back to indexMulti-Agent Frontiers: Transforming Customer Experience with Cisco
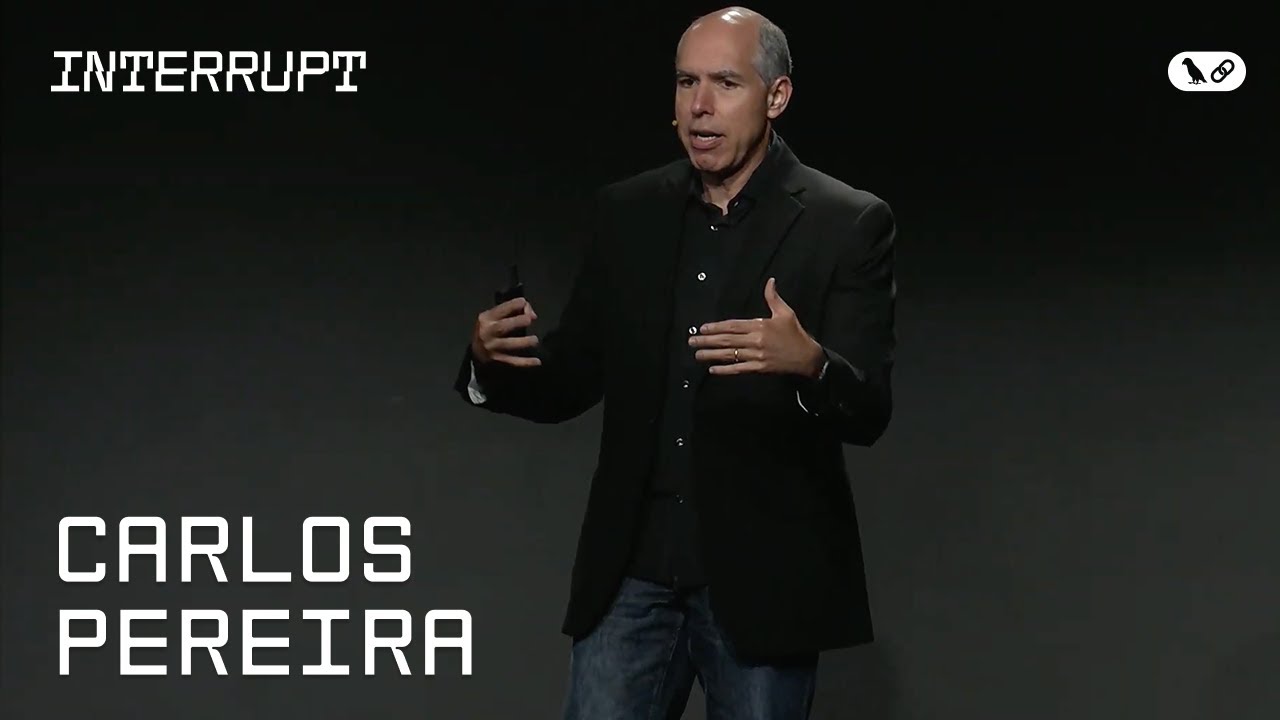
00:00:00.000 |
I know it's tough being after the break and after what was a nice conversation, fireside 00:00:15.320 |
And I was talking with Harrison backstage on how much of these beliefs that he has for 00:00:19.920 |
the future we have been implementing and the plan is to show with some of you today. 00:00:24.220 |
So Cisco is a company that many of you may know that there is a lot of networking stuff 00:00:32.360 |
We are over 40 year company and there is customer experience inside Cisco. 00:00:38.260 |
Customer experience for us go on a wide range of services that we provide. 00:00:42.640 |
I'll share with you a little bit on the first topic, then deep dive on what the use cases 00:00:46.880 |
and how we're using technology together with LangChain on the partnership to accomplish the 00:00:51.080 |
results that we're getting, and then share with you some key learners and takeaways along 00:00:55.920 |
Some of the good scarves, some of the learners don't do this, do that kind of thing, okay? 00:01:00.500 |
So fourth thing so we can get all the way so you can all understand what I'm talking about. 00:01:07.220 |
Cisco is a $56 billion plus company with half of the recurring revenue of the company. 00:01:13.020 |
So we have more than $26 billion that runs on this. 00:01:17.260 |
So talk with LangChain like, hey, you're not joking here. 00:01:19.920 |
So there is a lot of implications for that and everything on customer experience is meant 00:01:24.760 |
to maximize the value that the customer gets on investment they do on Cisco products or services 00:01:30.640 |
So we have the typical land, adopt, expand and renew framework that everybody has on the 00:01:40.340 |
But when you talk about any company, there is always process, people and technology. 00:01:46.040 |
In those kind of conferences, a lot of people think about the technology. 00:01:48.760 |
There is a lot of hype but they always come together with the teams that implement that and the process 00:01:59.440 |
So we have land, adopt, expand and renews and actually there are people and organizations 00:02:02.440 |
So we have a customer success organization that actually is in charge or when someone acquires 00:02:10.440 |
There is an adoption team that makes sure that you adopt whatever you buy for, right? 00:02:15.440 |
And there is an organization that is renews to make sure that when the end of the term 00:02:20.280 |
comes, you are happy enough to actually renew your subscription. 00:02:23.280 |
And usually more often than not, during that period, we expand our relationship with the customer. 00:02:29.120 |
There is obviously technical support, which a lot of you match to this, and other organizations 00:02:36.120 |
I'm talking about 20,000 people organization north of this. 00:02:40.120 |
So there's a lot of things that could be improved. 00:02:42.960 |
And from that lens, what you're doing is adopting AI to help us, not only on optimizing the process, 00:02:50.160 |
but optimizing for people and maximize their returns to the business. 00:02:53.960 |
And that's a very important thing because if you look on the left hand of this slide, I'm 00:02:58.320 |
talking about how we make customer experience unimaginable. 00:03:02.520 |
And by that, I'm going to hyper-personalization, proactive and predictiveness. 00:03:07.340 |
But we are not talking about agentic AI overall. 00:03:10.960 |
It doesn't come out of the blue and like, hey, agentic AI is a new thing. 00:03:14.760 |
We start with machine learning predictive AI models over a decade ago. 00:03:19.620 |
So treat very well your data science team because they're going to be critical now. 00:03:23.680 |
When it comes from the LLMs, Gen AI, which are very good for everything that has to do with 00:03:28.540 |
interactions on language, there is an L on the middle of this for a reason, LLM is really 00:03:35.480 |
So when you bring this together, we can have multiple agents that go for workflows. 00:03:41.120 |
So with that said, our vision and the future of Cisco customer experience, and you have been 00:03:47.280 |
leading on this for over two years now, and a lot of companies are coming to help understand 00:03:53.860 |
And that's part of the reason why in this conference on the very strong partnership we have with 00:03:57.820 |
and Harrison and his team, we are going for using agentic AI to elevate CX to become an agentic 00:04:04.860 |
CX by providing personalized, predictive and proactive experience to all of our customers, together, not separate. 00:04:13.680 |
Hyper-personalization, how we can predict fails before they happen, how it can be proactive of field notice, or even best practice that goes there. 00:04:20.720 |
We are leveraging multi-agents. By multi-agents, you're going to see on the next slide what I mean by that. 00:04:25.580 |
There's human and machine type of agents, Gen AI, and traditional AM. 00:04:30.440 |
We are providing services that goes for users that call them, like you can call on a video call, on a chat interface, or a phone call, or to have a tool. 00:04:40.040 |
And the agentic CX provides a lot of embedded value, like advanced technical support, predictive intelligence operations, and all of this is meant to help customers to have recommendations that are proactive. 00:04:56.500 |
So, we want to avoid them to face an issue, if we can, upfront. 00:05:00.340 |
We want to give them predictive insights, and make this hyper-personalized foresight on a sense that every customer should have an agent on themselves, which has context for that. 00:05:12.360 |
So, context is a very important thing for us, and we are going beyond what we call about MCP context. 00:05:22.500 |
With that said, let me go, now that you're all PhD, what we do at Cisco, let me talk a little bit about use cases, and why we are drive from a use cases approach, as opposed to a tools approach. 00:05:33.880 |
So, just sharing an experience for here, when you start about a year and a half ago, obviously, GPT was the big name in town, and everybody was trying to try, GPT 3.0 was really bad back then, but it still was novel, right, completely novel. 00:05:49.840 |
But, before we have everybody trying to do a chatbot for whatever reason there was, so the first thing is, before we even go to the use cases, we define the criteria that would make the use case belong in the first place. 00:06:04.800 |
Because, we have an advisory part of our services, we enter a customer that the customer had 412 use cases for AI, and when you talk with them, it ends up being five that actually collaborate to the business. 00:06:24.800 |
So, we define that criteria to say, any use case that we do on customer experience, remember, it's 20,000 people organization, there's a lot of creative people and a lot of ideas. 00:06:35.800 |
Like, it must fit one of those three buckets. 00:06:38.800 |
We must have use cases that help customers get immediate value and maximize what they invest in us, and that's where renews and adoption as an agent goes in. 00:06:48.760 |
Same applies on how you make the operations of these people more secure and reliable. 00:06:52.760 |
That's where everything with support would go in, and then the whole correlation and agentic workflows provide visibility and insights across the whole life cycle. 00:07:01.760 |
So, there is a method to the madness here, if you think, and if you leave it alone, people are going to do their own thing, and it's going to come to you and say, "Hey, how cool it is." 00:07:13.760 |
So, define the use case criteria first, then put the use case on top of this, and that's how we've been structured to work with LinkChain, because now we stitch the pieces. 00:07:22.760 |
Because the agents, developers, and multi-organizations make sense to the customer in the end. 00:07:26.760 |
So, with that said, we obviously have a high-level stack. 00:07:31.760 |
We have the team on the booth that goes deeper, including on what we demoed yesterday. 00:07:36.760 |
So, we start with the need, for our use case, to have flexible deployment models. 00:07:41.760 |
What I mean by that, we have customers, like federal customers, health care, and some others, that require on-premises deployment. 00:07:48.760 |
By on-premises, I'm not talking a VPC on AWS. 00:07:50.760 |
I'm talking a physical data center with the devices. 00:07:55.760 |
So, we need to choose some criteria, like security, compliance. 00:08:01.760 |
There are customers in Europe that have heavy regulations. 00:08:07.760 |
The bulk of our business is B2B, business-to-business, not only business-to-consumer. 00:08:11.760 |
So, when you do for that, we start to power the best-in-class AI technology. 00:08:16.760 |
So, we chose Mistral-Large, and we worked very closely with Mistral, even to the development of their models, to run on-premises. 00:08:23.760 |
And we have both Sonnet, the latest from Sonnet, 3.7, and shartgpt from 4.1 all the way to 0.3 for some of the use cases. 00:08:33.760 |
That is empowered all together for Langchain. 00:08:35.760 |
In the demo, you're going to see that you have the same agentic framework running on-premises on a data center, 00:08:43.760 |
So, what he's saying on the belief, we've been doing this in production for a long time at scale. 00:08:48.760 |
So, what Harrison said is not high level, it actually works. 00:08:52.760 |
It's an interesting thing, and we used the agentics on multiple agents on the top, 00:09:00.760 |
By custom AI models, I'm talking about both creating machine learning models that are for predictions, 00:09:05.760 |
that we train for the signals, and fine-tuning LLMs, especially the on-prems, 00:09:11.760 |
to accomplish high accuracy in some of our use cases. 00:09:14.760 |
With that said, here I want to do one slide before I deep dive on some of the tech aspects. 00:09:20.760 |
So, remember that I mentioned to you the process, land, adopt, expand, and renew, and the people. 00:09:25.760 |
So, now I'm going to land the technology on top of that. 00:09:28.760 |
So, if you look at the use cases, we have shipping deployed in production at scale for over six months. 00:09:37.760 |
Renews agent that applies to the renews team with predictive insights. 00:09:41.760 |
This is LLM combination with machine learning. 00:09:44.760 |
We have support with the virtual tech engineers that augment our support people to actually go, 00:09:53.760 |
Automate resolution of the low priority case without human touch at scale. 00:09:58.760 |
I'm talking about 1.6 to 1.8 million cases a year. 00:10:04.760 |
And beyond that, integrating this directly on the product and sentiment analysis across the whole life cycle. 00:10:12.760 |
But I want to highlight with you, we have stuff that is deployed. 00:10:18.760 |
Experimentation and production are two different beasts. 00:10:25.760 |
We have the subject matter experts and the cohort to work with us. 00:10:29.760 |
So, I understand what questions they're going to ask. 00:10:31.760 |
Because the renews people is going to ask renews related questions. 00:10:35.760 |
The adoption people, adoption related questions. 00:10:37.760 |
It sounds obvious, but a lot of people don't think this way. 00:10:41.760 |
They interact with the customer after they develop the cool stuff. 00:10:51.760 |
So, but at the same time, even though we have limited availability. 00:10:54.760 |
And extending with the length chain for the supervisor approach that we're going to show. 00:10:58.760 |
We also have a lot that is on experimentation. 00:11:01.760 |
Because we learn to build pipelines for the new use cases that are coming. 00:11:12.760 |
So, let me take one of those as an example on the renews system. 00:11:17.760 |
The business value that we start this is why do I need to build the renews agent? 00:11:23.760 |
Well, over half of my business is recurring revenue. 00:11:27.760 |
And I have a lot of time that these people is wasting just trying to chase dashboards and tools. 00:11:33.760 |
So, anything that I can give back and remove operational burden for them means and translates directly correlation 00:11:40.760 |
Less time spending on doing useless stuff means more renews that they're chasing, means more business that go on. 00:11:48.760 |
There is a direct correlation to the business. 00:11:54.760 |
But at the same time, we wanted to correlate this with real-time sentiment to the customers and provide summarization that are hyper-personalized to the person. 00:12:01.760 |
So, you are renews on financial services industry. 00:12:04.760 |
So, I only explain what's the new trends for financial services, which are completely different than healthcare or the government, people that deal with. 00:12:12.760 |
So, with that said, we had over multiple 50 different data sets that were going around. 00:12:19.760 |
a zoo of tools, as you can imagine, for every renew event. 00:12:23.760 |
And the results for us was actually a reduction on 20% of the time, used in less than three weeks, and limited availability. 00:12:32.760 |
So, the business impact was immediately, and we have high accuracy of risk recommendation. 00:12:39.760 |
I was the one that the team loves and hates me at that point, that I said, we're going to go 95% accuracy and higher. 00:12:47.760 |
We explained this yesterday, and people on the booth can tell you how we got there. 00:12:51.760 |
So, let me go a little bit on the weeds here. 00:12:54.760 |
So, let me go a little, explain how the agentic flow works for us. 00:12:59.760 |
So, here's a question, typical question for the renews person. 00:13:03.760 |
What's the upcoming renew status for customer X, Y, Z, and the actions required to minimize its potential risk? 00:13:10.760 |
It's a very fair question, but if you think about it, I need to know what's the customer, then I need to know what products the customer bought, 00:13:20.760 |
I need to know what dates these products were bought, so I can get the circle of renews. 00:13:26.760 |
I need to understand what the current status is, and I need to map all the signals for the risk. 00:13:31.760 |
And if the customer has multiple products, he may be happy with the product A and not happy with the product B. 00:13:39.760 |
So, there is a lot of signals and intelligence that goes from that. 00:13:43.760 |
So, having a single agent for this was not ideal, because you don't get to the accuracy level that we are targeted to. 00:13:50.760 |
So, we needed to go before supervisor was a thing on the Harrison this morning. 00:13:55.760 |
We came with a supervisor approach, which is basically receives the NLP and decomposes the NLP. 00:14:01.760 |
And because the question is about a renews question, it hits the renews agent immediately. 00:14:08.760 |
Then the renews agent gets the context, and then he calls himself the adoption agent and the delivery agent to understand what's the status of the customer now. 00:14:18.760 |
So, I can answer half of the question on the pipeline. 00:14:22.760 |
And you can see this on the demo, on the Langsmith and all the traces going, and how we decompose the questions there. 00:14:31.760 |
But that's active in parallel behind the scenes. 00:14:34.760 |
Not 100% autonomous yet, because we still have human in the loop. 00:14:38.760 |
But sentiment analysis is something that I can trigger any time. 00:14:42.760 |
If there is a question, I can proactively go and trigger the sentiment analysis and all the signals and come back. 00:14:47.760 |
Hey, this customer hates us on that product, he loves us, or something like that. 00:14:52.760 |
And at the same time, I want to make sure what is the install base that this customer has. 00:14:58.760 |
Is a competitive guide into this, because we open a breach, we are too expensive, or we miss a functionality, whatever that is. 00:15:06.760 |
So, I'm talking about Cisco, but I appreciate the fact that a lot of you have a similar scenario on your products, 00:15:12.760 |
especially if you have recurrent revenue and a lot of things that goes on. 00:15:16.760 |
So, then when you get out of the first part of the question, and you get the real focus on customer experience. 00:15:23.760 |
Add customer experience on any company is all about workflows. 00:15:34.760 |
So, if you think about this, LLMs are not very good on workflows. 00:15:41.760 |
They are trained on information that goes there. 00:15:44.760 |
When you do a genetic with a tool like LangGraph platform, it helps a lot with that. 00:15:48.760 |
So, we went on this, and we go to workflows, and if the second part of the question is about risk, I hit a predicted machine learning model. 00:16:00.760 |
So, we combine it both to get to the accuracy level, and we leverage the length change to make context carrying back and forward, and LLMs to trace it back. 00:16:08.760 |
Going to the point that we receive the answer, we do the final reasoning formatting, and answer it back to the internal user. 00:16:15.760 |
Now, you can go deeper on this, and I don't have time for that, but I want to share you something. 00:16:22.760 |
This, our CX Agentic AI in action, I have seven agents on that example. 00:16:28.760 |
Over time, we may decompose them on some other agents. 00:16:32.760 |
The point of Agentic AI, a lot of people think about agents. 00:16:37.760 |
It's more about the flexibility for the workflow. 00:16:42.760 |
This is a question, how can I maximize the value for what I've invested in Cisco in the last two years? 00:16:47.760 |
That's a very fair question, but it's meant for an external customer, not an internal user. 00:16:55.760 |
How can I dynamically change this for the understanding? 00:16:59.760 |
That's how the power of the supervisor and dynamic agents goes from there. 00:17:02.760 |
So, you can see the agentic power coming in reality, and this is running in production. 00:17:07.760 |
So, if you have time, I recommend you to go in the booth. 00:17:11.760 |
You have Vince and Amand that presented yesterday, myself and others. 00:17:14.760 |
That's going to show how we're running this on environment in production. 00:17:19.760 |
The interaction between the supervisors and the agents. 00:17:22.760 |
We use multiple models, as I said, deployment on-prem and on the cloud. 00:17:25.760 |
And a predictive machine learning pipeline using predicted models on ML integrated with LLMs to accomplish the results that we want. 00:17:33.760 |
With that said, I want to wrap this conversation, share with you some key learnings and takeaways that we went through that process for us. 00:17:45.760 |
As I mentioned before, please define the use cases and the metrics first. 00:17:50.760 |
Don't jump on the bandwagon because there is a new tool on the weekend because next weekend is going to have a new one. 00:17:57.760 |
And your team is going to get excited and AI is moving at unprecedented speed, which is great. 00:18:06.760 |
But at the same time, if you define the use case, it's much better for you to measure it. 00:18:11.760 |
At the same time, rags, prompts, field shots, supervised fine-tuning chains come after you have the use case. 00:18:20.760 |
I'm stating the obvious, but you wouldn't believe how many times this is not used. 00:18:26.760 |
Last, on the right side, experimentation writing prototype is key. 00:18:31.760 |
Sometimes, if you do have a team that's only focused on production, they have different metrics than the experimentation team. 00:18:37.760 |
The experimentation team has a latitude and degree of freedom to try and fail and fail fast. 00:18:43.760 |
So use that and have a dedicated team for evaluation too. 00:18:47.760 |
I talk to my team and they know that you don't make the dog the custodian of the sausage. 00:18:54.760 |
So you want the evaluation team to have the golden data sets and be able to say, 00:18:59.760 |
"Hey, this stuff is not hitting the performance you need, or the cost you need, or the metrics you need." 00:19:04.760 |
Because if it's the same team, people blend among themselves. 00:19:07.760 |
So create this isolation, which helps you to achieve what you want. 00:19:11.760 |
Last but not least, achieving high accuracy with stacks to SQL 00:19:16.760 |
SQL and enterprise use case is really, really hard. 00:19:19.760 |
The three-letter acronym is called SQL, and another three-letter acronym is called LLM. 00:19:30.760 |
So we actually leverage this snowflake context, semantic context on Cortex, just for the reference of metadata. 00:19:40.760 |
And if you believe on something that I'm saying, avoid using LLM for doing joints on SQL. 00:19:49.760 |
Avoid hype and inter-Asian context and collaboration is critical. 00:20:04.760 |
And both Cisco and LLM chain has been championing an initiative that we put out there that's called Agency, 00:20:10.760 |
which is a full architecture that we open source. 00:20:14.760 |
That goes beyond the only sharing of LLM protocols, MCP being one of those. 00:20:23.760 |
But it's how you leverage a semantic layer and syntactical layer across agents with a directory. 00:20:29.760 |
When you go to the internet, the first thing that happens is you go to a DNS server, right? 00:20:34.760 |
There is no notion of DNS servers with agents yet, if you think about it. 00:20:38.760 |
So it brings all these notions of how you authenticate. 00:20:43.760 |
We authenticate and make sure that you have this. 00:20:48.760 |
LLM chain and Cisco with others on that slide. 00:20:51.760 |
We are proposing a full architecture and open source so the industry will go to a Gentic AI fest. 00:20:56.760 |
With that said, I would like to thank you all for all the time that you have here. 00:21:05.760 |
We are going on the weeds and have the demo running in production. 00:21:07.760 |
If you go through the traces, brace yourself, and let's go together.