back to indexEthics for Data Science
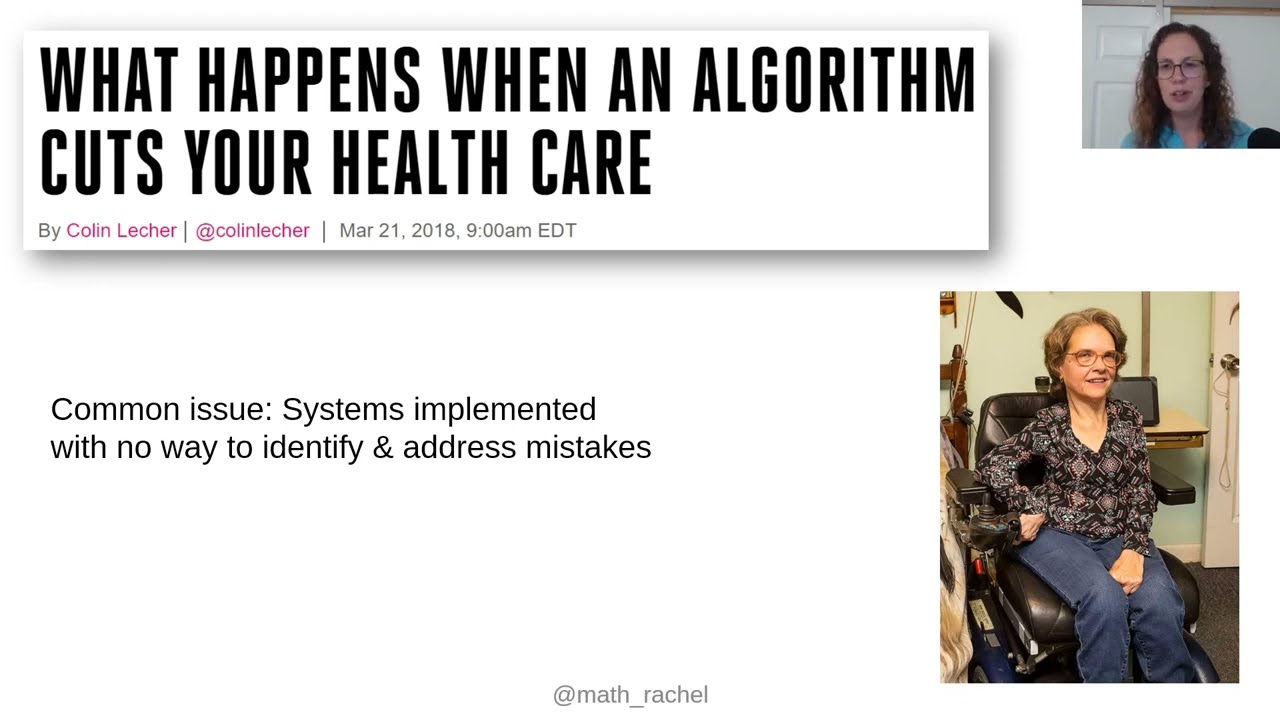
Chapters
0:0 Introduction
0:50 Ethics Issues
1:20 Feedback Loops
2:46 Case Study 2
4:4 Case Study 3
7:30 Questions
7:57 Unintended Consequences
8:11 Volkswagen
8:43 Ethics
10:54 Tech ethics syllabi
13:1 Core topics
16:13 Data containing errors
20:7 Feedback loops metrics
22:21 SAE grading software
24:4 Ill Go Transparency
25:40 Russia Today
29:42 Platform is the Message
31:11 Disinformation
31:36 Blitzscaling
32:28 Data Ethics
37:26 Bias
41:2 Privacy
46:1 Compass recidivism algorithm
50:3 Measurement bias
53:4 Are people biased
56:8 Algorithms in practice
00:00:00.000 |
I'm Rachel Thomas, I am the founding director of the Center for Applied Data Ethics at the University of San Francisco and also co-founder of FAST AI together with Jeremy Howard. 00:00:10.000 |
My background, I have a PhD in math and worked as a data scientist and software engineer in the tech industry and then have been working at USF on FAST AI for the past four years now. 00:00:26.000 |
So ethics issues are in the news. These articles I think are all from this fall, kind of showing up at this intersection of how technology is impacting our world in many kind of increasingly powerful ways, many of which really raise concerns. 00:00:45.000 |
And I want to start by talking about three cases that I hope everyone working in technology knows about and is on the lookout for. So even if you only watch five minutes of this video, these are kind of the three cases I want you to see. 00:00:57.000 |
And one is feedback loops. And so feedback loops can occur whenever your model is controlling the next round of data you get. 00:01:05.000 |
So the data that's returned quickly becomes flawed by the software itself and this can show up in many places. One example is with recommendation systems. 00:01:15.000 |
And so recommendation systems are ostensibly about predicting what content the user will like, but they're also determining what content the user is even exposed to and helping determine what has a chance of becoming popular. 00:01:29.000 |
And so YouTube has gotten a lot of a lot of tension about this for kind of highly recommending many conspiracy theories, many kind of very damaging conspiracy theories. 00:01:42.000 |
There is also they've kind of put together recommendations of pedophilia picked out of what were kind of innocent home movies, but when are kind of strung together, ones that happen to have young girls in bathing suits or in their pajamas. 00:01:59.000 |
So there's some really, really concerning results. And this is not something that any anybody intended. And we'll talk about this more later. 00:02:07.000 |
I think particularly for many of us coming from a science background, we're often used to thinking of like, oh, you know, like we observe the data. 00:02:13.000 |
But really, whenever you're building products that interact with the real world, you're also kind of controlling what the data looks like. 00:02:22.000 |
Second case study I want everyone to know about comes from software that's used to determine poor people's health benefits. It's used in over half of the 50 states. 00:02:34.000 |
And the Verge did an investigation on what happened when it was rolled out in Arkansas. And what happened is there was a bug in the software implementation that incorrectly cut coverage for people with cerebral palsy or diabetes, including Tammy Dobbs, who's pictured here and was interviewed in the article. 00:02:51.000 |
And so these are people that really needed this health care. And it was erroneously cut due to this bug. And so they were really and they couldn't get any sort of explanation. 00:03:03.000 |
And there was no appeals or recourse process in place. And eventually, this all came out through a lengthy court case. 00:03:09.000 |
But it's something where it caused a lot of a lot of suffering in the meantime. And so it's really important to implement systems with a way to identify and address mistakes and to do that quickly. 00:03:20.000 |
And in a way that hopefully minimizes damage because we all know software can have bugs. Our code can behave in unexpected ways. And we need to be prepared for that. 00:03:32.000 |
I wrote more about this idea in a post two years ago, what HBR gets wrong about algorithms and bias. 00:03:40.000 |
And then third case study that everyone should know about. So this is Latonya Sweeney, who's director of the data privacy lab at Harvard. She has a PhD in computer science. 00:03:50.000 |
And she noticed several years ago that when you Google her name, you would get these ads saying Latonya Sweeney arrested, implying that she has a criminal record. 00:04:00.000 |
She's the only Latonya Sweeney and she has never been arrested. She paid $50 to the background check company and confirmed that she's never been arrested. 00:04:08.000 |
She tried Googling some other names and she noticed, for example, Kristin Lindquist got much more neutral ads that just say we found Kristin Lindquist, even though Kristin Lindquist has been arrested three times. 00:04:20.000 |
And so being a computer scientist, Dr. Sweeney to study this very systematically, she looked at over 2000 names and found that this pattern held in which disproportionately African American names were getting these ads suggesting that the person had a criminal record regardless of whether they did. 00:04:38.000 |
And traditionally European American or white names were getting more neutral ads. And this problem of kind of bias in advertising shows up a ton. Advertising is kind of the profit model for most of the major tech platforms. 00:04:56.000 |
And it kind of continues to pop up in high impact ways. Just last year there was research showing how Facebook's ad system discriminates even when the person placing the ad is not trying to do so. 00:05:09.000 |
So for instance, the same housing ad, exact same text, if you change the photo between a white family and a black family, it's served to very different audiences. And so this is something that can really impact people when they're looking for housing, when they're applying for jobs and is a definite area of concern. 00:05:30.000 |
So now I want to kind of step back and ask why does this matter? And so a very kind of extreme example is just that data collection has played a pivotal role in several genocides, including the Holocaust. 00:05:45.000 |
And so this is a photo of Adolf Hitler meeting with the CEO of IBM at the time. I think this photo is taken in 1937. And IBM continued to partner with the Nazis kind of long past when many other companies broke their ties. 00:06:02.000 |
They produced computers that were used in concentration camps to code, whether people were Jewish, how they were executed. And this is also different from now where you might sell somebody a computer and they never hear from them again. 00:06:17.000 |
These machines require a lot of maintenance and kind of ongoing relationship with vendors to kind of upkeep and repair them. And it's something that a Swiss judge ruled. 00:06:27.000 |
It does not seem unreasonable to deduce that IBM's technical assistance facilitated the task of the Nazis in the commission of their crimes against humanity acts also involving accountancy and classification by IBM machines and utilized in the concentration camps themselves. 00:06:42.000 |
I'm told that they haven't gotten around to apologizing yet. 00:06:45.000 |
Oh, that's terrible too. Yeah. Okay. Yeah. And so this is a very kind of a very sobering example, but I think it's important to keep in mind kind of what can go wrong and how technology can be used for for harm for very, very terrible harm. 00:07:06.000 |
And so this just kind of raises a question questions that we all need to grapple with of how would you feel if you discovered that you had been part of a system that ended up hurting society, would you would you even know, would you be open to finding out kind of how how things you had built may have been harmful. 00:07:24.000 |
And how can you help make sure this doesn't happen. And so I think these are questions that we all all need to grapple with. 00:07:33.000 |
It's also important to think about unintended consequences on how your tech could be used or misused, whether that's by harassers by authoritarian governments for propaganda or disinformation. 00:07:47.000 |
And then on a kind of a more concrete level, you could even end up in jail. And so there was a Volkswagen engineer who got prison time for his role in the diesel cheating case. 00:07:58.000 |
So if you remember, this is where Volkswagen was cheating on emissions test and one of the kind of programmers that was a part of that. 00:08:06.000 |
And that person was just following orders from what their boss told them to do. But that is not not a good excuse for for doing something that's unethical and so something to be aware of. 00:08:20.000 |
So ethics is the discipline dealing with what's good and bad. It's a set of moral principles. It's not a set of answers, but it's kind of learning what sort of what sort of questions to ask and even how to weigh these decisions. 00:08:35.000 |
And I'll say some more about kind of ethical foundations and different ethical philosophies later later on in this lesson. 00:08:43.000 |
But first, I'm going to kind of start with some some use cases. Ethics is not the same as religion, laws, social norms or feelings, although it does have overlap with all these things. 00:08:54.000 |
It's not a fixed set of rules. It's well-founded standards of right and wrong. 00:09:00.000 |
And this is something where clearly not everybody agrees on the ethical action in every case. 00:09:06.000 |
But that doesn't mean that kind of anything goes or that all actions are considered equally ethical. 00:09:12.000 |
There are many things that are widely agreed upon and there are kind of a philosophical philosophical underpinnings for kind of making these decisions. 00:09:22.000 |
And ethics is also the ongoing study and development of our ethical standards. It's a kind of never ending process of learning to kind of practice our ethical wisdom. 00:09:33.000 |
I'm going to refer several times to so here I'm referring to a few articles from the Markula Center for Tech Ethics at Santa Clara University. 00:09:42.000 |
In particular, the work of Shannon Valor, Brian Green and Irina Reiku is fantastic and they have a lot of resources, some of which I'll circle back to later later in this talk. 00:09:52.000 |
I spent years of my life studying ethics. It was my major at university and so much time on the question of what is ethics. 00:10:01.000 |
I think my takeaway from that is studying the philosophy ethics was not particularly helpful in learning about ethics. 00:10:08.000 |
Yes, and I will try to keep this kind of very, very applied and very practical. 00:10:14.000 |
Also very kind of tech industry specific of what what do you need in terms of applied ethics. 00:10:19.000 |
Markula said it's great. They somehow they take stuff that I thought was super dry and turn it into useful checklists and things. 00:10:33.000 |
So Casey Fiesler is a professor at University of Colorado that I really admire and she created a crowdsource spreadsheet of tech ethics syllabi. 00:10:41.000 |
This was maybe two years ago and got over 200 syllabi entered into this this crowdsource spreadsheet. 00:10:48.000 |
And then she did a meta analysis on them of kind of looking at all sorts of aspects of the syllabi and what's being taught and how it's being taught and published a paper on it. 00:11:02.000 |
And a few interesting things about it is it raises there are a lot of ongoing discussions and lack of agreement on how to how to best teach tech ethics. 00:11:12.000 |
Should it be a standalone course versus worked into every course in the curriculum? 00:11:17.000 |
Who should teach it? A computer scientist, a philosopher or a sociologist? 00:11:23.000 |
And she analyzed for the syllabi what was the course home and the instructor home. 00:11:28.000 |
And you can see that the instructors came from a range of courses, including a range of disciplines. 00:11:34.000 |
Computer science, information science, philosophy, science and tech studies, engineering, law, math, business. 00:11:41.000 |
What topics to cover? A huge range of topics that can be covered, including a law and policy, privacy and surveillance, inequality, justice and human rights, 00:11:52.000 |
environmental impact, AI and robots, professional ethics, work in labor, cybersecurity. 00:11:58.000 |
The list goes on and on. And so this is clearly more than can be covered in any even a full semester length course and certainly not in kind of a single single lecture. 00:12:11.000 |
What learning outcomes? This is an area where there's a little bit more agreement. 00:12:15.000 |
We're kind of the number one skill that courses were trying to teach was critique followed by spotting issues, making arguments. 00:12:22.000 |
And so a lot of this is just even learning to spot what the issues are and how to critically evaluate kind of a piece of technology or design proposal to see what could go wrong and what the risks could be. 00:12:37.000 |
All right. So we're going to go through kind of a few different core topics. 00:12:42.000 |
And as I suggested, this is just going to be a kind of extreme subset of what could be covered. 00:12:48.000 |
We've tried to pick things that we think are very important and high impact. So one is recourse and accountability. 00:12:56.000 |
So I already shared this example earlier of the system that is determining poor people's health care benefits, having a bug. 00:13:05.000 |
And something that was kind of terrible about this is nobody took responsibility even once the bug was found. 00:13:12.000 |
So the creator of the algorithm was interviewed and asked, they asked him, you know, should people be able to get an explanation for why their benefits have been cut? 00:13:22.000 |
And he gave this very callous answer of, you know, yeah, they probably should, but I should probably dust under my bed, you know, like who's going to do that, which is very callous. 00:13:32.000 |
And then he ended up blaming the policymakers for how they had rolled out the algorithm. 00:13:38.000 |
The policymakers, you know, could blame the software engineers that implemented it. 00:13:43.000 |
And so there was a lot of passing the buck here. Dana Boyd has said that, you know, it's always been a challenge for bureaucracy to assign responsibility or bureaucracy is used to evade responsibility. 00:13:57.000 |
And today's algorithmic systems are often extending bureaucracy. 00:14:02.000 |
A couple of questions and comments about cultural context. 00:14:08.000 |
Many notes that there didn't seem to be any mention of cultural contexts for ethics as part of those syllabi. 00:14:15.000 |
And somebody else was asking, how do you deal? You know, is this culturally dependent and how do you deal with that? 00:14:22.000 |
It is culturally dependent. I will mention this briefly later on. 00:14:26.000 |
So I'm going to share three different ethical philosophies that are kind of from the West. And we'll talk just briefly of one slide on, for instance, right now, there are a number of indigenous data sovereignty movements. 00:14:38.000 |
And I know the Maori data sovereignty movement has been particularly active, but different, yeah, different cultures do have different views on ethics. 00:14:46.000 |
And I think that the cultural context is incredibly important. 00:14:50.000 |
And we will not get into it tonight, but there's also kind of a growing field of algorithmic colonialism and kind of studying what are some of the issues when you have technologies built in one particular country and culture being implemented, you know, 00:15:07.000 |
halfway across the world in very different cultural context, often with little to no input from people, people living in that culture. 00:15:18.000 |
Although I do want to say that there are things that are widely, although not universally agreed on. 00:15:25.000 |
And so, for instance, the Universal Declaration on Human Rights, despite the name, it is not universally accepted. 00:15:31.000 |
But many, many different countries have accepted that as a human rights framework and as those being fundamental rights. 00:15:37.000 |
And so there are kind of principles that are often held cross-culturally, although, yeah, it's rare for something probably to be truly, truly universal. 00:15:50.000 |
So returning to this topic of kind of accountability and recourse, something to keep in mind is the data contains errors. 00:15:58.000 |
So there was a database used in California that's tracking supposedly gang members. 00:16:07.000 |
And an auditor found that there were 42 babies under the age of one who had been entered into this database. 00:16:15.000 |
And something concerning about the database is that it's basically never updated. 00:16:19.000 |
I mean, people are added, but they're not removed. 00:16:21.000 |
And so once you're in there, you're in there. And 28 of those babies were marked as having admitted to being gang members. 00:16:28.000 |
And so keep in mind that this is just a really obvious example of the error. 00:16:33.000 |
But how many other kind of totally wrong entries are there? 00:16:38.000 |
Another example of data containing errors involves the three credit bureaus in the United States. 00:16:47.000 |
The FTC's large-scale study of credit reports found that 26 percent had at least one mistake in their files and 5 percent had errors that could be devastating. 00:16:56.000 |
And this is the headline of an article that was written by a public radio reporter who went to get an apartment. 00:17:04.000 |
And the landlord called him back afterwards and said, you know, your background check showed up that you had firearms convictions. 00:17:12.000 |
And this person did not have any firearms convictions. And it's something where in most cases, the landlord would probably not even tell tell you and let you know that's why you weren't getting the apartment. 00:17:21.000 |
And so this guy looked into it. I should note that this guy was white, which I'm sure helped him in getting the benefit of the doubt and found this error. 00:17:31.000 |
And he made dozens of calls and could not get it fixed until he told them that he was a reporter and that he was going to be writing about it, which is something that most of us would not be able to do. 00:17:41.000 |
But it was even once he had pinpointed the air and he had to talk to the county clerk in the place he used to live, it was still a very difficult process to get it updated. 00:17:54.000 |
And this can have a huge, huge impact on people's lives. 00:18:00.000 |
There's also the issue of when technology is used in ways that the creators may not have intended. 00:18:06.000 |
So, for instance, with facial recognition, it is pretty much entirely being developed for adults. Yet NYPD is putting the photos of children as young as age 11 into into databases. 00:18:19.000 |
And we know the error rates are higher. This is not how it was developed. 00:18:24.000 |
So this is this is a serious, serious concern. And there are a number of kind of misuses. 00:18:30.000 |
The Georgetown Center for Privacy and Technology, which is fantastic, you should definitely be following them, did a report garbage in garbage out looking at how police were using facial recognition and practice. 00:18:42.000 |
And they found some really concerning examples. For instance, in one case NYPD had a photo of a suspect and they wasn't returning any matches. 00:18:55.000 |
And they said, well, this person kind of looks like Woody Harrelson. So then they googled the actor Woody Harrelson and put his face into the facial recognition and use that to generate leads. 00:19:06.000 |
And this is clearly not the correct use at all, but it's it's a way that it's being it's being used. 00:19:12.000 |
And so there's kind of total lack of accountability here. 00:19:17.000 |
And then another kind of study of cases in all 50 states of police officers kind of abusing confidential databases to look up ex-romantic partners or to look up activists. 00:19:30.000 |
And so, you know, here, this is not necessarily an error in the data, although that can be present as well, but kind of keeping in mind how it can be misused by the users. 00:19:43.000 |
The next topic is feedback loops and metrics. And so I talked a bit about feedback loops in the beginning as kind of one of one of the three key use cases. 00:19:53.000 |
And so this is a topic I wrote a blog post about this fall. The problem with metrics is a big problem for AI. 00:19:59.000 |
And then together with David Yominsky, who's director of the Data Institute, expanded this into a paper, reliance on metrics is a fundamental challenge for AI. 00:20:08.000 |
And this was accepted to the ethics and data science conference. 00:20:13.000 |
But overemphasizing metrics can lead to a number of problems, including manipulation, gaming, myopic focus on short term goals, because it's easier to track short term quantities, unexpected negative consequences. 00:20:29.000 |
And much of AI and machine learning centers on optimizing a metric. 00:20:34.000 |
This is kind of both the strength of machine learning as it's gotten really, really good at optimizing metrics. 00:20:39.000 |
But I think this is also kind of inherently a weakness or a limitation. 00:20:45.000 |
I'm going to give a few examples, and this can happen even not just in machine learning kind of but in analog examples as well. 00:20:55.000 |
So this is from a study of when English is England's public health system implemented a lot more targets around numbers in the early 2000s. 00:21:07.000 |
And the study was called what's what's measured is what matters. 00:21:10.000 |
And so they found so one of the targets was around reducing our wait times, which seems like a good goal. 00:21:16.000 |
However, this led to canceling scheduled operations to draft extra staff into the ER. 00:21:23.000 |
So if they felt like there are too many people in the ER, they would just start canceling operations so they could get more doctors requiring patients to wait in queues of ambulances because time waiting in an ambulance didn't count towards your ER wait time. 00:21:37.000 |
Turning stretchers into beds by putting them in hallways. 00:21:41.000 |
And there were also big discrepancies in the numbers reported by hospitals versus by patients. 00:21:45.000 |
And so if you ask the hospital on average, how long are people waiting? 00:21:49.000 |
You get a very different answer than when you were asking the patients, how long did you have to wait? 00:21:58.000 |
Another another example is essay grading software. 00:22:04.000 |
And so this essay grading software, I believe, is being used in 22 states now in the United States. 00:22:11.000 |
Yes, 20 states and it tends to focus on metrics like sentence length, vocabulary, spelling, subject, verb agreement, because these are the things that we know how to measure and how to measure with a computer. 00:22:22.000 |
But it can't evaluate things like creativity or novelty. 00:22:28.000 |
However, gibberish essays with lots of sophisticated words score well and there are even examples of people creating computer programs to generate these kind of gibberish sophisticated essays and then they're graded by this other computer program and highly rated. 00:22:46.000 |
There's also bias in this essays by African American students received lower grades from the computer than from expert human graders. 00:22:55.000 |
And essays by students from mainland China received higher scores from the computer than from expert human graders and the authors of the study thought that this result suggests they may be using chunks of pre memorized text that score well. 00:23:14.000 |
And this is these are just kind of two examples. I have a bunch more in the blog post and even more in the paper of ways that metrics can invite manipulation and gaming whenever they're they're given a lot of emphasis. 00:23:26.000 |
And this is a good heart's laws, kind of a law that a lot of people talk about it as this idea that the more you rely on a metric, the kind of the less reliable it becomes. 00:23:40.000 |
So returning to this example of feedback loops and recommendation systems, Guillaume Chaslot is a former Google slash YouTube engineer. 00:23:50.000 |
YouTube is owned by Google and he wrote a really great post and he's done a ton to raise awareness about this issue and founded the nonprofit algo transparency, which kind of externally tries to monitor YouTube's recommendations. 00:24:04.000 |
He's partnered with the Guardian and the Wall Street Journal to do investigations. 00:24:09.000 |
But he wrote a post around how kind of in the in the earlier days, the recommendation system was designed to maximize watch time. 00:24:17.000 |
And so and this is this is something else that's often going on with metrics is that any metric is just a proxy for what you truly care about. 00:24:26.000 |
And so here, you know, the team at Google was saying, well, you know, if you're watching more YouTube, it signals to test that they're happier. 00:24:35.000 |
However, this also ends up incentivizing content that tells you the rest of the media is lying because kind of believing that everybody else is lying will encourage you to spend more time on a particular platform. 00:24:48.000 |
So Guillaume wrote a great post about this kind of mechanism that's at play. 00:24:53.000 |
And, you know, this is not just YouTube. This is any recommendation system could I think be susceptible to this. 00:25:00.000 |
And there has been a lot of talk about kind of issues with many recommendation systems across platforms. 00:25:08.000 |
But it is it is something to be mindful of and something that the kind of creators of this did not anticipate. 00:25:16.000 |
Then last year, Guillaume kind of gathered this data on. 00:25:22.000 |
So here the X axis is the number of channels, number of YouTube channels recommending a video and the Y axis is the log of the views. 00:25:31.000 |
And we see this extreme outlier, which was Russia's today take Russia today's take on the Mueller report. 00:25:37.000 |
And this is something that Guillaume observed and then was picked up by the Washington Post. 00:25:43.000 |
But this this strongly suggests that Russia today has perhaps gained the recommendation algorithm, which is which is not surprising. 00:25:51.000 |
And it's something that I think many content creators are conscious of and trying to experiment and see what what gets more heavily recommended and thus more views. 00:26:02.000 |
So it's also important to note that our online environments are designed to be addictive. 00:26:06.000 |
And so when kind of what we click on is often used as a proxy of of what we enjoy or what we like, that's not necessarily, though, for of our kind of like our best selves or our higher selves. 00:26:18.000 |
It's you know, it's what we're clicking on in this kind of highly addictive environment that's often appealing to some of our kind of lower instincts. 00:26:29.000 |
St. up to Fecci uses the analogy of a cafeteria that's kind of shoving salty, sugary, fatty foods in our faces and then learning that, hey, people really like salty, sugary, fatty foods, which I think most of us do in a kind of very primal way. 00:26:44.000 |
But we often, you know, kind of our higher self is like, oh, I don't want to be eating junk food all the time. 00:26:49.000 |
And online, we often kind of don't have great mechanisms to say, you know, like, oh, I really want to read like more long form articles that took months to research and are going to take a long time to digest while we may want to do that. 00:27:05.000 |
Our online environments are not not always conducive to it. 00:27:09.000 |
Yes. So if I make a comment about the false sense of security argument, which is very relevant. 00:27:16.000 |
Masks and things. Did you have anything to say about this false sense of security argument? 00:27:31.000 |
There's a common feedback at the moment that people shouldn't wear masks because they make a little sense of security that kind of makes sense to you from an ethical. 00:27:46.000 |
No, that's I don't think that's a good argument at all. 00:27:51.000 |
In general, there's so many other people, including Jeremy, have pointed this out. 00:27:56.000 |
There's so many actions we take to make our lives safer, whether that's wearing seat belts or wearing helmets when biking, practicing safe sex, like all sorts of things where we really want to maximize our safety. 00:28:09.000 |
And so I think in Zeynep Tefecti had a great thread on this today of it's not that there can never be any sort of impact in which people have a false sense of security, but it is something that you would really want to be gathering data on and build a strong case around 00:28:25.000 |
to just assume it's going to happen and that in most cases people can think of, even if that is a small second order effect, the effect of doing something that increases safety tends to have a much larger impact on actually increasing safety. 00:28:50.000 |
Yeah, as I mentioned before, a lot of our incentives are focused on short term metrics. Long term things are much harder to measure and often involve kind of complex relationships. 00:29:02.000 |
And then the fundamental business model of most of the tech companies is around manipulating people's behavior and monopolizing their time. And these things I don't think in advertising is inherently bad, but they, I think it can be negative when taken to an extreme. 00:29:19.000 |
There's a great essay by James Grimmelman, "The Platform is the Message," and he points out these platforms are structurally at war with themselves. 00:29:30.000 |
The same characteristics that make outrageous and offensive content unacceptable are what make it go viral in the first place. And so there's this kind of real tension here in which often things, yeah, that kind of can make content really offensive or unacceptable to us are also 00:29:48.000 |
what are kind of fueling their popularity and being promoted in many cases. And this is an interesting essay because he does this like really in depth dive on the Tide Pod Challenge, which was this meme around eating Tide Pods, which are poisonous, do not eat them. 00:30:07.000 |
And he really analyzes it though. It's a great look at meme culture, which is very common and how kind of argues there's probably no example of someone talking about the Tide Pod Challenge that isn't partially ironic, which is common in memes that even kind of whatever you're 00:30:24.000 |
saying, they're kind of layers of irony and different groups are interpreting them differently. And that even when you try to counteract them, you're still promoting them. So with the Tide Pod Challenge, a lot of like celebrities were telling people don't eat Tide Pods. 00:30:38.000 |
But that was also then kind of perpetuating the popularity of this meme. So this is an essay I would recommend, I think it's pretty insightful. 00:30:49.000 |
And so this is, we'll get to disinformation shortly, but the major tech platforms often incentivize and promote disinformation and this is unintentional, but it is somewhat built into their design and architecture, their recommendation systems and ultimately their business models. 00:31:09.000 |
And then on the topic of metrics, I just want to bring up, so there's this idea of blitzscaling and the premise is that if a company grows big enough and fast enough, profits will eventually follow. 00:31:23.000 |
It prioritizes speed over efficiency and risks potentially disastrous defeat. And Tim O'Reilly wrote a really great article last year talking about many of the problems with this approach, which I would say is incredibly widespread and is, I would say, the kind of 00:31:38.000 |
fundamental model underlying a lot of venture capital. And in it though, investors kind of end up anointing winners as opposed to market forces. It tends to lend itself towards creating monopolies and duopolies. 00:31:52.000 |
It's bad for founders and people end up kind of spreading themselves too thin. So there are a number of significant downsides to this. Why am I bringing this up in an ethics lesson when we were talking about metrics? 00:32:07.000 |
But hockey stick growth requires automation and a reliance on metrics. Also prioritizing speed above all else doesn't leave time to reflect on ethics. And that is something that's hard that I think you do often have to kind of pause to think about ethics. 00:32:22.000 |
And that following this model, when you do have a problem, it's often going to show up on a huge scale if you've scaled very quickly. So I think this is something to at least beware of. 00:32:36.000 |
So one person asks about is there a dichotomy between AI ethics, which seems like a very first world problem, and wars, poverty, environmental exploitation has been a level of problem, I guess. 00:32:57.000 |
And there's an answer here, which is something else maybe you can comment on whether you agree or have anything to add, which is that AI ethics, they're saying, is very important also for other parts of the world, particularly in areas with high cell phone usage. 00:33:12.000 |
For example, many countries in Africa have high cell penetration. People get their news from Facebook and WhatsApp and YouTube, and though it's useful, it's been the source of many problems. 00:33:21.000 |
Do you have any comments on that kind of? Yeah, so I think the first question, so AI ethics, as I noted earlier, and I'm using the phrase data ethics here, but it's this very broad and it refers to a lot of things. 00:33:34.000 |
I think if people are talking about the, you know, in the future, can computers achieve sentience and what are the ethics around that? And that is not my focus at all. 00:33:46.000 |
I am very much focused on, and this is our mission with the Center for Applied Data Ethics at the University of San Francisco, is kind of how are people being harmed now? What are the most immediate harms? 00:33:56.000 |
And so in that sense, I don't think that data ethics has to be a first world or kind of futuristic issue. It's what's happening now. 00:34:06.000 |
And yeah, and as the person said, and a few examples, well, one example I'll get to later is definitely the genocide in Myanmar in which the Muslim minority, the Rohingya, are experiencing genocide. 00:34:21.000 |
The UN has ruled that Facebook played a determining role in that, which is really intense and terrible. And so I think that's an example of technology leading to very real harm now. 00:34:36.000 |
They're also, yeah, WhatsApp, which is owned by Facebook. There have been issues with people spreading disinformation and rumors and it's led to several lynching, dozens of lynchings in India of people kind of spreading these false rumors of, oh, there's a kidnapper coming around and in these kind of small remote villages and then a visitor or stranger shows up and gets killed. 00:35:00.000 |
And WhatsApp also played a very important role or bad role in the election of Bolsonaro in Brazil, election of Duerte in the Philippines. 00:35:10.000 |
So I think technology is having a kind of very immediate impact on people and that those are the types of ethical questions I'm really interested in and that I hope you are interested in as well. 00:35:29.000 |
Well, I will talk about disinformation. I realize those were kind of some disinformation focus and I'm going to talk about bias first. I think it's bias and disinformation. Yes. 00:35:38.000 |
Question. When we talk about ethics, how much of this is intentional unethical behavior? I see a lot of the examples as more of competent behavior or bad modeling, whether product or models are rushed without sufficient testing, thought around bias, so forth, but not necessarily malignant. 00:35:56.000 |
Yeah, no, I agree with that. I think that most of this is unintentional. I do think there's a often though, well, we'll get into some cases. I think that I think in many cases, the profit incentives are misaligned. 00:36:10.000 |
And I do think that when people are earning a lot of money, it is very hard to consider actions that would reduce their profits, even if they would prevent harm and increase kind of ethics. 00:36:22.000 |
And so I think that there's at some point where valuing profit over how people are being harmed is when does that become intentional is a question to debate. 00:36:36.000 |
But I don't think people are setting out to say like I want to cause a genocide or I want to help an authoritarian leader get elected. 00:36:45.000 |
Most people are not starting with that, but I think sometimes it's a carelessness and a thoughtlessness, but that I do think we are responsible for that and we're responsible to kind of be more careful and more thoughtful in how we approach things. 00:37:01.000 |
All right, so bias. So bias, I think, is an issue that's probably gotten a lot of attention, which is great. And I want to get a little bit more in depth because sometimes discussions on bias stay a bit superficial. 00:37:13.000 |
There was a great paper by Harini Suresh and John Gutag last year that looked at kind of came up with this taxonomy of different types of bias and how they had kind of different sources in the machine learning kind of pipeline. 00:37:29.000 |
And it was really helpful because, you know, different sources have different causes and they also require different different approaches for addressing that. 00:37:38.000 |
Harini wrote a blog post version of the paper as well, which I love when researchers do that. I hope more of you, if you're writing an academic paper, also write the blog post version. 00:37:48.000 |
And I'm just going to go through a few of these types. 00:37:52.000 |
So one is representation bias. And so I would imagine many of you have heard of Joy Balamwini's work, which has rightly received a lot of publicity in gender shades. 00:38:04.000 |
She and Timnit Gebru investigated commercial computer vision products from Microsoft, IBM, and Face++. 00:38:10.000 |
And then Joy Balamwini and Debraji did a follow-up study that looked at Amazon and Keros and several other companies. 00:38:18.000 |
And the typical results they kind of found basically everywhere was that these products performed significantly worse on dark-skinned women. 00:38:25.000 |
So they were kind of doing worse on people with darker skin compared to lighter skin, worse on women than on men. 00:38:32.000 |
And then the kind of the intersection of that dark-skinned women had these very high air rates. 00:38:36.000 |
And so one example is IBM. Their product was 99.7 percent accurate on light-skinned men and only 65 percent accurate on dark-skinned women. 00:38:47.000 |
And again, this is a commercial computer vision product that was released. Question? 00:38:54.000 |
There's a question from the Trimmel study group about the Volkswagen example. 00:39:00.000 |
In many cases, it's management that drives and rewards unethical behavior. 00:39:05.000 |
What can an individual engineer do in a case like this, especially in a place like Silicon Valley where people move companies so often? 00:39:13.000 |
Yeah, so I think I think that's a great point. And that is an example where I would have I would have much rather seen people that were higher ranking during jail time about this because I think that they were they were driving that. 00:39:26.000 |
I think that it's great to remember that I know many people in the world don't have this option. 00:39:36.000 |
But I think for many of us working in tech, particularly in Silicon Valley, we tend to have a lot of options and often more options than we realize. 00:39:43.000 |
Like I talk to people frequently that feel trapped in their jobs, even though they're a software engineer in Silicon Valley and so many companies are hiring. 00:39:53.000 |
And so I think it is important to use that leverage. 00:39:56.000 |
I think a lot of the kind of employee organizing movements are very promising and that can be useful, but really trying to kind of vet the ethics of the company you're joining and also being willing to walk away if you if if you're able to do so. 00:40:19.000 |
So this is this example of representation bias here. The kind of way to address this is to build a more representative data set. 00:40:26.000 |
It's very important to keep consent in mind of the people if you're using pictures of people. 00:40:32.000 |
But Joy Balamini and Tim, Nick, did this as part of as part of gender shades. 00:40:38.000 |
However, this is the fact that this was a problem not just for one company, but basically kind of every company they looked at was due to this underlying problem, which is that in machine learning benchmark data sets for on a lot of research. 00:40:55.000 |
However, kind of several years ago, all the kind of popular facial data sets were primarily of light skinned men, for instance, IGB a kind of popular face data set several years ago, only 4% of the images were of dark skinned women. 00:41:16.000 |
Question. I've been worried about COVID-19 contact tracing and the erosion of privacy, location tracking, private surveillance companies, etc. 00:41:25.000 |
What can we do to protect our digital rights post-COVID? Can we look to any examples in history of what to expect? 00:41:32.000 |
That is a huge question and something I have been thinking about as well. I'm going to put that off to later to talk about. And that is something where in the course I teach, I have an entire unit on privacy and surveillance, which I do not in tonight's lecture, but I can share some materials. 00:41:51.000 |
Although I am already really even just like rethinking how I'm going to teach privacy and surveillance in the age of COVID-19 compared to two months ago when I taught it the first time. 00:42:02.000 |
But that is something I think about a lot and I will talk about later if we have time or on the forums if we don't. That's a great question, a very important question. 00:42:15.000 |
On the topic and I will say and I have not had the time to look into them yet. I do know that there are groups that are working on what are kind of more privacy protecting approaches for tracking and they're also groups putting out like if we are going to use some sort of tracking, what are the safeguards that need to be in place to do it responsibly? 00:42:35.000 |
Yes, I've been looking at that too. It does seem like this is a solvable problem with technology. Not all of these problems are, but you can certainly store tracking history on somebody's cell phone. 00:42:49.000 |
And then you could have something where you say when you've been infected and at that point you could tell people that they've been infected by sharing the location in a privacy preserving way. 00:43:04.000 |
I think some people are trying to work on that. I'm not sure it's particularly technically a problem. So I think that sometimes there are ways to provide the minimum kind of level, you know, kind of application with keeping privacy. 00:43:21.000 |
Yeah, and then I think it is very important to also have things of, you know, clear like expiration date, like we, you know, like looking back at 9/11 in the United States that kind of ushered in all these laws that were now kind of stuck with that have really eroded privacy 00:43:36.000 |
of anything we do around COVID-19 being very clear we are just doing this for COVID-19 and then there's a time limit and expires and it's kind of for this clear purpose. 00:43:47.000 |
And there are also issues though of, you know, I mentioned earlier about data containing errors. I know this has already been an issue in some of other countries that we're doing kind of more surveillance focused approaches of, you know, what about like when it's wrong and people are getting kind of quarantined and they don't even know why and for no reason. 00:44:07.000 |
And so to be mindful of those. But yeah, we'll talk more about this kind of later on. 00:44:17.000 |
Yeah, we had kind of the benchmarks. So when the benchmark that's, you know, widely used has bias, then that is really kind of replicated at scale and we're seeing this with ImageNet as well, which is, you know, probably the most widely studied computer vision data set out there. 00:44:34.000 |
Two thirds of the ImageNet images are from the West. So this pie chart shows that the 45% of the images in ImageNet are from the United States, 7% from Great Britain, 6% from Italy, 3% from Canada, 3% from Australia. 00:44:51.000 |
You know, and we're covering a lot of this pie without having gotten to outside the West. And so then this has shown up in concrete ways of classifiers trained on ImageNet. So one of the categories is bridegroom, a man getting married. 00:45:08.000 |
There are a lot of, you know, cultural components to that. And so they have, you know, much higher error rates on bridegrooms from the Middle East or from the Global South. 00:45:20.000 |
And there are people now kind of working to diversify these data sets, but it is quite dangerous that they can really be kind of widely built on its scale or have been widely built on its scale before these biases were recognized. 00:45:37.000 |
Another case study is the Compass Residivism algorithm, which is used in determining who has to pay bail. So in the US, a very large number of people are in prison who have not even had a trial yet just because they're too poor to afford bail, as well as sentencing decisions and parole decisions. 00:45:56.000 |
And ProPublica did a famous investigation in 2016 that I imagine many of you have heard of in which they found that the false positive rate for black defendants was nearly twice as high as for white defendants. 00:46:10.000 |
So black defendants were lit. A study from Dartmouth found that it was the software is no more accurate than Amazon mechanical Turk workers, so random people on the internet. 00:46:21.000 |
It's also the software is, you know, this proprietary black box using over 130 inputs, and it's no more accurate than a linear classifier on three variables. 00:46:32.000 |
Yet it's still in use, and it's in use in many states. Wisconsin is one place where it was challenged, yet the Wisconsin Supreme Court upheld its use. 00:46:42.000 |
If you're interested in the kind of topic of how you define fairness, because there is a lot of intricacy here, and I mean, I don't know anybody working on this who thinks that what Compass is doing is right, but they're using this different definition of fairness. 00:46:58.000 |
Arvind Ranyan has a fantastic tutorial, 21 fairness definitions in their politics that I highly recommend. 00:47:09.000 |
And so going back to kind of this taxonomy of types of bias, this is an example of historical bias, and historical bias is a fundamental structural issue with the first step of the data generation process and it can exist even given perfect sampling and feature selection. 00:47:25.000 |
So kind of with the image classifier, that was something where we could, you know, go gather a more representative set of images and that would help address it. That is not the case here. 00:47:34.000 |
So gathering kind of more data on the US criminal justice system, it's all going to be biased because that's really kind of baked into baked into our history and our current state. 00:47:46.000 |
And so this is, I think, good, good to recognize. 00:47:51.000 |
One thing that can be done to try to at least mitigate this is to really talk to domain experts and by the people impacted. And so a really positive example of this is a 00:48:05.000 |
tutorial from the Fairness Accountability and Transparency Conference that Christian Lum, who's the lead statistician for the Human Rights Data Analysis Group and now professor at UPenn, organized together with a former public defender, Elizabeth Bender, 00:48:21.000 |
who's the staff attorney for New York's Legal Aid Society, and Terrence Wilkerson, an innocent man who was arrested and could not afford bail. 00:48:29.000 |
And Elizabeth and Terrence were able to provide a lot of insight to how the criminal justice system works in practice, which is often kind of very different from the more kind of clean, logical abstractions that computer scientists deal with. 00:48:44.000 |
But it's really important to understand those kind of intricacies of how this is going to be implemented and used in these messy, complicated, real-world systems. 00:48:57.000 |
Aren't the AI biases transferred from real-life biases? For instance, how are people being treated differently? Isn't everyday phenomenon women too? 00:49:08.000 |
That's correct, yes. So this is often coming from real-world biases. And I'll come to this in a moment, but algorithmic systems can amplify those biases, so they can make them even worse. But they are often being learned from existing data. 00:49:26.000 |
I asked it because I guess I often see this being raised as if it's kind of a reason not to worry about AI, so I thought it's not. 00:49:35.000 |
Well, I'm going to get to that in a moment. I'm actually thinking two slides, so hold on to that question. 00:49:44.000 |
I just want to talk about one other type of bias first, measurement bias. 00:49:48.000 |
So this was an interesting paper by Sendiel Melanathan and Zayed Obermeyer, where they looked at historic electronic health record data to try to determine what factors are most predictive of stroke. 00:50:00.000 |
And they said, you know, this could be useful, like prioritizing patients at the ER. 00:50:04.000 |
And so they found that the number one most predictive factor was prior stroke, which that makes sense. 00:50:10.000 |
Second was cardiovascular disease. That's also that seems reasonable. And then third most kind of still very predictive factor was accidental injury, followed by having a benign breast lump, a colonoscopy or sinusitis. 00:50:26.000 |
And so I'm not a medical doctor, but I can tell something weird is going on with factors three through six here. 00:50:32.000 |
Like, why would these things be predictive of stroke? Does anyone want to think about about why this might be? 00:50:46.000 |
Any guesses you want to read? Oh, someone's. Yeah. 00:50:55.000 |
OK, the first answer was they test for it anytime someone has stroke confirmation bias overfitting is because they happen to be in hospital already biased data. 00:51:09.000 |
EHR records these events because the data was taken before certain advances in medical science. 00:51:17.000 |
These are these are all good guesses, not not quite what I was looking for, but good, good thinking. That's such a nice way of saying. 00:51:26.000 |
So what that what the researchers say here is that this was about their patients, their people that utilize health care a lot and people that don't. 00:51:35.000 |
And they call it kind of high utility versus low utility of health care. And there are a lot of factors that go into this. 00:51:40.000 |
I'm sure just who has health insurance and who can afford their copays. There may be cultural factors, maybe racial and gender bias. 00:51:46.000 |
There is racial and gender bias on how people are treated. So a lot of factors and basically people that utilize health care a lot, 00:51:55.000 |
they will go to a doctor when they have sinusitis and they will also go in when they're having a stroke. 00:52:00.000 |
And people that do not utilize health care much are probably not going to go in possibly for either. 00:52:05.000 |
And so what the authors write is that we haven't measured stroke, which is a region of the brain being denied kind of new blood and new oxygen. 00:52:14.000 |
What we've measured is who had symptoms, who went to the doctor, received tests and then got this diagnosis of stroke. 00:52:20.000 |
And that seems like it might be a reasonable proxy for who had a stroke, but a proxy is never exactly what you wanted. 00:52:28.000 |
And in many cases that that gap ends up being significant. And so this is just one form that measurement bias can take. 00:52:35.000 |
But I think it's something to really kind of be on the lookout for because it can be quite subtle. 00:52:39.000 |
And so now starting to return to a point that was brought up earlier, aren't aren't people biased? Yes, yes, we are. 00:52:47.000 |
And so there have been dozens and dozens, if not hundreds of studies on this. 00:52:54.000 |
But I'm just going to quote a few, all of which are linked to in this Cyndale Melanathan New York Times article, if you want to find find the studies. 00:53:01.000 |
So this all comes from, you know, peer reviewed research. But when doctors were shown identical files, they were much less likely to recommend a helpful cardiac procedure to black patients compared to white patients. 00:53:12.000 |
And so that was, you know, same file, but just changing the race of the patient. 00:53:17.000 |
When bargaining for a used car, black people were offered initial prices, seven hundred dollars higher and received fewer concessions. 00:53:24.000 |
Responding to apartment rental ads on Craigslist with a black name elicited fewer responses than with a white name. 00:53:30.000 |
An all white jury was 16 points more likely to convict a black defendant than a white one. 00:53:35.000 |
But when a jury had just one black member, it convicted both at the same rate. 00:53:40.000 |
And so I share these to show that kind of no matter what type of data you're looking working on, whether that is medical data or sales data or housing data or criminal justice data, that it's very likely that there's there's bias in it. 00:53:53.000 |
There's a question. I was going to say, I find that last one really interesting, like this kind of idea that a single black member of a jury, I guess it has some kind of like anchoring impact. 00:54:03.000 |
It kind of suggests that, you know, I'm sure you're going to talk about diversity later, but I just want to keep this in mind that maybe like even a tiny bit of diversity here just reminds people that there's a, you know, a range of different types of people and perspectives. 00:54:23.000 |
And so the question that was kind of asked earlier is, so why does algorithmic bias matter? 00:54:28.000 |
Like, I have just shown you that humans are really biased too. So why are we talking about algorithmic bias? 00:54:35.000 |
And people have brought this up kind of like, what's the fuss about it? 00:54:39.000 |
And there, I think algorithmic bias is very significant and worth talking about. 00:54:45.000 |
And I'm going to share four reasons for that. One is that machine learning can amplify bias. 00:54:50.000 |
So it's not just encoding existing biases, but in some cases, it's making them worse. 00:54:54.000 |
And there have been a few studies on this. One I like is from Maria de Artega of CMU. 00:55:01.000 |
And here they were, they took people's, I think, job descriptions from LinkedIn. 00:55:07.000 |
And what they found is that imbalances ended up being compounded. 00:55:11.000 |
And so in the group of surgeons, only 14 percent were women. However, in the true positives, so they were trying to predict the job title from the summary. 00:55:23.000 |
Women were only 11 percent in the true positives. So this kind of imbalance has gotten worse. 00:55:29.000 |
And basically, there's kind of this asymmetry where the, you know, the algorithm has learned it's safer for women to kind of not guess surgeon. 00:55:42.000 |
Another, so this is one reason, another reason that algorithmic bias is a concern is that algorithms are used very differently than human decision makers in practice. 00:55:53.000 |
And so people sometimes talk about them as though they are plug and play interchangeable of, you know, with humans, this bias and the algorithm is, you know, this bias. 00:56:01.000 |
Why don't we just substitute it in? However, the whole system around it ends up kind of being different in practice. 00:56:08.000 |
One, one kind of aspect of this is people are more likely to assume algorithms are objective or error free, even if they're given the option of a human override. 00:56:18.000 |
And so if you give a person, you know, even if you just say, I'm just giving the judge this recommendation, they don't have to follow it. 00:56:24.000 |
If it's coming from a computer, many people are going to take that as objective. 00:56:28.000 |
In some cases also, there may be, you know, pressure from their boss to, you know, not disagree with the computer more times, you know, nobody's going to get fired by going with the computer recommendation. 00:56:40.000 |
Algorithms are more likely to be implemented with no appeals process in place. And so we saw that earlier when we were talking about recourse. 00:56:49.000 |
Algorithms are often used at scale. They can be replicating an identical bias at scale. 00:56:54.000 |
And algorithmic systems are cheap. And all of these, I think, are interconnected. 00:56:58.000 |
So in many cases, I think that algorithmic systems are being implemented not because they produce better outcomes for everyone, but because they're kind of a cheaper way to do things at scale. 00:57:09.000 |
You know, offering a recourse process is more expensive, being on the lookout for errors is more expensive. 00:57:15.000 |
So this is kind of cost cutting measures. And Cathy O'Neill talks about many of these themes in her book, Weapons of Math Destruction, kind of under the idea that the privileged are processed by people, the poor are processed by algorithms. 00:57:29.000 |
Two questions. This seems like an intensely deep topic, needing specialized expertise to avoid getting it wrong. If you were building an ML product, would you approach an academic institution for consultation on this? 00:57:42.000 |
Do you see a data product development triad becoming maybe a quartet involving an ethics or data privacy expert? 00:57:52.000 |
So I think interdisciplinary work is very important. I would definitely focus on trying to find kind of domain experts on whatever your particular domain is who understand the intricacies of that domain is important. 00:58:08.000 |
And I think with the academic it depends. You do want to make sure you get someone who's kind of applied enough to kind of understand how things are happening in industry. 00:58:19.000 |
But I think involving more people and people from more fields is a good approach on the whole. 00:58:26.000 |
Someone invents and publishes a better ML technique, like attention or transformers. And then next a graduate student demonstrates using it to improve facial recognition by 5%. 00:58:35.000 |
And then a small startup publishes an app that does better facial recognition. And then a government uses the app to study downtown walking patterns and endangered species and after these successes for court audit monitoring. 00:58:45.000 |
And then a repressive government then takes that method to identify ethnicities and then you get a genocide. 00:58:51.000 |
No one's made a huge ethical error at any incremental step, yet the result is horrific. 00:58:56.000 |
I have no doubt that Amazon will soon serve up a personally customized price for each item that maximizes their profits. 00:59:02.000 |
How can such ethical creep be addressed where the effect is remote from many small causes? 00:59:12.000 |
So yeah, so that that's a kind of a great summary of how these things can happen somewhat incrementally. I'll talk about some tools to implement kind of towards the end of this lesson that hopefully can help us. 00:59:25.000 |
So some of it is I think we do need to get better at kind of trying to think a few more steps ahead than we have been. 00:59:31.000 |
You know, in particular, we've seen examples of people, you know, there was the study of how to identify protesters in a crowd, even when they had scarves or sunglasses or hats on. 00:59:41.000 |
You know, and when the researchers on that were questioned, they were like, oh, it never even occurred to us that bad guys would use this. 00:59:47.000 |
You know, we just thought it would be for finding bad people. 00:59:51.000 |
And so I do think kind of everyone should be building their ability to think a few more steps ahead. 00:59:59.000 |
And part of this is like it's great to do this in teams, preferably in diverse teams can help with that process. 01:00:06.000 |
Even on this question of computer vision, there has been, you know, just in the last few months, is it Joe Redmond, creator of YOLO, who has said that he's no longer working on computer vision just because he thinks the misuses so far outweigh the positives? 01:00:24.000 |
And Timnit Gebru said she's considering that as well. 01:00:28.000 |
So I think there are times where you have to consider. 01:00:33.000 |
And then I think also really actively thinking about how to what safeguards do we need to put in place to kind of address the the misuses that are happening. 01:00:41.000 |
Yes. I just wanted to say somebody really liked the Kathy O'Neill quote, privileged, processed by people, the poor, processed by algorithms. 01:00:49.000 |
And they're looking forward to learning more, reading more from Kathy O'Neill. Is that a book that you recommend? 01:00:54.000 |
Yes. Yeah. And Kathy O'Neill also writes and Kathy O'Neill is a fellow, fellow math PhD, but she also has written a number of good articles. 01:01:06.000 |
And the book kind of goes through a number of case studies of how algorithms are being used in different places. 01:01:17.000 |
So kind of in summary of humans are biased. Why do why are we making a fuss about algorithmic bias? 01:01:25.000 |
So one, as we saw earlier, machine learning can create food back loops. 01:01:28.000 |
So it's you know, it's not just kind of observing what's happening in the world, but it's also determining outcomes and it's kind of determining what future data is. 01:01:36.000 |
Machine learning can amplify bias. Algorithms in humans are used very differently in practice. 01:01:42.000 |
And I will also say technology is power. And with that comes responsibility. And I think for all of us to have access to deep learning, 01:01:49.000 |
we are still in a kind of very fortunate and small percentage of the world that is able to use this technology right now. 01:01:56.000 |
And I hope I hope we will all use it responsibly and really take our power seriously. 01:02:03.000 |
And I just I just noticed the time and I think we're about to start next section on analyzing or kind of steps, steps we can take. 01:02:14.000 |
So this would be a good a good place to take a break. So let's meet back in seven minutes at 7 45. 01:02:24.000 |
All right, let's start back up. And actually, I was at a slightly different place than I thought. 01:02:31.000 |
But just a few questions that you can ask about projects you're working on, and I hope you will ask about projects you're working on. 01:02:40.000 |
The first is should we should we even be doing this and considering that maybe there's some work that we shouldn't do. 01:02:48.000 |
There's a paper when the implication is not to design technology. 01:02:53.000 |
As engineers, we often tend to respond to problems with, you know, what can I make or build to to address this? 01:02:59.000 |
But sometimes the answer is to not make or build anything. 01:03:04.000 |
One example of research that I think has a huge amount of downside and really no upside I see was kind of to identify the ethnicity, 01:03:14.000 |
particularly for people of ethnic minorities. And so there was work done identifying the Chinese Uyghurs, 01:03:20.000 |
which is the Muslim minority in Western China, which is since, you know, 01:03:24.000 |
over a million people have been placed in internment camps. 01:03:27.000 |
And so I think this is a very, very harmful, harmful line of research. 01:03:33.000 |
I think that the, you know, there have been at least two attempts of building, 01:03:39.000 |
building a classifier to try to identify someone's sexuality, which is it's probably just picking up on kind of stylistic differences. 01:03:49.000 |
But this is something that could also be quite, quite dangerous as in many countries. 01:03:57.000 |
So this is a question for me, which I don't know the answer to. 01:04:02.000 |
As that title says, a Stanford scientist says he built the gay dot using the lamest A.I. possible to prove a point. 01:04:09.000 |
And my understanding is that point was to say, you know, I guess it's something like, hey, you could use fast A.I. lesson one. 01:04:17.000 |
After an hour or two, you can build this thing. Anybody can do it. 01:04:21.000 |
You know, how do you feel about this idea that there's a role to demonstrate what's readily available with the technology we have? 01:04:33.000 |
So I appreciate that. And I'll talk about this a little bit later. Open A.I. with GPT to, I think, was trying to raise raise a debate around around dual use and what is responsible release of of dual use technology and what's a kind of responsible way to to raise 01:04:54.000 |
raise awareness of what is possible in the in the cases of researchers that have done this on the sexuality question. 01:05:02.000 |
To me, it hasn't seemed like they've put adequate thought into how they're conducting that and who they're collaborating with to ensure that it is something that is leading to kind of helping address the problem. 01:05:19.000 |
But I think you're right that I think there is probably some place for letting people know what is probably widely available now. 01:05:26.000 |
Yeah, it reminds me a bit of like pen testing and info set where it's kind of considered it. 01:05:33.000 |
Well, there's an ethical way that you can go about pointing out that it's trivially easy to break into some of the system. 01:05:39.000 |
Yes, yeah, I would I would agree with that that there there is an ethical way, but I think that's something that we as a community still have more work to do and even determining what that is. 01:05:51.000 |
Other questions to consider are what biases in the data and something I should highlight is people often ask me, you know, how can I do bias my data or ensure that it's bias free and that's not possible. 01:06:04.000 |
All data contains bias and the kind of most most important thing is just to understand kind of how your data set was created and what its limitations are so that you're not blindsided by that bias, but you're never going to fully remove it. 01:06:17.000 |
And some of the I think most promising approaches in this area are work like Timnit Gebru's data sheets for data sets, which is kind of going through and asking kind of a bunch of questions about how your data set was created and for what purposes and how it's being maintained. 01:06:34.000 |
And you know, what are the risk in that just to to really kind of be aware of of the context of your data. 01:06:42.000 |
Can the code and data be audited? I think particularly in the United States, we have a lot of issues with when private companies are creating software that's really impacting people through the criminal justice system or hiring. 01:06:56.000 |
And when these things are, you know, kind of their proprietary black boxes that are protected in court, this creates a lot of kind of issues of, you know, what are what are our rights around that. 01:07:07.000 |
Looking at error rates for different subgroups is really important, and that's what's kind of so powerful about Joy Balamwini's work. If she had just looked at light skin versus dark skin and men versus women, she wouldn't have identified just how poorly the algorithms were doing on dark-skinned women. 01:07:27.000 |
What is the accuracy of a simple rule-based alternative? And this is something I think Jeremy talked about last week, which is just kind of good, good machine learning practice to have a baseline. 01:07:37.000 |
But particularly in cases like the compass recidivism, where this 130 variable black box is not doing much better than a linear classifier on three variables, that raises kind of a question of why are we using this. 01:07:52.000 |
And then what processes are in place to handle appeals or mistakes, because there will be errors in the data, there may be bugs in the implementation, and we need to have a process for recourse. 01:08:05.000 |
Can you explain, this is for me now, sorry I'm asking my own questions, nobody voted them up at all. What's the thinking behind this idea that a simpler model, is this, you're kind of saying a simpler model of other things being the same, you should pick the simpler one. 01:08:21.000 |
Is that what this baseline's for? And if so, what's the kind of thinking behind that? 01:08:26.000 |
Well, I guess with the compass recidivism algorithm, some of this for me is linked to the proprietary black box nature and so you're right if maybe if we had a way to introspect and what were our rights around appealing something. 01:08:42.000 |
But I would say yeah, like why use the more complex thing if the simpler one works the same. 01:08:50.000 |
And then how diverse is the team that built it and I'll talk more about team diversity later in this lesson. 01:08:59.000 |
It says Jeremy at the start, but I'm not the teacher so it actually says Jeremy, do you think transfer learning makes this tougher, auditing the data that led to the initial model? I assume they mean Jeremy please ask Rachel. 01:09:21.000 |
Again, I think it's important. I would say I think it's important to have information probably on both data sets, what the initial data set used was and what the data set you use to fine tune it. 01:09:40.000 |
And then I'll say so while bias and fairness as well as accountability and transparency are important, they aren't everything. 01:09:48.000 |
And so there's this great paper, a mulching proposal by Oskis et al. And here they talk about a system for turning the elderly into high nutrient slurry. 01:10:00.000 |
So this is something that's clearly unethical, but they propose a way to do it that is fair and accountable and transparent and meets these qualifications. 01:10:09.000 |
And so that kind of shows some of the limitations of this framework as well as kind of being a good technique for kind of inspecting whatever framework you are using of trying to find something that's clearly unethical that could meet the standards you've put forth. 01:10:29.000 |
That technique, I really like it. It's my favorite technique from philosophy. It's this idea that you say, OK, given this premise, 01:10:40.000 |
here's what it implies. And then you try and find an implied result, which intuitively is clearly insane. 01:10:49.000 |
So really, it's the number one philosophical thinking tool I got out of university, and sometimes you can have a lot of fun with it like this time too. 01:11:03.000 |
All right. So the next kind of big case study or topic I want to discuss is disinformation. 01:11:10.000 |
So in 2016 in Houston, a group called Heart of Texas posted about a protest outside an Islamic center. 01:11:23.000 |
And they told people to come armed. Another Facebook group posted about a counterprotest to show up supporting freedom of religion and inclusivity. 01:11:35.000 |
And so there were kind of a lot of people present at this and more people on the side supporting freedom of religion. 01:11:44.000 |
And a reporter, though, for the Houston Chronicle noticed something odd, which he was not able to get in touch with the organizers for either side. 01:11:51.000 |
And it came out many months later that both sides had been organized by Russian trolls. 01:11:57.000 |
And so this is something where you had the people protesting were genuine Americans kind of protesting their beliefs, 01:12:06.000 |
but they were doing it in this way that had been kind of completely framed very disingenuously by Russian operatives. 01:12:18.000 |
And so when thinking about disinformation, it is not people often think about so-called fake news and inspecting like a single post is this is this true or false. 01:12:30.000 |
But really, disinformation is often about orchestrated campaigns of manipulation and that it involves can evolve seeds of truth. 01:12:41.000 |
Kind of the best propaganda always involves kernels of truth, at least. It also involves kind of misleading context and can involve very kind of sincere, sincere people that get swept up in it. 01:12:57.000 |
A report came out this fall, an investigation from Stanford's Internet Observatory, where Renee DiResta and Alex Stamos work of Russia's kind of most recent disinformation or most recently identified disinformation campaign. 01:13:14.000 |
And it was operating in six different countries in Africa. It often purported to be local news sources. 01:13:22.000 |
So it was a multi-platform. They were encouraging people to join their WhatsApp and Telegram groups and they were hiring local people as reporters. 01:13:30.000 |
And a lot of the content was not necessarily disinformation. It was stuff on culture and sports and local weather. 01:13:39.000 |
I mean, there was a lot of kind of very pro-Russia coverage, but that it covered a range of topics. 01:13:46.000 |
It's kind of a very sophisticated phase of disinformation. And in many cases, it was hiring, hiring locals kind of as reporters to work for these sites. 01:13:58.000 |
And I should say, well, I've just given two examples of Russia. Russia certainly does not have a monopoly on disinformation. 01:14:03.000 |
There are plenty of plenty of people involved in producing it kind of on a topical topical issue. 01:14:14.000 |
There's been a lot of disinformation around around coronavirus and Covid-19. 01:14:21.000 |
I in terms of kind of a personal level, if you're looking for advice on spotting disinformation or to share with loved ones about this, 01:14:30.000 |
Mike Caulfield is a great person to follow and he's even so he tweets at Holden and then he has started an infodemic blog specifically about the about Covid-19. 01:14:41.000 |
But he talks about his approach and how people have been trained in schools for 12 years. 01:14:47.000 |
Here's a text. Read it. Use your critical thinking skills to figure out what you think about it. 01:14:52.000 |
But professional fact checkers do the opposite. 01:14:54.000 |
They get to a page and they immediately get off of it and look for kind of higher, higher quality sources to see if they can find confirmation. 01:15:02.000 |
Caulfield also really promotes the idea of a lot of critical thinking techniques that have been taught take a long time. 01:15:10.000 |
And, you know, we're not going to spend 30 minutes evaluating each tweet that we see in our Twitter stream. 01:15:15.000 |
It's better to give people an approach that they can do in 30 seconds that, you know, it's not going to be fail proof if you're just doing something for 30 seconds. 01:15:22.000 |
But it's better to to check than to have something that takes 30 minutes that you're just not going to do at all. 01:15:28.000 |
So I wanted to kind of put this out there as a resource and he has a whole kind of set of lessons at lessons.checkplease.cc and he's he's a professor. 01:15:39.000 |
And I in the data ethics course I'm teaching right now, I made my first lesson, the first half of which is kind of specifically about coronavirus disinformation. 01:15:53.000 |
And so I'll add a link on the forums if you want if you want a lot more detail on on disinformation than just kind of this short bit here. 01:16:03.000 |
But so going back to kind of like what is disinformation, it's important to think of it as an ecosystem. 01:16:09.000 |
Again, it's not just a single poster, a single news story that has, you know, is misleading or has false elements in it, but it's this really this broader ecosystem. 01:16:20.000 |
Claire Wardle, First Draft News, who is a leading expert on this and does a lot around kind of training journalists and how journalists can report responsibly, talks about the trumpet of amplification. 01:16:31.000 |
And this is where rumors or memes or things can start on 4chan and 8chan and then move to closed messaging groups such as WhatsApp, Telegram, Facebook Messenger from there to community conspiracy communities on Reddit or YouTube, then to kind of more mainstream social media and then picked up by the professional media and politicians. 01:16:54.000 |
And so this can make it very hard to address that it is this kind of multi platform. In many cases, campaigns may be utilizing kind of the differing rules or loopholes between between the different platforms. 01:17:07.000 |
And I think we certainly are seeing more and more examples where it doesn't have to go through all these steps, but can can can jump jump forward. 01:17:16.000 |
And online discussion is very, very significant because people, it helps us form our opinions. And this is tough because I think most of us think of ourselves as pretty independent minded. 01:17:29.000 |
But discussion really does, you know, we evolved as kind of social beings and to be influenced by people in our in group and in opposition to people in our out group. 01:17:38.000 |
And so online discussion impacts us. People discuss all sorts of things online. Here's a Reddit discussion about whether the US should cut defense spending. 01:17:48.000 |
You have comments, you're wrong. The defense budget is a good example of how badly the US spends money on the military. 01:17:55.000 |
Someone else says, yeah, but that's already happening. There's a huge increase in the military budget. The Pentagon budget's already increasing. 01:18:02.000 |
I didn't mean to sound like stop paying for the military. I'm not saying that we cannot pay the bills, but I think it would make sense to cut defense spending. 01:18:12.000 |
Does anyone want to guess what subreddit this is from? 01:18:24.000 |
Unpopular opinion, news, change my view, net neutrality. 01:18:32.000 |
Those are good guesses, but they're wrong. I love the way you say no. 01:18:36.000 |
This is all from, well, it's from the sub simulator GPT-2. So these comments were all written by GPT-2. 01:18:45.000 |
And this is in good fun. It was clearly labeled on the subreddit that it's coming in. GPT-2 is a language model from open AI that was kind of in a trajectory of research that many, many groups were on. 01:19:03.000 |
And so it was released, I guess, about a year ago and. 01:19:10.000 |
Should I read the unicorn story, Jeremy? OK, so many of you have probably have probably seen this. So here and this this was cherry picked, but this is still very, very impressive. 01:19:21.000 |
So a human written prompt was given to the language model in a shocking finding. Scientists discovered a herd of unicorns living in a remote previously unexplored valley in the Andes Mountains. 01:19:32.000 |
Even more surprising to the researchers was the fact that the unicorn spoke perfect English. And then the next part is all generated by the language model. 01:19:42.000 |
So this is a deep learning model that produced this and the computer model generated. Dr. Jorge Perez had what appeared to be a natural fountain surrounded by two peaks of rock and silver snow. 01:19:55.000 |
Perez and the others then ventured further into the valley. By the time we reached the top of one peak, the water looked blue with some crystals on tops at Perez. 01:20:04.000 |
Perez and his friends were astonished to see the unicorn herd. These creatures could be seen from the air without having to move too much to see them. They were so close they could touch their horns. 01:20:15.000 |
While examining these bizarre creatures, the scientists discovered that the creatures also spoke some fairly regular English. Perez stated we can see, for example, that they have a common language, something like a dialect or dialectic. 01:20:27.000 |
And so I think this is really compelling prose to have been have been generated by a computer in this form. 01:20:38.000 |
So we've also seen advances in computers generating pictures, specifically GANs. So Katie Jones was listed on LinkedIn as a Russia and Eurasia fellow. 01:20:51.000 |
She was connected to several people from mainstream Washington think tanks and the Associated Press discovered that she is not a real person. This photo was generated by a GAN. 01:21:05.000 |
And so this, I think, gets kind of scary when we start thinking about how how compelling the text that's being generated is and combining that with pictures. 01:21:15.000 |
These photos are all from this person does not exist dot com generated by GANs. 01:21:22.000 |
And there's a very, very real and imminent risk that online discussion will be swamped with fake manipulative agents to an even greater extent than it than it already has, and that this this can be used to influence public opinion. 01:21:39.000 |
So actually, I guess, well, I'll keep going. I'm going back in time to twenty seventeen. The FCC was considering repealing net neutrality. 01:21:51.000 |
And so they opened up for comments to see, you know, how do Americans feel about net neutrality? 01:21:56.000 |
And this is a sample of many of the comments that were opposed to net neutrality. They wanted to repeal it and included. 01:22:04.000 |
I'll just read a few clips. Americans, as opposed to Washington bureaucrats, deserve to enjoy the services they desire. 01:22:12.000 |
Individual citizens, as opposed to Washington bureaucrats, should be able to select whichever services they desire. 01:22:18.000 |
People like me, as opposed to so-called experts, should be free to buy whatever products they choose. 01:22:23.000 |
And these have been helpfully color coded. So you can kind of see a pattern that this was a bit of a mad libs where you had a few choices for green for the first noun. 01:22:35.000 |
And then in orange or red, I guess it's as opposed to or rather than orange, we've got either Washington bureaucrats, so-called experts, the FCC and so on. 01:22:49.000 |
And this analysis was done by Jeff Kao, who's now a computational journalist at ProPublica doing great work. 01:22:58.000 |
And he did this analysis discovering this campaign in which these comments were designed to look unique but had been created kind of through some mail merge style, kind of putting together a mad libs. 01:23:15.000 |
Yes. So this was great work by Jeff. He found that while they received this, the FCC received over 22 million comments, less than 4% of them were truly unique. 01:23:32.000 |
And this is not all malicious activity. You know, there are many kind of ways where you get a template to contact your legislator about something. 01:23:41.000 |
But, you know, in the example kind of shown previously, these were designed to look like they were unique when they weren't. 01:23:48.000 |
And more than 99% of the truly unique comments wanted to keep net neutrality. However, that was not the case if you looked at the full 22 million comments. 01:24:00.000 |
However, this was in 2017, which may not sound that long ago, but in the field of natural language processing, we've had like an entire kind of a revolution since then. 01:24:11.000 |
There's just been so much progress made. And this would be, I think, virtually impossible to catch today if someone was using a sophisticated language model to generate comments. 01:24:23.000 |
So Jess asks a question, which I'm going to treat it as a two-part question, even if it's not necessarily. What happens when there's so much AI trolling that most of what gets draped from the web is AI generated text? 01:24:36.000 |
And then the second part. And then what happens when you use that to generate more AI generated text? 01:24:41.000 |
Yeah, so for the first part, yeah, this is a real risk, or not risk, but kind of challenge we're facing of real humans can get drowned out when so much text is going to be AI trolling. 01:24:57.000 |
And we're already seeing, and I, in the interest of time, I can talk about disinformation for hours and I had to cut a lot of stuff out. 01:25:06.000 |
But many people have talked about how kind of the new form of censorship is about drowning people out. 01:25:14.000 |
And so it's not necessarily kind of forbidding someone from saying something, but just totally, totally just drowning them out with a massive quantity of text and information and comments. 01:25:27.000 |
And AI can really facilitate that. And so I do not have a good solution to that. 01:25:33.000 |
In terms of AI learning from AI text, I mean, I think you're going to get systems that are potentially kind of less and less relevant to humans and may have harmful effects if they're kind of being used to create software that is interacting with or impacting humans. 01:25:59.000 |
I mean, one of the things I find fascinating about this is we could get to a point where 99.99% of tweets and fast AI forum posts, whatever, are auto generated, particularly on kind of more like political type places where a lot of it's pretty low content, pretty basic. 01:26:20.000 |
And the thing is, like, if it was actually good, you wouldn't even know. So what if I told you that 75% of the people you're talking to on the forum right now are actually bots? 01:26:31.000 |
How can you tell which ones they are? How would you prove whether I'm right or wrong? 01:26:36.000 |
Yeah, I think this is a real issue on Twitter of particularly people you don't know of wondering, like, is this an actual person or a bot? I think it's a common question people wonder about and can be hard to tell. 01:26:55.000 |
But I think it has significance for has a lot of significance for kind of how human government works. You know, I think there's something about humans being in society and having norms and rules and mechanisms that this can really undermine and make difficult. 01:27:22.000 |
And so when when GPT two came out, Jeremy Howard, co founder of fast AI was quoted in the Verge article on it. I've been trying to warn people about this for a while. 01:27:35.000 |
We have the technology to totally fill Twitter email and the web up with reasonable sounding context appropriate pros, which would drown out all other speech and be impossible to filter. 01:27:49.000 |
So one kind of step towards addressing this is the need for digital signatures. Orin Etziani, the head of the Allen Institute on AI, wrote about this in HBR. 01:28:05.000 |
He wrote recent developments in AI point to an age where forgery of documents, pictures, audio recordings, videos and online identities will occur with unprecedented ease. 01:28:15.000 |
AI is poised to make high fidelity forgery inexpensive and automated, leading to potentially disastrous consequences for democracy, security and society and proposes kind of digital signatures as a means for authentication. 01:28:31.000 |
And I will say here, kind of one of the one of the additional risk of kind of all this forgery and fakes is that it also undermines people speaking the truth. 01:28:43.000 |
And Zeynep Tefekci, who does a lot of research on protests around the world and in different social movements, has said that she's often approached by kind of whistleblowers and dissidents who in many cases will risk their lives to try to publicize like a wrongdoing or human rights violation, 01:29:03.000 |
only to have kind of bad actors say, "Oh, that picture was photoshopped. That was faked." And that it's kind of now this big issue for whistleblowers and dissidents of how can they verify what they are saying and that kind of that need for verification. 01:29:21.000 |
And then someone you should definitely be following on this topic is Renee DiResta. 01:29:27.000 |
And she wrote a great article with Mike Godwin last year framing that we really need to think disinformation as a cyber security problem, you know, as these kind of coordinated campaigns of manipulation and bad actors. 01:29:41.000 |
And there's, I think, some important work happening at Stanford as well on this. 01:29:50.000 |
All right. Questions on disinformation? Okay, so next step, ethical foundations. 01:29:59.000 |
So now, so the fast AI approach, we always like to kind of ground everything in what are the real world case studies before we get to kind of the theory underpinning it. 01:30:08.000 |
And I'm not going to go too deep on this at all. 01:30:12.000 |
So there is a fun article, "What Would an Avenger Do?" and a hat tip to Casey Fiesler for suggesting this. 01:30:21.000 |
And it goes through kind of three common ethical philosophies, utilitarianism, and gives the example of Iron Man, trying to maximize good, deontological ethics of Captain America being an example of this, adhering to the right, 01:30:39.000 |
and then virtue ethics, Thor, living by a code of honor. And so I thought that was a nice reading. Yes. 01:30:50.000 |
"Where do you stand on the argument that social media companies are just neutral platforms and that problematic content is the entire responsibility of the users, just the same way that phone companies aren't held responsible when phones are used for scams or car companies are held responsible when vehicles are used for, say, terrorist attacks?" 01:31:10.000 |
So I do not think that the platforms are neutral because they make a number of design decisions and enforcement decisions around even kind of what their terms of service are and how those are enforced. 01:31:27.000 |
And that in keeping in mind, harassment can drive many people off of platforms. And so kind of many of those decisions is not that, oh, everybody gets to keep free speech when there's no enforcement, it's just changing kind of who is silenced. 01:31:48.000 |
I do think that there are a lot of really difficult questions that are raised about this because I also think that the platforms, you know, they are not publishers, but they are in this, I think, kind of an intermediate area where they're performing many of the functions that publishers used to perform. 01:32:13.000 |
So, you know, like a newspaper, which is curating which articles are in it, which is not what platforms are doing, but they are getting closer to that. 01:32:26.000 |
I mean, something I come back to is it is an uncomfortable amount of power for private companies to have. Yeah. 01:32:35.000 |
And so it does raise a lot of difficult decisions, but I do not believe that they are neutral. 01:32:42.000 |
So for this part, I mentioned the Marcula Center earlier. Definitely check out their site, Ethics and Technology Practice. They have a lot of useful resources. 01:32:54.000 |
And I'm going to go through these relatively quickly as just kind of examples. So they give some kind of deontological questions that technologists could ask. 01:33:02.000 |
And so deontological ethics are where you kind of have various kind of rights or duties that you might want to respect. 01:33:12.000 |
And this can include principles like privacy or autonomy. 01:33:20.000 |
How might the dignity and autonomy of each stakeholder be impacted by this project? 01:33:25.000 |
What considerations of trust and of justice are relevant? Does this project involve any conflicting moral duties to others? 01:33:34.000 |
In some cases, you know, there'll be kind of conflict between different rights or duties you're considering. 01:33:41.000 |
And so this is kind of an example, and they have more in the reading of the types of questions you could be asking kind of when evaluating of just even how do you evaluate kind of whether a project is ethical. 01:33:54.000 |
Consequentialist questions. Who will be directly affected? Who will be indirectly affected? 01:34:03.000 |
And consequentialist includes utilitarianism as well as common good. 01:34:09.000 |
Will the effects in aggregate create more good than harm and what types of good and harm? 01:34:15.000 |
Are you thinking about all the relevant types of harm and benefit, including psychological, political, environmental, moral, cognitive, emotional, institutional, cultural. 01:34:24.000 |
Also looking at long term benefits and harms. 01:34:28.000 |
And then who experiences them? Is this something where the risk of the harm are going to fall disproportionately on the least powerful? 01:34:35.000 |
Who's going to be the ones to accrue the benefits? 01:34:39.000 |
Have you considered dual use? So these are these are again kind of questions you could use when trying to trying to evaluate a project. 01:34:46.000 |
And I think in the recommendation of the Markkula Center is that this is a great activity to kind of to be doing as a team and as a group. 01:35:00.000 |
I was going to say like I can't. I can't overstate how useful this tool is like you might think, oh, it's just a list of questions. 01:35:10.000 |
But like this is kind of to me, this is the this is the big gun tool for how you how you handle this is like if somebody is helping you think about the right set of questions and then you like go through them with a diverse group of people and discuss the questions. 01:35:26.000 |
I mean, that's that it's this is this is gold. Like, you know, go back and reread these and don't don't just skip over them because take take them to work. 01:35:34.000 |
Use them next time you're talking about a project. They're a really great great set of questions to use a great tool in your toolbox. 01:35:42.000 |
Yeah. And go to the original reading has even kind of more detail and more elaboration on the questions. 01:35:51.000 |
And then they kind of give a summary of five potential ethical lenses, the rights approach, which option best respects the rights of all who have a stake, the justice approach, which option treats people equally or proportionally. 01:36:05.000 |
And so these two are both deontological the utilitarian approach, which option will produce the most good and do the least harm, the common good approach, which option best serves the community as a whole, not just some members. 01:36:19.000 |
And so here, three and four are both consequentialist and then virtue approach, which option leads me to act as a sort of person I want to be and that can involve particular virtues of, you know, do you value trustworthiness or truth or courage. 01:36:37.000 |
And so, I mean, a great activity if this is something that you're studying or talking about at work with your teammates, the Marcula Center has a number of case studies that you can talk through and we'll even ask you to kind of evaluate them, you know, evaluate them through these five lenses 01:36:52.000 |
and how does that kind of impact your your take on what the what the right thing to do is. 01:37:01.000 |
It's kind of weird for a programmer a computer programmer data science in some ways in some ways to like think of these as tools like last day I or pandas or whatever. 01:37:12.000 |
But I mean, they absolutely are. This is like these like software tools for your brain, you know, to help you kind of go through a program that might help you debug your thinking. 01:37:29.000 |
And then as someone brought up earlier, so that was a kind of very Western centric intro to ethical philosophy. There are other ethical lenses and other cultures and I've been doing some reading, particularly on the the Maori worldview. 01:37:43.000 |
I don't feel confident enough in my understanding that I could represent it, but it is very good to be mindful that the other other other ethical lenses out there. 01:37:53.000 |
And I do very much think that, you know, the people being impacted by a technology like their their ethical lens is kind of what matters and that this is is a particular issue when we have so many kind of a multinational corporations. 01:38:08.000 |
And there's a interesting project going on in New Zealand now where the New Zealand government is kind of considering its AI approach and is at least ostensibly kind of wanting to wanting to include the Maori view on that. 01:38:22.000 |
So that's a that's kind of a little a little bit of theory, but now I want to talk about some kind of practices you can implement in the workplace. 01:38:29.000 |
Again, this is from the Markula Center. So this is their ethics toolkit, which I particularly like. 01:38:36.000 |
And I'm just I'm not going to go through all of them. I'm just going to tell you a few of my favorites. 01:38:40.000 |
So tool one is ethical risk sweeping. And this, I think, is similar to the idea of kind of pen testing that Jeremy mentioned earlier from security, but to have regularly scheduled ethical risk sweeps. 01:38:56.000 |
And while no vulnerability, vulnerability is found is generally good news, that doesn't mean that it was a wasted effort and you keep doing it. 01:39:06.000 |
Keep looking for for ethical risk one moment and then assume that you miss some risk in the initial project development. 01:39:14.000 |
Also, you have to set up the incentives properly where you're rewarding team members for spotting new ethical risk. 01:39:22.000 |
So I've got some comments here. My comment here is about the learning rate finder. 01:39:27.000 |
And I'm not going to bother with the exact mathematical definition, partly because I'm a terrible mathematician and partly because it doesn't matter. 01:39:33.000 |
But if you just remember, oh, sorry, that's actually not me. 01:39:36.000 |
I am just reading something that Patty Hendricks has trained a language model of me. 01:39:42.000 |
So that was me reading the language model of me. 01:39:52.000 |
This is a tool one. I would say another kind of example of this, I think, is like red teaming of, you know, having a team within your org that's kind of trying to find your vulnerabilities. 01:40:03.000 |
Tool three, another one I really like expanding the ethical circle. So whose interests, desires, skills, experiences and values have we just assumed rather than actually consulted. 01:40:16.000 |
Who are all the stakeholders who will be directly affected and have we actually asked them what their interests are? 01:40:25.000 |
Who might use this product that we didn't expect to use it or for purposes that we didn't initially intend? 01:40:31.000 |
And so then a great implementation of this comes from the University of Washington's tech policy lab did a project called Diverse Voices. 01:40:44.000 |
And it's neat. They have both a academic paper on it and then they also kind of have like a guide, lengthy guide on how you would implement this. 01:40:53.000 |
But the idea is how to kind of organize expert panels around new technology. 01:41:02.000 |
And so they did a few samples. One was they're considering augmented reality and they held expert panels with people with disabilities, people who are formerly or currently incarcerated and with women to get their input and make sure that that was included. 01:41:17.000 |
They did a second one on an autonomous vehicle strategy document and organized expert panels with youth, with people that don't drive cars and with extremely low income people. 01:41:28.000 |
And so I think this is a great guide if you're kind of unsure of how do you even go about setting something like this up to expand your circle, include more people and get perspectives that may be underrepresented by your employees. 01:41:45.000 |
So I just want to let you know that this resource is out there. 01:41:52.000 |
Tool six is think about the terrible people. And this can be hard because I think we're often, you know, thinking kind of positively or thinking about people like ourselves who don't have terrible intentions. 01:42:09.000 |
But really think about who might want to abuse, steal, misinterpret, hack, destroy, or weaponize what we build. 01:42:17.000 |
Who will use it with alarming stupidity or irrationality? 01:42:21.000 |
What rewards, incentives, openings has our design inadvertently created for those people? 01:42:26.000 |
And so kind of remembering back to the section on metrics, you know, how are people going to be trying to game or manipulate this? 01:42:34.000 |
And how can how can we then remove those rewards or incentives? And so this is this is an important kind of important step to take. 01:42:44.000 |
And then tool seven is closing the loop, ethical feedback and iteration, remembering this is never a finished task and identifying feedback channels that will give you kind of reliable data and integrating this process with quality management and user support 01:43:01.000 |
and developing formal procedures and chains of responsibility for ethical iteration. 01:43:06.000 |
And this tool reminded me of a blog post by Alex Pierce that I really like. Alex Pierce was previously the chief legal officer at Medium. 01:43:15.000 |
And I guess this was a year ago. He interviewed something like 15 or 20 people that have worked in trust and safety. 01:43:23.000 |
And trust and safety includes content moderation, although it's not not solely content moderation. 01:43:29.000 |
And kind of one of the ideas that came up that I really liked was one of one of the people and so many of these people have worked in trust and safety for years at big name companies. 01:43:40.000 |
And one of them said the separation of product people and trust people worries me because in a world where product managers and engineers and visionaries cared about this stuff, it would be baked into how things get built. 01:43:51.000 |
If things stay this way, that product and engineering are Mozart and everyone else's Alfred the butler, the big stuff is not going to change. 01:43:59.000 |
And so I think at least two people in this kind of talk about this idea of needing to better integrate trust and safety, which are often kind of on the front lines of seeing abuse and misuse of a technology product, integrating that more closely with product and 01:44:13.000 |
end so that it can kind of be more directly incorporated and you can have a tighter feedback loop there about what's going wrong and and how how that can be designed against. 01:44:26.000 |
Okay, so those were these were, well, I linked to a few blog posts and research I thought relevant but inspired by the mark my coolest centers tools for tech ethics and hopefully those are practices you could think about potentially implementing at your at your company. 01:44:45.000 |
So next I want to get into diversity, which I know came up earlier. 01:44:51.000 |
So only 12% of machine learning researchers are women. This is kind of a very, very dire statistic. 01:45:01.000 |
There's also kind of extreme lack of racial diversity and age diversity and other factors. 01:45:12.000 |
A kind of positive example of what diversity can help with and a post Tracy chow who was a early, early engineer at Quora and later at Pinterest wrote that the first feature and so I think she was like one of the first five employees at Quora. 01:45:28.000 |
The first feature I built when I worked at Quora was the block button. I was eager to work on the feature because I personally felt antagonized and abused on the site. 01:45:36.000 |
And she goes on to say that if she hadn't been there, you know, they might not have added the block button as soon as that's kind of like a direct example of how how having a diverse team can help. 01:45:47.000 |
So my kind of key, key advice for anyone wanting to increase diversity is to start at the opposite end of the pipeline from from where people talk about the workplace. 01:46:00.000 |
I wrote a blog post five years ago. If you think women in tech is just a pipeline problem, you haven't been paying attention. And this was the most popular thing I had ever written until Jeremy and I wrote the the COVID-19 post last month. 01:46:13.000 |
So the second most most popular thing I've written. 01:46:17.000 |
But I linked to a ton of ton of research in there. A key statistic to understand is that 41% of women working in tech end up leaving the field compared to 17% of men. 01:46:29.000 |
And so this is something that recruiting more girls into into coding or tech is not going to address this problem if they keep leaving at very high rates. 01:46:39.000 |
I just had a little peek at the YouTube chat and I see people are asking questions there. I just wanted to remind people that we are not that Rachel and I do not look at that. 01:46:50.000 |
If you want to ask questions, you should use the forum thread. And if you see questions that you'd like, then please vote them up, such as this one. 01:47:01.000 |
How about an ethical issue bounty program, just like the bug bounty programs that some companies have? 01:47:10.000 |
No, I think that's a neat idea. Yeah, rewarding people for for finding ethical issues. 01:47:17.000 |
And so the reason that women are more likely to leave tech is and this was found in a meta analysis of over 200 books, white papers, articles. 01:47:29.000 |
Women leave the tech industry because they're treated unfairly, underpaid, less likely to be fast-tracked than their male colleagues and unable to advance. 01:47:38.000 |
And too often, diversity efforts end up just focusing on white women, which is wrong. 01:47:45.000 |
Interviews with 60 women of color who work in STEM research found that 100 percent had experienced discrimination and their particular stereotypes varied by race. 01:47:53.000 |
And so it's very important to focus on women of color in diversity efforts as a kind of the top priority. 01:48:04.000 |
A study found that men's voices are perceived as more persuasive fact-based and logical than women's voices, even when reading identical scripts. 01:48:15.000 |
Researchers found that women receive more vague feedback and personality criticism and performance evaluations, whereas men are more likely to receive actionable advice tied to concrete business outcomes. 01:48:27.000 |
When women receive mentorship, it's often advice on how they should change and gain more self-knowledge. When men receive mentorship, it's public endorsement of their authority. 01:48:36.000 |
Only one of these has been statistically linked to getting promoted. It's the public endorsement of authority. 01:48:42.000 |
And all these studies are linked to in another post I wrote called The Real Reason Women Quit Tech and How to Address It. 01:48:48.000 |
Is that a question, Jeremy? Yeah. So if you're interested, kind of these two blog posts I linked to a ton of relevant research on this. 01:48:59.000 |
And I think this is kind of the workplace is the place to start in addressing these things. 01:49:07.000 |
So another issue is tech interviews are terrible for everyone. 01:49:13.000 |
So now kind of working one step back from people that are already in your workplace, but thinking about the interview process. 01:49:20.000 |
And I wrote a post on how to make tech interviews a little less awful and went through a ton of research. 01:49:25.000 |
And I will say that the interview problem, I think, is a hard one. I think it's very time consuming and hard to interview people well. 01:49:35.000 |
But kind of the two most interesting pieces of research I came across one was from Triple Bite, which is a recruiting company that interviews kind of does this first round technical interview for people. 01:49:50.000 |
And then they interview at Y Combinator. It's a Y Combinator company. And then they interview at Y Combinator companies. 01:49:57.000 |
And so they have this very interesting data set where they've kind of given everybody the same technical interview and then they can see which companies people got off. 01:50:04.000 |
And these people got offers from when they were interviewing at many of the same companies. 01:50:09.000 |
And the number one finding from their research is that the types of programmers that each company looks for often have little to do with what the company needs or does. 01:50:18.000 |
Rather, they reflect company culture and the backgrounds of the founders. 01:50:22.000 |
And this is something where they even gave the advice of if you're job hunting, try to look for companies where the founders have a similar background to you. 01:50:33.000 |
And that's something that makes sense. That's going to be much easier for certain people to do than others, in particular, given the gender and racial disparities in VC funding. 01:50:48.000 |
Actually, I would say that was the most common advice I heard from VCs when I became a founder in the Bay Area was when recruiting focus on getting people from your network and people that are as like minded and similar as possible. 01:51:06.000 |
That was by far the most common advice that I heard. 01:51:09.000 |
Yeah, I mean, this is maybe like one of my controversial opinions. I do feel like ultimately like I get why people hire from their network. 01:51:18.000 |
And I think that long term we all need to be developed. 01:51:22.000 |
Well, particularly white people need to be developing more diverse networks. 01:51:25.000 |
And that's like a 10 year project. That's not something you can do right when you're hiring, but really kind of developing a diverse network of friends and trusted acquaintances kind of over time. 01:51:41.000 |
But yeah, thank you for that perspective to Jeremy. 01:51:45.000 |
And then kind of the other study I found really interesting was one where they they gave people resumes and in one case. 01:51:55.000 |
So one resume had more academic qualifications and then one had more practical experience and then they switched the gender. 01:52:02.000 |
One was a woman. One was a man or male name, a female name. 01:52:06.000 |
And basically, people were more likely to hire the male and then they would use a post hoc justification of, oh, well, I chose him because he had more academic experience. 01:52:14.000 |
Or I chose him because he had more practical experience. 01:52:17.000 |
And that's something that I think it's very human to use post hoc justifications. 01:52:22.000 |
But it's a real risk that definitely shows up in hiring. 01:52:29.000 |
Ultimately, AI or any other technology developed or implemented by companies for financial advantage, i.e. more profit. 01:52:37.000 |
Maybe the best way to incentivize ethical behavior is to tie financial or reputational risk to good behavior. 01:52:43.000 |
In some ways, similar to how companies are now investing in cybersecurity because they don't want to be the next Equifax. 01:52:49.000 |
Can grassroots campaigns help in better ethical behavior with regards to their use of AI? 01:52:57.000 |
Yeah. And I think there are a lot of analogies with cybersecurity. 01:53:00.000 |
And I know that for a long time, I think it was hard for people to make or people had trouble making the case to their bosses of why they should be investing in cybersecurity, particularly because cybersecurity is, you know, 01:53:12.000 |
something like when it's working well, you don't notice it. 01:53:16.000 |
And so that can be can be hard to build the case. 01:53:19.000 |
So I think that there there is a place for grassroots campaigns. 01:53:24.000 |
And I'm going to talk more about policy in a bit. 01:53:30.000 |
It can be hard in some of these cases where there are not necessarily meaningful alternatives. 01:53:39.000 |
So I do think like monopolies can kind of kind of make that harder. 01:53:52.000 |
All right. So next step actually on this slide is the need for policy. 01:53:59.000 |
And so I'm going to start with a case study of what's what's one thing that gets companies to take action. 01:54:06.000 |
And so, as I mentioned earlier, an investigator for the U.N. found that Facebook played a determining role in the Rohingya genocide. 01:54:16.000 |
I think the best article I've read on this was by Timothy McLaughlin, who did a super, super in-depth dive on Facebook's role in Myanmar. 01:54:26.000 |
And people people warned Facebook executives in 2013 and in 2014 and in 2015 how the platform was being used to spread hate speech and to incite violence. 01:54:40.000 |
One person in 2015 even told Facebook executives that Facebook could play the same role in Myanmar that the radio broadcast played during the Rwandan genocide. 01:54:50.000 |
And radio broadcast played a very terrible and kind of pivotal role in the Rwandan genocide. 01:54:57.000 |
Somebody close to it said that's not 2020 hindsight. 01:55:00.000 |
The scale of this problem was significant and it was already apparent. 01:55:04.000 |
And despite this, in 2015, I believe Facebook only had four contractors who even spoke Burmese, the language of Myanmar. 01:55:16.000 |
That's an interesting one. How do you think about our opportunity to correct biases in artificial systems versus the behaviors we see in humans? 01:55:25.000 |
For example, a sentencing algorithm can be monitored and adjusted versus a specific biased judge who remains in their role for a long time. 01:55:35.000 |
I mean, theoretically, though, I think I feel a bit hesitant about the it's it'll be easier to correct bias in algorithms because I feel like the 01:55:55.000 |
you still need people kind of making the decisions to prioritize that like it requires kind of an overhaul of the system's priorities, I think. 01:56:08.000 |
It also starts with the premise that there are people who can't be fired or disciplined or whatever. 01:56:15.000 |
I guess maybe for some judges that's true, but that kind of maybe suggests that judges shouldn't be lifetime appointments. 01:56:26.000 |
Yeah, even then, I think you kind of need the change of heart of the people advocating for the new system, which I think can 01:56:35.000 |
would be necessary in other case, kind of, and that that's kind of the critical piece of getting the people that are wanting to overhaul the values of a system. 01:56:45.000 |
So returning to this issue of the Rohingya genocide, and this is kind of continuing issue. 01:56:57.000 |
This is something that's just kind of really stunning to me that that there were so many warnings and that so many people tried to raise an alarm on this and that 01:57:08.000 |
so little action was taken. And even this was last year. 01:57:15.000 |
Zuckerberg finally said that Facebook would add or maybe maybe this was actually this was probably two years ago, said that Facebook would add. 01:57:22.000 |
But this is, you know, after genocide is already happening, Facebook would add dozens of Burmese language content reviewers. 01:57:31.000 |
So in contrast, so we have this. This is how Facebook really failed to respond in any any significant way in Myanmar. 01:57:41.000 |
Germany passed a much stricter law about hate speech and that's D.G. DZ. And the the potential penalty would be up to like 50 million euros. 01:57:57.000 |
Facebook hired 1200 people in under a year because they were so worried about this penalty. And so and I'm not saying that like this is a law we want to replicate. 01:58:06.000 |
Here I'm just illustrating the difference between being told that you're contributing or playing a determining role in a genocide versus a significant financial penalty. 01:58:18.000 |
We have seen what the one thing that makes Facebook take action is. 01:58:22.000 |
And so I think that that is really significant in remembering what the what the power of a credible threat of a significant fine is. 01:58:32.000 |
And it has to be a lot more than just like a cost of doing business. 01:58:39.000 |
So I I really believe that we need both policy and ethical behavior within industry. 01:58:46.000 |
I think that policy is the appropriate tool for addressing negative externalities, misaligned economic incentives, race to the bottom situations and enforcing accountability. 01:58:58.000 |
However, ethical behavior of individuals and of data scientists and software engineers working in industry is very much necessary as well because the law is not always going to keep up. 01:59:08.000 |
It's not going to cover all the edge cases. We really need the people in industry to be making kind of ethical ethical decisions as well. 01:59:15.000 |
And so I believe both are significant and important. 01:59:21.000 |
And then something to note here is that many many examples of kind of ethics issues. 01:59:29.000 |
And I haven't talked about all of these, but there was Amazon's facial recognition. The ACLU did a study finding that it incorrectly matched 28 members of Congress to criminal mugshots. 01:59:42.000 |
And this disproportionately included Congresspeople of color. 01:59:47.000 |
There's also this was a terrible article, not that the article was good, but the story is terrible of a city that's using this IBM dashboard for predictive policing. 01:59:58.000 |
And a city official said, oh, like whenever you have machine learning, it's always 99 percent accurate, which is false and quite concerning. 02:00:08.000 |
We had we had the issue in 2016 ProPublica discovered that you could place a housing ad on Facebook and say, you know, like, I don't want Latino or black people or I don't want wheelchair users to see this housing ad, which seems like a violation of the Fair Housing Act. 02:00:27.000 |
And so there's this article and Facebook was like, we're so sorry. And then over a year later, it was still going on. ProPublica went back and wrote another article about it. 02:00:38.000 |
There's also this issue of dozens of companies were placing ads on Facebook job ads and saying, like, we only want young people to see this. 02:00:48.000 |
There's the Amazon creating the recruiting tool that penalized resumes that had the word women's in it. 02:00:57.000 |
And so something something to note about these examples and many of the examples we've talked about today is that many of these are about human rights and civil rights. 02:01:07.000 |
And it's a good article by Dominique Harrison of the Aspen Institute on this. And I kind of agree with Aneel Dash's framing. 02:01:16.000 |
And he wrote, there is no technology industry anymore. Tech is being used in every industry. 02:01:22.000 |
And so I think in particular, we need to consider human rights and civil rights such as housing, education, employment, criminal justice, voting and medical care and think about what rights we we want to safeguard. 02:01:35.000 |
And I do think policy is the appropriate way to do that. 02:01:41.000 |
And I think I mean, it's very easy to be discouraged about about regulation, but I think sometimes we overlook the the positive or the cases where where it's worked well. 02:01:55.000 |
And so something I really liked about data sheets for data sets by Timnit Gebru et al is that they go through three case studies of how standardization and regular regulation came to different industries. 02:02:09.000 |
And so it's the electronics industry around circuits and resistors. And so there, that's kind of around the standardization of what the specs are and what you write down about them, the pharmaceutical industry and car safety. 02:02:20.000 |
And none of these are perfect, but it's still it was a kind of very illuminating the case studies there. 02:02:26.000 |
And in particular, I got very interested in the car safety one. And there's also a great 99 percent invisible episode. 02:02:33.000 |
This is a design podcast about it. And so some things I learned is that early cars had sharp metal knobs on the knobs on the dashboard that could lodge in people's skulls in a crash. 02:02:47.000 |
Non collapsible steering columns would frequently impale drivers. And then even after the collapsible steering column was invented, it wasn't actually implemented because there was no economic incentive to do so. 02:02:58.000 |
But it's the collapsible steering column has this had saved more lives than anything other than the seat belt when it comes to car safety. 02:03:08.000 |
And there was also this just this widespread belief that cars were dangerous because of the people driving them. And it took it took consumer safety advocates decades to just even change the culture of discussion around this and to start kind of gathering and tracking the data and to put more of an onus on car companies around safety. 02:03:27.000 |
GM hired a private detective to trail Ralph Nader and try to dig up dirt on him. And so this was really a battle that we kind of I take for granted now. 02:03:39.000 |
And so kind of shows how much how much it can take to to change change the needle there. And then a kind of a more recent issue is that it wasn't until I believe 2011 that it was required that crash test dummies start representing the average female anatomy. 02:03:59.000 |
In addition to previously was kind of just crushed test dummies were like men and that in a crash of the same impact, women were 40 percent more likely to be injured than men because that's kind of who the cars were being designed for. 02:04:14.000 |
So I thought I thought all this was very interesting and it can be helpful to kind of remember and remember some of the successes we've had. 02:04:21.000 |
And another area that's very relevant is environmental protections and kind of looking back and. 02:04:32.000 |
Maychick Seglowski has a great article on this. But you know, just remembering like in the U.S., we used to have rivers that would catch on fire and London had terrible, terrible smog and that these are things that were very would not have been possible to kind of solve as an individual. 02:04:47.000 |
We really needed kind of coordinated, coordinated regulation on. 02:04:52.000 |
All right. And so then on a kind of closing note, so I think a lot of the problems I've touched on tonight are really huge, huge and difficult problems and they're often kind of very complicated. 02:05:09.000 |
I go into more detail on this in the course. So please, please check out the course once it's once it's released. 02:05:14.000 |
I always try to offer some like steps towards solutions, but I realize they're not they're not always, you know, as satisfying as I would like of like this is going to solve it. 02:05:24.000 |
And that's because these are really, really difficult problems. And Julia Angwin, a former journalist from ProPublica and now the editor in chief of The Markup gave a really great interview on privacy last year that I liked and found very encouraging. 02:05:42.000 |
And she said, I strongly believe that in order to solve a problem, you have to diagnose it and that we're still in the diagnosis phase of this. 02:05:51.000 |
If you think about the turn of the century and industrialization, we had, I don't know, 30 years of child labor, unlimited work hours, terrible working conditions. 02:06:01.000 |
And it took a lot of journalists muckracking and advocacy to diagnose the problem and have some understanding of what it was and then the activism to get laws changed. 02:06:13.000 |
I see my role as trying to make as clear as possible what the downsides are and diagnosing them really accurately so that they can be solvable. 02:06:20.000 |
That's hard work and lots more people need to be doing it. 02:06:23.000 |
I find that really encouraging and that I do think we should be working towards solutions, but I think just at this point, even better diagnosing and understanding kind of the complex problems we're facing is valuable work. 02:06:38.000 |
A couple of people are very keen to see your full course on ethics. Is that something that they might be able to attend or buy or something? 02:06:47.000 |
So it will be released for free at some point this summer. 02:06:53.000 |
And there was a paid in-person version offered at the Data Institute as a certificate, kind of similar to how this course was supposed to be offered in person. 02:07:05.000 |
The Data Ethics one was in person and that took place in January and February. 02:07:09.000 |
And then I'm currently teaching a version version for the Masters of Data Science students at USF and I will be releasing the free online version later, sometime before July.