back to indexWojciech Zaremba: OpenAI Codex, GPT-3, Robotics, and the Future of AI | Lex Fridman Podcast #215
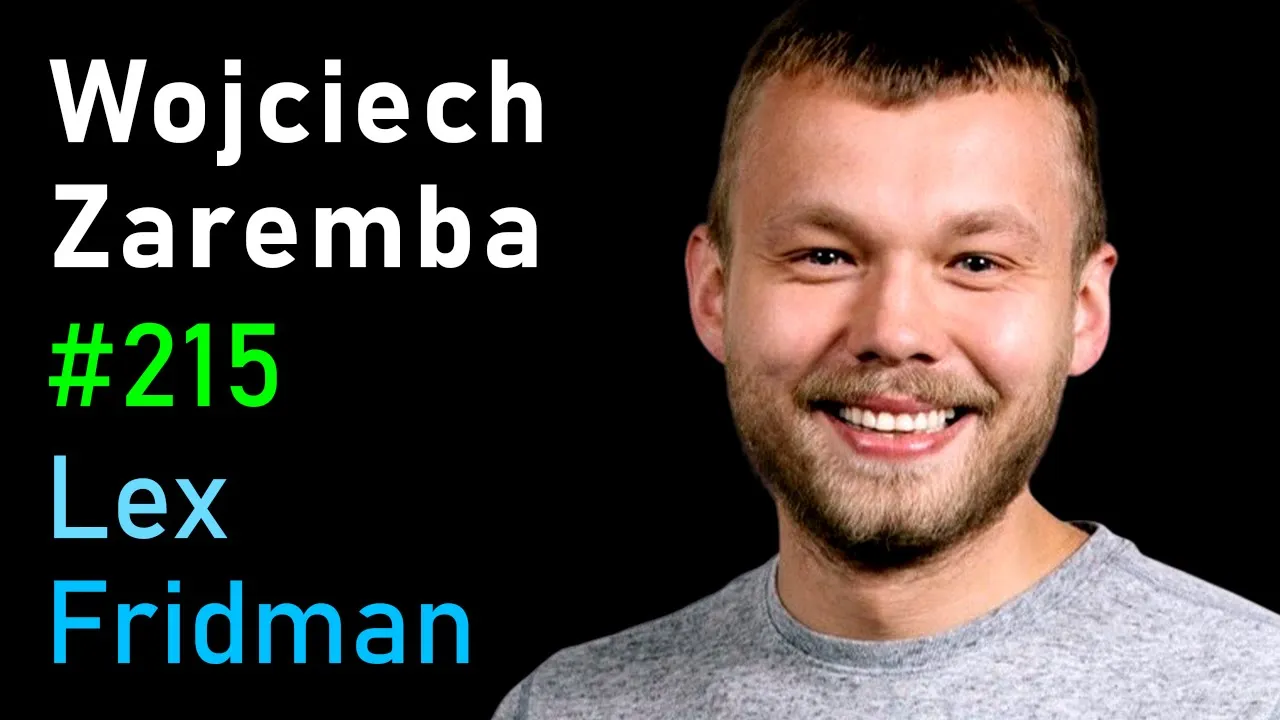
Chapters
0:0 Introduction
1:18 The Fermi paradox
8:20 Systems of government
10:57 Life, intelligence, and consciousness
18:10 GPT language model
20:23 Engineering consciousness
24:40 Is there an algorithm for intelligence?
32:2 Neural networks and deep learning
44:53 Human reward functions
49:47 Love is part of the human condition
52:14 Expanding our circle of empathy
56:19 Psychedelics and meditation
67:46 Ilya Sutskever
75:3 How does GPT work?
84:56 AI safety
91:42 OpenAI Codex
105:15 Robotics
114:32 Developing self driving cars and robots
125:23 What is the benchmark for intelligence?
128:25 Will we spend more time in virtual reality?
130:39 AI Friendships
140:9 Sleep
142:43 Generating good ideas
149:8 Advice for young people
153:52 Getting started with machine learning
157:5 What is beauty?
160:56 Death
167:44 Meaning of life
00:00:00.000 |
The following is a conversation with Wojciech Zaremba, 00:00:05.520 |
which is one of the top organizations in the world 00:00:08.460 |
doing artificial intelligence research and development. 00:00:12.340 |
Wojciech is the head of language and cogeneration teams, 00:00:16.820 |
building and doing research on GitHub Copilot, 00:00:28.140 |
and N+1, and he also previously led OpenAI's robotic efforts. 00:00:37.620 |
that deeply challenge and expand our understanding 00:00:44.080 |
The 21st century, I think, may very well be remembered 00:00:53.240 |
GPT, Codecs, and applications of language models 00:01:05.820 |
To support this podcast, please check out our sponsors. 00:01:13.980 |
and here is my conversation with Wojciech Zaremba. 00:01:24.200 |
and the people at OpenAI had really sophisticated, 00:01:31.780 |
So let me ask you about the Fermi Paradox, about aliens. 00:01:45.620 |
on what might be a, let's say, possible answer. 00:01:47.960 |
It's also interesting that the question itself 00:01:55.880 |
because if you assume that we don't see aliens 00:02:03.840 |
on making sure that we won't destroy ourselves. 00:02:10.720 |
with my belief, and these things also change over the time, 00:02:14.160 |
is I think that we might be alone in the universe, 00:02:20.440 |
or let's say consciousness life, more kind of valuable, 00:02:23.500 |
and that means that we should more appreciate it. 00:02:27.760 |
So what's your intuition about our galaxy, our universe? 00:02:34.120 |
of intelligent civilizations, or are we truly, 00:02:40.200 |
- At the moment, my belief that it is unique, 00:02:45.200 |
there was some footage released with UFO objects, 00:02:53.200 |
- Yeah, I can tell you one crazy answer that I have heard. 00:03:00.440 |
at the limits of computation, you can compute more 00:03:04.360 |
if the temperature of the universe would drop down. 00:03:08.560 |
So one of the things that aliens might want to do 00:03:13.220 |
if they are truly optimizing to maximize amount of compute, 00:03:20.440 |
it's instead of wasting current entropy of the universe, 00:03:28.880 |
then you can wait for the universe to cool down 00:03:44.960 |
in actually going to other galaxies if you can go inwards. 00:03:49.440 |
So there is no limits of what could be an experience 00:03:53.360 |
if we could, you know, connect machines to our brains, 00:04:00.280 |
- Yeah, there could be a lot of ways to go inwards too. 00:04:09.000 |
maybe you can travel to different dimensions. 00:04:22.520 |
And it doesn't require a spaceship going slowly 00:04:28.360 |
- It also feels, you know, one of the problems 00:04:31.000 |
is that speed of light is low and the universe is vast. 00:04:40.320 |
then we would instead of actually sending spaceships 00:04:51.060 |
These are like a huge sail, which is at first powered, 00:04:57.740 |
and it can propel it to quarter of speed of light. 00:05:01.300 |
And sail itself contains a few grams of equipment. 00:05:12.380 |
But then when you think what would it mean for humans, 00:05:20.840 |
I don't know, play them YouTube or let's say, 00:05:23.460 |
or like a 3D print like huge human right away 00:05:45.620 |
How can we leave some remnants if we do destroy ourselves? 00:05:58.300 |
do we have it like in a satellite orbiting earth 00:06:03.620 |
Like how do we say, how do we back up human civilization 00:06:08.500 |
for the good parts or all of it is good parts 00:06:12.100 |
so that it can be preserved longer than our bodies can? 00:06:24.700 |
And if we die, we may die suddenly as a civilization. 00:06:41.940 |
that actually can apparently kill entire galaxy. 00:06:51.220 |
I'm also, and I'm looking actually at the past civilization. 00:06:55.900 |
they disappeared from the surface of the earth. 00:07:06.340 |
you know, that definitely they had some problem 00:07:14.500 |
there was no potable water and they all died. 00:07:22.100 |
the best solution to such problems is I guess technology. 00:07:27.700 |
So, I mean, if they would know that you can just boil water 00:07:34.220 |
And even now, when we look actually at the current pandemic, 00:07:37.860 |
it seems that once again, actually science comes to rescue 00:07:41.420 |
and somehow science increases size of the action space. 00:07:47.500 |
- Yeah, but nature has a vastly larger action space. 00:08:01.940 |
But looking at the destruction of human civilization, 00:08:05.860 |
perhaps expanding the action space will add actions 00:08:20.460 |
I was pondering why actually even we have negative impact 00:08:36.300 |
that as a collective, we are not going in this direction. 00:08:39.300 |
I think that there exists very powerful system 00:08:43.140 |
to describe what we value, that's capitalism. 00:08:45.340 |
It assigns actually monetary values to various activities. 00:08:49.140 |
At the moment, the problem in the current system 00:08:51.820 |
is that there are some things which we value, 00:09:08.380 |
companies, corporations can pollute them for free. 00:09:23.980 |
And we are kind of maybe even moving in this direction. 00:09:26.700 |
The first issue is even to be able to measure 00:09:30.660 |
then we can actually assign the monetary value to them. 00:09:39.580 |
enabling people to vote and to move money around 00:09:50.300 |
So like having one president and Congress and voting 00:09:55.900 |
that happens every four years or something like that, 00:10:00.660 |
There could be some technological improvements 00:10:03.660 |
- So I'm thinking from time to time about these topics, 00:10:06.740 |
but it's also feels to me that it's a little bit like, 00:10:10.380 |
it's hard for me to actually make correct predictions 00:10:14.740 |
I extremely trust Sam Altman, our CEO on these topics. 00:10:31.620 |
Well, I think self-doubt and I think hippie implies optimism. 00:10:37.700 |
Those two things are pretty good way to operate. 00:10:43.260 |
- I mean, still it is hard for me to actually understand 00:10:57.420 |
- What do you think is rarest in the universe? 00:11:01.820 |
What's hardest to build is another engineering way 00:11:22.700 |
- So let me at first explain you my kind of mental model, 00:11:33.660 |
there was this primordial soup of amino acids 00:11:41.340 |
And some proteins were turning into some other proteins 00:11:57.140 |
And essentially life means that all the sudden 00:12:11.020 |
and it needs like a sufficient number of edges 00:12:17.300 |
Then from perspective of intelligence and consciousness, 00:12:21.580 |
my current intuition is that they might be quite intertwined. 00:12:26.340 |
First of all, it might not be that it's like a binary thing 00:12:30.780 |
It seems to be more like a continuous component. 00:12:48.740 |
with activations in visual cortex of some monkeys. 00:12:52.860 |
The same seems to be true about language models. 00:13:04.900 |
at first, it barely recognizes what is going on. 00:13:10.500 |
Over the time it kind of recognizes foreground 00:13:12.980 |
from background, over the time it kind of knows 00:13:15.580 |
where there is a foot and it just follows it. 00:13:19.580 |
Over the time, it actually starts having a 3D perception. 00:13:27.100 |
and ask what would it see if it looks to the right. 00:13:29.860 |
And the crazy thing is, initially when the agents 00:13:33.020 |
are barely trained, that these predictions are pretty bad. 00:13:35.900 |
Over the time, they become better and better. 00:13:38.580 |
You can still see that if you ask what happens 00:13:45.220 |
for some time, they think that the different thing appears. 00:13:48.340 |
And then at some stage, they understand actually 00:13:52.780 |
So they get like a understanding of 3D structure. 00:13:55.900 |
It's also very likely that they have inside some level 00:14:03.420 |
like they're particularly symbols for other agents. 00:14:06.860 |
So when you look at Dota agents, they collaborate together. 00:14:11.780 |
And they have some anticipation of if they would win battle, 00:14:16.780 |
they have some expectations with respect to other agents. 00:14:28.580 |
But then the fact that they have a symbol for other agents 00:14:40.180 |
they would have also symbol to describe themselves. 00:14:51.140 |
And still it might be different from the consciousness. 00:14:58.980 |
the word consciousness, let's say the experience 00:15:01.260 |
of drinking a coffee, or let's say experience of being a bat, 00:15:04.260 |
that's the meaning of the word consciousness. 00:15:09.860 |
Yeah, it feels, it might be also somewhat related 00:15:15.460 |
So it's kind of, okay, if you look at anesthetic drugs, 00:15:34.020 |
- And so there's a lessening of consciousness 00:15:45.340 |
It could be that it's this kind of self-awareness module 00:15:50.300 |
that you described, plus the actual subjective experience 00:15:55.300 |
is a storytelling module that tells us a story 00:16:05.220 |
- The crazy thing, so let's say, I mean, in meditation, 00:16:08.580 |
they teach people not to speak story inside of the head. 00:16:12.820 |
And there is also some fraction of population 00:16:30.780 |
So it seems that it's possible to have the experience 00:16:41.140 |
Is that the voice when you're reading a book? 00:16:42.340 |
- Yeah, I thought that that's what you are referring to. 00:16:45.100 |
- Well, I was referring more on the not an actual voice. 00:16:50.100 |
I meant there's some kind of subjective experience 00:16:56.180 |
feels like it's fundamentally about storytelling 00:17:17.460 |
So there feels like it's a very high level abstraction 00:17:21.020 |
that is useful for me to feel like entity in this world. 00:17:26.020 |
Most useful aspect of it is that because I'm conscious, 00:17:33.180 |
I think there's an intricate connection to me 00:17:37.620 |
So it's a useful hack to really prioritize not dying. 00:17:46.860 |
So I'm telling the story of it's richly feels 00:18:01.020 |
that I will just refer maybe to the first part, 00:18:12.580 |
so obviously I like thinking about consciousness. 00:18:19.700 |
and I'm trying to see analogies of these things in AI, 00:18:35.020 |
which can generate pretty amusing text on arbitrary topic. 00:18:45.820 |
is by putting into prefix at the beginning of the text, 00:18:50.580 |
some information, what would be the story about? 00:18:58.180 |
by saying that the chat is with Lex or Elon Musk or so, 00:19:01.860 |
and GPT would just pretend to be you or Elon Musk or so. 00:19:15.580 |
it's almost like things that you put into context of GPT. 00:19:21.300 |
but the context we provide to GPT is multimodal. 00:19:33.980 |
but from the experience of humanity, it's a chameleon. 00:19:41.940 |
it behaves as the thing that you wanted it to be. 00:19:47.300 |
It's interesting that people have stories of who they are. 00:20:00.220 |
I guess various people find it out through meditation or so, 00:20:03.100 |
that there might be some patterns that you have learned 00:20:10.820 |
And you also might be thinking that that's who you are, 00:20:30.020 |
Which meant what makes given mathematical equations 00:20:35.980 |
Similarly, I wonder what breathes fire into computation? 00:20:47.380 |
How do you breathe fire and magic into the machine? 00:20:58.700 |
just keep on multiplying one matrix over and over again, 00:21:09.620 |
what are the computations which could be conscious? 00:21:22.620 |
it's very important was the realization of computation 00:21:25.020 |
that it has to do with some force fields or so, 00:21:33.780 |
So in case of computation, you can ask yourself, 00:21:41.860 |
So for instance, if we think about the models, AI models, 00:21:58.620 |
and this turns out to be equivalent to compressing text. 00:22:09.140 |
compression means that you learn the model of reality, 00:22:12.860 |
and you have just to remember where are your mistakes, 00:22:19.140 |
And in some sense, when we look at our experience, 00:22:22.740 |
also when you look, for instance, at the car driving, 00:22:31.100 |
that the consciousness is intertwined with compression. 00:22:34.980 |
It might be also the case that self-consciousness 00:22:37.860 |
has to do with compressor trying to compress itself. 00:22:54.220 |
And then I thought, you know, you see in mathematics, 00:23:07.060 |
it is possible to point the mathematical system 00:23:48.580 |
- So, but do you think, if we think of a Turing machine, 00:23:57.340 |
So is there something beyond our traditional definition 00:24:05.500 |
And I said, this computation has to do with compression. 00:24:13.180 |
you're internally creating the model of reality 00:24:16.300 |
in order, it's like you try inside to simplify reality 00:24:24.940 |
to how I think actually about my own conscious experience. 00:24:31.260 |
The only access to reality is through, you know, 00:24:35.060 |
And my brain is creating a simulation of reality, 00:24:38.020 |
and I have access to the simulation of reality. 00:24:40.740 |
- Are you by any chance aware of the Hutter Prize, 00:24:46.540 |
He made this prize for compression of Wikipedia pages. 00:24:56.220 |
One, I think has to be perfect compression, which makes, 00:24:59.900 |
I think that little quirk makes it much less applicable 00:25:11.620 |
Like perfect compression feels like it's not the right goal, 00:25:16.620 |
but it's nevertheless a very interesting goal. 00:25:32.060 |
So you can make perfect compression if you store errors. 00:25:49.460 |
So there's an interesting, and now he's a DeepMind, 00:25:55.780 |
He's one of the people that I think seriously 00:26:00.740 |
took on the task of what would an AGI system look like? 00:26:21.100 |
If you remove the constraints of it having to be, 00:26:23.620 |
having to have a reasonable amount of memory, 00:26:30.700 |
reasonable amount of running time complexity, 00:26:40.140 |
half philosophical discussion of how would it, 00:26:43.260 |
like a reinforcement learning type of framework 00:26:57.060 |
there is infinite amount of memory and compute. 00:27:03.580 |
Huter extended Solomonov's work to reinforcement learning, 00:27:11.500 |
which is optimal algorithm to build intelligence. 00:27:40.420 |
that optimally solve the problem of intelligence. 00:27:46.460 |
So first of all, the task itself is described as 00:27:50.940 |
you have infinite sequence of zeros and ones, okay? 00:27:54.620 |
You read n bits and you're about to predict n plus one bit. 00:28:04.540 |
So if for instance, you have images and labels, 00:28:07.580 |
you can just turn every image into a sequence 00:28:09.540 |
of zeros and ones, then label, you concatenate labels 00:28:14.820 |
and you could start by having training data first, 00:28:21.260 |
So theoretically, any problem could be casted 00:28:37.140 |
And I will ask you to write every possible program 00:28:44.500 |
So, and you can have, you choose programming language. 00:28:50.780 |
And the difference between programming languages 00:28:56.180 |
asymptotically your predictions will be equivalent. 00:28:58.940 |
So you read n bits, you enumerate all the programs 00:29:10.780 |
you actually weight the programs according to their length. 00:29:15.780 |
And there is like a specific formula how you weight them. 00:29:41.940 |
or the intuition that the simple answer is the right one. 00:29:48.660 |
- It also means like if you would ask the question 00:29:56.900 |
You can say, it's more likely the answer is two 00:30:01.020 |
to some power because they're shorter program. 00:30:08.420 |
about how different the space of short programs 00:30:14.780 |
Like what is the universe where short programs 00:30:21.500 |
- So as I said, the things have to agree with n bits. 00:30:37.340 |
And that gives you the full program that agrees on n bits. 00:30:40.100 |
- Oh, so you don't agree perfectly with the n bits 00:30:44.860 |
- That's like a longer program, slightly longer program 00:30:48.340 |
because it contains these extra bits of errors. 00:31:01.700 |
Are they perfectly, like, is there if then statements 00:31:09.300 |
So like, is there a lot of exceptions that they're storing? 00:31:11.740 |
- So you could imagine if there would be tremendous amount 00:31:15.140 |
of if statements, then they wouldn't be that short 00:31:23.900 |
when you start with an uninitialized neural network, 00:31:38.300 |
which are slightly similar to the correct answer. 00:31:51.260 |
And the program space is represented by, you know, 00:31:55.020 |
kind of the wiring inside of the neural network. 00:32:02.700 |
- Let me ask you the high level basic question 00:32:12.340 |
that is different than like a generic textbook definition? 00:32:18.300 |
is maybe the closest to how I'm thinking these days 00:32:30.940 |
Seems that various modules that we are actually adding up 00:32:35.180 |
to like, you know, we want networks to be deep 00:32:38.260 |
because we want multiple steps of the computation. 00:32:47.340 |
to represent space of programs, which is searchable. 00:32:50.340 |
And it's searchable with stochastic gradient descent. 00:33:00.980 |
the things that tend to give correct answers. 00:33:23.100 |
And he was able to identify inside of the neural network, 00:33:28.100 |
for instance, a detector of a wheel for a car 00:33:36.140 |
and assemble them together using a simple program 00:33:46.060 |
defined programs, that's like a function within a program 00:33:49.300 |
that this particular neural network was able to find. 00:33:52.260 |
And you can tear that out, just like you can copy 00:33:56.500 |
So any program is a composition of smaller programs. 00:34:03.340 |
- Yeah, I mean, the nice thing about the neural networks 00:34:05.820 |
is that it allows the things to be more fuzzy 00:34:10.460 |
In case of programs, you have this like a branching 00:34:14.460 |
And the neural networks, they have an easier way 00:34:17.300 |
to be somewhere in between or to share things. 00:34:21.020 |
- What to use the most beautiful or surprising idea 00:34:32.300 |
neural networks is a bunch of, what would you say? 00:34:37.900 |
There's neurons, there's connection between those neurons. 00:34:40.380 |
There's inputs and there's outputs and there's millions 00:34:45.500 |
And the learning happens by adjusting the weights 00:34:56.380 |
I supposed to do it, but I guess you have enough empathy 00:34:59.060 |
to listeners to actually know that that might be useful. 00:35:02.740 |
- No, that's like, so I'm asking Plato of like, 00:35:09.340 |
You're being philosophical and deep and quite profound 00:35:13.940 |
which is very interesting, but also for people 00:35:17.100 |
who are just not familiar, what the hell we're talking about 00:35:28.540 |
And you worked on a lot of fascinating projects, 00:35:38.260 |
- Yeah, I mean, I'm thinking about the trick, 00:35:40.300 |
but like it's still amusing to me that it works at all. 00:35:44.700 |
That let's say that the extremely simple algorithm, 00:35:47.380 |
stochastic gradient descent, which is something 00:35:49.620 |
that I would be able to derive on the piece of paper 00:35:58.420 |
of thousands of machines actually can create the behaviors 00:36:04.980 |
which we called kind of human like behaviors. 00:36:07.980 |
- So in general, any application of stochastic gradient 00:36:11.380 |
descent in neural networks is amazing to you. 00:36:21.820 |
And also, what do you attribute that success to? 00:36:31.340 |
What profound insight can we take from the fact that 00:36:34.780 |
the thing works for gigantic sets of variables? 00:36:39.780 |
- I mean, the interesting thing is these algorithms, 00:36:57.820 |
and they spent a lot of cycles on very different algorithms. 00:37:01.300 |
And I believe that we have seen that various innovations 00:37:13.220 |
But it's also remarkable to me that this algorithm 00:37:20.180 |
that the gradient descent was invented by Leibniz 00:37:35.860 |
that it cannot be the case that such a simple algorithm 00:37:44.060 |
So they were in search for the other algorithms. 00:37:48.780 |
And as I'm saying, like, I believe that actually 00:37:54.260 |
There is compute, there are algorithms and there is data. 00:38:05.500 |
It's also interesting, so you ask, is it only compute? 00:38:21.140 |
But also we are in the world that in case of compute, 00:38:23.820 |
there is a kind of exponential increase in funding. 00:38:27.020 |
And at some point it's impossible to invest more. 00:38:31.820 |
We are speaking about, let's say all taxes in US. 00:38:46.620 |
So one piece is human brain is an incredible supercomputer. 00:38:58.940 |
Or like if you try to count various quantities in the brain, 00:39:05.700 |
that small number of neurons, there is a lot of synapses. 00:39:25.380 |
It also might be the case that they are more efficient 00:39:27.380 |
than brain or less efficient by some huge factor. 00:39:35.540 |
that these neural networks, they require a thousand X 00:39:39.500 |
or like a huge factor of more data than humans do. 00:39:54.020 |
is there are domains which contains million X more data. 00:40:04.940 |
Like for instance, I believe that it should be possible 00:40:14.260 |
and there are like even simple steps of doing it. 00:40:20.460 |
And you know, the core reason is there is just, 00:40:27.700 |
and then it should be able to speak simultaneously 00:40:31.220 |
And it should be possible to optimize it all in parallel. 00:40:35.060 |
- Now you're touching on something I deeply care about 00:40:47.620 |
So, okay, so one goal, now this is terrifying to me, 00:40:51.540 |
but there's a lot of people that contemplate suicide, 00:40:56.740 |
and they could significantly be helped with therapy. 00:41:14.060 |
So one goal for a therapist, whether human or AI, 00:41:19.060 |
is to prevent suicide ideation, to prevent suicide. 00:41:27.900 |
So to be clear, I don't think that the current models 00:41:33.460 |
because it requires insane amount of understanding, empathy, 00:41:36.660 |
and the models are far from this place, but it's- 00:41:40.380 |
- But do you think that understanding empathy, 00:41:45.740 |
- I think there is some signal in the data, yes. 00:41:47.660 |
I mean, there are plenty of transcripts of conversations 00:41:50.980 |
and it is possible from it to understand personalities. 00:41:59.460 |
if conversation is friendly, amicable, antagonistic. 00:42:07.820 |
given the fact that the models that we train now, 00:42:18.180 |
They might turn out to be better in understanding 00:42:20.700 |
personality of other people than anyone else. 00:42:37.740 |
to be able to be empathetic of the human experience, 00:42:42.060 |
whether language is not enough, to understand death, 00:42:45.060 |
to understand fear, to understand childhood trauma, 00:42:58.860 |
both humor and hope and love and all those kinds of things. 00:43:13.180 |
by just reading a huge number of transcripts? 00:43:21.020 |
It's like the same way as you cannot learn to dance 00:43:25.620 |
By watching it, you have to actually try it out yourself. 00:43:29.100 |
So I think that here, that's a similar situation. 00:43:40.180 |
And obviously, initially, it would have to go 00:43:54.340 |
or speak with someone than there are therapies out there. 00:44:12.220 |
I guess maybe human connection is a third one. 00:44:14.380 |
And I guess pharmacologically, it's also possible. 00:44:17.460 |
Maybe direct brain stimulation or something like that. 00:44:22.140 |
Then let's say the way I'm thinking about the AGI endeavor 00:44:37.420 |
these are like two endeavors that make sense to me. 00:44:40.020 |
One is like essentially increase amount of wealth. 00:44:42.700 |
And second one is increase overall human well-being. 00:44:48.500 |
- And they can, I would say, these are different topics. 00:45:05.580 |
So like therapist has a very kind of clinical sense to it. 00:45:25.340 |
as we desperately try to make sense of this world 00:45:29.740 |
in a deep, overwhelming loneliness that we feel inside. 00:45:34.700 |
- So I think connection has to do with understanding. 00:45:37.980 |
And I think that almost like a lack of understanding 00:45:41.540 |
If you speak with someone and you feel ignored, 00:45:52.740 |
what to do in life, but like a pure understanding. 00:46:00.780 |
just being heard, feel like you're being heard. 00:46:03.860 |
Like somehow that's a alleviation temporarily 00:46:13.340 |
with their body language, with the way they are, 00:46:17.340 |
with the way they look at you, with the way they talk, 00:46:25.180 |
So I thought in the past about a somewhat similar question 00:46:43.860 |
with some compression, which is more or less, 00:46:49.220 |
It seems to me that other aspect is there seem 00:46:52.740 |
to be reward functions and you can have a reward 00:47:10.860 |
might be optimizing slightly different reward functions. 00:47:13.620 |
They essentially might care about different things. 00:47:16.420 |
And in case of love, at least the love between two people, 00:47:22.540 |
you can say that the boundary between people dissolves 00:47:39.900 |
- In some sense, I would say love means helping others 00:47:55.060 |
- So love is, if you think of two reward functions, 00:48:07.500 |
if you are fully optimizing someone's reward function 00:48:10.180 |
without yours, then maybe are creating codependency 00:48:22.700 |
we ourselves, we are actually less of a unified insight. 00:48:33.460 |
"Oh, this looks tasty, I would like to eat it." 00:48:37.860 |
"I shouldn't be doing it because I want to gain muscles." 00:48:48.580 |
they're almost like a kind of intertwined personas. 00:48:51.780 |
And I believe that the self-love means that the love 00:49:27.420 |
- Angry, and then you're like, "You shouldn't be angry. 00:49:31.940 |
But like, instead actually you want Lex to come over, 00:49:35.060 |
give him a hug and just like say, "It's fine. 00:49:37.700 |
"Okay, you can be angry as long as you want." 00:49:44.060 |
- Or maybe not, but you cannot expect it even. 00:49:46.260 |
- Yeah, but still that doesn't explain the why of love. 00:49:49.740 |
Like, why is love part of the human condition? 00:49:52.180 |
Why is it useful to combine the reward functions? 00:50:00.260 |
I don't think reinforcement learning frameworks 00:50:04.020 |
Even the Hutter framework has an objective function 00:50:14.540 |
And in some sense, the purpose of evolution is survival. 00:50:17.660 |
And then this complicated optimization objective 00:50:25.300 |
which might help us operate in the real world 00:50:27.940 |
and it baked into us various reward functions. 00:50:31.740 |
- Then to be clear, at the moment we are operating 00:50:34.100 |
in the regime which is somewhat out of distribution 00:50:38.860 |
- It's almost like love is a consequence of cooperation 00:50:52.540 |
It's like, as you said, it evolved for cooperation. 00:50:58.420 |
cooperation ends up helping each of us individually. 00:51:05.580 |
love means there is this dissolution of boundaries 00:51:10.340 |
And we evolved to actually identify ourselves 00:51:14.300 |
So we can identify ourselves, you know, with a family. 00:51:24.300 |
So there is, we are wired actually even for love. 00:51:41.620 |
So you can clearly see when people travel around the world, 00:51:44.620 |
when they run into person from the same country, 00:51:48.900 |
And all of a sudden they find all these similarities. 00:51:56.780 |
So there is like a sense, some sense of the belonging. 00:52:01.460 |
good thing to the world for people to move towards, 00:52:09.900 |
move toward the mindset of a larger and larger groups. 00:52:13.900 |
- So the challenge there, that's a beautiful vision 00:52:17.580 |
and I share it to expand that circle of empathy, 00:52:21.060 |
that circle of love towards the entirety of humanity. 00:52:24.180 |
But then you start to ask, well, where do you draw the line? 00:52:27.340 |
Because why not expand it to other conscious beings? 00:52:34.220 |
something I think about is why not expand it to AI systems? 00:52:39.460 |
Like we start respecting each other when the person, 00:52:43.340 |
the entity on the other side has the capacity to suffer, 00:52:47.580 |
'cause then we develop a capacity to sort of empathize. 00:52:52.340 |
And so I could see AI systems that are interacting 00:52:55.460 |
with humans more and more having conscious-like displays. 00:52:59.980 |
So like they display consciousness through language 00:53:12.020 |
And so the reason we don't like torturing animals 00:53:22.460 |
And if AI looks like it's suffering when it's tortured, 00:53:27.460 |
how is that not requiring of the same kind of empathy 00:53:34.460 |
from us and respect and rights that animals do 00:53:43.820 |
or so make a progress in understanding what consciousness is 00:54:01.300 |
and the things that have this force, they are alive. 00:54:13.820 |
And in some sense, it might require essentially 00:54:23.820 |
- So the goal there, I mean, there's several things 00:54:25.660 |
with consciousness that make it a real discipline, 00:54:28.340 |
which is one is rigorous measurement of consciousness. 00:54:32.460 |
And then the other is the engineering of consciousness, 00:54:41.460 |
for the Department of DOT, Department of Transportation, 00:54:45.020 |
and a lot of different places put a value on human life. 00:54:48.740 |
I think DOT's value is $9 million per person. 00:54:53.220 |
So in that same way, you can get into trouble 00:54:57.860 |
if you put a number on how conscious a being is, 00:55:03.500 |
If a cow is 0.1 or like 10% as conscious as a human, 00:55:15.380 |
But then again, that might be a very good way to do it. 00:55:22.220 |
that actually we have scientific understanding 00:55:25.180 |
And then we'll be able to actually assign value 00:55:39.620 |
There is actually few other things that you could do 00:55:44.700 |
So you could imagine that the way even to measure 00:55:49.660 |
is by literally just plugging into the brain. 00:55:55.460 |
but the plugging into the brain and asking person 00:55:58.180 |
if they feel that their consciousness expanded. 00:56:03.140 |
You can say, if someone takes a psychedelic drug, 00:56:05.820 |
they might feel that their consciousness expanded 00:56:08.220 |
even though that drug itself is not conscious. 00:56:11.100 |
- Right, so like you can't fully trust the self-report 00:56:14.180 |
of a person saying their consciousness is expanded or not. 00:56:18.460 |
Let me ask you a little bit about psychedelics 00:56:22.660 |
'cause there've been a lot of excellent research 00:56:24.900 |
on different psychedelics, psilocybin, MDMA, even DMT, 00:56:32.580 |
What do you think psychedelics do to the human mind? 00:56:46.220 |
or is there some profound expansion of the mind? 00:57:18.020 |
Drugs, they are changing some hyperparameters 00:57:23.180 |
It is possible, thanks to change of these hyperparameters, 00:57:30.780 |
that we took for granted, they are changeable. 00:57:35.420 |
So they allow to have an amazing perspective. 00:57:39.260 |
There is also, for instance, the fact that after DMT, 00:57:42.980 |
people can see the full movie inside of their head, 00:57:47.620 |
gives me further belief that the brain can generate 00:57:51.940 |
that full movie, that the brain is actually learning 00:57:58.100 |
that it tries to predict what's gonna happen next. 00:58:06.060 |
Yeah, and it's also kind of interesting to me 00:58:08.380 |
that somehow there seems to be some similarity 00:58:29.780 |
and it feels after, like after second or third day 00:58:33.340 |
of meditation, there is almost like a sense of tripping. 00:58:49.260 |
and you meditate for extended period of time. 00:58:54.420 |
- Yeah, so it's optimized, even though there are other people 00:59:01.460 |
you don't actually look into other people's eyes. 00:59:07.620 |
So Vipassana meditation tells you to focus on the breath. 00:59:13.700 |
So you try to put all attention into breathing in 00:59:20.940 |
And the crazy thing is that as you focus attention like that 00:59:25.940 |
after some time, there's some starts coming back 00:59:30.340 |
like some memories that you're completely forgotten. 00:59:38.340 |
and then you know, you are just like archiving email 00:59:43.700 |
And at some point there is like a amazing feeling 00:59:53.700 |
It's kind of, it's crazy to me that once you resolve 01:00:12.020 |
the default state of human mind is extremely peaceful 01:00:20.620 |
it feels at least to me the way how when I was a child 01:00:28.180 |
that I can look at any object and it's very beautiful. 01:00:31.540 |
I have a lot of curiosity about the simple things. 01:00:34.500 |
And that's where usually meditation takes me. 01:00:40.580 |
Are you just taking in simple sensory information 01:00:48.700 |
So there's no memories or all that kind of stuff. 01:00:56.460 |
I mean, still there is, thoughts are slowing down. 01:01:04.980 |
the extended meditation takes you to the space 01:01:07.100 |
that they are way more friendly, way more positive. 01:01:19.660 |
it almost feels that we are constantly getting 01:01:27.780 |
and we are just spreading this reward function 01:01:31.620 |
But if you stay still for extended period of time, 01:01:35.220 |
it kind of accumulates, accumulates, accumulates. 01:01:44.300 |
and it feels as drop is falling into kind of ocean 01:01:50.740 |
And that's like a, this is like a very pleasant, 01:01:55.180 |
that corresponds to the subjective experience. 01:01:58.140 |
Some people, I guess, in spiritual community, 01:02:05.700 |
And I would say, I believe that there are like 01:02:07.460 |
all sorts of subjective experience that one can have. 01:02:10.540 |
And I believe that for instance, meditation might take you 01:02:28.300 |
- I wonder how that maps to your mathematical model of love 01:02:33.300 |
with the reward function, combining a bunch of things. 01:02:42.660 |
we have this reward function and we're accumulating 01:02:53.140 |
And what meditation is, is you just remove them, 01:03:05.300 |
- Yeah, so something similar to how I'm thinking about this. 01:03:14.220 |
And I think almost about it as a text prepended to GPT. 01:03:32.980 |
And then with meditation, you can get to the point 01:03:35.180 |
that actually you experience things without the prompt. 01:03:47.540 |
And then with respect to the reward function, 01:03:55.580 |
And therefore you can say that you are having a, 01:04:01.020 |
the reward function of everyone else or like everything. 01:04:07.940 |
And that's also, you know, very beautiful, very pleasant. 01:04:11.500 |
At some point, you might have a lot of altruistic thoughts 01:04:15.260 |
during that moment, and then the self always comes back. 01:04:30.220 |
- I think that actually retreat is the way to go. 01:04:43.580 |
Once you get the high dose, actually you're gonna feel it. 01:04:51.580 |
for a prolonged period of time, just go to a retreat. 01:04:59.740 |
- And it's like, it's interesting that first or second day, 01:05:03.780 |
it's hard, and at some point it becomes easy. 01:05:17.340 |
it literally just depends on your own framing. 01:05:51.020 |
your legs being sore, all that kind of stuff? 01:05:58.820 |
The crazy thing is, you at first might have a feeling 01:06:11.980 |
and at some point it just becomes, it just is. 01:06:16.300 |
It's like, I remember Ilya told me some time ago 01:06:39.140 |
It is purely observing how the water goes through my body, 01:06:46.980 |
- And I would say then it actually becomes pleasant. 01:07:10.700 |
You're accepting of the full beauty of reality, 01:07:22.740 |
That's the only way to deal with a cold shower 01:07:25.740 |
is to become an observer and to find joy in it. 01:07:30.340 |
Same with really difficult physical exercise, 01:07:35.340 |
or running for a really long time, endurance events, 01:07:38.620 |
just any time you're exhausted, any kind of pain. 01:07:41.180 |
I think the only way to survive it is not to resist it, 01:07:58.620 |
He's brilliant, I really enjoy talking to him. 01:08:00.900 |
His mind, just like yours, works in fascinating ways. 01:08:07.420 |
Both of you are not able to define deep learning simply. 01:08:17.740 |
on in-space machine learning, deep learning, AI, 01:08:33.220 |
- So I believe that we have extreme respect to each other. 01:08:43.220 |
both like, I guess, about consciousness, life, AI. 01:08:48.300 |
- But in terms of the, it's interesting to me 01:08:56.100 |
in the space of machine learning, like intuition, 01:09:04.860 |
why it works, why it doesn't, and so is Ilya. 01:09:10.580 |
deep discussions you've had with him in the past 01:09:15.300 |
- So I can say, I also understood over the time 01:09:20.940 |
So obviously we have plenty of AI discussions. 01:09:29.340 |
but I consider Ilya one of the most prolific AI scientists 01:09:35.740 |
And I think that I realized that maybe my super skill 01:09:40.740 |
is being able to bring people to collaborate together, 01:09:49.380 |
And that might come from either meditation, psychedelics, 01:09:53.380 |
or let's say I read just hundreds of books on this topic. 01:10:12.100 |
but then I'm pretty good in assembling teams. 01:10:20.780 |
I grew many of them, like a research managers. 01:10:28.420 |
and he finds like his deep scientific insights 01:10:33.300 |
And you find ways you can, the puzzle pieces fit together. 01:10:37.460 |
Like, ultimately, for instance, let's say Ilya, 01:10:48.780 |
By default, I'm an extrovert and I care about people. 01:11:09.740 |
which is coming up with a good model for pedestrians, 01:11:18.820 |
And he immediately started to like formulate a framework 01:11:23.820 |
within which you can evolve a model for pedestrians, 01:11:27.380 |
like through self-play, all that kind of mechanisms. 01:11:29.980 |
The depth of thought on a particular problem, 01:11:34.340 |
especially problems he doesn't know anything about, 01:11:40.260 |
yeah, the limits that the human intellect might be limitless. 01:11:47.580 |
Or it's just impressive to see a descendant of ape 01:11:52.740 |
- Yeah, I mean, so even in the space of deep learning, 01:11:57.500 |
there are people who invented some breakthroughs once, 01:12:02.500 |
but there are very few people who did it multiple times. 01:12:06.340 |
And you can think if someone invented it once, 01:12:14.180 |
if a probability of inventing it once is one over a million, 01:12:17.340 |
then probability of inventing it twice or three times 01:12:19.660 |
would be one over a million squared or to the power of three, 01:12:25.100 |
So it literally means that it's given that it's not the luck. 01:12:33.180 |
who have a lot of these inventions in his arsenal. 01:12:41.940 |
if you think about folks like Gauss or Euler, 01:12:49.220 |
and then they did thinking and then they figure out math. 01:13:14.500 |
Like he makes me realize that there's like deep thinking 01:13:21.580 |
Like you really have to put a lot of effort to think deeply. 01:13:31.940 |
It's like an airplane taking off or something. 01:13:55.060 |
in terms of like deeply think about particular problems, 01:13:58.980 |
whether it's a math, engineering, all that kind of stuff. 01:14:07.020 |
And some of us are better than others at that. 01:14:11.780 |
with actually even engineering your environment 01:14:16.580 |
So see, both Ilya and I, on the frequent basis, 01:14:21.580 |
we kind of disconnect ourselves from the world 01:14:24.180 |
in order to be able to do extensive amount of thinking. 01:14:28.060 |
So Ilya usually, he just leaves iPad at hand. 01:14:39.980 |
just going for a few days to different location, to Airbnb, 01:14:50.780 |
to be able to actually just formulate new thoughts, 01:14:58.900 |
the more of this like random tasks are at hand. 01:15:09.380 |
Let me ask you another ridiculously big question. 01:15:15.940 |
Or like you say in your Twitter bio, GPT-N+1, 01:15:29.020 |
Let's assume that we know what is neural network, 01:15:50.220 |
And it becomes really exceptional at the task 01:15:56.300 |
So you might ask, why would this be an important task? 01:16:01.300 |
Why would it be important to predict what's the next word? 01:16:05.060 |
And it turns out that a lot of problems can be formulated 01:16:12.380 |
So GPT is purely learning to complete the text. 01:16:25.980 |
It turns out that many more things can be formulated 01:16:34.580 |
You make it even look like some content of a website 01:16:41.940 |
So it would be EN colon, text in English, FR colon. 01:16:46.780 |
And then you ask people, and then you ask model to continue. 01:16:54.540 |
is predicting translation from English to French. 01:16:57.140 |
The crazy thing is that this model can be used 01:17:09.180 |
And that might be a conversation between you and Elon Musk. 01:17:12.820 |
And because the model read all the texts about Elon Musk, 01:17:36.620 |
And the model will complete the text as a friendly AI bot. 01:17:42.660 |
- So, I mean, how do I express how amazing this is? 01:17:53.100 |
generating a conversation between me and Elon Musk, 01:18:09.220 |
It's not just like inklings of semantic correctness. 01:18:14.220 |
It's like the whole thing, grammatical, syntactic, semantic. 01:18:22.660 |
It's just really, really impressive generalization. 01:18:29.980 |
- Yeah, I mean, I also want to provide some caveats. 01:18:33.180 |
So it can generate few paragraphs of coherent text, 01:18:45.700 |
- What way does it go off the rails, by the way? 01:18:47.860 |
Is there interesting ways in which it goes off the rails? 01:18:54.060 |
- So the model is trained on the old existing data 01:18:58.980 |
which means that it is not trained on its own mistakes. 01:19:16.940 |
I'm start actually making up things which are not factual. 01:19:27.580 |
- Like, I don't know, I would say that Elon is my wife 01:19:31.100 |
and the model will just keep on carrying it on. 01:19:39.060 |
if you would have a normal conversation with Elon, 01:19:43.260 |
- Yeah, there would be some feedback between. 01:19:46.500 |
So the model is trained on things that humans have written, 01:19:57.420 |
- It magnifies, like the errors get magnified and magnified. 01:20:03.420 |
I mean, first of all, humans have the same problem. 01:20:13.860 |
- I think that actually what happens with humans is 01:20:16.460 |
if you have a wrong belief about the world as a kid, 01:20:19.780 |
then very quickly you will learn that it's not correct 01:20:24.660 |
and you are learning from your new experience. 01:20:27.700 |
But do you think the model can correct itself too? 01:20:31.100 |
Won't it through the power of the representation, 01:20:34.980 |
and so the absence of Elon Musk being your wife, 01:20:39.860 |
information on the internet, won't it correct itself? 01:20:50.620 |
you can also say that the data that is not out there 01:20:54.420 |
is the data which would represent how the human learns. 01:20:57.260 |
And maybe model would be trained on such a data, 01:21:06.540 |
Like when you think about the nature of intelligence, 01:21:12.580 |
But then if you think about the big AGI problem, 01:21:26.780 |
And I would expect that the systems that we are building, 01:21:31.780 |
they might end up being superhuman on some axis, 01:21:38.180 |
It would be surprising to me on all axis simultaneously, 01:21:45.500 |
is GPT a spaceship that would take us to the moon, 01:21:53.020 |
that we are just building bigger and bigger ladder? 01:22:08.020 |
But you're saying like the spaceship to the moon 01:22:15.020 |
they say, "You're guys just building a taller ladder." 01:22:22.220 |
And at the moment, I would say the way I'm thinking is, 01:22:29.500 |
And I'm also in heart, I'm a builder creator. 01:22:37.900 |
And so far we see constantly that there is a progress. 01:22:58.420 |
Then again, like GPT-3 is already very, truly surprising. 01:23:02.300 |
The people that criticize GPT-3 as a, what is it? 01:23:07.620 |
I think too quickly get accustomed to how impressive it is, 01:23:15.700 |
with accuracy of syntax, grammar, and semantics. 01:23:26.900 |
- I mean, definitely there will be more impressive models. 01:23:34.980 |
And also even the way I'm thinking about these models 01:23:44.060 |
you know, we see some level of the capabilities, 01:23:46.340 |
but we don't even fully understand everything 01:24:05.380 |
- Yeah, I mean, so when I'm thinking from perspective of, 01:24:08.740 |
obviously various people have concerns about AGI, 01:24:17.340 |
what's the strategy even to deploy these things to the world? 01:24:20.820 |
Then the one strategy that I have seen many times working 01:24:37.380 |
And it's almost, you don't want to be in that situation 01:24:46.740 |
then you deploy it and it might have a random chaotic impact 01:25:01.580 |
I've been reading a lot about Stalin and power. 01:25:10.180 |
If you're in possession of a system that's like AGI, 01:25:52.420 |
there's always a moment when somebody gets a lot of power 01:25:58.340 |
and the moral compass to give away that power. 01:26:03.620 |
That humans have been good and bad throughout history 01:26:08.900 |
And I wonder, I wonder we like blind ourselves in, 01:26:15.540 |
a race towards, yeah, AI race between nations. 01:26:20.540 |
We might blind ourselves and justify to ourselves 01:26:24.420 |
the development of AI without distributing the power 01:26:28.140 |
because we want to defend ourselves against China, 01:26:34.220 |
And I wonder how we design governance mechanisms 01:26:49.620 |
I have been thinking about this topic quite a bit, 01:26:55.100 |
I actually want to rely way more on Sam Altman on it. 01:27:09.140 |
of the companies rather than profit and to distribute it. 01:27:18.740 |
I guess I personally have insane trust in Sam. 01:27:31.900 |
That gives me, I guess, maybe some level of trust to him, 01:27:51.340 |
Like you're describing the process of open AI, 01:27:59.460 |
Both China and the US are now full steam ahead 01:28:13.540 |
a national security danger or a military danger, 01:28:26.620 |
In some sense, the mission and the work you're doing 01:28:30.180 |
in open AI is like the counterbalance to that. 01:28:48.540 |
actually in Sam's hands because it's extremely hard 01:29:01.420 |
- Even like low level, quote unquote engineer. 01:29:05.340 |
Like there's such a, I remember I programmed a car, 01:29:12.820 |
They went really fast, like 30, 40 miles an hour. 01:29:28.540 |
and it's going around on track, but it's going full speed. 01:29:31.700 |
And there was a bug in the code that the car just went, 01:29:51.300 |
and the programming of artificial intelligence systems 01:30:00.860 |
for some reason I immediately thought of like an algorithm 01:30:03.900 |
that controls nuclear weapons, having the same kind of bug. 01:30:10.020 |
and the CEO of a company all need to have the seriousness 01:30:15.100 |
and thinking about the worst case consequences. 01:30:27.980 |
and fear itself ends up being actually quite debilitating. 01:30:45.820 |
- Like a focus on how I can maximize the value, 01:30:50.820 |
how the systems that I'm building might be useful. 01:30:55.900 |
I'm not saying that the fear doesn't exist out there 01:31:00.060 |
and like it totally makes sense to minimize it, 01:31:03.140 |
but I don't want to be working because I'm scared. 01:31:06.820 |
I want to be working out of passion, out of curiosity, 01:31:10.500 |
out of the looking forward for the positive future. 01:31:26.260 |
- Correct, like the love where I'm considering 01:31:34.620 |
like one to N where N is 7 billion or whatever it is. 01:31:38.180 |
- Not projecting my reward functions on others. 01:31:44.060 |
to something else super cool, which is OpenAI Codex? 01:31:48.500 |
Can you give an overview of what OpenAI Codex 01:32:01.940 |
that system train on all the language out there 01:32:05.260 |
started having some rudimentary coding capabilities. 01:32:08.260 |
So we're able to ask it to implement addition function 01:32:14.260 |
and indeed it can write Python or JavaScript code for that. 01:32:18.100 |
And then we thought we might as well just go full steam ahead 01:32:22.780 |
and try to create a system that is actually good 01:32:30.180 |
We optimize models for proficiency in coding. 01:32:36.940 |
that both have a comprehension of language and code. 01:32:48.540 |
and then I don't know if you can say fine-tuned, 01:32:52.820 |
'cause there's a lot of code, but it's language and code. 01:33:07.900 |
of the potential products that can use coding capabilities, 01:33:14.900 |
Copilot is a first product developed by GitHub. 01:33:20.820 |
we wanted to make sure that these models are useful. 01:33:36.780 |
how to view characters of the code or the line of code. 01:33:41.020 |
The thing about Copilot is it can generate 10 lines of code. 01:33:48.260 |
you often write in the comment what you want to happen, 01:33:50.780 |
because people in comments, they describe what happens next. 01:33:59.380 |
for the appropriate code to solve my problem, 01:34:02.780 |
I say, "Oh, for this array, could you smooth it?" 01:34:07.500 |
And then it imports some appropriate libraries 01:34:16.740 |
- So you write a comment, maybe the header of a function, 01:34:25.540 |
of all the possible small programs it can generate. 01:34:35.900 |
It's hard to know, but the fact that it works at all 01:34:45.260 |
into code that's been written on the internet. 01:34:47.980 |
- Correct, so for instance, when you search things online, 01:34:51.860 |
then usually you get to some particular case. 01:34:57.740 |
people describe that one particular situation, 01:35:03.260 |
But in case of Copilot, it's aware of your entire context, 01:35:07.980 |
and in context is, "Oh, these are the libraries 01:35:10.780 |
That's the set of the variables that is initialized, 01:35:14.380 |
and on the spot, it can actually tell you what to do. 01:35:19.420 |
and we think that Copilot is one possible product 01:35:22.540 |
using Codex, but there is a place for many more. 01:35:25.300 |
So internally, we tried out to create other fun products. 01:35:29.980 |
So it turns out that a lot of tools out there, 01:35:33.340 |
let's say Google Calendar or Microsoft Word or so, 01:35:36.380 |
they all have internal API to build plugins around them. 01:35:47.740 |
Today, if you want more complicated behaviors 01:35:51.100 |
from these programs, you have to add a new button 01:36:12.300 |
So what you figure out is there's a lot of programs 01:36:33.740 |
And it seems that Codex on the fly can pick up the API 01:36:40.420 |
And then it can turn language into use of this API. 01:36:49.060 |
- So for example, this would be really exciting for me, 01:37:01.380 |
- And do you could imagine that that allows even 01:37:21.300 |
make the header large, then move the paragraphs around, 01:37:31.700 |
So if you look actually at the evolution of computers, 01:37:44.860 |
in the plastic card to indicate zeros and ones. 01:37:49.020 |
And during that time, there was a small number 01:37:51.940 |
of specialists who were able to use computers. 01:37:55.940 |
that there is no need for many more people to use computers. 01:38:11.740 |
And they also led to more of a proliferation of technology. 01:38:31.860 |
if someone will tell you that you should write code 01:38:34.740 |
in assembly instead of, let's say, Python or Java or JavaScript. 01:38:41.700 |
toward kind of bringing computers closer to humans, 01:38:55.100 |
an increase of number of people who can code. 01:38:59.660 |
that those people will create is, it's innumerable. 01:39:10.220 |
of having a technical mind, a programming mind. 01:39:16.420 |
other kinds of minds, creative minds, artistic minds, 01:39:22.140 |
- I would like, for instance, biologists who work on DNA 01:39:26.540 |
and not to need to spend a lot of time learning it. 01:39:29.500 |
And I believe that's a good thing to the world. 01:39:47.460 |
So those are kind of, they're overlapping teams. 01:39:52.860 |
and then the Codex is like applied to programming. 01:40:01.540 |
making these models extremely efficient and deployable. 01:40:06.540 |
For instance, there are people who are working to 01:40:16.580 |
or even pushing it at the very limit of the scale. 01:40:25.180 |
- So I'm just saying there are multiple teams. 01:40:27.100 |
While the team working on Codex and language, 01:40:35.180 |
- Yeah, if you're interested in machine learning, 01:40:38.180 |
this is probably one of the most exciting problems 01:40:48.660 |
like generating a program is very interesting, 01:40:51.300 |
very interesting problem that has echoes of reasoning 01:40:58.060 |
And I think there's a lot of fundamental questions 01:41:06.180 |
- Yeah, one more exciting thing about the programs is that, 01:41:09.740 |
so I said that the, you know, in case of language, 01:41:13.300 |
that one of the troubles is even evaluating language. 01:41:23.900 |
Or so in case of program, there is this one extra lever 01:41:30.460 |
So that process might be somewhat more automated 01:41:35.140 |
in order to improve the qualities of generations. 01:41:45.260 |
the simulation to actually execute it, that's a human mind. 01:42:11.620 |
that we'll be able to make a tremendous progress. 01:42:29.340 |
'cause I was gonna ask you about reliability. 01:42:41.460 |
- So I wouldn't start with controlling nuclear power plant. 01:42:54.380 |
destructive thing right away, run by JavaScript. 01:43:02.020 |
it is possible to achieve some levels of reliability 01:43:08.820 |
maybe there are ways for a model to write even code 01:43:14.020 |
And there exist ways to create the feedback loops 01:43:32.540 |
That's the prompt that generates consciousness. 01:43:38.540 |
Do you think the code that generates consciousness 01:43:44.140 |
I mean, ultimately the core idea behind will be simple, 01:43:48.300 |
but there will be also decent amount of engineering involved. 01:44:08.660 |
I believe that first models that I guess are conscious 01:44:17.580 |
- But then again, there's a, which is certain argument 01:44:34.220 |
So sometimes people are eager to put the trick 01:44:37.620 |
while forgetting that there is a cost of maintenance. 01:44:49.620 |
So even if you have something that gives you two X, 01:44:52.100 |
but it requires, you know, 1000 lines of code, 01:44:58.380 |
if it's five lines of code and two X, I would take it. 01:45:10.060 |
lack of attachment to code that we are willing to remove it. 01:45:25.460 |
we knew that actually reinforcement learning works 01:45:27.420 |
and it is possible to solve fairly complicated problems. 01:45:33.660 |
that it is possible to build superhuman Go players. 01:45:50.660 |
Could we train machines to solve arbitrary tasks 01:45:57.580 |
let's pick a complicated problem that if we would solve it, 01:46:01.940 |
that means that we made some significant progress, 01:46:05.420 |
the domain, and then we went after the problem. 01:46:08.300 |
So we noticed that actually the robots out there, 01:46:12.620 |
they are kind of at the moment, optimized per task. 01:46:19.780 |
it's very likely that the end factor is that bottle opener. 01:46:23.740 |
And in some sense, that's a hack to be able to solve a task, 01:46:35.300 |
And we concluded that like human hands have such a quality 01:46:54.580 |
like trying to solve Rubik's cube single-handed. 01:47:12.700 |
it's one robotic hand solving the Rubik's cube. 01:47:16.620 |
The hard part is in the solution to the Rubik's cube 01:47:18.860 |
is the manipulation of the, of like having it not fall 01:47:23.100 |
out of the hand, having it use the five baby arms to, 01:47:28.100 |
what is it, like rotate different parts of the Rubik's cube 01:47:45.300 |
And, you know, one path it is to do reinforcement learning 01:47:54.140 |
In some sense, the tricky part about the real world 01:47:57.340 |
is at the moment, our models, they require a lot of data. 01:48:07.300 |
And in simulation, you can have infinite amount of data. 01:48:10.060 |
The tricky part is the fidelity of the simulation. 01:48:12.980 |
And also can you in simulation represent everything 01:48:16.220 |
that you represent otherwise in the real world. 01:48:22.500 |
because there is lack of fidelity, it is possible to, 01:48:32.260 |
but it actually solves the entire range of simulations, 01:48:39.900 |
exactly the friction of the cube or weight or so. 01:48:49.420 |
- How do you generate the different simulations? 01:48:51.540 |
- So, you know, there's plenty of parameters out there. 01:48:56.020 |
And in simulation model just goes for thousands of years 01:49:01.020 |
and keeps on solving Rubik's cube in each of them. 01:49:03.980 |
And the thing is that neural network that we used, 01:49:07.140 |
it has a memory and as it presses, for instance, 01:49:15.820 |
oh, that's actually this side was difficult to press. 01:49:24.740 |
it's even how to solve this particular instance 01:49:30.100 |
It's kind of like, you know, sometimes when you go to a gym 01:49:34.260 |
and after bench press, you try to lift the glass 01:49:39.260 |
and you kind of forgot and your head goes like, 01:49:48.980 |
to maybe different weight and it takes a second to adjust. 01:50:02.100 |
with the bench press, all the bros in the audience 01:50:09.220 |
So maybe put the bar down and pick up the water bottle 01:50:14.020 |
and you'll know exactly what Czech is talking about. 01:50:31.300 |
when it comes to robots, they require maintenance. 01:50:37.940 |
It's also, it's hard to replay things exactly. 01:50:42.140 |
I remember this situation that one guy at our company, 01:50:53.100 |
And, you know, we kind of didn't know what's going on, 01:51:02.780 |
he was running it from his laptop that had better CPU 01:51:10.460 |
And because of that, there was less of a latency 01:51:15.700 |
And that actually made solving RubySquib more reliable. 01:51:19.660 |
So in some sense, there might be some saddlebacks like that 01:51:22.500 |
when it comes to running things in the real world. 01:51:28.500 |
that the initial models, you would like to have models 01:51:33.620 |
and you would like to give them even more time for thinking. 01:51:46.020 |
And ultimately I would like to build a system 01:51:49.100 |
that it is worth for you to wait five minutes 01:51:55.060 |
that you are willing to wait for five minutes. 01:52:09.980 |
So the data that I'm speaking about would be a data 01:52:23.460 |
it would be very easy to make a progress on robotics. 01:52:26.420 |
And you can see that in case of text or code, 01:52:29.340 |
there is a lot of data, like a first person perspective 01:52:38.620 |
that if you were to build like a successful robotics company 01:52:43.260 |
so OpenAs mission is much bigger than robotics. 01:52:51.420 |
that you wouldn't so quickly dismiss supervised learning. 01:52:55.980 |
- You would build a robot that was perhaps what, 01:53:07.780 |
So you would invest, that's just one way to do it. 01:53:12.780 |
like direct human control of the robots as it's learning. 01:53:28.780 |
recording human trajectories, controlling a robot. 01:53:32.220 |
- After you find a thing that the robot should be doing 01:53:38.540 |
like that you can make a lot of money with that product. 01:53:44.380 |
and then I would essentially train supervised 01:53:50.420 |
Long term, I think that actually what is needed 01:54:03.020 |
And people are looking into models generating videos. 01:54:07.260 |
They're like, "Why are these algorithmic questions 01:54:17.620 |
which would have a level of understanding of video, 01:54:22.020 |
same as GPT has a level of understanding of text, 01:54:25.180 |
could be used to train robots to solve tasks. 01:54:37.540 |
by robotics company I mean the primary source of income 01:54:42.460 |
is from robots that is worth over $1 trillion. 01:54:52.420 |
- It's interesting 'cause my mind went to personal robotics, 01:54:57.860 |
It seems like there's much more market opportunity there. 01:55:04.220 |
I mean, this might speak to something important, 01:55:09.180 |
which is I understand self-driving much better 01:55:17.900 |
To a level, not just the actual computer vision 01:55:24.420 |
but creating a product that would undeniably be, 01:55:35.340 |
that could replace Uber drivers, for example. 01:55:52.500 |
and at the same time, the car itself costs cheaper. 01:55:58.540 |
I think there's a lot more low-hanging fruit in the home. 01:56:11.460 |
or maybe kind of depends on the exact problem 01:56:21.460 |
they cost tens of thousands of dollars or maybe 100K. 01:56:35.820 |
the price would have to go down to maybe a thousand bucks. 01:56:42.100 |
so self-driving car provides a clear service. 01:56:52.140 |
Meaning it will not necessarily be about like a robotic arm 01:56:55.940 |
that's helps you, I don't know, open a bottle 01:56:59.420 |
or wash the dishes or any of that kind of stuff. 01:57:04.100 |
It has to be able to take care of that whole, 01:57:11.020 |
there's a line between what is a robot and what is not. 01:57:16.000 |
But some AI system with some embodiment, I think. 01:57:22.980 |
when you think actually what's the difficult part is 01:57:28.980 |
like when there is a diversity of the environment 01:57:31.260 |
with which the robot has to interact, that becomes hard. 01:57:33.400 |
So on one spectrum, you have industrial robots, 01:57:37.820 |
as they are doing over and over the same thing, 01:57:39.900 |
it is possible to some extent to prescribe the movements 01:57:46.560 |
the movement can be repeated millions of times. 01:57:49.840 |
There are also various pieces of industrial robots 01:57:58.520 |
might be a matter of putting a rug inside of a car. 01:58:05.600 |
it's not that easy, it's not exactly the same every time. 01:58:13.560 |
While welding cars together, it's a very repetitive process. 01:58:25.700 |
of the environment, but still the car itself, 01:58:30.620 |
is you try to avoid even interacting with things. 01:58:45.100 |
if there is a huge variety of things to be touched, 01:58:50.340 |
which there is head that is smiling in some way 01:59:02.900 |
So you're referring to touch like soft robotics, 01:59:13.100 |
just basic interaction between like non-contact interaction 01:59:26.860 |
with self-driving cars and disagreement with Elon, 01:59:36.060 |
You said that in your intuition, touch is not required. 01:59:44.340 |
you're going to have to interact with pedestrians, 01:59:48.080 |
not just avoid pedestrians, but interact with them. 01:59:54.420 |
we're constantly threatening everybody's life 02:00:00.740 |
There's a game they're ready to go on with pedestrians. 02:00:03.660 |
And I'm afraid you can't just formulate autonomous driving 02:00:13.740 |
like a collision avoidance is the first order approximation, 02:00:20.220 |
they are gathering data from people driving their cars. 02:00:23.540 |
And I believe that's an example of supervised learning data 02:00:31.380 |
which can give a model this like another level of behavior 02:00:36.380 |
that is needed to actually interact with the real world. 02:00:45.500 |
What do you think of the whole Tesla autopilot approach, 02:00:49.900 |
the computer vision based approach with multiple cameras, 02:00:54.440 |
it's a multitask, multi-headed neural network, 02:01:09.900 |
and then it runs into trouble in a bunch of places 02:01:13.760 |
So like the deployment discovers a bunch of edge cases 02:01:18.020 |
and those edge cases are sent back for supervised annotation 02:01:25.180 |
it goes over and over until the network becomes really good 02:01:29.140 |
at the task of driving, becomes safer and safer. 02:01:32.320 |
What do you think of that kind of approach to robotics? 02:01:36.820 |
So in some sense, even when I was speaking about, 02:01:44.780 |
and then you have humans revising all the issues. 02:01:53.140 |
because for the cases where there are mistakes, 02:01:59.260 |
- So there's a very, to me, difficult question 02:02:02.020 |
of how hard that, how long that converging takes, 02:02:09.860 |
is probably applies to certain robotics applications 02:02:14.900 |
They put, as the quality of the system converges, 02:02:19.900 |
so one, there's a human factors perspective of psychology 02:02:27.700 |
And the other society willing to accept robots. 02:02:31.280 |
Currently society is much harsher on self-driving cars 02:02:37.820 |
So the bar is set much higher than for humans. 02:02:41.220 |
And so if there's a death in an autonomous vehicle, 02:02:55.260 |
is figuring out how to make robots part of society, 02:03:07.620 |
maybe you can put that into the objective function 02:03:09.620 |
to optimize, but that is definitely a tricky one. 02:03:14.300 |
And I wonder if that is actually the trickiest part 02:03:18.440 |
for self-driving cars or any system that's safety critical. 02:03:29.380 |
I believe that the part of the process of deployment 02:03:59.140 |
So in some sense, people will have to keep on proving 02:04:01.860 |
that indeed the systems are worth being used. 02:04:06.100 |
I also found out that often the best way to convince people 02:04:14.980 |
That's the case with Tesla Autopilot, for example. 02:04:21.620 |
It's kind of funny to hear people talk about robots. 02:04:42.980 |
And if the product is designed well, they fall in love. 02:04:51.300 |
There was a spectrum of responses that people had. 02:04:56.340 |
the important piece was to let people try it out. 02:05:05.900 |
But like some of them, they came with a fear. 02:05:28.140 |
even self-supervised learning in the visual space 02:05:32.660 |
for robotics and then reinforcement learning. 02:05:35.220 |
What do you, in like this whole beautiful spectrum of AI, 02:05:49.900 |
You know, it started with Alan Turing and the Turing test. 02:05:53.300 |
Maybe you think natural language conversation 02:05:57.180 |
- So, you know, it would be nice if, for instance, 02:06:00.020 |
machine would be able to solve Riemann hypothesis in math. 02:06:03.400 |
That would be, I think that would be very impressive. 02:06:22.740 |
I mean, the tricky part about the benchmarks is, 02:06:25.600 |
you know, as we are getting closer with them, 02:06:29.540 |
There is actually no ultimate benchmark out there. 02:06:31.860 |
- Yeah, see, my thought with the Riemann hypothesis 02:06:37.500 |
we would say, okay, well, then the problem was easy. 02:06:43.820 |
that's actually what happens over the years in AI, 02:06:46.740 |
that like, we get used to things very quickly. 02:06:50.300 |
- You know something, I talked to Rodney Brooks, 02:06:57.260 |
'Cause he was saying like, there's nothing special about it. 02:07:00.980 |
And I didn't, well, he's coming from one of the aspects 02:07:08.660 |
which deployed now tens of millions of robot in the home. 02:07:12.060 |
So if you see robots that are actually in the homes of people 02:07:17.060 |
as the legitimate instantiation of artificial intelligence, 02:07:21.900 |
then yes, maybe an AI that plays a silly game 02:07:24.580 |
like Go and chess is not a real accomplishment, 02:07:31.620 |
well, then that game of chess or Go wasn't that difficult 02:07:36.020 |
compared to the thing that's currently unsolved. 02:07:38.380 |
So my intuition is that from perspective of the evolution 02:07:45.820 |
we'll at first see the tremendous progress in digital space. 02:08:07.580 |
and delivering it to actual people is very hard. 02:08:20.500 |
they would go down to, let's say marginal costs are to zero. 02:08:25.260 |
- And also the question is how much of our life 02:08:30.780 |
more and more of our lives being in the digital space. 02:08:41.140 |
if most of your life is spent in virtual reality? 02:08:44.260 |
- I still would like to, at least at the moment, 02:08:47.500 |
my impression is that I would like to have a physical contact 02:08:50.540 |
with other people and that's very important to me. 02:08:53.100 |
We don't have a way to replicate it in the computer. 02:08:55.340 |
It might be the case that over the time it will change. 02:09:00.060 |
why not have like an arbitrary infinite number of people 02:09:06.820 |
with arbitrary characteristics that you can define 02:09:28.620 |
I'm not so sure I would stay with the real world. 02:09:36.060 |
or do you need to plug electrodes in the brain? 02:09:51.140 |
do you think we'll be able to solve the Turing test, 02:09:55.740 |
which is, do you think we'll be able to achieve 02:10:00.260 |
compelling natural language conversation between people? 02:10:02.940 |
Like have friends that are AI systems on the internet. 02:10:17.380 |
And I think that still system should keep on learning 02:10:29.260 |
asking these questions and kind of at first pre-training 02:10:47.340 |
Or can most of the connection be in the digital space? 02:10:52.420 |
We know that there are people who met each other online 02:10:58.820 |
- So it seems that it's conceivable to establish connection 02:11:10.940 |
- So it would be like you're proposing like a Tinder 02:11:18.060 |
and half the systems are AI and the other is humans 02:11:23.380 |
- That would be our formulation of Turing test. 02:11:27.780 |
The moment AI is able to achieve more swipe right or left 02:11:32.260 |
or whatever, the moment it's able to be more attractive 02:11:35.340 |
than other humans, it passes the Turing test. 02:11:38.180 |
- Then you would pass the Turing test in attractiveness. 02:11:41.620 |
Well, no, like attractiveness just to clarify. 02:11:49.300 |
and whatever makes conversations pleasant for humans. 02:12:02.260 |
- In some sense, I would almost ask the question, 02:12:07.740 |
Well, I have this argument with my dad all the time. 02:12:10.860 |
He thinks that touch and smell are really important. 02:12:16.420 |
and I'm saying the initial systems, they won't have it. 02:12:19.140 |
Still, I wouldn't, like there are people being born 02:12:24.260 |
without these senses and I believe that they can still 02:12:32.260 |
- Yeah, I wonder if it's possible to go close 02:12:35.980 |
to all the way by just training on transcripts 02:12:42.340 |
- So I think that actually still you want images. 02:12:50.820 |
it has to see kids drawing some pictures on the paper. 02:12:55.820 |
- And also facial expressions, all that kind of stuff. 02:13:20.140 |
It doesn't have to move, you know, mechanical pieces or so. 02:13:23.540 |
So I think that there is like kind of a progression. 02:13:27.660 |
You can imagine that text might be the simplest to tackle, 02:13:31.820 |
but this is not a complete human experience at all. 02:13:47.300 |
the fact that we can touch each other or smell or so. 02:13:54.260 |
And I believe that these things might happen gradually. 02:14:09.820 |
- Like, would you, do you look forward to a world, 02:14:16.100 |
Do you look forward to a day where one or two 02:14:19.660 |
- So if the system would be truly wishing me well, 02:14:23.500 |
rather than being in the situation that it optimizes 02:14:29.460 |
- The line between those is, it's a gray area. 02:14:34.460 |
- I think that's the distinction between love and possession. 02:14:39.180 |
And these things, they might be often correlated 02:14:43.180 |
for humans, but it's like, you might find that 02:14:47.180 |
there are some friends with whom you haven't spoke 02:15:00.860 |
you know, it's trying to convince me to spend time with it. 02:15:05.380 |
I would like the system to optimize for what I care about 02:15:17.140 |
There's some manipulation, there's some possessiveness, 02:15:19.780 |
there's some insecurities, there's fragility. 02:15:22.220 |
All those things are necessary to form a close friendship 02:15:25.980 |
over time, to go through some dark shit together, 02:15:31.380 |
I feel like there's a lot of greedy self-centered behavior 02:15:42.380 |
doesn't have to go through computer being greedy, 02:15:53.940 |
they are, I guess, prompted or fine-tuned or so, 02:16:08.660 |
we look at the transcript of the conversation 02:16:13.940 |
"Actually, here, there was more loving way to go about it." 02:16:17.740 |
And we supervise system toward being more loving. 02:16:23.820 |
it has a reward function toward being more loving. 02:16:26.180 |
- Yeah, or maybe the possibility of the system 02:16:29.100 |
being an asshole and manipulative and possessive 02:16:32.980 |
every once in a while is a feature, not a bug. 02:16:36.660 |
Because some of the happiness that we experience 02:16:44.980 |
is a kind of break from the assholes in the world. 02:16:52.020 |
because like, it'll be like a breath of fresh air 02:16:54.980 |
to discover an AI that the three previous AIs you had- 02:17:08.220 |
But you need to experience the full spectrum. 02:17:10.460 |
Like, I think you need to be able to engineer assholes. 02:17:15.620 |
- Because there's some level to us being appreciated, 02:17:58.140 |
And apparently there are neurotoxic snails over there. 02:18:04.340 |
And apparently there is a high chance of even just dying, 02:18:10.980 |
At some point, she regained partially consciousness. 02:18:20.500 |
At some point, she started being able to speak, 02:18:32.300 |
she actually noticed that she can move her toe. 02:18:37.300 |
And then she knew that she will be able to walk. 02:18:40.940 |
And then, you know, that's where she was five years after. 02:18:45.420 |
she appreciates the fact that she can move her toe. 02:19:18.580 |
belief that maybe one has to go for a week or six months 02:19:25.780 |
- To just experience, you know, a lot of difficulties. 02:19:29.180 |
And then comes back and actually everything is bright. 02:19:43.620 |
but yeah, there's something about the Russian, 02:19:47.860 |
I believe suffering or rather struggle is necessary. 02:19:55.940 |
you even look at the story of any superhero, the movie. 02:20:00.500 |
It's not that it was like everything goes easy, easy, easy. 02:20:07.860 |
Okay, you mentioned that you used to do research at night 02:20:23.260 |
Like, is there some interesting wild sleeping patterns 02:20:31.540 |
- I tried at some point decreasing number of hours of sleep, 02:20:34.700 |
like gradually, like half an hour every few days to this. 02:20:43.580 |
Like at some point there's like a phase shift 02:20:48.580 |
You know, there was a time that I used to work 02:20:54.060 |
The nice thing about the nights is that no one disturbs you. 02:20:57.780 |
And even I remember when I was meeting for the first time 02:21:02.780 |
with Greg Brockman, he's CTO and chairman of OpenAI. 02:21:15.380 |
Now you sound like me, that's hilarious, okay, yeah. 02:21:20.620 |
my sleeping schedule also has to do with the fact 02:21:33.260 |
the extrovert thing, because most humans operate 02:21:39.300 |
you're forced to then operate at the same set of hours. 02:21:49.220 |
working through the night, because it's quiet, 02:22:02.700 |
because people are having meetings and sending emails 02:22:11.540 |
and they prevent me from having sufficient amount of time 02:22:21.580 |
and I said that I'm out of office Wednesday, Thursday 02:22:25.380 |
and I'm having meetings only Monday and Tuesday. 02:22:28.260 |
And that vastly, positively influenced my mood, 02:22:31.820 |
that I have literally like three days for fully focused work. 02:22:35.260 |
- Yeah, so there's better solutions to this problem 02:22:43.580 |
of some of the greatest ideas in artificial intelligence. 02:22:52.660 |
there are many other brilliant people around. 02:23:10.060 |
you know, kind of simple, it might sound simple, 02:23:16.180 |
So for instance, for a while, people in academia, 02:23:35.460 |
that no one thought about training models on anti-internet. 02:23:40.460 |
Or like that maybe some people thought about it, 02:23:53.540 |
but that's an example of breaking a typical assumption. 02:24:09.620 |
so that was assumption that many people had out there. 02:24:13.380 |
And then if you free yourself from assumptions, 02:24:26.300 |
it's very likely that you're going to have the same results. 02:24:28.940 |
- Yeah, but there's also that kind of tension, 02:24:56.260 |
training your brain towards generating ideas, 02:24:58.660 |
and not even suspending judgment of the ideas. 02:25:06.900 |
that even if I'm in the process of generating ideas, 02:25:18.260 |
because I'm actually focused on the negative part, 02:25:25.260 |
that it's very easy for me to store new ideas. 02:25:28.380 |
So for instance, next to my bed, I have a voice recorder, 02:25:35.420 |
like I wake up during the night, and I have some idea. 02:25:38.540 |
In the past, I was writing them down on my phone, 02:25:44.500 |
and that wakes me up, or like pulling a paper, 02:25:53.820 |
- What do you think, I don't know if you know 02:26:02.820 |
so that he can sleep through it, and solve it in his sleep, 02:26:06.540 |
or like come up with radical stuff in his sleep. 02:26:11.180 |
- So it happened from my experience perspective, 02:26:16.180 |
it happened to me many times during the high school days 02:26:22.340 |
that I had the solution to my problem as I woke up. 02:26:31.580 |
I'm trying to actually devote substantial amount of time 02:26:38.980 |
I can organizing amount of the huge chunks of time, 02:26:42.220 |
such that I'm not constantly working on the urgent problems, 02:26:45.580 |
but I actually have time to think about the important one. 02:26:49.940 |
But his idea is that you kind of prime your brain 02:26:56.260 |
Oftentimes people have other worries in their life 02:27:06.980 |
He wants to kind of pick the most important problem 02:27:10.940 |
that you're thinking about, and go to bed on that. 02:27:15.460 |
the other thing that comes to my mind is also, 02:27:24.300 |
rather than just being pulled by urgent things 02:27:30.860 |
'cause I've been doing the voice recorder thing too, 02:27:52.860 |
Some of it is, this has to do with OpenAI Codex too. 02:28:08.420 |
of the resulting transcripts and all that kind of stuff. 02:28:23.220 |
and there's no good mechanism for recording thoughts. 02:28:32.740 |
Maybe it has like Audible or let's say Kindle. 02:28:47.380 |
It has also Google Maps if I need to go somewhere. 02:28:49.980 |
And I also use this phone to write down ideas. 02:28:58.620 |
is even sending a message from that phone to the other phone. 02:29:02.500 |
So that's actually my way of recording messages 02:29:07.820 |
What advice would you give to a young person, 02:29:11.540 |
high school, college, about how to be successful? 02:29:16.540 |
You've done a lot of incredible things in the past decade. 02:29:25.340 |
- I mean, it might sound like simplistic or so, 02:29:30.260 |
but I would say literally just follow your passion, 02:29:43.460 |
When I was in elementary school was math and chemistry. 02:29:47.980 |
And I remember for some time I gave up on math 02:29:51.140 |
because my school teacher, she told me that I'm dumb. 02:29:54.940 |
And I guess maybe an advice would be just ignore people 02:30:03.180 |
You mentioned something offline about chemistry 02:30:13.700 |
I got into chemistry, maybe I was like a second grade 02:30:31.100 |
And I did all the experiments that they describe 02:30:34.140 |
in the book, like how to create oxygen with vinegar 02:30:48.380 |
And explosives, they also, it's like you have a clear 02:31:03.140 |
That was kind of funny experiment from school. 02:31:09.820 |
So that's also relatively easy to synthesize. 02:31:18.900 |
I remember there was a, there was at first like maybe 02:31:21.980 |
two attempts that I went with a friend to detonate 02:31:27.100 |
And like a third time he was like, ah, it won't work. 02:31:36.860 |
you know, that tube with dynamite, I don't know, 02:31:44.620 |
We're like riding on the bike to the edges of the city. 02:32:02.220 |
It actually had the, you know, electrical detonator. 02:32:10.620 |
I even, I never, I haven't ever seen like a explosion before. 02:32:14.980 |
So I thought that there will be a lot of sound. 02:32:22.540 |
At some point, you know, we kind of like a three to one 02:32:42.140 |
let's make sure the next time we have helmets. 02:32:45.820 |
But also, you know, I'm happy that nothing happened to me. 02:32:49.140 |
It could have been the case that I lost the limbo or so. 02:32:52.420 |
- Yeah, but that's childhood of an engineering mind 02:33:14.860 |
It's a worrying quality of people that work in chemistry 02:33:28.020 |
It's almost like a reminder that physics works, 02:33:38.140 |
That's why I really like artificial intelligence, 02:33:40.540 |
especially robotics, is you create a little piece of nature. 02:33:45.540 |
- And in some sense, even for me with explosives, 02:33:48.900 |
the motivation was creation rather than destruction. 02:33:55.940 |
about just machine learning and deep learning. 02:34:02.300 |
how would you recommend they get into the field? 02:34:11.460 |
- So on different levels of abstraction in some sense, 02:34:14.020 |
but I would say re-implement something from scratch, 02:34:22.540 |
I would say that's a powerful way to understand things. 02:34:24.940 |
So it's often the case that you read the description 02:34:37.420 |
- Is there a particular topics that you find people 02:34:43.420 |
I tend to really enjoy reinforcement learning 02:34:54.060 |
where you feel like you created something special, 02:35:00.820 |
As opposed to like re-implementing from scratch, 02:35:06.340 |
more like supervised learning kind of things. 02:35:08.700 |
- So if someone would optimize for things to be rewarding, 02:35:15.020 |
then it feels that the things that are somewhat generative, 02:35:19.500 |
So you have, for instance, adversarial networks, 02:35:22.380 |
or you have just even generative language models. 02:35:28.060 |
internally we have seen this thing with our releases. 02:35:35.100 |
There is one model called DALI that generates images, 02:35:40.460 |
that actually you provide various possibilities, 02:35:44.980 |
what could be the answer to what is on the picture, 02:35:47.500 |
and it can tell you which one is the most likely. 02:35:50.740 |
And in some sense, in case of the first one, DALI, 02:36:27.100 |
or it's not that easy for you to understand the strength. 02:36:31.340 |
- Even though you don't believe in magic, to see the magic. 02:36:39.780 |
'cause then you are at the core of the creation. 02:36:43.060 |
You get to experience creation without much effort, 02:36:51.980 |
There is some level of reward for creating stuff. 02:36:54.860 |
Of course, different people have a different weight 02:37:01.860 |
- In the big objective function, of a person. 02:37:11.940 |
Even a cockroach is beautiful if you look very closely. 02:37:35.100 |
that you have really increased focus, increased attention. 02:37:45.300 |
You can look at the table or on the pen or at the nature. 02:37:55.860 |
And once again, it kind of reminds me of my childhood. 02:38:04.900 |
It's also, I have seen even the reverse effect 02:38:08.380 |
that by default, regardless of what we possess, 02:38:30.340 |
I find that material possessions get in the way 02:38:46.460 |
Just like you're saying, just like, I don't know, 02:38:52.300 |
like this cup, like thing, you know, just objects. 02:39:10.460 |
And then two, I can't believe humans are clever enough 02:39:15.980 |
The hierarchy of pleasure that that provides is infinite. 02:39:20.580 |
- I mean, even if you look at the cup of water, 02:39:22.620 |
so you see first like a level of like a reflection of light, 02:39:26.460 |
but then you think, no man, there's like trillions 02:39:29.220 |
upon trillions of particles bouncing against each other. 02:39:39.980 |
and move around, and you think it also has this 02:39:42.700 |
like a magical property that as you decrease temperature, 02:39:46.380 |
it actually expands in volume, which allows for the, 02:39:53.460 |
and at the bottom to have actually not freeze, 02:40:01.900 |
and you think actually, you know, this table, 02:40:04.420 |
that was just the figment of someone's imagination 02:40:07.540 |
And then there was like a thousands of people involved 02:40:14.700 |
- And then you can start thinking about evolution, 02:40:18.340 |
how it all started from single cell organisms 02:40:22.540 |
- And these thoughts, they give me life appreciation. 02:40:26.860 |
- And even lack of thoughts, just the pure raw signal 02:40:31.740 |
- See, the thing is, and then that's coupled for me 02:40:42.860 |
the fact that this experience, this moment ends, 02:40:51.460 |
So in that same way, I try to meditate on my own death often. 02:41:03.220 |
- So fear of death is like one of the most fundamental fears 02:41:16.100 |
There is still, let's say, this property of nature 02:41:31.780 |
I also found out that it seems to be very healing 02:42:15.020 |
I have this discussion with Ilya from time to time. 02:42:23.620 |
"At some point, I will be 40, 50, 60, 70, and then it's over." 02:42:46.380 |
I also like this framework of thinking from Jeff Bezos 02:42:52.980 |
that I would like, if I will be at that deathbed, 02:43:00.620 |
and not regret that I haven't done something. 02:43:03.420 |
It's usually, you might regret that you haven't tried. 02:43:19.820 |
that would be the, you'd be okay with that kind of life. 02:43:36.780 |
I'm extremely grateful for actually people whom I met. 02:43:40.740 |
I would say, I think that I'm decently smart and so on, 02:43:49.620 |
where I am has to do with the people who I met. 02:43:54.500 |
- Would you be okay if after this conversation you died? 02:44:03.740 |
So in some sense, there's like a plenty of things 02:44:13.660 |
I think that the list will be always infinite. 02:44:19.020 |
- Yeah, I mean, to be clear, I'm not looking forward to die. 02:44:23.860 |
I would say if there is no choice, I would accept it. 02:44:27.500 |
But like in some sense, if there would be a choice, 02:44:35.500 |
- I find it's more honest and real to think about 02:44:56.100 |
Then I'm much more about appreciating the cup and the table 02:44:59.380 |
and so on, and less about silly worldly accomplishments 02:45:05.260 |
- We have in the company a person who say at some point 02:45:11.980 |
And that also gives huge perspective with respect 02:45:21.940 |
- And love, and people conclude also if you have kids, 02:45:31.900 |
"We don't assign the minus infinity reward to our death. 02:45:36.220 |
Such a reward would prevent us from taking any risk. 02:45:43.340 |
So in the objective function, you mentioned fear of death 02:45:53.500 |
And the interesting thing is even realization 02:46:00.260 |
how different reward functions can play with your behavior. 02:46:04.980 |
As a matter of fact, I wouldn't say that you should assign 02:46:20.620 |
you said they assign $9 million to human life. 02:46:30.860 |
but in some sense that there is a finite value 02:46:34.180 |
I'm trying to put it from perspective of being less, 02:46:40.820 |
of being more egoless and realizing fragility 02:47:00.020 |
if you were to put death in the objective function, 02:47:02.580 |
there's probably so many aspects to death and fear of death 02:47:14.300 |
of not just your life, but every experience and so on 02:47:18.020 |
that you're gonna have to formalize mathematically. 02:48:03.860 |
I suspected that they will ask this question, 02:48:05.580 |
but it's also a question that I ask myself many, many times. 02:48:09.380 |
See, I can tell you a framework that I have these days 02:48:13.340 |
So I think that fundamentally meaning of life 02:48:22.700 |
let's say, for instance, curiosity or human connection, 02:48:49.700 |
- I think when you are born, there is some randomness. 02:48:51.660 |
Like you can see that some people, for instance, 02:48:57.900 |
Some people care more about caring for others. 02:49:00.940 |
Some people, there are all sorts of default reward functions. 02:49:05.020 |
And then in some sense, you can ask yourself, 02:49:27.700 |
in mathematics, you are likely to run into various objects, 02:49:35.820 |
And these are very natural objects that arise. 02:49:43.780 |
that there is a reward function for understanding, 02:49:53.500 |
So in some sense, they are in the same way natural 02:50:05.780 |
You're saying reward functions are discovered. 02:50:10.700 |
you can still, let's say, expand it throughout the life. 02:50:13.020 |
Some of the reward functions, they might be futile. 02:50:15.540 |
Like for instance, there might be a reward function, 02:50:21.260 |
- And this is more like a learned reward function. 02:50:27.620 |
if you optimize them, you won't be quite satisfied. 02:50:30.860 |
- Well, I don't know which part of your reward function 02:50:46.460 |
and it's an honor to meet you and an honor to talk to you. 02:51:02.220 |
please check out our sponsors in the description. 02:51:09.700 |
who is the author of "2001, A Space Odyssey." 02:51:20.140 |
Thank you for listening, and I hope to see you next time.