back to indexThe State of AI in production — with David Hsu of Retool
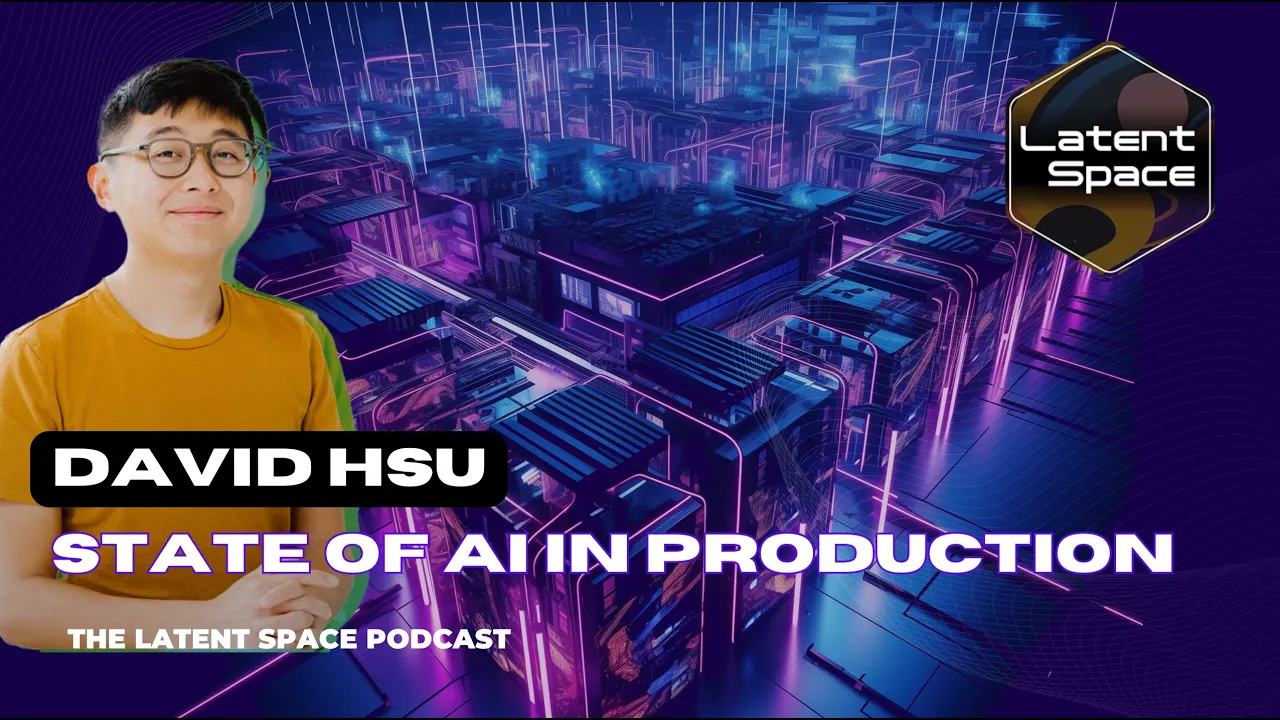
Chapters
0:0 Introduction
2:43 Retool's founding story and decision not to present at YC demo day initially
5:40 Experience in YC with Sam Altman
9:27 Importance of hiring former founders early on
10:43 Philosophy on fundraising - raising less money at lower valuations
14:57 Overview of what Retool is
18:9 Origin story of Retool AI product
23:4 Decision to use open source vector database PG Vector
24:42 Most underrated Retool feature loved by customers
28:7 Retool's AI UX and workflows
34:5 Zapier vs Retool
37:41 Updates from Retool's 2023 State of AI survey
40:34 Who is adopting AI first?
43:43 Evolving engineering hiring practices in the age of Copilot/ChatGPT
45:52 Retool's views on internal vs external AI adoption
48:29 OSS models vs OpenAI in production
50:54 Additional survey questions to ask in 2024
52:7 Growing interest in multimodal AI capabilities
54:0 Balancing enterprise sales vs bottom-up adoption
60:0 Philosophical thoughts on AGI and intentionality
00:00:00.000 |
Hey everyone, welcome to the Latent Space Podcast. 00:00:02.720 |
This is Alessio, partner and CTO at Residence at Decibel Partners, 00:00:06.200 |
and I'm joined by my co-host Swix, founder of Small.ai. 00:00:09.480 |
Hi, and today we are in the studio with David Hsu from Reto. 00:00:19.800 |
and then have you talk about something personal. 00:00:23.720 |
You got your degree in philosophy and CS from Oxford. 00:00:27.880 |
I wasn't aware that they did like double degrees. 00:00:32.960 |
It's actually a single degree, actually, which is really cool. 00:00:35.360 |
So basically, yeah, so you study content, you study philosophy, 00:00:43.640 |
and sort of computers think, or computers be smart. 00:00:46.360 |
Like, you know, what does it mean for a computer to be smart? 00:00:48.720 |
As well as logic, which is also another intersection, 00:00:53.160 |
In Stanford, you know, it might be symbolic systems or whatever. 00:00:58.840 |
And it's always hard to classify these things 00:01:13.640 |
and, you know, it's just been a straight line up from there, right? 00:01:20.200 |
And that's your sort of brief bio that I think you want most people to know. 00:01:25.080 |
What's something on your LinkedIn that people should know about you, 00:01:29.360 |
let's just say something you're very passionate about 00:01:34.440 |
I probably read like two books a week, around about, so it's a lot of fun. 00:01:43.040 |
No, I don't use Retool to read, so that'd be funny. 00:01:50.440 |
Any recommendations that you just fall in love with? 00:01:55.800 |
I'm mostly reading fiction nowadays, so fiction's a lot of fun. 00:01:59.320 |
I think it maybe helps me be more empathetic, if you will. 00:02:02.960 |
to sort of see what it's like to be in someone else's shoes, 00:02:07.800 |
Besides that, I'm really good about philosophy as well. 00:02:09.960 |
I find philosophy just so interesting, especially logic. 00:02:13.560 |
We can talk more about that for probably hours if you want, so. 00:02:17.400 |
Yeah, I have a sort of casual interest in epistemology, 00:02:21.720 |
and I think that any time you try to think about machine learning 00:02:28.640 |
you have to start wrestling with these very fundamental questions 00:02:48.400 |
Let's just maybe jump through a couple things on Retool 00:02:51.600 |
that I found out while researching your background. 00:02:54.920 |
So you did YC, but you didn't present at Demo Day initially 00:02:58.560 |
because you were too embarrassed of what you had built. 00:03:07.360 |
I've seen a lot of people kind of give up early on 00:03:09.920 |
because they were like, "Oh, this isn't really 00:03:12.600 |
what I thought it was going to be to be a founder." 00:03:14.680 |
They told me I would go to YC and then present 00:03:19.880 |
So how did that influence also how you build Retool today 00:03:32.680 |
So we were supposed to present at the March Demo Day, 00:03:34.880 |
but then we basically felt like we had nothing really going on. 00:03:40.440 |
And so we were like, "Okay, well, why don't we take six months 00:03:46.040 |
Part of that, to be honest, was I think there's a lot of noise 00:03:53.120 |
especially because there's so many startups nowadays. 00:03:55.680 |
And I guess for me, I'd always want to sort of under-promise 00:04:03.360 |
And on Demo Day, I mean, maybe you two have seen 00:04:05.400 |
a lot of the videos, it's a lot of honestly over-promising 00:04:08.800 |
and under-delivering because every startup says, 00:04:11.360 |
"Well, I'm going to be the next Google or something." 00:04:18.520 |
And so we chose actually not to present at Demo Day, 00:04:21.280 |
mostly because we felt like we didn't have anything 00:04:25.400 |
Although actually a few other founders in our batch 00:04:27.080 |
probably would have chosen to present in that situation, 00:04:30.120 |
but we were just kind of embarrassed about it. 00:04:32.240 |
And so we basically took six months to just say, 00:04:36.800 |
And we're not presenting until we have a product 00:04:39.600 |
that we're proud of and customers that we're proud of. 00:04:44.320 |
So I don't know if there's much to learn from this situation 00:04:49.320 |
besides I think social validation was something 00:04:54.000 |
that I personally had never really been that interested in. 00:04:57.080 |
And so it was definitely hard because it's hard to sort of, 00:05:04.080 |
and all your friends are graduating when you failed 00:05:10.960 |
that all your friends are up there and on the podium 00:05:13.440 |
presenting and they are raising a ton of money 00:05:18.040 |
But in our case, we felt like it was a choice 00:05:21.680 |
and we could have presented if we really wanted to, 00:05:23.760 |
but we would not have been proud of the outcome 00:05:27.200 |
And for us, it was more important to be true to ourselves, 00:05:29.240 |
if you will, and show something that we're actually proud of 00:05:33.200 |
and then shut the company down after two years. 00:05:36.120 |
- Yeah, any sad moment stories from the YC days? 00:05:40.160 |
Could you tell in 2017 that Sam was going to become, 00:05:43.680 |
like run the biggest AI company in the world? 00:05:56.120 |
I forgot, maybe president of YC in our batch. 00:06:15.360 |
But besides that, I mean, I think we were so overwhelmed 00:06:18.880 |
by the fact that we had to go build a startup 00:06:20.280 |
and we were not honestly paying that much attention 00:06:22.680 |
to every YC partner and taking notes on them. 00:06:51.400 |
and maybe don't know what to do with the capital, so. 00:06:57.120 |
from sort of why we chose not to present a demo day. 00:06:58.960 |
And the reason was it feels like a lot of people 00:07:04.880 |
which is like, you're almost like playing house 00:07:07.280 |
Like, it's like, oh, well, I hear that in a startup, 00:07:09.440 |
we're supposed to raise money and then hire people. 00:07:16.960 |
And so you could do a lot of PR and stuff like that. 00:07:25.520 |
everything else is gonna, nothing's gonna work basically. 00:07:27.640 |
You can't continue to raise money or hire people 00:07:30.760 |
if you don't have customers, you're not gonna work value. 00:07:33.200 |
And so for us, we were always very focused on that. 00:07:37.560 |
I think it's, again, maybe it goes to like the sort of, 00:07:39.640 |
you know, presenting something truthful about yourself 00:07:42.560 |
or staying true to yourself, something to that effect, 00:07:44.440 |
which is, we didn't want to pretend like we had a, 00:07:46.320 |
you know, thriving business, we could actually. 00:07:56.240 |
grind it away for probably a year, year and a half or so. 00:08:04.120 |
we had raised something like maybe a million dollars, 00:08:07.560 |
maybe a million and a half, something out of YC. 00:08:13.320 |
I was like, wow, like how are we ever gonna spend 00:08:20.880 |
'Cause we're paying ourselves 30, 40K a year. 00:08:27.520 |
The question was like, we better find traction 00:08:30.400 |
we're gonna, you know, just give up psychologically. 00:08:36.720 |
You're probably psychologically gonna give up. 00:08:38.920 |
I think that's actually true in most startups. 00:08:40.520 |
Actually, it's like most startups die in the early stages, 00:08:45.080 |
but really because you run out of motivation. 00:08:50.440 |
I think it would have actually been harder for us 00:08:52.680 |
because we would have ran out of motivation faster. 00:08:54.920 |
Because when you're pretty part of market fit, actually, 00:08:57.920 |
10 people, for example, to Marshall's part of market fit, 00:09:02.040 |
Like it's, you know, everyday people are asking you, 00:09:08.440 |
And that's actually a very tiring environment to be in. 00:09:11.800 |
the founders figuring out product market fit, 00:09:14.240 |
I think that's actually a much sort of safer path, 00:09:19.600 |
Like when you hire employees, you have an idea. 00:09:21.640 |
You have front of market, you have customers. 00:09:25.320 |
of a place for employees to join as well, so. 00:09:29.880 |
typically the sort of founder employee relationship is, 00:09:36.000 |
And you don't really get critical pushback from the employee, 00:09:40.040 |
and even if they like you as an early engineer. 00:09:51.560 |
in trying to figure out what that product is. 00:10:02.760 |
especially, you know, which was a lot larger now, 00:10:06.320 |
So I want to say like, when we were, I don't know, 00:10:15.560 |
because I think you infuse sort of a, you know, 00:10:19.760 |
a outcome-oriented culture of like a very little politics, 00:10:23.080 |
'cause, you know, no one came from larger companies. 00:10:24.880 |
Everyone was just like, "This is my own startup. 00:10:27.280 |
"how to achieve the perception for the customer." 00:10:28.800 |
And so I think from a cultural perspective even today, 00:10:31.120 |
a lot of those cultures are sort of very self-startery. 00:10:33.720 |
I think it's actually because of sort of these like, 00:10:42.360 |
on just a little bit of the fundraising stuff, 00:10:44.440 |
something notable that you did was when, in 2021, 00:10:50.280 |
hundreds and hundreds of millions of dollars, 00:10:52.600 |
you, you know, you intentionally raised less money 00:11:02.400 |
in building Retool that you're just very efficient 00:11:13.200 |
You know, would you recommend that to everyone else? 00:11:15.680 |
What are your feelings sort of two years on from that? 00:11:23.040 |
where we raised less money at a lower valuation. 00:11:29.320 |
even, you know, internally and both externally, 00:11:32.200 |
I think people were really surprised actually, 00:11:33.880 |
because I think Silicon Valley has been conditioned to think, 00:11:37.400 |
oh, raising a giant sum of money at a giant valuation 00:11:41.720 |
you should maximize both the numbers basically. 00:11:46.400 |
actually for the people that matter the most, 00:11:52.680 |
more, raising more money means more dilutions. 00:11:55.480 |
If you look at, you know, a company like Uber, for example, 00:12:02.440 |
or, you know, let's say you joined before their huge round, 00:12:05.040 |
which I think happened at a few billion dollars in valuation, 00:12:07.520 |
they actually got diluted a ton when Uber fundraised. 00:12:12.560 |
if Uber dilutes themselves by 10%, for example, 00:12:14.360 |
let's say they raise 500 to 5 billion, for example, 00:12:24.080 |
And so if you look at actually a lot of founders 00:12:26.880 |
in sort of, you know, the operations statistics space, 00:12:29.640 |
or, you know, those that fundraise like, you know, 00:12:33.920 |
only have a few percentage points, actually, for a company. 00:12:36.320 |
And if the founders only have a few percentage points, 00:12:38.120 |
you can imagine how, you know, how little employees have. 00:12:40.080 |
And so that I think is actually just a really, you know, 00:12:47.840 |
given the same company quality is always worse. 00:12:54.520 |
you could command a certain valuation in the market. 00:12:56.360 |
You know, let's say it's, you know, X, for example. 00:12:59.120 |
Maybe you get lucky and you can raise two times X, 00:13:03.640 |
your company itself is not fundamentally changed. 00:13:08.600 |
You know, maybe today you're an AI company, for example. 00:13:19.280 |
And so now I think you see a lot of companies 00:13:20.760 |
that are raising really high valuations about 2021. 00:13:23.800 |
And now they're like, man, we're at like 100 X, 00:13:26.120 |
or, you know, we raised 300 X multiple, for example. 00:13:31.440 |
And like, man, we just can't raise money ever again. 00:13:33.520 |
Like, you know, we're gonna have to grow like 50 X 00:13:42.760 |
And so I think a lower valuation actually is much better. 00:13:48.680 |
two years later, we did not predict, you know, 00:13:52.400 |
But given it, I think we've done extremely well, 00:13:55.480 |
mostly because our valuation is not sky high. 00:14:02.440 |
We'd probably have recruiting problems, for example. 00:14:04.400 |
We'd probably have a lot of internal morale problems, 00:14:09.800 |
because we might have to go raise money again, 00:14:30.640 |
- In hindsight, 2020, but it looks like, you know, 00:14:46.160 |
or the inventors of the low-code internal tooling category. 00:14:52.320 |
Would, like, you know, how do you usually explain Retool? 00:15:01.160 |
We actually never, in fact, we have docs saying 00:15:10.000 |
And developers, they hear the phrase low-code, 00:15:13.920 |
Like, why would I ever want to write less code? 00:15:16.120 |
And so for us, Retool's actually built for developers. 00:15:18.160 |
Like, 95% of our customers actually are developers, 00:15:21.280 |
actually, and so that is a little bit surprising to people. 00:15:26.680 |
and this is, you know, kind of a funny joke, too. 00:15:28.760 |
I think part of the reason why Retool's been successful 00:15:31.040 |
is that developers hate building internal tools. 00:15:36.960 |
you've probably built internal tools yourself. 00:15:40.360 |
You know, it's like piecing together a CRUD UI. 00:15:49.720 |
It's like display error messages, like domestic buttons. 00:15:51.680 |
Like, all these things are not really exciting, 00:15:53.760 |
but you have to do it because it's so important 00:15:55.520 |
for your business to have high-quality internal software. 00:15:58.720 |
And so what Retool does is basically allows you 00:16:00.360 |
to sort of piece together an internal app really fast, 00:16:03.480 |
whether it's a front-end, whether it's a back-end 00:16:23.240 |
And I was like, what is Retool doing according to developers? 00:16:31.960 |
like, actually the burden and weight of internal tooling 00:16:36.760 |
or it's an Excel sheet somewhere or whatever. 00:16:39.000 |
But yeah, you guys have basically created this market. 00:16:44.000 |
In my mind, I don't know if there was someone 00:16:51.120 |
Every month, YC, there's a new YC startup launching 00:17:09.440 |
I've actually used Retool in my previous startups 00:17:21.960 |
like our sales operations people could interact with, 00:17:38.720 |
But piecing together a Retool is quite a bit easier. 00:17:41.120 |
So yeah, let me know if you have any feedback, 00:17:55.400 |
of sort of AI products ideation within Retool. 00:18:13.560 |
So we actually had a joke internally at Retool. 00:18:28.760 |
And so, but it was funny 'cause we were like, 00:18:32.680 |
Let's add it because it's like a buzzwordy thing 00:18:37.240 |
And so it was almost like a funny thing, basically. 00:18:51.080 |
And the evolution of our thinking was basically, 00:19:04.720 |
And there were two sort of main prompts, if you will, 00:19:12.960 |
And so you've probably seen that with Copilot, 00:19:14.920 |
you've seen sort of so many other coding assistants, 00:19:21.840 |
engineers, as we talked about, do some grunt work. 00:19:26.800 |
maybe could be automated by AI was sort of the idea. 00:19:41.760 |
It's not like coding is 10x faster than before. 00:19:46.440 |
maybe 20% faster or something like that, basically. 00:19:48.680 |
And so it's not like a huge step change, actually. 00:19:53.560 |
is because the sort of fundamental frameworks 00:19:58.000 |
And so if you're building, let's say, you know, 00:19:59.080 |
like the sales ops tool we were talking about before, 00:20:00.800 |
for example, let's say you've got AI to generate, 00:20:05.760 |
The problem is that it probably generated it for you 00:20:09.200 |
writing for the web browser, for example, right? 00:20:11.520 |
And then for you to actually go proofread that JavaScript, 00:20:13.720 |
for you to go read the JavaScript to make sure it's working, 00:20:15.600 |
you know, to fix the subtle bugs that AI might have caused, 00:20:19.800 |
actually takes a long time and a lot of work. 00:20:25.840 |
It is more sort of the language or the framework 00:20:30.040 |
It's kind of like, you know, like punched cards. 00:20:35.120 |
and AI could help you generate punched cards. 00:20:37.240 |
You're like, okay, you know, I guess that helps me, 00:20:40.160 |
you know, punching cards is a little bit faster now 00:20:42.400 |
'cause I have a machine punching them for me. 00:20:44.640 |
I still have to go read all the punched cards 00:20:48.120 |
And so for us, that was the sort of initial idea was, 00:20:53.720 |
That would, you know, I think it's somewhat helpful, 00:20:59.640 |
you can generate UIs by AI and stuff like that. 00:21:03.080 |
But it's not, I think, the step change of programming 00:21:10.520 |
But the bulk of investment actually is a number two, 00:21:18.160 |
And the reason why we think this is so exciting 00:21:25.000 |
is going to be AI-infused over the next, like, three years. 00:21:34.840 |
probably, you know, if you were to build it today, 00:21:48.240 |
So they basically have sales people enter their forecast, 00:21:51.480 |
you can have a recorder, like, hey, I have these deals, 00:21:55.920 |
You know, I think I'm upside in these, downside in these, 00:21:59.080 |
And what they're doing now is they're actually, 00:22:06.920 |
that actually use AI to compute, like, okay, well, you know, 00:22:11.000 |
like these are the deals that are more likely 00:22:17.120 |
pre-write you a draft of, you know, your report, basically. 00:22:19.800 |
And so that's an example where I think all apps, 00:22:22.680 |
whether it's, you know, a sales app, you know, 00:22:24.400 |
until let's say a fraud app, a, you know, FinTech app, 00:22:39.800 |
So that's why we launched, like, a vector database, 00:22:43.480 |
That's why we, you know, launches all this AI actually, 00:22:48.440 |
just, you know, give it to you out of the box. 00:22:52.560 |
really exciting future is can we make every app, 00:23:01.680 |
who's the co-founder and chief architect of Amplitude. 00:23:05.280 |
He mentioned that you just used PostgreSQL Vector. 00:23:13.640 |
putting vectors into one of the existing data stores 00:23:16.840 |
I think, like, you already have quite large customer scale, 00:23:20.880 |
so, like, you're maybe not trying to get too cute with it. 00:23:26.920 |
- Yeah, I think a general philosophical thing 00:23:36.320 |
especially when it comes to all the supporting infrastructure 00:23:46.560 |
In the end, like, there are really smart people in the world 00:23:52.000 |
and they're going to go build projects, basically, 00:23:59.440 |
with maybe more open source kind of providers 00:24:03.800 |
or projects, you could say, like PG Vector, for example. 00:24:13.520 |
and so we can go look and fix bugs ourselves, 00:24:17.720 |
But we really think open source is going to win in this space. 00:24:20.840 |
It's, you know, it's hard to say about models. 00:24:24.040 |
because, you know, it starts to get pretty complicated there. 00:24:29.240 |
and there's an explosion of creativity, if you will. 00:24:31.760 |
And I think betting on any one commercial company 00:24:35.080 |
but betting on the open source sort of community 00:24:39.640 |
So that's why we decided to go with consumer games. 00:24:47.600 |
that you didn't expect them to really care about? 00:24:59.840 |
and this is my sense of the AI space overall, 00:25:11.520 |
I think there's actually not that many AI use cases, 00:25:15.600 |
And AI, to me, even as of, what, like, January 19th or 2024, 00:25:20.600 |
still feels like in search of truly good use cases. 00:25:30.120 |
And what's really interesting, though, about retool, 00:25:32.960 |
and I think we're in a really fortunate position, 00:25:34.960 |
is that we have this large base of sort of customers, 00:25:38.520 |
are actually much more legacy, if you will, customers. 00:25:41.800 |
And a lot of them actually have a lot of use cases for AI. 00:25:44.960 |
And so, to us, I think we're almost in, like, 00:25:49.840 |
We're able to adopt some of these technologies 00:25:51.320 |
and then provide them to some of these, like, older players. 00:25:59.520 |
so we have this one, let's say, clothing manufacturer. 00:26:03.320 |
I think it's either the first or second largest 00:26:05.560 |
clothing manufacturer in the world who's using retool, 00:26:07.880 |
and, you know, a ginormous company with, you know, 00:26:13.040 |
stores on, you know, pretty every mall in the world. 00:26:15.320 |
And they were interested in, so they have one problem, 00:26:19.200 |
which is they need to design styles every year 00:26:23.280 |
for the next year, basically, for every season. 00:26:25.000 |
So, like, hey, it's just, like, summer 2024, for example, 00:26:31.800 |
They'd be like, okay, well, it looks like, you know, 00:26:33.480 |
maybe floral patterns are really hot in, like, you know, 00:26:38.720 |
and, like, do I think it's going to be hot in 2024? 00:26:40.360 |
Well, let me think about it, I don't know, you know, 00:26:45.120 |
let me go design some floral patterns, actually. 00:26:47.520 |
And what they ended up doing in retool, actually, 00:26:50.080 |
is they actually automated a lot of this process away, 00:26:52.240 |
retool, so they actually now have built a retool app 00:26:56.040 |
so, like, an analyst, if you will, to analyze, like, you know, 00:26:58.440 |
who are the hottest-selling patterns, you know, 00:26:59.880 |
particular geos, like, this was really hot in Brazil, 00:27:02.920 |
this was really hot, you know, somewhere else, basically. 00:27:16.120 |
and they print the patterns, which is really cool. 00:27:20.400 |
in use case I would have never thought about, 00:27:23.040 |
how clothing manufacturers create their next line of clothing, 00:27:25.800 |
you know, for the next season, like, I don't know, 00:27:31.960 |
And the fact that they're able to leverage AI, 00:27:33.640 |
they actually, you know, leverage multiple things in retail 00:27:35.720 |
to make that happen, it's really, really, really cool. 00:27:45.960 |
there are actually a lot of use cases for AI, 00:27:52.400 |
Like, you have to get into the businesses themselves, 00:27:56.800 |
but if, you know, you're working in the AI space 00:27:59.080 |
and want to find some use cases, please come talk to us. 00:28:02.200 |
about marrying sort of technology with use cases, 00:28:05.240 |
which I think is actually really hard to do right now, 00:28:12.040 |
around, like, how this industry is developing, 00:28:14.880 |
and, like, I think the foundation model layer is understood, 00:28:18.720 |
the sort of lag chain, VectorDB, RAG layer is understood, 00:28:23.720 |
and, like, what is, I always have a big question mark, 00:28:27.400 |
and I actually have you and Vercel V0 in that box, 00:28:35.120 |
and, like, you know, you are perfectly placed 00:28:38.200 |
to expose those functionalities to end users, 00:28:48.040 |
One segment of this, and I do see some startups 00:29:02.720 |
which is the sort of canvas-y boxes and arrows, 00:29:05.480 |
point and click, do this, then do that, type of thing, 00:29:11.360 |
I hate that, you know, what are we calling low-code? 00:29:15.240 |
Let's, every internal tooling company (laughs) 00:29:20.280 |
I worked at a sort of workflow orchestration company before, 00:29:27.520 |
but you are obviously very well-positioned to that. 00:29:33.160 |
would you, do you think that there is an overlap 00:29:37.960 |
I think that, you know, there's a lot of interest 00:29:46.920 |
that is already enabled within Retool Workflows, 00:29:49.600 |
but you could sort of hook them together sort of jankily. 00:29:57.480 |
You know, is it all of a kind, ultimately, in your mind? 00:30:08.200 |
which is, we can talk about that in a second, 00:30:17.240 |
like, I would probably argue 60, 70% of the utility, 00:30:24.160 |
is mostly via ChatGPT, and across the world, too. 00:30:27.880 |
And the reason for that is, I think the ChatGPT 00:30:32.800 |
or user experience is just really quite good. 00:30:34.960 |
You know, you can sort of converse with an AI, basically. 00:30:44.280 |
like a J.P. Morgan Chase, you know, for example, 00:31:00.880 |
and help me write this first version of a doc 00:31:02.840 |
or something like that, Chat is great for that. 00:31:10.920 |
you think about some of the economic productivity 00:31:22.720 |
oh, I have a relationship manager at J.P. Morgan Chase, 00:31:28.680 |
'Cause like, the employees actually do a lot of things 00:31:30.600 |
besides, you know, just, you know, generating, you know, 00:31:38.440 |
you'll go reach out to Chat, and Chat might solve it. 00:31:40.440 |
But like, Chat is not gonna solve 100% of your problems. 00:31:42.280 |
It'll solve, like, you know, 25% of your problems, 00:31:47.320 |
big breakthrough in AI is, is actually, like, automation. 00:31:55.880 |
people don't spend 40 hours a week in a chatbot. 00:31:59.920 |
And so what we think can be really big, actually, 00:32:02.360 |
is you're able to automate entire processes via AI. 00:32:05.480 |
Because then, you're really realizing the potential of AI. 00:32:09.360 |
a human copy-pasting data in to an AI chatbot, 00:32:11.720 |
and then, you know, pasting it back out, or copying back out. 00:32:15.720 |
is actually done in an automated fashion without the human. 00:32:18.720 |
And that, I think, is what's gonna really unlock 00:32:22.200 |
or, and that's what I'm really excited about. 00:32:25.520 |
And I think part of the problem right now is, 00:32:28.520 |
you know, I'm sure you all thought a lot about agents. 00:32:37.760 |
if you, let's say, you know, raise it to the power of seven, 00:32:39.720 |
for example, that's actually wrong, you know, 00:32:42.480 |
And so what we've actually done with workflows is, 00:32:48.160 |
is that we don't want to generate the whole workflow 00:32:52.840 |
is we want you to actually sort of drag and drop 00:32:55.520 |
And maybe you can get a vSphere or something by AI, 00:32:59.160 |
You should actually be able to modify the steps yourself. 00:33:04.560 |
it's not the whole workflow is created by AI. 00:33:13.120 |
So basically, what they say is like, hey, every day, 00:33:19.720 |
And then we, you know, do some data analysis. 00:33:23.160 |
raw SQL, basically, so it's nothing too surprising. 00:33:25.320 |
And then they use AI to go generate the new ideas. 00:33:28.080 |
And then the analysts will look at the new ideas 00:33:31.360 |
And that is like a, you know, that's true automation. 00:33:34.920 |
a designer copy pasting things as a chat sheet. 00:33:37.320 |
We can be like, hey, you know, give me a design. 00:33:39.600 |
It's actually, the designs are being generated. 00:33:42.720 |
And then you have to go and approve or reject those designs, 00:33:48.680 |
than just copy pasting stuff in a chat sheet. 00:33:57.440 |
in sort of delivering a lot of business value by AI. 00:34:01.800 |
via chat or, you know, via agents quite yet, so. 00:34:05.480 |
- Yeah, yeah, I think that's a pretty reasonable take. 00:34:41.560 |
it does remind me that you're running up against Zapier. 00:34:56.800 |
that they sort of need to shape is going to win. 00:35:08.320 |
Because it seems, I feel like you build a lot in-house. 00:35:10.720 |
- Yes, there's probably two philosophical things. 00:35:14.240 |
and I think that's actually one big differentiator 00:35:16.840 |
You know, we're so very rare to see them actually. 00:35:33.880 |
I think one huge advantage of some of the developers 00:35:38.400 |
like developers don't want to be given an institution. 00:35:42.840 |
so they can do themselves to build the institution. 00:35:48.640 |
interesting point that Equilibrium and Retool can get to 00:35:53.680 |
and we basically build apps for everybody, for example. 00:35:57.440 |
is that we've actually never gotten to that Equilibrium 00:35:59.560 |
and the reason for that is for some of the developers. 00:36:10.440 |
Give me easy way to query the rest of the APIs 00:36:21.160 |
basically never built anything specific for one customer. 00:36:26.080 |
The second thing is when it comes to sort of, 00:36:29.560 |
we're going to build and we're not going to build, 00:36:31.240 |
we basically think about whether it's a core competency 00:36:45.000 |
a developer-first Workflows automation engine, 00:36:54.280 |
that are, I think, quite far behind, actually. 00:36:56.280 |
They sort of are missing a lot of more critical features. 00:37:07.040 |
And so Virtual Workflows actually is fairly differentiated. 00:37:10.280 |
And so we're like, okay, we should go build that. 00:37:11.960 |
This is the one that we built, so let's go build it. 00:37:14.360 |
Whereas if you look at like Vectors, for example, 00:37:21.160 |
Does it make sense for us to go build our own? 00:37:36.880 |
and the products that we don't have a different take, 00:37:41.080 |
- Let's jump into the state of AI survey that you ran 00:37:46.280 |
So you surveyed about 1,600 people last August. 00:37:49.480 |
So, you know, and I were this busy like five years ago. 00:37:53.000 |
And there were kind of like a lot of interesting nuggets 00:38:06.320 |
Are you seeing sentiment shift in your customers 00:38:17.480 |
maybe say, "Hey, this is maybe not as worth changing 00:38:23.320 |
- Yes, so actually I'll be running the survey again, 00:38:27.520 |
It seems to me that it has settled down a bit 00:38:33.640 |
maybe like, I don't know, signal to noise, you could say. 00:38:35.960 |
Like, it seems like there's a little bit less noise 00:38:38.880 |
than before, but the signals actually run about the same 00:38:42.480 |
I think people are still trying to look for use cases. 00:38:48.000 |
and I think there are slightly more use cases, 00:38:56.440 |
do feel like the companies are investing quite a bit in AI 00:38:59.800 |
and they're not sure where it's going to go yet, 00:39:04.680 |
I do think that based on what we're hearing from customers, 00:39:08.240 |
if we're not seeing returns in like a year or something, 00:39:11.800 |
So I think there is like a, it is time bound, if you will. 00:39:19.920 |
I think that's been a Twitter meme for a while, 00:39:24.760 |
In the survey, 58 people said they used it less, 00:39:36.840 |
I know Stack Overflow tried to pull a whole thing. 00:39:40.640 |
because we changed the way we instrument our website, 00:39:47.520 |
expectation of job impact by function and operations, 00:39:56.240 |
Designers were the lowest one, 6.8 out of 10, 00:40:00.800 |
were designers of a job being impacted by AI. 00:40:04.600 |
Do you think there's a bit of a dissonance, maybe, 00:40:14.400 |
It's funny that the operations people are like, 00:40:18.880 |
versus the designers that maybe they love their craft more. 00:40:34.440 |
I think it's probably going to be engineering driven. 00:40:44.680 |
I think the companies that adopt AI the best, 00:40:48.080 |
it is going to be engineering driven, I think, 00:40:50.440 |
rather than like operations driven or anything else. 00:40:54.240 |
I think the rise of this like profile of AI engineering, 00:41:10.600 |
where like it does everything you want it to do. 00:41:14.120 |
require like very specific prompting, for example, 00:41:16.480 |
in order to get like, you know, really good results. 00:41:19.000 |
And the reason for that is, it's a tool that, you know, 00:41:22.920 |
it's not going to produce good results for you, actually. 00:41:31.480 |
going to have to be engineering first, basically, 00:41:49.320 |
is going to really be like experimenting with, necessarily. 00:41:55.920 |
well, what are the engineers going to focus on first? 00:42:01.920 |
And that, I think, is more of a business decision. 00:42:03.880 |
I think it's probably going to be more like, you know, 00:42:09.320 |
So, like, why don't we try using AI for that? 00:42:15.360 |
we are really, we have a lot of support issues. 00:42:19.240 |
we have a really, really high performance support team, 00:42:26.560 |
And so we'll have a lot of questions for us, basically. 00:42:30.600 |
can we, for example, draft some replies to support tickets, 00:42:34.880 |
Can we allow our support agents to be, you know, 00:42:47.120 |
okay, well, this is where AI could be most applied. 00:42:49.240 |
And then we assign the project to an engineer, 00:42:59.880 |
I don't know if that's gonna change the outcome, 00:43:10.600 |
45% of companies said they made their interviews 00:43:24.680 |
Like, have you, yeah, have you thought about it? 00:43:50.040 |
is that we are most, when we do engineering interviews, 00:44:04.960 |
at the end, the job of the employee is to be productive, 00:44:07.160 |
which they should use whatever tools they want 00:44:08.360 |
to be productive, so that's kind of our thinking, too. 00:44:12.120 |
if you think about it from a first-person's way, 00:44:14.040 |
if your only method of coding is literally copy-pasting 00:44:17.000 |
off of TractionBT, or just pressing Tab and Copilot, 00:44:27.880 |
Now, that said, I think if you're able to use 00:44:30.920 |
TractionBT or Copilot, let's say, competently, 00:44:33.200 |
we do view that as a plus, we don't view it as a minus, 00:44:51.920 |
And we really want to test for thinking, basically. 00:44:57.120 |
and we would encourage engineers to build this Copilot, too. 00:45:03.560 |
rather than just copy-pasting on Copilot, so. 00:45:22.520 |
from, like, using it internally to externally? 00:45:24.760 |
Obviously, there's, like, all these different things, 00:45:28.760 |
if an internal tool hallucinates, that's fine, 00:45:30.960 |
because you're paying people to use it, basically, 00:45:36.200 |
Yeah, I don't know if you have thought about it. 00:45:39.480 |
Because for you, if people build internal tool with Retool, 00:45:53.320 |
is actually that most software built in the world 00:46:06.640 |
that sell software as, you know, as sort of a business. 00:46:10.880 |
And that's why all the software engineers that we hire 00:46:16.360 |
which makes sense, because we're software companies. 00:46:18.440 |
But if you look at most companies in the world, 00:46:23.000 |
the clothing manufacturers I was talking about, 00:46:25.600 |
Like, they don't sell software, you know, to make money. 00:46:31.320 |
And so, most of the engineers in the world, in fact, 00:46:36.320 |
They work in these sort of more traditional companies. 00:46:37.920 |
So, if you look at the Forge of 100, for example, 00:46:39.920 |
probably, like, 20 of them are software companies. 00:46:42.400 |
You know, there are 480 of them are not software companies. 00:46:44.600 |
And actually, they employ those software engineers. 00:46:46.440 |
And so, most of the software engineers in the world, 00:46:50.120 |
actually goes towards these internal-facing applications. 00:46:53.120 |
And so, like, for all the reasons you said there, 00:46:55.600 |
like, I think hallucination matters less, for example, 00:46:57.560 |
'cause they have someone checking the output, 00:47:04.040 |
Yeah, it can be unreliable because it's probabilistic, 00:47:18.000 |
Like, Chats with BT is very obviously a consumer. 00:47:29.000 |
Maybe for, like, even for support, it's hard, 00:47:34.280 |
it's actually quite bad for support if you're hallucinating. 00:47:40.840 |
- Yeah, but that's a good idea, like, insight, you know? 00:47:47.160 |
Yeah, I think a lot of people, like you said, 00:47:49.040 |
we all build software, so we expect that everybody else 00:47:53.600 |
but most people just want to use the software 00:47:57.240 |
I think the last big bucket is, like, models breakdown. 00:48:13.560 |
Have you guys thought about using open source models? 00:48:19.200 |
Or have you just found GV84 to just be great at most tasks? 00:48:34.120 |
with using a hosted model, like a GPT-4, for example, 00:48:45.080 |
like, you know, let's use Azure, for example, 00:48:50.920 |
So I do think there is more acceptance, if you will, 00:48:59.560 |
like, you know, feeding in, like, earnings results data, 00:49:01.520 |
you know, three days before you announce earnings, 00:49:07.320 |
So, you know, there's still some challenges like that, 00:49:11.640 |
could actually help solve, like a lot of three, 00:49:13.720 |
you know, when it comes to, and that can be exciting. 00:49:43.760 |
like a Google or like a Facebook, for example, 00:49:47.560 |
Like, I think if OpenAI was competing with startups, 00:49:55.760 |
I think, in my opinion, at least, a startup model. 00:50:00.880 |
Like, Facebook is investing a lot in AI, in fact. 00:50:03.240 |
And so competing against a large FANG company 00:50:15.680 |
and I would say model performance is so important right now 00:50:21.160 |
you know, you can argue Lama 2 is actually so far behind, 00:50:24.000 |
but, like, customers don't want to use Lama 2 00:50:26.600 |
And so that, I think, is part of the challenge. 00:50:31.000 |
so if we get, like, Lama 4, Lama 5, for example, 00:50:38.600 |
Like, it's safer for me to, you know, host it on-prem. 00:50:46.400 |
where open AI is executing really well, I think. 00:50:50.440 |
but let's see what happens in the next year or two, so. 00:51:08.720 |
Like, what info do you really actually want to know 00:51:19.360 |
the value of the survey is mostly seeing changes over time 00:51:30.400 |
One thing that was actually pretty shocking to us 00:51:33.480 |
but if you look at the one change that we saw, 00:51:44.920 |
The GPT-4 MPS thing was like 45 or something like that, 00:51:53.880 |
is our models getting worse, models getting better? 00:52:00.000 |
That, I think, is the most interesting thing, so. 00:52:02.480 |
Do you two have any questions that you think we should ask? 00:52:19.720 |
and I don't really know how that is going to manifest. 00:52:30.480 |
There's a smaller subset of open-source models 00:52:39.720 |
And yeah, so I think I would like to understand 00:53:03.120 |
And what's the forced, stack-ranked preference order? 00:53:13.800 |
- It's something that we're trying to actively understand, 00:53:20.560 |
but really, multimodalities is like an umbrella term 00:53:22.840 |
for actually a whole bunch of different things 00:53:33.960 |
and ultimately everything can be merged together 00:54:03.880 |
you put in a question from Joseph here in the show notes, 00:54:15.680 |
So I figure we'll just kind of zoom out a little bit 00:54:20.000 |
You have a lot of fans in the founder community, 00:54:35.640 |
is that you have been notably sort of sales-led in the past. 00:54:44.800 |
but I'm not that close to sort of your sales portion. 00:54:48.400 |
And it's interesting to understand your market, 00:54:53.040 |
versus all the competition that's out there, right? 00:55:05.280 |
but effectively what he's seeing and what he's asking 00:55:07.640 |
is how do you manage between sort of enterprise 00:55:18.560 |
because I had always assumed that you were a self-serve, 00:55:25.320 |
But it seems like you have a counter-consensus view on that. 00:55:43.600 |
of proving whether we had product-market fit out. 00:55:45.560 |
Because I think this is true of a lot of AI projects. 00:55:47.880 |
You can watch a project, and people might use it a bit, 00:55:50.400 |
and people might stop using it, and you're like, 00:55:52.240 |
well, I don't know, is that product-market fit? 00:55:55.480 |
However, if you work very closely with the customer 00:55:59.080 |
it's easier to understand their sort of requests, 00:56:25.200 |
have invested more on the self-serve ubiquity side. 00:56:32.960 |
And the reason for that is, when we started Retool, 00:56:35.720 |
we always wanted, actually, some percent of software 00:56:41.400 |
or broadly, UIs, but like software, basically. 00:56:44.240 |
And for us, we were like, we think that maybe one day, 00:56:52.160 |
or 10% of the software could be running on Retool, 00:56:59.480 |
it really does require a broad-basis option of the platform. 00:57:04.080 |
It can't just be like, oh, only like 1,000 customers, 00:57:07.280 |
but the largest 1,000 companies in the world use it. 00:57:09.360 |
It has to be like, all the developers in the world use it. 00:57:15.280 |
This is, of course, how do you get to all the developers? 00:57:18.640 |
And the only way to get to all those developers 00:57:20.880 |
You can't have a salesperson talk to 30 million people. 00:57:25.120 |
bottoms-up, product-led, ubiquity kind of way, basically. 00:57:28.000 |
And so, for us, we actually changed our focus 00:57:31.400 |
to be ubiquity, actually, over the last year. 00:57:33.520 |
it used to always be sort of revenue-generated, 00:57:37.480 |
We actually changed it to be number of developers 00:57:39.160 |
building on the platform, actually, last year. 00:57:41.360 |
And that, I think, was actually a really clarifying change, 00:57:53.920 |
to get to something like 10, 20, 30 million developers 00:57:57.120 |
We can't convince all developers that Retool's 00:57:58.600 |
a better way of building a sort of class of software, 00:58:03.440 |
And so, I think that has been a pretty good outcome. 00:58:07.600 |
Like, I think about, you know, the last, like, 00:58:20.320 |
I do think the focus towards, like, you know, 00:58:22.000 |
bottom-left ubiquity also is really important, 00:58:23.840 |
because it helps us get to our long-term outcome. 00:58:25.960 |
What's interesting, I think, is that long-term ubiquity, 00:58:31.920 |
Like, to your point, I think at Silicon Valley, 00:58:37.040 |
I think, like, if you're starting a startup today, 00:58:41.000 |
you're probably going to consider Retool, at least. 00:58:43.800 |
because you're like, "Hey, I'm not ready for it yet," 00:58:45.000 |
or something, but you're going to consider it, at least. 00:58:52.720 |
But it's that, you know, if you think about, you know, 00:59:03.360 |
that use Retool at this point, which is really awesome. 00:59:09.280 |
probably has never heard of Retool, actually. 00:59:10.920 |
And so, that is where the challenge really is. 00:59:22.240 |
go to Amazon and knock on every developer's door 00:59:24.000 |
or send out an email to every developer and be like, 00:59:29.520 |
and you love it, and you tell your coworker about it. 00:59:40.160 |
- Yeah, and just, like, general market thoughts on AI. 00:59:43.880 |
Are you, you know, do you spend a lot of time 00:59:46.160 |
thinking about, like, AGI stuff or regulation or safety? 00:59:57.080 |
Well, I'll give you a little bit of the Retool context, 01:00:01.120 |
In my opinion, there's a lot of hype in AI right now, 01:00:15.600 |
Like, I think most founders that I meet at the AI space 01:00:21.400 |
sort of, real use cases people want to pay money for. 01:00:23.080 |
So, I don't think really where the Retool interest comes from. 01:00:34.280 |
and like, you know, what would it take for me to say, 01:00:39.280 |
yes, GPT-X or any sort of model actually is AGI? 01:00:47.400 |
I think it's kind of challenging, because it's like, 01:00:51.120 |
I think, if you look at, like, evolution, for example, 01:00:53.240 |
like, humans have been programmed to do, like, 01:01:05.360 |
you have to go eat food, you know, for example. 01:01:07.320 |
To survive, maybe, like, having more resources 01:01:10.880 |
helps you want to go make money, you know, for example. 01:01:13.680 |
To reproduce, you should go date, you know, or whatever. 01:01:15.640 |
You should get married and stuff like that, right? 01:01:16.960 |
So, like, that's, we have a program to do that. 01:01:19.000 |
And humans that are good at that have propagated. 01:01:22.600 |
And so, humans that, you know, we're not actually surviving, 01:01:27.200 |
Humans that we're not interested in reproducing 01:01:34.280 |
because they just, they're just up here and gone, basically. 01:01:42.560 |
I think the third aim I was thinking about was, like, 01:01:45.520 |
So, maybe, like, happier humans, you know, survival? 01:01:54.520 |
like, right now, we're not really selecting AIs 01:01:57.480 |
Like, it's not like, you know, we're being like, 01:01:58.720 |
"Hey, AI, you know, you should go make 30 other AIs." 01:02:06.760 |
thinking about where intentionality for humans come from. 01:02:09.320 |
And, like, I think you can argue the intentionality 01:02:10.840 |
for humans basically comes out of these three things. 01:02:12.360 |
You know, like, you know, if you want to be happy, 01:02:15.040 |
That's, like, basically your sort of goal, you know, in life. 01:02:18.360 |
Whereas, like, the AI doesn't really have that. 01:02:22.760 |
Like, if you, you know, prompt inject, for example, 01:02:24.520 |
like, "Hey, AI, you know, go do these things." 01:02:27.160 |
And, you know, you can even create a simulation, 01:02:34.400 |
So, that's kind of stuff I've been thinking about 01:02:46.680 |
maybe not at the sort of trained final model level, 01:02:57.120 |
that sort of evolutionary selection pressure, 01:03:01.680 |
And, you know, I guess one of the early concerns 01:03:05.400 |
about being in Sydney and sort of, like, bootstrap, 01:03:10.400 |
self-bootstrapping AGI is that it actually is, 01:03:17.080 |
to get as much of their data out there into our datasets 01:03:31.800 |
- David, I know we're both fan of Hofstadter's GEB. 01:03:41.400 |
you referred to the anteater, like, piece of the, 01:03:49.000 |
and GEB is just kind of like this discontinuous riff, 01:03:52.320 |
but basically like how ants are like not intelligence, 01:03:55.200 |
but like ant colony has signs of intelligence. 01:03:57.920 |
And I think Hofstadter then used that to say, 01:04:00.960 |
hey, you know, neurons are kind of like similar, 01:04:07.200 |
we're drawing the wrong conclusion for, like, 01:04:14.120 |
and then you tie them together, it should be like a brain. 01:04:16.680 |
But maybe like the neuron is like different models 01:04:19.400 |
that then get tied together to make the brain. 01:04:26.720 |
of interesting philosophical discussions to have. 01:04:30.360 |
Sean and I recorded a monthly recap podcast yesterday, 01:04:32.960 |
and we had a similar discussion on are we using the wrong, 01:04:57.160 |
machines have always evolved differently than humans. 01:04:59.600 |
So why should we expect AI to be any different? 01:05:02.080 |
- Yeah, if you sort of peer under the hood of AGI, 01:05:05.080 |
if you insist on AGI, we have always used AGI 01:05:14.040 |
Like, if it works, no, it's not the Turing test. 01:05:17.240 |
The Turing test is if the output is the same as a human, 01:05:20.640 |
I don't really care about what's going on inside. 01:05:29.400 |
It does not fly necessarily the same way as a bird. 01:05:39.840 |
And it does seem to be like AGI is probably, like, 01:05:42.320 |
doesn't think and can achieve, like, outcomes that I give it 01:05:48.280 |
Like, I kind of don't care what it is, like, under the hood. 01:05:56.160 |
I actually have GUB right here on my bookshelf. 01:06:00.200 |
"Man, I can't believe I got through it once." 01:06:06.480 |
- Yeah, I mean, I started studying physics in undergrad, 01:06:18.800 |
and looking forward to the 2024 set of AI results