03:02.640 |
I guess is the word people might use nowadays 01:03:05.640 |
They're libraries that get used over and over again. 01:03:08.760 |
So once you have the library for building something 01:03:11.720 |
with multiple digits, you can use it for a hand, 01:03:20.640 |
which means that the speed over time picks up. 01:03:25.560 |
because you have bigger and bigger libraries. 01:03:35.320 |
start with libraries that are very, very minimal, 01:03:40.320 |
like almost nothing, and then progress is slow 01:03:44.240 |
and it's hard for someone to get a good PhD thesis out of it 01:03:52.580 |
that had an originate structure to begin with, 01:03:56.800 |
- Or more PhD students, if the evolutionary process 01:03:59.920 |
is indeed in a meta way, runs away with good ideas, 01:04:06.720 |
pool of ideas in order for it to discover one 01:04:10.240 |
And PhD students representing individual ideas as well. 01:04:16.200 |
- Yeah, the monkeys are typewriters with Shakespeare, yeah. 01:04:19.160 |
- Well, I mean, those aren't cumulative, right? 01:04:26.720 |
So if you have a billion monkeys independently, 01:04:33.760 |
and I think Dawkins made this point originally, 01:04:37.560 |
in either "Selfish Teen" or "Blind Watchmaker". 01:04:55.600 |
- Do you think something like the process of evolution 01:05:10.560 |
which distribute the load across a horizontal surface. 01:05:14.200 |
A good engineer could come up with that idea. 01:05:16.960 |
I mean, sometimes good engineers come up with ideas 01:05:25.960 |
We should look at the biology of thought and understanding. 01:05:32.520 |
intuitively reason about physics or other agents. 01:05:39.640 |
If we could understand, at my college we joked dognition. 01:05:46.280 |
and how it was implemented, that might help us with our AI. 01:05:53.780 |
that the kind of timescale that evolution took 01:06:00.520 |
Or can we significantly accelerate that process 01:06:04.020 |
- I mean, I think the way that we accelerate that process 01:06:09.720 |
Not slavishly, but I think we look at how biology 01:06:15.680 |
does that inspire any engineering solutions here? 01:06:22.360 |
- Yeah, I mean, there's a field called biomimicry 01:06:25.000 |
and people do that for like material science all the time. 01:06:28.960 |
We should be doing the analog of that for AI. 01:06:34.440 |
is to look at cognitive science or the cognitive sciences, 01:06:53.360 |
And my hope is that Francois is actually gonna take, 01:06:59.920 |
I just don't have a place in my busy life at this moment. 01:07:03.460 |
But the notion is that there'll be many tests 01:07:06.420 |
and not just one because intelligence is multifaceted. 01:07:17.320 |
the SAT has a verbal component and a math component 01:07:21.320 |
And Howard Gardner has talked about multiple intelligence, 01:07:23.640 |
like kinesthetic intelligence and verbal intelligence 01:07:27.740 |
There are a lot of things that go into intelligence 01:07:34.680 |
has developed a very specific kind of intelligence. 01:07:37.200 |
And then there are people that are generalists. 01:07:43.360 |
which doesn't mean I know anything about quantum mechanics, 01:07:45.600 |
but I know a lot about the different facets of the mind. 01:07:57.440 |
There are people that are much better at that than I am. 01:08:00.120 |
- Sure, but what would be really impressive to you? 01:08:07.040 |
especially if somebody like Francois is running it. 01:08:28.580 |
a kind of comprehension that relates to what you just said. 01:08:30.680 |
So I wrote a piece in the New Yorker in I think 2015, 01:08:34.980 |
right after Eugene Guzman, which was a software package, 01:08:54.460 |
is you're evasive, you pretend to have limitations 01:08:58.020 |
so you don't have to answer certain questions and so forth. 01:09:00.620 |
So this particular system pretended to be a 13 year old boy 01:09:08.140 |
and wouldn't answer your questions and so forth. 01:09:09.740 |
And so judges got fooled into thinking briefly 01:09:12.540 |
with very little exposure, he was a 13 year old boy, 01:09:18.460 |
how do you make the machine actually intelligent? 01:09:22.140 |
And so in the New Yorker, I proposed an alternative, 01:09:31.060 |
'cause I've already given you one Breaking Bad example, 01:09:37.660 |
you should be able to watch an episode of Breaking Bad, 01:09:46.980 |
Right, so if you could answer kind of arbitrary questions 01:09:55.380 |
They could watch a film or there are different versions. 01:09:58.540 |
And so ultimately I wrote this up with Praveen Paritosh 01:10:04.060 |
that basically was about the Turing Olympics. 01:10:11.700 |
was trying to figure out like how we would actually run it. 01:10:17.340 |
or you could have an auditory podcast version, 01:10:23.740 |
if you can do, let's say, human level or better than humans 01:10:29.500 |
You know, why did this person pick up the stone? 01:10:31.580 |
What were they thinking when they picked up the stone? 01:10:36.180 |
And I mean, ideally these wouldn't be multiple choice either 01:10:38.620 |
because multiple choice is pretty easily gamed. 01:10:41.060 |
So if you could have relatively open-ended questions 01:10:44.140 |
and you can answer why people are doing this stuff, 01:11:07.700 |
"I am Spartacus," you know, this famous scene. 01:11:14.140 |
that everybody or everybody minus one has to be lying. 01:11:21.820 |
to know they couldn't all have the same name. 01:11:32.380 |
They can say, "This is why these guys all got up 01:11:52.860 |
with essentially arbitrary films from a large set. 01:11:56.580 |
because it's possible such a system would discover 01:12:09.140 |
boy meets girl, boy loses girl, boy finds girl. 01:12:17.980 |
or you could have your system watch a lot of films. 01:12:26.260 |
But even if you could do it for all Westerns, 01:12:35.860 |
because you've put so many interesting ideas out there 01:12:56.900 |
So do you think that way often as a scientist? 01:13:03.020 |
that deep learning could actually run away with it? 01:13:22.340 |
and they will relabel their hybrids as deep learning. 01:13:25.820 |
So AlphaGo is often described as a deep learning system, 01:13:29.580 |
but it's more correctly described as a system 01:13:31.700 |
that has deep learning, but also Monte Carlo Tree Search, 01:13:45.780 |
that's a branding question, and that's a giant mess. 01:13:59.260 |
One is a guy at DeepMind thought he had finally outfoxed me. 01:14:06.940 |
And he said, he specifically made an example. 01:14:16.420 |
that is so smart that OpenAI couldn't release it 01:14:26.060 |
and my example was something like a rose is a rose, 01:14:34.060 |
And I wrote back and I said, "That's impressive, 01:14:43.260 |
Which was part of what I was talking about in 1998 01:14:49.420 |
And he sheepishly wrote back about 20 minutes later. 01:14:53.060 |
And the answer was, "Well, it had some problems with those." 01:14:55.380 |
So I made some predictions 21 years ago that still hold. 01:15:00.380 |
In the world of computer science, that's amazing, right? 01:15:02.700 |
Because there's a thousand or a million times more memory 01:15:15.340 |
And there's been advances in replacing sigmoids 01:15:25.420 |
but the fundamental architecture hasn't changed 01:15:35.260 |
And the book went to press before GPT-2 came out, 01:15:42.300 |
and all the inferences that you make in this story 01:15:48.260 |
And for fun, in the "Wired" piece, we ran it through GPT-2. 01:15:55.460 |
and your viewers can try this experiment themselves. 01:16:06.420 |
with the conceptual underpinnings of the story. 01:16:16.660 |
I was just updating their claim for a slightly new text. 01:16:31.300 |
- So 20 years ago, you said the emperor has no clothes. 01:16:42.340 |
- And we found out some things to do with naked emperors. 01:16:50.860 |
And so, I mean, they are great at speech recognition, 01:16:56.420 |
'cause I didn't literally say the emperor has no clothes. 01:17:04.340 |
But I said, if you wanna build a neural model 01:17:10.380 |
you're gonna have to change the architecture. 01:17:19.100 |
but you're also very optimistic and a dreamer 01:17:27.860 |
people overselling technology in the short run 01:17:39.260 |
with an optimistic chapter, which kind of killed Ernie, 01:17:44.420 |
He describes me as a contrarian and him as a pessimist. 01:17:47.620 |
But I persuaded him that we should end the book 01:17:57.340 |
and so forth, the things that we counseled for. 01:17:59.660 |
And we wrote it, and it's an optimistic chapter 01:18:02.140 |
that AI suitably reconstructed so that we could trust it, 01:18:05.900 |
which we can't now, could really be world-changing. 01:18:09.500 |
- So, on that point, if you look at the future trajectories 01:18:13.100 |
of AI, people have worries about negative effects of AI, 01:18:21.060 |
or smaller, short-term scale of negative impact on society. 01:18:27.140 |
How can we build AI systems that align with our values, 01:18:32.820 |
that we can interact with, that we can trust? 01:18:34.740 |
- The first thing we have to do is to replace 01:18:44.620 |
and doesn't understand concepts like bottles or harm. 01:18:54.060 |
And you can quibble about the details of Asimov's laws, 01:18:56.860 |
but we have to, if we're gonna build real robots 01:19:04.260 |
That means we have to have these more abstract ideas 01:19:06.620 |
that deep learning's not particularly good at. 01:19:12.380 |
about probabilities of given harms or whatever, 01:19:17.420 |
that a bottle isn't just a collection of pixels. 01:19:20.660 |
- And also be able to, you're implying that you need 01:19:24.460 |
to also be able to communicate that to humans. 01:19:26.900 |
So the AI systems would be able to prove to humans 01:19:31.660 |
that they understand that they know what harm means. 01:19:38.660 |
So we probably need to have committees of wise people, 01:19:47.540 |
And we shouldn't just leave it to software engineers. 01:20:00.300 |
But, you know, there should be some assembly of wise people, 01:20:06.140 |
that tries to figure out what the rules ought to be, 01:20:11.580 |
You can argue, or code or neural networks or something. 01:20:27.100 |
and we decide that Asimov's first law is actually right. 01:20:34.060 |
and so we've represented a sample of the world 01:20:40.500 |
okay, Asimov's first law is actually pretty good. 01:20:53.300 |
into a computer program or a neural network or something. 01:21:00.300 |
is that we just don't know how to do that yet. 01:21:03.580 |
if we think that we can build trustworthy AI. 01:21:19.980 |
And if we can't, then we should be thinking really hard, 01:21:27.980 |
to make job interviews or to do surveillance, 01:21:31.100 |
not that I personally want to do that, or whatever. 01:21:33.800 |
in ways that have practical impact on people's lives, 01:21:42.860 |
is that a lot of people in the deep learning community, 01:21:50.260 |
just people in all general groups and walks of life, 01:21:53.260 |
have different levels of misunderstanding of AI. 01:22:23.560 |
was to inspire a future generation of students 01:22:25.680 |
to solve what we think are the important problems. 01:22:31.240 |
where we think effort would most be rewarded. 01:22:36.720 |
who talk about AI, but aren't experts in the field 01:22:41.000 |
to understand what's realistic and what's not. 01:22:48.400 |
So like number one is if somebody talks about something, 01:22:58.400 |
So if, we don't have this example in the book, 01:23:13.080 |
I'm alluding to Google Duplex, when it was announced, 01:23:22.240 |
- And I'm not gonna ask your thoughts about Sophia. 01:23:24.560 |
But yeah, I understand that's a really good question 01:23:30.200 |
- Sophia has very good material written for her, 01:23:32.560 |
but she doesn't understand the things that she's saying. 01:23:38.200 |
on the science of learning, which I think is fascinating. 01:23:40.520 |
But the learning case studies of playing guitar. 01:23:57.120 |
But I'll say that my favorite rock song to listen to 01:24:07.040 |
I've been trying to put it on YouTube myself, singing. 01:24:11.280 |
If you could party with a rock star for a weekend, 01:24:15.240 |
And pick their mind, it's not necessarily about the party. 01:24:22.720 |
I guess John Lennon's such an intriguing person. 01:24:27.160 |
I mean, I think a troubled person, but an intriguing one. 01:24:32.480 |
- Well, Imagine is one of my favorite songs, so.