back to indexThe AI opportunity: Sequoia Capital's AI Ascent 2024 opening remarks
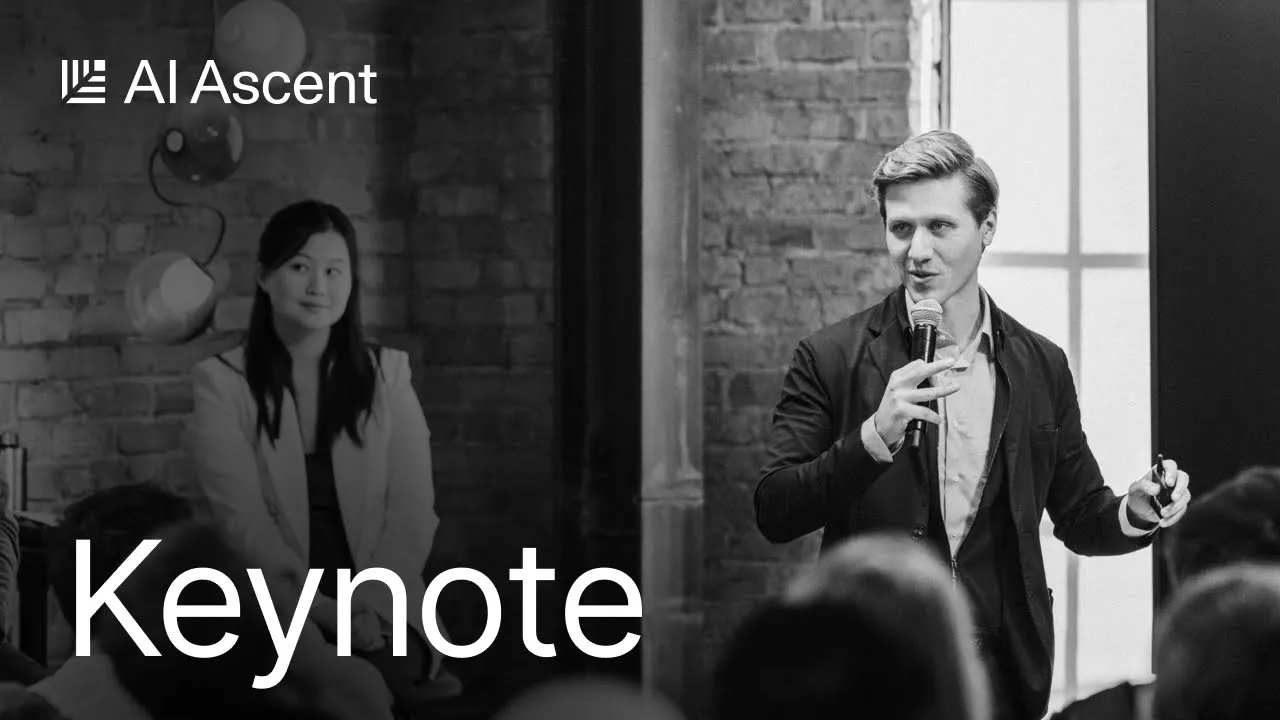
00:00:25.680 |
We have an objective to meet a few people who can be 00:00:30.000 |
today and hopefully, we'll have a little bit of fun. 00:00:32.400 |
So just to frame the opportunity, what is it? 00:00:44.740 |
we've been through this contracted form of the hype cycle. 00:00:51.320 |
we're crawling back out into the plateau of productivity. 00:01:00.240 |
be woven into a wide variety of magical applications. 00:01:11.240 |
Not something software has been able to do before. 00:01:17.520 |
could be one-shot, could be multi-step agentic type reasoning. 00:01:20.800 |
But again, not something software has been able to do before. 00:01:28.100 |
we've got the right brain and the left brain covered, 00:01:30.740 |
which means that software can also for the first time, 00:01:41.620 |
that we're going to mention on the next slide. 00:01:49.940 |
In this case, the best analogy that we can come up with, 00:02:00.700 |
the technology landscape that led to new business models, 00:02:03.100 |
new applications, new ways for people to interact with technology. 00:02:06.940 |
If we go back to some of the early days of that Cloud transition, 00:02:12.260 |
the entire pie, the entire global TAM for software is about 350 billion, 00:02:25.580 |
but that slice has become 400 billion of revenue. 00:02:41.740 |
Because of what I mentioned about the ability to 00:02:46.580 |
one of the big opportunities for AI is to replace services with software. 00:02:54.380 |
the starting point is not hundreds of billions, 00:02:56.420 |
the starting point is possibly tens of trillions. 00:03:00.220 |
So you can really dream about what this has a chance to become. 00:03:10.280 |
we would posit that we are standing at the precipice of 00:03:12.860 |
the single greatest value creation opportunity mankind has ever known. 00:03:16.900 |
Why now? One of the benefits of being part of Sequoia is that we have 00:03:26.100 |
the different waves of technology and understand how they 00:03:28.620 |
interact and understand how they lead us to the present moment. 00:03:31.300 |
We're going to take a quick trip down memory lane. 00:03:37.580 |
was actually the guy who ran the go-to-market for Fairchild Semiconductor, 00:03:40.980 |
which gave Silicon Valley its name with Silicon-based transistors. 00:03:45.500 |
We got to see the 1970s when systems were built on top of those chips. 00:03:49.940 |
We got to see the 1980s when they were connected up by 00:03:53.300 |
networks with PCs as the endpoint and the advent of package software. 00:03:57.620 |
We got to see the 1990s when those networks went public-facing in the form of the Internet, 00:04:04.700 |
We got to see the 2000s when the Internet matured to 00:04:07.820 |
the point where it could support sophisticated applications, 00:04:12.260 |
We got to see the 2010s where all those apps showed up in 00:04:15.500 |
our pocket in the form of mobile devices and changed the way we work. 00:04:19.700 |
So why do we bother going through this little build? 00:04:23.220 |
Well, the point here is that each one of these waves is 00:04:32.860 |
I think neural nets first became an idea in the 1940s. 00:04:36.180 |
But the ingredients required to take AI from idea, 00:04:43.780 |
into reality, to actually solve real-world problems in 00:04:47.620 |
a unique and compelling way that you can build a durable business around. 00:04:52.020 |
The ingredients required to do that did not exist until the past couple of years. 00:04:56.500 |
We finally have compute that is cheap and plentiful. 00:04:59.980 |
We have networks that are fast and efficient and reliable. 00:05:05.100 |
the planet have a supercomputer in their pockets. 00:05:12.900 |
all of these delightful experiences is readily available. 00:05:19.380 |
become the theme of the next 10, probably 20 years. 00:05:23.900 |
So we have as strong conviction as you could possibly 00:05:30.620 |
that the next couple of decades are going to be the time of AI. 00:05:40.020 |
the Cloud transition and the mobile transition. 00:05:44.380 |
those are most of the companies born as a result of 00:05:46.860 |
those transitions that got to a billion dollars plus of revenue. 00:05:51.140 |
but this is probably 80 percent or so of the companies 00:05:54.140 |
formed in those transitions that got to a billion plus of revenue, 00:05:59.540 |
The most interesting thing about this slide is the right side. 00:06:03.100 |
It's not what's there, it's what isn't there. 00:06:14.820 |
We think if we were standing here 10 or 15 years from today, 00:06:19.660 |
that right side is going to have 40 or 50 logos in it. 00:06:25.620 |
the logos of companies that are in this room. 00:06:46.980 |
I think it's been a whirlwind for everybody here. 00:06:49.340 |
It probably feels like just about all of us have been going 00:06:51.860 |
non-stop with the ground shifting under our feet constantly. 00:06:59.580 |
Last year, we were talking about how AI was going to 00:07:06.020 |
A year later, it's starting to come into focus. 00:07:09.220 |
Who here has seen this tweet from Sebastian at Klarna? 00:07:18.380 |
handle two-thirds of customer service inquiries. 00:07:20.980 |
They've automated the equivalent of 700 full-time agents jobs. 00:07:25.460 |
We think there are tens of millions of call center agents 00:07:31.900 |
product market fit is in this customer support markets. 00:07:38.340 |
the law was considered one of the least tech forward industries, 00:07:43.980 |
Now, we have companies like Harvey that are automating away 00:07:46.740 |
a lot of the work that lawyers do from day-to-day grunt work and 00:07:50.140 |
drudgery all the way to more advanced analysis. 00:07:53.340 |
Or software engineering. I'm sure a bunch of people in 00:07:56.900 |
the demos floating around on Twitter recently. 00:07:59.660 |
It's remarkable that we've gone from a year ago, 00:08:05.420 |
entirely self-contained AI software engineers. 00:08:18.060 |
Now, the other day, I was in a Zoom with Pat, 00:08:20.580 |
and I noticed that he looked a little bit suspicious, 00:08:29.500 |
his virtual AI avatar and was actually hitting the gym, 00:08:34.260 |
>> Hi, this is Pat Grady. This is definitely me. 00:08:37.020 |
I'm definitely here and not at the gym right now. 00:08:48.620 |
This is how far technology has come in a year. 00:08:55.100 |
to think about how this all plays out in the coming decade. 00:09:01.500 |
when we thought that Generative AI might usher 00:09:15.060 |
revenue momentum has surprised just about everybody. 00:09:20.260 |
is now clocking in around $3 billion of revenues in aggregate, 00:09:23.780 |
and that's before you count all the incremental revenue 00:09:26.180 |
generated by the FANG companies and the Cloud providers in AI. 00:09:36.020 |
Generative AI got there its first year out the gate. 00:09:44.740 |
The customer pull in AI isn't restricted to one or two apps. 00:09:49.580 |
It's everywhere. I'm sure everyone's aware of 00:09:54.020 |
But when you look at the revenue and the usage numbers, 00:09:58.060 |
both consumer companies and enterprise companies, 00:10:02.940 |
many AI products are actually striking a chord with 00:10:09.140 |
We find the diversity of use cases that are starting to 00:10:24.740 |
a layer cake where you have foundation models on the bottom, 00:10:37.060 |
the new enabling technology in the foundation layer. 00:10:55.780 |
and posed a thought-provoking question last year with 00:11:05.300 |
that companies are pouring into GPUs right now, 00:11:08.300 |
we spent about $50 billion on NVIDIA GPUs just last year. 00:11:12.940 |
Everybody's assuming if you build it, they will come. 00:11:22.060 |
AI revenue plus change from the Cloud providers. 00:11:26.940 |
plus energy, plus data center costs and more. 00:11:31.220 |
To me, that means the math isn't mathing yet. 00:11:36.540 |
this stuff has vastly exceeded the amount of money 00:11:40.140 |
So we've got some real problems to fix still. 00:11:46.780 |
even though the revenue and the user numbers in AI 00:11:52.420 |
And so if you look at, for example, the ratio of daily 00:11:54.820 |
to monthly active users, or if you look at one month 00:12:03.580 |
To me, that is both a problem and an opportunity. 00:12:08.540 |
is a once a week, once a month kind of tinkery phenomenon 00:12:14.260 |
But we have the opportunity to use AI to create apps 00:12:16.620 |
that people want to use every single day of their lives. 00:12:20.180 |
When we interview users, one of the biggest reasons 00:12:22.780 |
they don't stick on AI apps is the gap between expectations 00:12:27.780 |
So that magical Twitter demo becomes a disappointment 00:12:30.940 |
when you see that the model just isn't smart enough 00:12:33.080 |
to reliably do the thing that you asked it to do. 00:12:40.520 |
have smarter and smarter base models to build on. 00:12:47.760 |
And so as the level of intelligence of the baseline 00:12:49.920 |
rises, we should expect AI's product market fit 00:12:53.320 |
So unlike in some markets where the future of the market 00:12:57.840 |
is you can draw a very clear line to how those apps will 00:13:05.480 |
We said this at last year's AI Ascent, and we'll say it again. 00:13:08.600 |
If you look at the iPhone, some of the first apps in the V1 00:13:12.800 |
of the App Store were the beer drinking app or the lightsaber 00:13:34.120 |
It wasn't until 2010 that you saw Instagram and DoorDash 2013. 00:13:39.360 |
So it took time for companies to discover and harness 00:13:42.720 |
the net new capabilities of the iPhone in creative ways 00:13:47.080 |
We think the same thing is playing out in AI. 00:13:52.520 |
into what some of those next legendary companies might be. 00:13:55.840 |
Here are a few of the ones that have captured our attention 00:13:58.880 |
recently, but I think it's much broader than the set of use 00:14:05.060 |
is one of the first handful of use cases that's really hitting 00:14:14.220 |
AI Friendship has been one of the most surprising 00:14:18.340 |
I think it took a few months of thinking for us 00:14:22.980 |
But I think the user and the usage metrics in this category 00:14:32.620 |
We'll hear more from Glean and Dusk later today. 00:14:35.620 |
We think that enterprise knowledge is finally 00:14:48.900 |
from co-pilots that are kind of helpers on the side 00:14:54.180 |
to agents that can actually take the human out 00:14:58.180 |
AI that feels more like a co-worker than a tool. 00:15:01.140 |
We're seeing this start to work in domains like software 00:15:12.540 |
Prediction number two, one of the biggest knocks against LLMs 00:15:17.420 |
the statistical patterns in text and aren't actually 00:15:20.180 |
taking the time to reason and plan through the tasks 00:15:23.460 |
That's starting to change with a lot of new research, 00:15:26.300 |
like inference time compute and gameplay-style value 00:15:30.140 |
What happens when you give the model the time 00:15:36.940 |
thrust for many of the foundation model companies. 00:15:41.540 |
more capable of higher-level cognitive tasks like planning 00:15:52.820 |
Prediction number three, we are seeing an evolution 00:15:58.860 |
where you don't really care if the AI says something 00:16:02.180 |
wrong or crazy occasionally, to real enterprise applications, 00:16:09.900 |
The good thing is that there's different tools 00:16:11.860 |
and techniques emerging to help bring these LLMs sometimes 00:16:17.060 |
from RLHF to prompt training to vector databases. 00:16:19.660 |
And I'm sure that's something that you guys can 00:16:31.060 |
expect to see a lot of AI prototypes and experiments 00:16:43.300 |
And it means we expect the balance of compute 00:16:55.780 |
And it's really important that we get it right. 00:16:58.980 |
With that, I'll transition to Konstantin, who 00:17:00.940 |
will help us dream about AI over an even longer time horizon. 00:17:10.380 |
And thank you, everyone, for being here today. 00:17:17.700 |
And Sonia just walked us through the "what now?" 00:17:22.260 |
This section is going to be about what's next. 00:17:34.900 |
So there are many types of technology revolution. 00:17:38.380 |
There are communication revolutions, like telephony. 00:17:52.900 |
We believe that AI is primarily a productivity revolution. 00:18:04.020 |
That transitions into a human with a machine assistant. 00:18:07.860 |
And eventually, that moves into a human with a machine network. 00:18:12.460 |
The two predictions that we're going to talk about 00:18:21.300 |
The sickle has been around as a tool for the human 00:18:26.300 |
The mechanical reaper, which is a human and a machine assistant, 00:18:29.340 |
was invented in 1831, a single machine system 00:18:35.860 |
Today, we live in an era where we have a combine harvester. 00:18:42.540 |
of machine systems working together as a complex network. 00:18:48.740 |
We're starting to use language in AI to describe this. 00:18:51.460 |
Language like individual machine participants in the system 00:19:04.060 |
In essence, we're creating very complicated layers 00:19:12.660 |
two examples that we're experiencing right in front 00:19:18.300 |
So software started off as a very manual process. 00:19:21.140 |
Here's Ada Lovelace, who wrote logical programming 00:19:24.580 |
with pen and paper, was able to do these computations, 00:19:31.820 |
we have significant machine assistants for computation, 00:19:35.780 |
not just the computer, but the integrated development 00:19:38.020 |
environment, and increasingly more and more technologies 00:19:53.420 |
that are working together in order to produce 00:19:57.740 |
And what you would see here is agents working together 00:20:07.460 |
Writing was a human process, human and a tool. 00:20:09.780 |
Over time, this has progressed to human and a machine 00:20:14.780 |
leveraging not one, but a network of assistants. 00:20:22.060 |
I'm not just calling GPT-4, I'm calling Mistral-Large, 00:20:26.140 |
I'm having them work together and also against each other 00:20:30.620 |
This is the future that we're seeing right in front of us. 00:20:36.140 |
mean for everyone in this room, and frankly, everyone 00:20:40.100 |
In cold, hard economic terms, what this means 00:20:49.540 |
needed at an S&P 500 company to generate 1 million of revenue. 00:20:54.860 |
We're entering an era where this will continue to decline. 00:21:01.020 |
The good news is it's not so that we can do less. 00:21:04.460 |
And we'll get to that in the next set of predictions. 00:21:13.100 |
I'll call out computer software and accessories. 00:21:16.820 |
because we're constantly building on each other, 00:21:22.420 |
But some of the most important things to our society-- 00:21:27.060 |
education, college tuition, medical care, housing-- 00:21:37.900 |
to help drive down costs in these and many other crucial 00:21:42.380 |
So that's the first conclusion about the long-term future 00:21:46.340 |
As a massive cost driver, a productivity revolution 00:21:56.340 |
The second is related to, what is it really doing? 00:21:59.940 |
One year ago on the stage, we had Jensen Huang 00:22:10.800 |
Any given image, even information, will be generated. 00:22:18.340 |
historically, images have been stored as rote memory. 00:22:21.980 |
So let's think about the letter A, ASCII character number 97. 00:22:33.900 |
Well, we're entering a period in which we already 00:22:36.340 |
are representing concepts, like the letter A, 00:22:39.300 |
not as rote storage, not as a presence or absence of pixels, 00:22:47.100 |
is the concept of an A which is generalizable to any given 00:22:50.260 |
format for that letter A. So many different typefaces 00:23:05.100 |
to take it and render it and generate that image, 00:23:11.380 |
going to be able to contextualize that understanding. 00:23:15.660 |
be able to render it, understand it's an alphabet, 00:23:19.660 |
and understand what that means in the broader context 00:23:23.340 |
Computer is going to look at the word multidimensional 00:23:26.780 |
understand the full context of why that's being brought up. 00:23:34.180 |
No longer are we going to be storing the rote pixels 00:23:41.180 |
as the presence or absence of a pixel on a page. 00:23:44.780 |
Instead, we're going to be thinking about that as a concept. 00:23:47.540 |
Powerfully, this is how we've thought about it 00:23:51.180 |
Here's my fellow Greek Plato 2,500 years ago, 00:23:56.380 |
is what we all ascribe to, are all striving for, 00:23:59.100 |
that you have this concept, in this case of a letter A, 00:24:03.340 |
that we actually are able to build a model around. 00:24:07.020 |
Now, we've talked about the second pattern, this idea 00:24:09.180 |
that we're going to have generalization inside computing 00:24:13.180 |
Well, it's going to mean a lot for company building. 00:24:25.860 |
They know that they have certain KPIs that they can drive 00:24:28.140 |
towards, and they can have a system that's actually 00:24:30.300 |
delivering information, generating great customer 00:24:33.860 |
Tomorrow-- and this is already happening alongside-- 00:24:39.300 |
for how the support is actually being communicated. 00:24:42.380 |
And this is what I'm personally incredibly excited about, 00:24:45.000 |
is because of this future in which concepts are rendered, 00:24:47.860 |
because of this future in which everything is generated, 00:24:53.740 |
Let me break that down in a specific example. 00:25:03.220 |
This is a caricature of the customer support process. 00:25:06.500 |
You have customer service that has certain KPIs. 00:25:10.820 |
language generation, customer personalization, and the like. 00:25:21.180 |
You're actually going to have feedback from the language 00:25:23.560 |
generation to the end KPI for the servicing of the customers. 00:25:29.300 |
a layer of abstraction, where customer support is managed, 00:25:31.960 |
optimized, and improved by the neural network. 00:25:36.760 |
another part of the important job of building a business. 00:25:41.540 |
of artificial intelligence, from language generation 00:25:43.740 |
to a growth engine to add customization and optimization. 00:25:46.820 |
This will all feed into each other, once again. 00:25:53.660 |
interoperable to the point where the entire company 00:26:00.260 |
Here comes the rise of the one-person company. 00:26:04.920 |
The one-person company is going to enable us not to do less, 00:26:19.520 |
You are the ones who are building this future. 00:26:22.100 |
We personally are very excited about the future, 00:26:26.300 |
to help drive down costs and increase productivity 00:26:28.780 |
in some of the most crucial areas in our society-- 00:26:36.260 |
And that's the purpose of convening this group today. 00:26:44.300 |
and actually build something that's much more 00:26:48.120 |
I'll hand it off to Sonia to introduce our first speaker.