back to indexSebastian Thrun: Flying Cars, Autonomous Vehicles, and Education | Lex Fridman Podcast #59
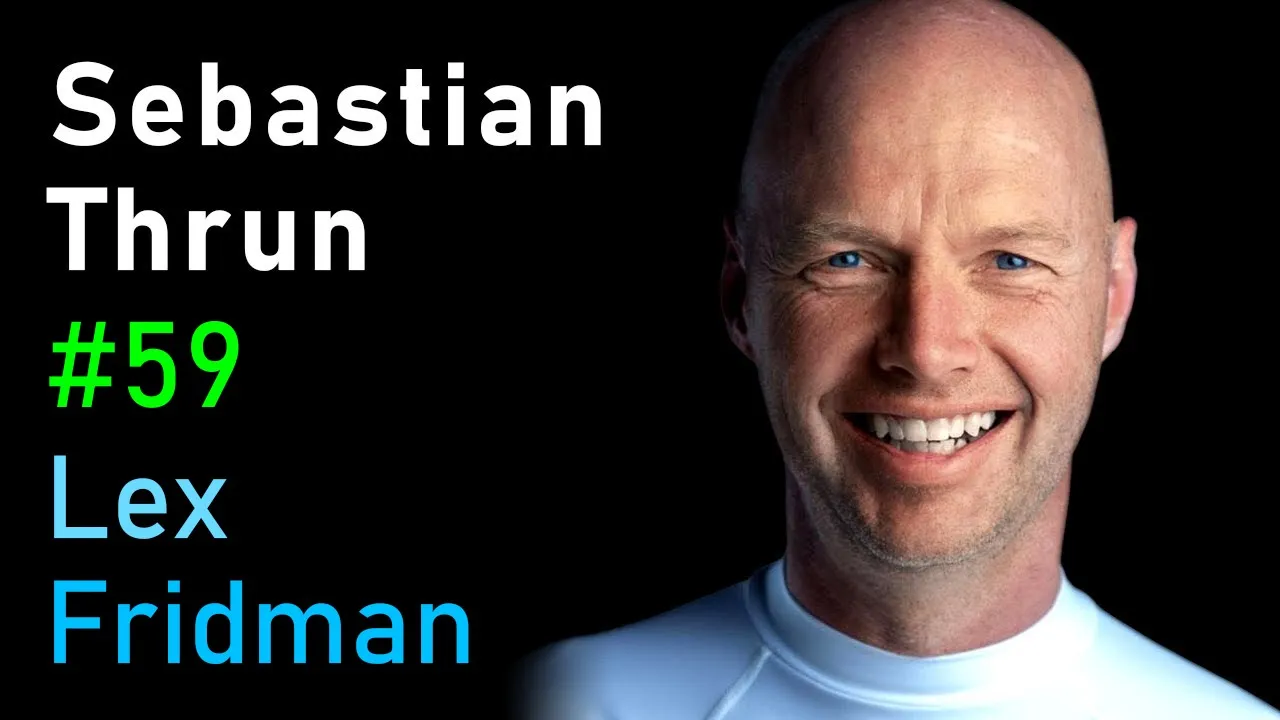
Chapters
0:0 Intro
3:24 Simulation
4:29 Computing
6:12 Machine Learning
7:39 Expert Systems
13:55 Software and Clocks
15:29 Advice for Future Work
17:23 Leadership
20:35 Intentions
22:36 Stephen Schwarzman
26:53 Lessons Learned
28:51 What is Academia
31:54 What makes you upset
33:10 Challenges of autonomous vehicles
36:12 Leadership style
38:42 Autonomous vehicle approaches
42:10 Selfdriving car nanodegree
43:46 Impact on society
45:17 Deep learning in autonomous vehicles
47:28 What is machine learning
51:8 Machine learning in the medical field
54:6 Nearterm impacts of AI
57:52 nanodegree
00:00:00.000 |
The following is a conversation with Sebastian Thrun. 00:00:05.280 |
computer scientists, and educators of our time. 00:00:08.120 |
He led the development of the autonomous vehicles 00:00:10.600 |
at Stanford that won the 2005 DARPA Grand Challenge 00:00:14.280 |
and placed second in the 2007 DARPA Urban Challenge. 00:00:18.160 |
He then led the Google Self-Driving Car Program 00:00:20.960 |
which launched the self-driving car revolution. 00:00:29.080 |
which was one of the first massive open online courses 00:00:43.320 |
Their self-driving car program, for example, is excellent. 00:01:02.720 |
but also, as many know, he's just a really nice guy. 00:01:06.840 |
It was an honor and a pleasure to talk with him. 00:01:27.840 |
or YouTube or Twitter, consider mentioning ideas, 00:01:40.160 |
This podcast is a side project for me, as many people know, 00:01:47.720 |
from an amazing community, from you, really help. 00:01:52.220 |
I recently started doing ads at the end of the introduction. 00:01:55.240 |
I'll do one or two minutes after introducing the episode 00:02:05.420 |
I provide timestamps for the start of the conversation 00:02:08.000 |
that you can skip to, but it helps if you listen to the ad 00:02:11.480 |
and support this podcast by trying out the product 00:02:21.460 |
I personally use Cash App to send money to friends, 00:02:31.080 |
You can buy fractions of a stock, say $1 worth, 00:02:36.600 |
Brokerage services are provided by Cash App Investing, 00:02:44.720 |
to support one of my favorite organizations called FIRST, 00:02:47.920 |
best known for their FIRST robotics and Lego competitions. 00:02:51.340 |
They educate and inspire hundreds of thousands of students 00:02:56.520 |
and have a perfect rating on Charity Navigator, 00:03:03.120 |
When you get Cash App from the App Store or Google Play 00:03:13.960 |
that I've personally seen inspire girls and boys 00:03:19.760 |
And now, here's my conversation with Sebastian Thrun. 00:03:29.020 |
So let's start with a crazy philosophical question. 00:03:34.860 |
And in general, do you find the thought experiment 00:03:43.780 |
but it's completely irrelevant to the way we should act. 00:03:57.340 |
these kinds of questions might be kind of interesting, 00:03:59.620 |
looking at the universe as a information processing system. 00:04:03.820 |
- Universe is an information processing system. 00:04:05.980 |
It's a huge physical, biological, chemical computer. 00:04:18.860 |
I think it's just the world evolves the way it evolves, 00:04:35.300 |
is just a Turing test to see if indeed you are. 00:04:39.360 |
You've also said that one of the first programs 00:04:56.460 |
So if you were to place yourself back into that time, 00:05:02.140 |
could you have predicted the evolution of computing, 00:05:05.620 |
AI, the internet, technology, in the decades that followed? 00:05:13.180 |
which I'd seen on television once and thought, 00:05:43.740 |
So we weren't at the scale yet where we are today. 00:05:54.340 |
I felt it was super cool to build an artificial human. 00:05:57.940 |
And the best way to build an artificial human 00:06:00.660 |
because that's kind of the closest we could do. 00:06:15.140 |
what do you think it takes to build an intelligent system 00:06:29.740 |
I'd say everybody pretty much knows how to walk. 00:06:33.100 |
And we learn how to walk in the first year or two 00:06:56.780 |
that you have to give them rules for every contingencies. 00:07:05.500 |
And because it's so hard to get this instruction set right, 00:07:13.140 |
which has been to make for like 30, 40 years, 00:07:15.860 |
is an idea that computers can find their own rules. 00:07:18.500 |
So they can learn from falling down and getting up 00:07:28.700 |
Today's computers can watch experts do their jobs, 00:07:39.380 |
- So the dream of in the '80s of expert systems, 00:07:49.340 |
So to sort of reduce, sort of be able to explain to machines 00:07:55.460 |
So do you think, what's the use of human expertise 00:08:01.740 |
will come from machines learning from experience 00:08:20.020 |
And I think the expert systems was our best attempt in AI 00:08:31.260 |
"and your heel backwards and here how you stop stumbling." 00:08:41.180 |
The majority of the human brain doesn't deal with language. 00:08:43.780 |
It deals with like subconscious numerical perceptual things 00:08:48.180 |
that we don't even, that we're self aware of. 00:08:50.420 |
Now, when a AI system watches an expert do their job 00:08:57.900 |
it can pick up things that people can't even put into 00:09:07.140 |
look over the shoulders of highly paid human doctors 00:09:16.980 |
- So you were a key person in launching three revolutions, 00:09:30.220 |
and I apologize for all the philosophical questions. 00:09:36.180 |
- How do you choose what problems to try and solve? 00:09:40.540 |
What drives you to make those solutions a reality? 00:09:44.820 |
I wanna literally make the lives of others better. 00:09:50.460 |
maybe jokingly, make the world a better place. 00:10:04.260 |
the chances for me to learn something interesting 00:10:22.220 |
what it actually means to build something like this. 00:10:27.500 |
after I finished my professorship at Stanford, 00:10:31.060 |
they really focused on like what has the maximum impact 00:10:34.820 |
Like transportation is something that has transformed 00:10:37.500 |
the 21st or 20th century more than any other invention, 00:10:45.020 |
women's rights are different because of transportation. 00:11:01.100 |
where we are extremely inefficient resource wise, 00:11:10.380 |
or where we spend endless hours in traffic jams. 00:11:13.820 |
And very, very simple innovations like a self-driving car 00:11:20.220 |
And it's there, I mean, the technology is basically there. 00:11:42.820 |
I think that inspired an entire generation of roboticists 00:11:49.460 |
about this particular kind of four wheeled robots 00:11:52.620 |
we called autonomous cars, self-driving cars. 00:11:58.180 |
the autonomous car that won the race to the desert, 00:12:20.580 |
- Oh my God, painful were all these incredibly complicated, 00:12:33.020 |
our car that eventually won the DARPA urban challenge 00:12:43.500 |
of two computer clocks, occasionally a clock went backwards 00:12:59.300 |
immediately focused on machine learning and on software, 00:13:07.620 |
with an existing rental car can perfectly drive the course. 00:13:14.780 |
And the human being to me was a conjunction of three steps. 00:13:23.740 |
and then we had actuators, our hands and our feet. 00:13:38.180 |
So we would build massive machine learning into our machine. 00:13:44.780 |
from human drivers, we had the entire speed control 00:13:47.340 |
of the vehicle was copied from human driving, 00:13:49.780 |
but also have the robot learn from experience 00:13:51.620 |
where it made a mistake and go to recover from it 00:13:55.580 |
- You mentioned the pain point of software and clocks. 00:14:22.100 |
It's the first time that people really even explore. 00:14:28.340 |
of the desert the year before nobody finished. 00:14:30.620 |
What does it take to scramble and finish a product 00:14:40.460 |
It was four because five couldn't comfortably sit 00:14:45.300 |
And I, as a team leader, my job was to get pizza 00:14:47.700 |
for everybody and wash the car and stuff like this 00:15:00.820 |
What we did really, really well was time management. 00:15:03.300 |
We were done with everything a month before the race. 00:15:06.220 |
And we froze the entire software a month before the race. 00:15:11.420 |
every other team complained if they had just one more week, 00:15:15.420 |
And we decided, we're not gonna fall into that mistake. 00:15:19.860 |
And we had an entire month to shake the system. 00:15:22.700 |
And we actually found two or three minor bugs 00:15:27.060 |
And we were completely prepared when the race occurred. 00:15:34.620 |
in terms of being able to be done on time or ahead of time. 00:15:38.980 |
What do you, how do you do that in your future work? 00:15:46.340 |
especially in highly innovative projects like this. 00:15:50.860 |
- Well, the nice thing about the Dark Background Challenge 00:15:52.540 |
is that the problem was incredibly well-defined. 00:15:56.540 |
to drive the old Dark Background Challenge course, 00:16:00.780 |
And then at some reason, we were kicked out of the region. 00:16:04.020 |
So we had to go to different deserts, the Snorren Desert, 00:16:20.380 |
Then I studied my own life and life of others 00:16:23.780 |
and realized that the typical mistake that people make 00:16:41.060 |
We had a testing team that built a testing booklet 00:16:46.740 |
just to make sure we shake out the system appropriately. 00:16:49.940 |
- And the testing team was with us all the time 00:16:55.540 |
tomorrow we do, we practice the start of the event. 00:17:03.180 |
"Oh my God, it doesn't do a railroad crossing, why not? 00:17:05.100 |
"Oh my God, it mistakes the rails for metal barriers. 00:17:24.780 |
To me as an engineer, it's just super exciting 00:17:33.380 |
It may be to linger on the point of leadership. 00:17:35.740 |
I think it's one of the first times you were really a leader 00:17:41.700 |
and you've led many very successful teams since then. 00:18:00.220 |
I'm an engineer at heart, so I care about engineering. 00:18:03.740 |
So I don't know what the chicken and the egg is, 00:18:11.540 |
And you could like in the middle of the night, 00:18:12.740 |
wake up at one in the morning and switch on your computer. 00:18:15.180 |
And what you told you to yesterday, it would still do. 00:18:19.380 |
Unfortunately, that didn't quite work with people. 00:18:22.820 |
and they don't do it and they hate you for it. 00:18:25.780 |
Or you do it today and then they go a day later 00:18:31.460 |
how can you put yourself in the brain of people 00:18:35.100 |
And in terms of computers, that's super dumb. 00:18:41.260 |
But people are smart and people are emotional 00:18:43.580 |
and people have pride and people have aspirations. 00:18:48.860 |
And that's the thing where most of our leadership just fails 00:18:56.220 |
believe they can treat their team just the same 00:19:10.980 |
Like your job is to make your students look great. 00:19:20.820 |
they actually love you and their parents love you. 00:19:24.700 |
Turns out all my students were smarter than me. 00:19:35.100 |
So the question really is, can you take a team of people 00:19:40.380 |
to connect to what they actually want in life 00:19:50.100 |
I've really rarely met a person with bad intentions. 00:19:55.900 |
I think every person I've met wants to help others. 00:20:01.860 |
not to just help ourselves, but to help others. 00:20:19.180 |
and just having enormous confidence and pride out of this. 00:20:22.900 |
And that's when my environment works the best. 00:20:27.220 |
These are moments where I can disappear for a month 00:20:37.260 |
It's not often heard that most people in the world 00:20:53.460 |
talking this, that we judge ourselves by our intentions 00:21:01.860 |
I mean, here in Silicon Valley, we're full of engineers 00:21:05.140 |
and are kind of befuddled by why it doesn't work for them. 00:21:09.180 |
The biggest skill I think that people should acquire 00:21:13.060 |
is to put themselves into the position of the other 00:21:16.860 |
and listen, and listen to what the other has to say. 00:21:19.980 |
And they'd be shocked how similar they are to themselves. 00:21:24.580 |
how their own actions don't reflect their intentions. 00:21:33.380 |
"And by the way, what you just did has the following effect. 00:21:38.820 |
And then people would say, "Oh my God, not I wasn't 00:21:53.380 |
And I've had many instances where people say, 00:21:56.460 |
"because it wasn't my intention to look like an idiot. 00:21:59.180 |
"It wasn't my intention to help other people. 00:22:07.420 |
"How to Make Friends and How to Influence Others." 00:22:14.020 |
And I wish I was good enough to apply it every day. 00:22:18.940 |
Like be positive, remember people's names, smile, 00:22:27.460 |
and you think is an idiot is actually just like yourself. 00:22:30.500 |
It's a person who's struggling, who means well, 00:22:36.620 |
I've recently spoken with Stephen Schwarzman. 00:22:47.720 |
But he said, sort of to expand on what you're saying, 00:22:56.020 |
is hear people when they tell you what their problem is 00:23:09.540 |
- And because it's right there in front of you 00:23:15.220 |
And in fact, yourself and everybody around you 00:23:18.020 |
by just hearing the problems and solving them. 00:23:20.860 |
- I mean, that's my little history of engineering. 00:23:23.980 |
That is, while I was engineering with computers, 00:23:28.260 |
I didn't care at all what the computer's problems were. 00:23:34.820 |
And it just doesn't work this way with people. 00:23:38.500 |
If you come to me and say, "Do A," I do the opposite. 00:23:43.620 |
- But let's return to the comfortable world of engineering. 00:23:47.140 |
And can you tell me in broad strokes in how you see it? 00:23:55.100 |
the technical evolution of autonomous vehicles 00:24:00.420 |
to the incredible success we see with the program 00:24:05.420 |
and Waymo and the entire industry that sprung up 00:24:08.340 |
of different kinds of approaches, debates, and so on. 00:24:11.220 |
- Well, the idea of self-driving car goes back to the '80s. 00:24:14.180 |
There was a team in Germany, another team in Carnegie Mellon 00:24:18.700 |
But back in the day, I'd say the computers were so deficient 00:24:21.780 |
that even the best professors and engineers in the world 00:24:27.300 |
It then folded into a phase where the US government 00:24:37.980 |
a successful stack of paper describing lots of stuff 00:24:43.900 |
was a successful product of a research project. 00:24:47.660 |
So we trained our researchers to produce lots of paper. 00:24:50.540 |
That all changed with the DARPA Grand Challenge. 00:24:54.340 |
And I really gotta credit the ingenious people at DARPA 00:25:05.580 |
And it sounds very trivial, but there was no tax code 00:25:08.820 |
that allowed the use of congressional tax money for a price. 00:25:15.100 |
So if you put in 100 hours in, you could charge 100 hours. 00:25:17.460 |
If you put in 1,000 hours in, you could build 1,000 hours. 00:25:20.660 |
By changing the focus and say, "We're making the price. 00:25:28.940 |
all these contractors who are used to the drug 00:25:33.380 |
And they drew in a whole bunch of new people. 00:25:37.620 |
They were people who had a car and a computer 00:25:42.420 |
The million bucks was the original price money, 00:25:45.460 |
And they felt, "If I put my computer in my car 00:25:52.060 |
Like half the teams, there was a team that was surfer dudes 00:25:55.500 |
and they had like two surfboards on their vehicle 00:26:02.820 |
And you could tell these guys were not your common 00:26:06.700 |
fails babe bandit who gets all these big multi-million 00:26:10.860 |
and billion dollar countries from the US government. 00:26:18.580 |
I was very fortunate at Stanford that I'd just received tenure 00:26:25.140 |
And I had enough money to finance this thing. 00:26:28.260 |
And I was able to attract a lot of money from third parties. 00:26:34.060 |
because they were super scared to be embarrassed 00:26:43.380 |
So it kind of reset the entire landscape of people. 00:26:54.260 |
Can you just comment quickly on your sense of lessons learned 00:27:06.100 |
Is there something to be learned and scaled up bigger? 00:27:19.620 |
I'm a really big believer in systems building. 00:27:21.900 |
I've always built systems in my academic career, 00:27:23.660 |
even though I do a lot of math and abstract stuff, 00:27:33.780 |
and say, let me solve a component of a problem. 00:27:35.740 |
Like with someone, there's fields like non-monetary logic 00:27:49.500 |
hey, what problem would my grandmother care about 00:27:58.460 |
Because only then do I have an impact on the world. 00:28:04.700 |
but impressing my grandmother is very, very hard. 00:28:07.580 |
So I would always thought if I can build a self-driving car 00:28:31.420 |
Like it takes sometimes tens of thousands of people 00:28:35.340 |
There's no way you can fund an army of 10,000 at Stanford. 00:28:42.500 |
And the Dark Background Challenge was beautiful 00:28:43.900 |
because it told me what this prototype had to do. 00:28:46.340 |
I didn't have to think about what it had to do. 00:28:52.820 |
what academia could aspire to is to build a prototype 00:29:03.540 |
- First of all, I wanna emphasize what academia really is. 00:29:13.340 |
First and foremost, a professor is an educator. 00:29:18.580 |
or whether you are a Harvard or Stanford professor. 00:29:21.980 |
That's not the way most people think of themselves 00:29:25.020 |
in academia because we have this kind of competition 00:29:42.900 |
the great research comes out of universities. 00:29:48.860 |
So there's nothing really fundamentally broken here. 00:29:53.420 |
And I think America has the finest university system 00:29:56.820 |
We can talk about reach and how to reach people 00:30:07.020 |
it'd be really great if there was more debate 00:30:10.860 |
about what the great big problems are in society. 00:30:30.460 |
by what your closest colleagues believe the problem is. 00:30:35.300 |
well, there's an entire new field of problems. 00:30:41.660 |
I was a roboticist and a machine learning expert. 00:30:48.540 |
It's a very methods driven kind of viewpoint of the world. 00:30:51.540 |
I built robots that acted in museums as tour guides, 00:30:55.660 |
It is something that at the time was moderately challenging. 00:31:16.420 |
"there's no complexity, it's just too simple." 00:31:25.020 |
Among all robotic problems that I've seen in my life, 00:31:27.260 |
I would say the self-driving car, transportation, 00:31:29.780 |
is the one that has the most hope for society. 00:31:35.140 |
And it was because we focused on methods and solutions 00:31:39.900 |
Like if you go around today and ask your grandmother, 00:31:59.300 |
The other thing that's really nice in Silicon Valley 00:32:00.900 |
is Silicon Valley is full of smart people outside academia. 00:32:04.380 |
So there's the Larry Pages and Mark Zuckerbergs 00:32:06.340 |
in the world who are anywhere as smart or smarter 00:32:11.460 |
And what they do is they are at a different level. 00:32:27.540 |
They hire the best PhDs from the best universities 00:32:31.180 |
So I think there's kind of vertical integration 00:32:42.780 |
It did not work as well in other places in this nation. 00:32:46.700 |
we had the world's finest computer science university, 00:33:00.620 |
That symbiosis seemed to exist pretty much only 00:33:03.900 |
in Silicon Valley and maybe a bit in Boston and Austin. 00:33:17.180 |
and the launch of the Google self-driving car, 00:33:22.060 |
the challenges of autonomous vehicles as they are now? 00:33:31.700 |
- I'm extremely proud of what has been accomplished. 00:33:35.300 |
And again, I'm taking a lot of credit for the work that I do. 00:33:45.900 |
It is actually quite an interesting hard problem. 00:33:51.660 |
to build one that manages 90% of the problems 00:34:12.900 |
the remaining 1% is actually still relatively easy. 00:34:15.780 |
But now you're down to like a hundredth of 1% 00:34:18.220 |
and it's still completely unacceptable in terms of safety. 00:34:21.580 |
So the variety of things you encounter are just enormous. 00:34:24.260 |
And that gives me enormous respect for human beings 00:34:26.500 |
that we're able to deal with the couch on the highway, 00:34:31.900 |
or the blown tire that we've never been trained for. 00:35:00.980 |
and basically flawlessly are able to drive people around 00:35:24.740 |
but the glitches have become very, very rare. 00:35:26.580 |
I think the next step is gonna be to down cost it 00:35:33.780 |
are not quite an automotive grade standard yet. 00:35:37.820 |
to really kind of go somewhere and make the business case. 00:35:42.580 |
It's not just, oh my God, we have this capability 00:36:08.140 |
wait for the news that Waymo has just done this. 00:36:10.700 |
- So, you know, it's interesting you mentioned gutsy. 00:36:34.660 |
But if we compare Tesla and Waymo, for example, 00:36:53.900 |
And on Waymo side, there's more sort of cautious, 00:37:10.660 |
- Look, I don't sit in either of those teams. 00:37:17.980 |
In the end of the day, every innovator in that space 00:37:23.140 |
And I would say you could put aerospace titans 00:37:27.140 |
into the same bucket, which is you have to balance 00:37:34.300 |
And this country in particular, in the States, 00:37:40.620 |
Air travel is what a hundred times as safe per mile 00:37:48.500 |
because people have found ways to be very methodological 00:38:12.740 |
There's always the possibility that someone makes a mistake 00:38:17.140 |
We can never guarantee to a hundred percent absolute safety 00:38:23.340 |
But I think I'm very proud of the history of the United States 00:38:27.100 |
I mean, we've dealt with much more dangerous technology 00:38:32.740 |
We have nuclear weapons and we keep those safe. 00:38:39.460 |
that really balance these two things very, very successfully. 00:38:42.980 |
- You've mentioned a lot of great autonomous vehicle 00:38:44.900 |
companies that are taking sort of the level four, level five 00:38:48.780 |
they jump in full autonomy with a safety driver 00:38:55.780 |
There's also the approach that Tesla Autopilot is doing 00:38:59.580 |
which is kind of incrementally taking a level two vehicle 00:39:05.860 |
from the driving of human beings and trying to creep up 00:39:12.380 |
until it's able to achieve level four autonomy. 00:39:15.580 |
So perfect autonomy in certain kind of geographical regions. 00:39:19.820 |
What are your thoughts on these contrasting approaches? 00:39:23.180 |
- Well, so first of all, I'm a very proud Tesla owner 00:39:37.660 |
because then it turns me into a much safer driver 00:39:42.260 |
and that I'm a hundred percent confident that's the case. 00:39:49.900 |
since I ran the Waymo team is this thing called deep learning. 00:39:54.500 |
Deep learning was not a hot topic when I started Waymo 00:39:59.420 |
It was there, in fact, we started Google Brain 00:40:02.820 |
So I invested in deep learning, but people didn't talk 00:40:19.660 |
towards a more human-like, oh, let's just learn about it. 00:40:24.180 |
This looks like the thing I've seen 10,000 times before. 00:40:26.540 |
So maybe it's the same thing, machine learning perspective. 00:40:34.740 |
At Udacity, we teach a course in self-driving cars. 00:40:38.740 |
In fact, I think we've graduated over 20,000 or so people 00:40:49.260 |
And in this course, the very first homework assignment 00:40:56.940 |
what this means is you put a camera into your car 00:40:59.900 |
and you wouldn't know where the lane is, right? 00:41:01.820 |
So you can stay inside the lane with your car. 00:41:06.540 |
You just look and you know where the lane is, 00:41:10.180 |
For machines, for a long time, it was super hard 00:41:12.180 |
because people would write these kind of crazy rules. 00:41:29.260 |
between lane markings or not, or 12 feet, whatever it is. 00:41:53.820 |
that's never done any programming before in this space 00:42:15.220 |
the fact that you launched that many years ago now, 00:42:22.060 |
That's a great example of system level thinking. 00:42:24.740 |
So just taking an entire course that teaches you 00:42:32.380 |
and it's become actually incredibly high quality. 00:42:38.060 |
And we find that engineers from Tesla and Waymo 00:42:45.500 |
one is existing universities will be very slow to move 00:42:50.500 |
and there's no department for self-driving cars. 00:42:52.380 |
So between McGee and EE and computer science, 00:42:59.660 |
And every professor listening here will know, 00:43:02.940 |
And secondly, even if all the great universities 00:43:11.060 |
it is just a few thousand students that can partake 00:43:13.660 |
because all the great universities are super selective. 00:43:18.060 |
How about people in China or in the Middle East 00:43:25.100 |
from the skill of building self-driving cars? 00:43:30.140 |
And the answer is, we should just give everybody the skill 00:43:37.380 |
then we have like a thousand self-driving car startups. 00:43:52.500 |
the, you know, over a period of several decades, 00:44:05.340 |
So it's a show, it's interesting to think about 00:44:18.620 |
That's, you know, there's other more specific disciplines 00:44:21.820 |
like deep learning and so on that Udacity is also teaching, 00:44:28.420 |
It came at a time when there were a bunch of acqui-hires. 00:44:34.180 |
not for its technology or its products or business, 00:44:38.300 |
So acqui-hire means maybe the company of 70 people, 00:44:40.620 |
they have no product yet, but they're super smart people 00:44:44.340 |
So I took acqui-hires like GM Cruise and Uber and others 00:44:48.420 |
and did the math and said, hey, how many people are there 00:44:56.980 |
of a self-driving car engineer in these acquisitions 00:45:02.220 |
So think about this, you get yourself a skill 00:45:10.820 |
I mean, what other thing could you do in life 00:45:17.620 |
But to come back for a moment onto deep learning 00:45:23.760 |
what are your thoughts on Elon Musk's statement, 00:45:28.500 |
provocative statement perhaps, that lighter is a crutch? 00:45:31.100 |
So this geometric way of thinking about the world 00:45:34.060 |
may be holding us back if what we should instead be doing 00:45:41.020 |
of autonomous vehicles is using camera as a primary sensor 00:45:46.020 |
and using computer vision and machine learning 00:45:50.620 |
I think first of all, we all know that people can drive cars 00:45:55.420 |
without lighters in their hands because we only have eyes 00:46:02.140 |
Maybe we use some other perception about our bodies, 00:46:19.480 |
and then gave us the camera image with no latency, 00:46:23.460 |
we would be able to drive a car that way, the same way. 00:46:28.780 |
Secondly, I really love the idea that in the Western world, 00:46:47.980 |
And I promise you the spread out solution will be better 00:46:58.340 |
and then they're gonna discuss again for another week. 00:47:00.540 |
Whereas if all these ants go in a random direction, 00:47:03.540 |
and they're gonna come back and claim victory 00:47:05.620 |
and get the Nobel prize or whatever the ant equivalent is. 00:47:08.700 |
And then they all march in the same direction. 00:47:13.220 |
We're not plan-based, we're not central-based. 00:47:15.540 |
We don't have a Soviet Union style central government 00:47:23.140 |
We get investor money, go out and try it out. 00:47:36.940 |
as fundamentally being able to solve most of the problems? 00:47:54.700 |
of repetitive patterns and find those patterns. 00:47:59.700 |
So an example, we did a study at Stanford two years ago 00:48:15.100 |
that all had been biopsied for what the actual situation was. 00:48:22.700 |
also included rashes and other skin conditions, lesions. 00:48:26.460 |
And then we had a network find those patterns 00:48:30.740 |
and it was by and large able to then detect skin cancer 00:48:36.700 |
as the best board-certified Stanford-level dermatologist. 00:48:45.940 |
finding skin cancer, but it couldn't drive a car. 00:48:53.260 |
and we can often learn from a very small data set 00:48:59.580 |
very large data sets and things that would be very repetitive. 00:49:04.660 |
because almost everything we do is repetitive. 00:49:06.420 |
So that's gonna really transform human labor, 00:49:09.980 |
but it's not this almighty general intelligence. 00:49:17.300 |
To that end, I actually commiserate the naming a little bit 00:49:21.300 |
because artificial intelligence, if you believe Hollywood, 00:49:24.420 |
is immediately mixed into the idea of human suppression 00:49:30.340 |
I don't think that we're gonna see this in my lifetime. 00:49:32.940 |
I don't think human suppression is a good idea. 00:49:44.300 |
that's able to extract patterns from large data sets. 00:49:48.420 |
And in doing so, it can be super impactful, super impactful. 00:49:53.420 |
Let's take the impact of artificial intelligence 00:49:57.620 |
We all know that it takes something like 10,000 hours 00:50:06.300 |
it takes a certain amount of time to become experts. 00:50:12.220 |
to observe people become experts and observe experts 00:50:18.460 |
in some interesting way that could go from law to sales 00:50:35.460 |
It's been done commercially in many, many instantiations. 00:50:40.780 |
to make people expert on the very first day of their work. 00:50:46.540 |
If your doctor is still in their first 10,000 hours, 00:50:51.020 |
you have a doctor who's not quite an expert yet. 00:50:53.780 |
Who would not want a doctor who is the world's best expert? 00:50:59.180 |
to really eradicate error in decision-making, 00:51:02.700 |
error in lack of expertise for human doctors. 00:51:10.300 |
in which way do you hope machines in the medical field 00:51:16.340 |
You mentioned this sort of accelerating the learning curve 00:51:24.500 |
or in the first 10,000 hours can be assisted by machines. 00:51:32.340 |
that can detect and classify and diagnose skin cancer. 00:51:36.300 |
And we proved two years ago that it does pretty much 00:51:40.540 |
as good or better than the best human doctors. 00:51:43.580 |
So there's a friend of mine, let's call him Ben. 00:51:47.660 |
He goes to his doctor and the doctor looks at a mole 00:51:50.700 |
and says, "Hey, that mole is probably harmless." 00:52:02.620 |
And the app says, "No, no, no, no, this is a melanoma." 00:52:08.660 |
and skin cancer is the most common cancer in this country. 00:52:12.380 |
Melanomas can go from stage zero to stage four 00:52:18.100 |
Stage zero means you can basically cut it out yourself 00:52:27.980 |
So it's a very serious, serious, serious condition. 00:52:36.140 |
looked at the iPhone and was a little bit puzzled. 00:52:47.660 |
And it came back as cancerous, as a melanoma. 00:52:56.220 |
And I said, I told him I do this book on skin cancer. 00:53:00.660 |
My doctor just had an iPhone that found my cancer." 00:53:21.020 |
Most cancers can actually be cured relatively easily 00:53:25.820 |
And the reason why we don't tend to catch them early 00:53:30.540 |
Like your very first symptom of a gallbladder cancer 00:53:41.580 |
and you're being imaged, it's usually stage four plus. 00:53:45.860 |
And that's the time when the occurring chances 00:53:48.180 |
might be dropped to a single digit percentage. 00:53:50.820 |
So if we could leverage AI to inspect your body 00:53:54.500 |
on a regular basis without even a doctor in the room, 00:53:58.060 |
maybe when you take a shower or what have you, 00:54:06.260 |
- You've mentioned there's a concern that people have 00:54:09.420 |
about near-term impacts of AI in terms of job loss. 00:54:12.780 |
So you've mentioned being able to assist doctors, 00:54:17.860 |
Do you have a worry of people losing their jobs 00:54:21.060 |
or the economy being affected by the improvements in AI? 00:54:32.300 |
and we have our kind of implicit job promise. 00:54:38.700 |
we spend way over 50% of our graduates in new jobs 00:54:51.180 |
guaranteeing that you will help give scholarships 00:54:54.940 |
that educate people in this kind of situation. 00:55:00.020 |
on the idea of basically rebuilding the American dream. 00:55:03.900 |
So Udacity has just dedicated 100,000 scholarships 00:55:07.460 |
for citizens of America for various levels of courses 00:55:22.100 |
And they range from inter-level digital marketing 00:55:24.980 |
to very advanced self-driving car engineering. 00:55:30.900 |
It's an issue that if you wanna really make America great, 00:55:43.020 |
to be proactive about our education and our skillset. 00:55:58.220 |
and we can squeeze in the evenings and things to online. 00:56:14.060 |
in the train compartment next to me was one of my students. 00:56:24.020 |
I believe officially six countries or five countries. 00:56:26.500 |
Mostly in the Middle East, like Saudi Arabia and in Egypt. 00:56:37.300 |
that went through programming skills proficient 00:56:40.340 |
at the level of a computer science undergrad. 00:56:46.260 |
It's kind of tough, but we kind of trying to figure out 00:56:52.540 |
The vision is education ought to be a basic human right. 00:56:57.540 |
It cannot be locked up behind ivory tower walls 00:57:03.620 |
for the parents who might be bribe themselves 00:57:08.380 |
and only for people from the right demographics 00:57:10.460 |
and the right geography and possibly even the right race. 00:57:20.620 |
we're gonna open up education to everybody in the world. 00:57:27.180 |
I think is the biggest pledge of scholarships ever 00:57:30.740 |
And we're working, as I said, with the White House 00:57:37.460 |
to really bring education to everywhere in the world. 00:57:40.100 |
- Not to ask you to pick the favorite of your children, 00:57:55.780 |
what set of courses are you most excited about at Udacity? 00:58:05.460 |
which is when we talk to our partner companies, 00:58:09.100 |
we have now a very strong footing in the enterprise world. 00:58:14.580 |
we've kind of always focused on these hard skills 00:58:39.260 |
And that's something that we haven't done very well 00:58:45.180 |
because we are so obsessed with individual test scores 00:58:47.900 |
and pay so little attention to teamwork in education. 00:58:52.620 |
So that's something I see us moving into as a company 00:58:56.940 |
And I think, look, we can teach people tech skills 00:59:14.380 |
It's weird, we literally went in school in K through 12, 00:59:18.180 |
we teach kids like get the highest math score. 00:59:26.740 |
and the best English scores, make me the best leader. 00:59:32.860 |
because making, first of all, in terms of math scores, 00:59:40.620 |
You can hire someone with great empathy for you, 00:59:42.740 |
that's much harder, but it can always hire someone 00:59:59.700 |
in the workplace, I think we're gonna be super successful. 01:00:17.980 |
the Wright brothers flew in Kitty Hawk for the first time. 01:00:22.660 |
And you've launched a company of the same name, Kitty Hawk, 01:00:26.980 |
with the dream of building flying cars, EVtols. 01:00:31.980 |
So at the big picture, what are the big challenges 01:00:35.780 |
of making this thing that actually have inspired 01:00:38.700 |
generations of people about what the future looks like? 01:00:53.820 |
I think my, honestly, my single most remembered 01:00:58.340 |
where I was sitting on a pillow and I could fly. 01:01:02.060 |
I remember like maybe three dreams of my childhood, 01:01:20.220 |
for this super impactful stuff like flying cars. 01:01:29.620 |
They take off vertically and they fly horizontally, 01:01:34.380 |
One difference is that they are much quieter. 01:01:37.740 |
We just released a vehicle called Project Heaviside 01:01:41.620 |
that can fly over you as low as a helicopter. 01:01:50.260 |
but anywhere outdoors, your ambient noise is higher. 01:01:57.060 |
They're much more affordable than helicopters. 01:02:03.060 |
There's lots of single point of figures in a helicopter. 01:02:16.380 |
There is no second solution in helicopter flight. 01:02:23.740 |
you can now have many, many, many small motors 01:02:27.260 |
And that means if you lose one of those motors, 01:02:29.620 |
Heaviside, if it loses a motor, has eight of those, 01:02:45.500 |
flight is actually easier than ground transportation. 01:02:50.700 |
the world is full of like children and bicycles 01:03:00.500 |
When you go above the buildings and tree lines, 01:03:06.100 |
look outside and count the number of things you see flying. 01:03:09.420 |
I'd be shocked if you could see more than two things. 01:03:12.860 |
In the Bay Area, the most I've ever seen was six. 01:03:20.420 |
So the sky is very ample and very empty and very free. 01:03:23.980 |
So the vision is, can we build a socially acceptable 01:03:27.820 |
mass transit solution for daily transportation 01:03:57.340 |
which means we would take your 300 hours of daily, 01:04:05.180 |
Who wouldn't want, I mean, who doesn't hate traffic? 01:04:07.660 |
Like I hate, give me the person who doesn't hate traffic. 01:04:17.540 |
we have technology, we can free the world from traffic. 01:04:25.420 |
- Do you think there is a future where tens of thousands, 01:04:29.300 |
maybe hundreds of thousands of both delivery drones 01:04:34.300 |
and flying cars of this kind, EV towers fill the sky? 01:04:40.900 |
And there's obviously the societal acceptance 01:04:48.020 |
we're gonna exceed ground transportation safety 01:04:50.300 |
as has happened for aviation already, commercial aviation. 01:04:58.260 |
That's why we are focusing relentlessly on noise 01:05:00.900 |
and we built perhaps the quietest electric VTOL vehicle 01:05:06.420 |
The nice thing about the sky is it's three dimensional. 01:05:09.700 |
So any mathematician will immediately recognize 01:05:12.460 |
the difference between 1D of like a regular highway 01:05:24.980 |
because you believe building 100 vertical lanes 01:05:30.140 |
to stack 100 vertical lanes physically onto 101. 01:05:34.300 |
That would be consuming the world's GDP for an entire year 01:05:49.180 |
with another vehicle would just go to different altitudes 01:05:55.300 |
that's exactly how commercial aviation works. 01:06:01.380 |
another plane flies from San Francisco to New York, 01:06:10.300 |
and it will be a solved problem for the urban space. 01:06:12.660 |
There's companies like Google, Bing and Amazon 01:06:18.500 |
They use exactly the same principles as we use today 01:06:25.860 |
- Do you envision autonomy being a key part of it 01:06:28.940 |
so that the flying vehicles are either semi-autonomous 01:06:37.820 |
You don't want idiots like me flying in the sky. 01:06:49.420 |
- And a centralized, that's a really interesting idea 01:06:58.780 |
similar as we have in the current commercial aviation 01:07:05.540 |
That's a really interesting optimization problem. 01:07:07.660 |
- It is mathematically very, very straightforward. 01:07:11.060 |
Like the gap we leave between jets is gargantuous. 01:07:13.500 |
And part of the reason is there isn't that many jets. 01:07:18.820 |
Today, when you get vectored by air traffic control, 01:07:23.900 |
So an ATC controller might have up to maybe 20 planes 01:07:28.140 |
And then they talk to you, you have to talk back. 01:07:30.340 |
And that feels right because there isn't more than 20 planes 01:07:37.980 |
So we have to do something that's called digital, 01:07:43.060 |
Like we have what, four or five billion smartphones 01:07:47.740 |
And somehow we solve the scale problem for smartphones. 01:07:51.940 |
They can talk to somebody and they're very reliable. 01:08:01.060 |
So instead of me as a pilot talking to a human being 01:08:06.260 |
receiving a new frequency, like how ancient is that? 01:08:11.260 |
and digitally transmit the right flight coordinates. 01:08:22.180 |
Do you think we'll one day build an AI system 01:08:25.780 |
that a human being can love and that loves that human back? 01:08:37.020 |
And the ethics of using the shovel are always 01:08:44.060 |
In terms of emotions, I would hate to come into my kitchen 01:08:49.060 |
and see that my refrigerator spoiled all my food, 01:08:54.220 |
then have it explained to me that it fell in love 01:08:56.500 |
with the dishwasher and I wasn't as nice as the dishwasher. 01:09:07.060 |
I would probably not recommend this refrigerator 01:09:12.900 |
I think to me, technology has to be reliable. 01:09:24.620 |
I want it to compliment me, not to replace me. 01:09:40.780 |
I can run across the Atlantic near the speed of sound 01:09:49.580 |
My voice now carries me all the way to Australia 01:09:56.580 |
And it's not the speed of sound, which would take hours. 01:10:06.300 |
I would even argue my flushing toilet makes me superhuman. 01:10:10.500 |
Just think of the time before flushing toilets. 01:10:13.780 |
And maybe you have a very old person in your family 01:10:18.460 |
or take a trip to rural India to experience it. 01:10:25.780 |
So to me, what technology does, it compliments me. 01:10:30.900 |
Therefore, words like love and compassion have very little, 01:10:34.900 |
have very little interest in this for machines. 01:10:40.700 |
- You don't think, first of all, beautifully put, 01:10:45.660 |
but do you think love has use in our tools, compassion? 01:10:55.380 |
love is a means to convey safety, to convey trust. 01:11:00.380 |
I think trust has a huge need in technology as well, 01:11:11.220 |
the same way we, or in a similar way we trust people. 01:11:23.900 |
that are able to convey sense of trust, sense of safety, 01:11:38.820 |
And because all of us, we have this beautiful system, 01:11:42.140 |
I wouldn't just blindly copy this to the machines. 01:11:53.260 |
of the 19th century, almost all transportation 01:12:01.660 |
And you could have concluded that is the right way 01:12:06.780 |
We've made the exception of birds, who use flapping wings. 01:12:10.820 |
that flapped wings to their arms and jumped from cliffs. 01:12:15.100 |
Then the interesting thing is that the technology solutions 01:12:21.580 |
Like, in technology, it's really easy to build a wheel. 01:12:23.900 |
In biology, it's super hard to build a wheel. 01:12:25.700 |
There's very few perpetually rotating things in biology, 01:12:52.420 |
So the solutions have used very different physical laws 01:13:00.100 |
oh, this is how nature does it, let's just replicate it, 01:13:05.380 |
to the agricultural revolution was a humanoid robot, 01:13:12.540 |
You said that you don't take yourself too seriously. 01:13:25.740 |
But you have a humor and a lightness about life 01:13:29.100 |
that I think is beautiful and inspiring to a lot of people. 01:13:35.020 |
The smile, the humor, the lightness amidst all the chaos 01:13:40.020 |
of the hard work that you're in, where does that come from? 01:13:53.620 |
People say 52 is a new 51, so now I feel better. 01:14:09.340 |
But for the first 300,000 years minus the last 100, 01:14:17.060 |
plus or minus 30 years, roughly, give or take. 01:14:22.620 |
That makes me just enjoy every single day of my life 01:14:28.260 |
Why am I born today when so many of my ancestors 01:14:32.460 |
died of horrible deaths, like famines, massive wars 01:14:37.460 |
that ravaged Europe for the last 1,000 years, 01:14:44.540 |
when the Americans and the Allies did something amazing 01:14:52.620 |
And then when you're alive and feel this every day, 01:14:56.940 |
then it's just so amazing what we can accomplish, 01:15:10.980 |
from your smartphone to your flushing toilet, 01:15:16.180 |
your new clothes you're wearing, your watch, your plane, 01:15:19.580 |
penicillin, I don't know, anesthesia for surgery, 01:15:25.780 |
penicillin, have been invented in the last 150 years. 01:15:30.020 |
So in the last 150 years, something magical happened. 01:15:35.900 |
to disseminate information more efficiently than before, 01:15:38.780 |
that all of a sudden we're able to invent agriculture 01:15:42.860 |
and nitrogen fertilization that made agriculture 01:15:45.940 |
so much more potent that we didn't have to work 01:15:48.020 |
in the farms anymore and we could start reading and writing 01:15:50.140 |
and we could become all these wonderful things 01:15:52.300 |
we are today, from airline pilot to massage therapist 01:16:03.340 |
Steven Pinker, who is a very famous author and philosopher 01:16:07.340 |
whom I really adore, wrote a great book called 01:16:09.420 |
"Enlightenment Now" and that's maybe the one book 01:16:14.500 |
a single article written in the 20th century, 01:16:42.620 |
And in doing so, together with an older invention 01:16:45.540 |
of irrigation, was able to increase the yield 01:17:05.700 |
if this man hadn't done what he had done, okay? 01:17:08.420 |
Think about that impact and what that means to society. 01:17:17.020 |
And I'm so glad I lived after Carl Bosch and not before. 01:17:21.340 |
- I don't think there's a better way to end this, Sebastian. 01:17:28.340 |
- Thanks for coming on, it's a real pleasure. 01:17:31.020 |
- Thank you for listening to this conversation 01:17:34.420 |
And thank you to our presenting sponsor, Cash App. 01:17:47.500 |
to learn and to dream of engineering our future. 01:17:50.580 |
If you enjoy this podcast, subscribe on YouTube, 01:17:53.380 |
get five stars on Apple Podcast, support on Patreon, 01:17:58.860 |
And now let me leave you with some words of wisdom 01:18:09.820 |
if you say, wow, I failed, I tried, I was wrong, 01:18:18.300 |
And when your fear goes away, you can move the world. 01:18:21.660 |
Thank you for listening and hope to see you next time.