back to indexAI Semiconductor Landscape feat. Dylan Patel | BG2 w/ Bill Gurley & Brad Gerstner
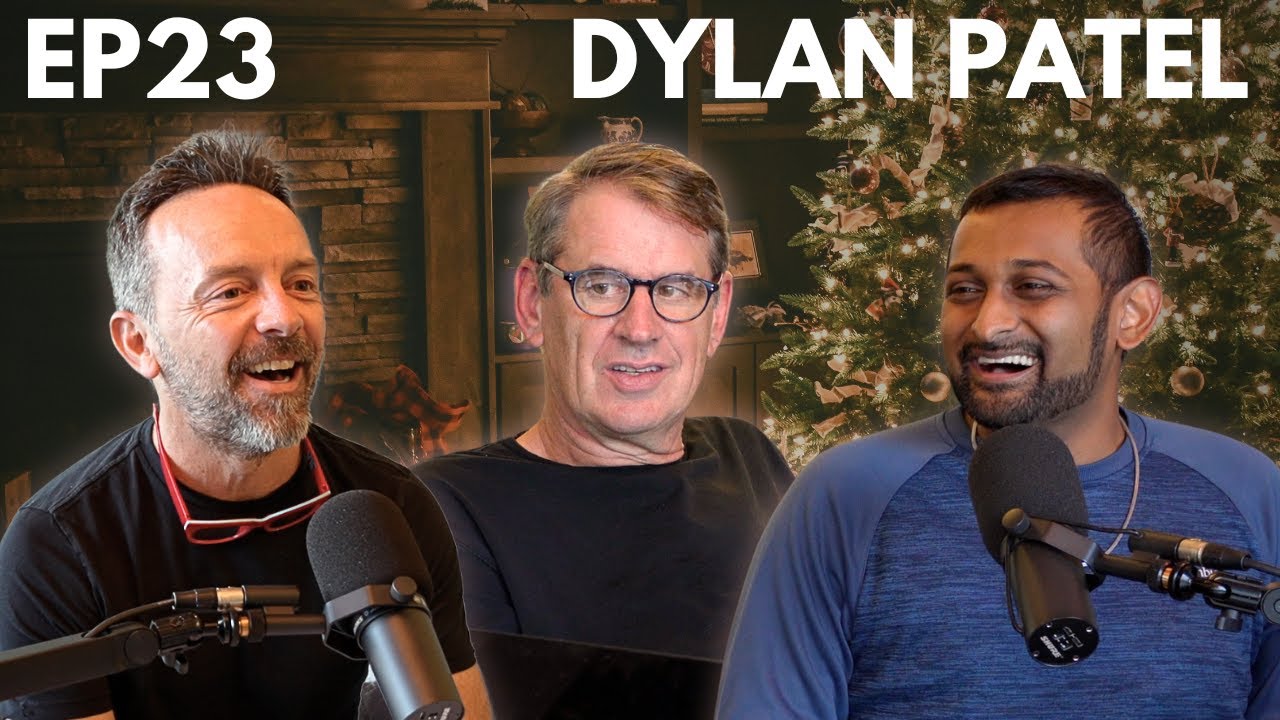
Chapters
0:0 Intro
1:50 Dylan Patel Backstory
2:36 SemiAnalysis Backstory
4:18 Google's AI Workload
6:58 NVIDIA's Edge
10:59 NVIDIA's Incremental Differentiation
13:12 Potential Vulnerabilities for NVIDIA
17:18 The Shift to GPUs: What It Means for Data Centers
22:29 AI Pre-training Scaling Challenges
29:43 If Pretraining Is Dead, Why Bigger Clusters?
34:0 Synthetic Data Generation
36:26 Hyperscaler CapEx
38:12 Pre-training and Inference-tIme Reasoning
41:0 Cisco Comparison to NVIDIA
44:11 Inference-time Compute
53:18 The Future of AI Models and Market Dynamics
60:58 Evolving Memory Technology
66:46 Chip Competition
67:18 AMD
70:35 Google’s TPU
74:56 Cerebras and Grok
77:33 Predictions for 2025 and 2026
00:00:01.900 |
building a two gigawatt data center in Louisiana? 00:00:04.140 |
Why is Amazon building these multi-gigawatt data centers? 00:00:10.820 |
plus buying billions and billions of dollars of fiber 00:00:17.860 |
so let me just connect all the data centers together 00:00:20.580 |
so then I can make them act like one data center, right? 00:00:24.380 |
So this whole, like, is scaling over narrative 00:00:30.060 |
what the people who know the best are spending on. 00:00:51.920 |
how the world of compute is radically changing. 00:00:59.300 |
- Yeah, we're thrilled to have Dylan Patel with us 00:01:17.900 |
from a technical perspective about the architectures 00:01:22.540 |
about the key players in the market globally, 00:01:25.220 |
the supply chain, and the best and the brightest 00:01:32.160 |
And then connect it to some of the business issues 00:01:38.820 |
What I was hoping to do is kind of get a moment in time 00:01:57.860 |
so I didn't have much to do besides be a nerd, 00:02:03.000 |
I had to open it up, short the temperature sensor, 00:02:11.220 |
You know, you see those people in the comments 00:02:17.140 |
As a child, and you didn't know I was a child then, 00:02:26.420 |
I was reading earnings from semiconductor companies, 00:02:28.220 |
and investing in them, you know, with my internship money, 00:02:30.900 |
and yeah, reading technical stuff as well, of course, 00:02:34.740 |
and then working a little bit, and then, yeah. 00:02:36.620 |
- And just tell us, give us a quick thumbnail 00:02:41.420 |
- Yeah, so today we are a semiconductor research firm, 00:02:52.660 |
and we sell data around where every data center 00:02:55.300 |
in the world is, what the power is in each quarter, 00:03:04.740 |
but like, you know, all 1,500 fabs around the world. 00:03:14.740 |
We try and track all of this on a very number-driven basis, 00:03:19.300 |
And then we do consulting around those areas. 00:03:27.100 |
our team talks with Dylan and Dylan's team all the time. 00:03:31.620 |
He's quickly emerged, really just through hustle, hard work, 00:03:40.260 |
for what's going on in the semiconductor industry. 00:03:44.500 |
we're two years into this, maybe, you know, this build-out, 00:03:49.700 |
And one of the things Bill and I are talking about 00:03:51.740 |
is we enter the end of 2024 taking a deep breath, 00:04:02.220 |
And it's gonna have consequence for trillions of dollars 00:04:05.300 |
of value in the public markets, in the private markets, 00:04:17.260 |
- Well, so I think if you're gonna talk about AI 00:04:19.740 |
and semiconductors, there's only one place to start, 00:04:25.420 |
Dylan, what percentage of global AI workloads 00:04:31.460 |
- So I would say if you ignored Google, it'd be over 98%. 00:04:37.500 |
it's actually more like 70, 'cause Google is really 00:04:46.180 |
- Production, you mean in-house workloads for Google? 00:04:48.820 |
- Production as in things that are making money. 00:04:51.020 |
Things that are making money, they're actually probably, 00:04:54.420 |
'Cause you think about Google Search and Google Ads 00:04:58.380 |
two of the largest AI-driven businesses in the world, right? 00:05:02.020 |
You know, the only things that are even comparable 00:05:06.780 |
- And those Google workloads, I think it's important 00:05:09.260 |
just to kind of frame this, those are running 00:05:20.460 |
- So Google's production workloads for non-LLM and LLM 00:05:27.940 |
And I think one of the interesting things is, yes, 00:05:30.260 |
you know, everyone will say Google dropped the ball 00:05:35.860 |
And not Google, but Google was running transformers 00:05:39.060 |
even in their search workload since 2018, 2019. 00:05:42.820 |
The advent of BERT, which was one of the most well-known, 00:05:46.580 |
most popular transformers before we got to the GPT madness, 00:05:50.100 |
has been in their production search workloads for years. 00:06:03.860 |
If you just look at, I guess, workloads people 00:06:09.660 |
So you take the captives out, you're at 98, right? 00:06:12.900 |
This is a dominant landslide at this moment in time. 00:06:18.700 |
They also are one of the big customers of Nvidia. 00:06:28.300 |
So not everything internal is like, is a GPU, right? 00:06:33.300 |
They do buy some for some other internal workloads, 00:06:35.780 |
but by and large, their GPU purchases are for Google Cloud 00:06:42.220 |
Because they are, while they do have some customers 00:06:45.300 |
for their internal silicon externally, such as Apple, 00:06:49.460 |
the vast majority of their external rental business for AI 00:07:03.140 |
- So I like to think of it as like a three-headed dragon, 00:07:06.940 |
I would say every semiconductor company in the world 00:07:14.500 |
People don't realize that Nvidia is actually just much better 00:07:18.580 |
They get to the newest technologies first and fastest 00:07:23.540 |
towards hitting certain production goals, targets. 00:07:31.540 |
And then the networking side of things, right? 00:07:33.700 |
They bought Mellanox and they've driven really hard 00:07:42.020 |
that no other semiconductor company can do alone. 00:07:47.180 |
where you helped everyone visualize the complexity 00:07:50.660 |
of one of these modern cutting edge Nvidia deployments 00:07:54.660 |
that involves the racks, the memory, the networking, 00:08:05.380 |
between companies that are truly standalone chip companies. 00:08:09.900 |
They're not infrastructure companies and Nvidia. 00:08:13.100 |
But I think one of the things that's deeply underappreciated 00:08:15.900 |
is the level of competitive moats that Nvidia has. 00:08:19.580 |
You know, software is becoming a bigger and bigger component 00:08:25.660 |
total cost of operation out of these infrastructures. 00:08:29.020 |
So talk to us a little bit about that schema, you know, 00:08:33.860 |
like there are many different layers of systems architecture 00:08:37.340 |
and how that's differentiated from maybe, you know, 00:08:43.220 |
- Right, so when you look broadly at the GPU, right, 00:08:46.900 |
no one buys one chip for running an AI workload, right? 00:08:53.700 |
You look at, you know, today's leading edge models 00:09:00.900 |
A trillion parameters is over a terabyte of memory. 00:09:07.180 |
A chip can't have enough performance to serve that model, 00:09:11.340 |
So therefore you must tie together many chips together. 00:09:14.780 |
And so what's interesting is that Nvidia has seen that 00:09:19.020 |
and built an architecture that has many chips networked 00:09:24.100 |
But funnily enough and the thing that many ignore 00:09:27.060 |
is that Google actually did this alongside Broadcom, 00:09:30.660 |
you know, and they did it before Nvidia, right? 00:09:33.020 |
You know, today everyone's freaking out about, 00:09:41.980 |
It's not one server, it's not one chip, it's a rack. 00:09:48.180 |
and all these things that Jensen will probably tell you, 00:09:52.220 |
Interestingly, Google did something very similar in 2018, 00:09:59.860 |
They know what the compute element needs to be, 00:10:02.980 |
They can't do a lot of the other difficult things 00:10:16.340 |
they actually were able to build this system, 00:10:18.980 |
this system architecture that was optimized for AI, right? 00:10:26.580 |
I'm sure they could have tried to scale up bigger, 00:10:30.140 |
didn't require scaling to that degree, right? 00:10:42.140 |
to help them get into the system design, right? 00:10:46.900 |
but building many chips that connect together, 00:10:49.260 |
cooling them appropriately, networking them together, 00:10:55.660 |
that semiconductor companies don't have the engineers for. 00:10:59.140 |
- Where would you say NVIDIA has been investing the most 00:11:08.860 |
NVIDIA has primarily focused on supply chain things, 00:11:15.380 |
"Oh, well like, yeah, they're just like ordering stuff." 00:11:18.020 |
You have to work deeply with the supply chain 00:11:33.900 |
Jensen is probably the most paranoid man in the world, right? 00:11:41.340 |
all of his biggest customers were building AI chips, right? 00:11:43.900 |
Before the LLM craze, his main competitors were like, 00:11:50.340 |
because he's bringing to market technologies at volume 00:12:02.480 |
Whether it be in all sorts of other power delivery, 00:12:05.940 |
all these things, he's bringing to market technologies 00:12:17.740 |
And NVIDIA is trying to do this on an annual cadence now. 00:12:21.740 |
- Blackwell, Blackwell Ultra, Rubin, Rubin Ultra, 00:12:28.520 |
"Oh, no, there are some delays in Blackwell." 00:12:37.580 |
is the fact that they're now on this annual cadence, right? 00:12:43.100 |
it almost precludes their competitors from catching up, 00:12:46.740 |
because even if you skate to where Blackwell is, right, 00:12:49.580 |
you're already on next generation within 12 months. 00:12:52.540 |
He's already planning two or three generations ahead 00:12:57.560 |
- Well, the funny thing is a lot of people at NVIDIA 00:12:59.700 |
will say Jensen doesn't plan more than a year, 00:13:05.160 |
and they'll deploy them out that fast, right? 00:13:07.460 |
Every other semiconductor company takes years to deploy, 00:13:16.940 |
like what would be their area of vulnerability 00:13:24.580 |
for other alternatives to take more share of the workload? 00:13:29.420 |
- Yeah, so the main thing for NVIDIA is, you know, 00:13:35.100 |
It's well over a hundred billion dollars of spend 00:13:46.260 |
how to run my model on other hardware, right? 00:13:50.820 |
how to run it for inference on other hardware. 00:14:02.540 |
It means capital costs and it means operation costs 00:14:11.460 |
if they stand still, their performance TCO doesn't grow. 00:14:16.020 |
Like with Blackwell, not only is it way, way, way faster, 00:14:22.740 |
because they've optimized it for very large language models, 00:14:26.920 |
"Hey, we're going to cut our margin too somewhat 00:14:28.940 |
because I'm competing with Amazon's, you know, 00:14:38.140 |
they've decided that they need to push performance TCO, 00:14:45.620 |
They've decided they need to push performance TCO 5X, 00:14:56.020 |
for performance TCO is an insane pace, right? 00:15:01.300 |
"Hey, AI models are actually getting a lot better 00:15:14.160 |
I think when you said the software is more important 00:15:16.720 |
for training, you meant CUDA is more of a differentiator 00:15:22.680 |
- So, I think a lot of people in the investor community, 00:15:25.120 |
you know, call CUDA, which is just like one layer 00:15:32.720 |
regarding networking or what runs on switches 00:15:35.300 |
or what runs on, you know, all sorts of things, 00:15:43.020 |
But all of this software is stupendously difficult 00:15:48.020 |
In fact, no one else has deployments to do that 00:15:57.860 |
So, when you talk about, "Hey, what is the difficulty here?" 00:16:01.460 |
On training, this is users constantly experimenting, right? 00:16:12.160 |
I rely on NVIDIA's performance to be quite good 00:16:15.160 |
with existing software stacks or very little effort, right? 00:16:28.200 |
plus whatever they have on copilot and all that. 00:16:31.380 |
- Yeah, so they have $10 billion of revenue here 00:16:44.100 |
So, it's like they're deploying very few models 00:16:46.620 |
and those change, what, every six months, right? 00:16:55.140 |
And so, Microsoft has deployed GPT-style models 00:17:04.840 |
And so, they can wring that out with software 00:17:06.920 |
because they can spend hundreds of engineers, 00:17:12.520 |
or thousands of engineer hours on working this out 00:17:14.640 |
because it's such a unified sort of workload, right? 00:17:20.440 |
This is a chart we showed earlier in the year 00:17:23.320 |
that I think was kind of a moment for me with Jensen 00:17:32.680 |
not only are we gonna have a trillion dollars 00:17:34.840 |
of new AI workloads over the course of the next four years, 00:17:38.880 |
he said, but we're also going to have a trillion dollars 00:17:42.200 |
of CPU replacement, of data center replacement workloads 00:17:49.960 |
And I, you know, we referenced it on the pod with him 00:17:56.800 |
That he still believes that it's not just about, 00:18:00.400 |
because there's a lot of fuss in the world about, 00:18:03.400 |
you know, pre-training and what if pre-training 00:18:07.360 |
And it seemed to suggest that there was a lot of AI workloads 00:18:15.000 |
but also that they had all of this data center replacement. 00:18:23.960 |
rebuild a CPU data center with a bunch of NVIDIA GPUs. 00:18:29.440 |
But his argument is that an increasing number 00:18:32.920 |
of these applications, even things like Excel 00:18:35.360 |
and PowerPoint are becoming machine learning applications 00:18:43.880 |
for accelerators for a very long time, right? 00:18:51.800 |
all these Siemens engineering applications, right? 00:19:11.880 |
So, you know, yes, no one in the bay uses mainframes 00:19:14.640 |
or talks about mainframes, but they're still growing, right? 00:19:18.960 |
And so, like, I would say the same applies to CPUs, right? 00:19:26.280 |
doesn't mean web serving is like gonna slow down 00:19:29.560 |
Now, what does happen is that line is like this 00:19:40.080 |
hey, these applications, they're now AI, right? 00:19:42.040 |
You know, Excel with Copilot or Word with Copilot, 00:19:46.520 |
they're still gonna have all of those classic operations. 00:19:48.320 |
You don't get rid of what you used to have, right? 00:19:52.560 |
They just run AI analytics on top of their flights 00:19:54.440 |
to maybe, you know, do pricing better or whatever, right? 00:20:03.480 |
Which is given how much people are deploying, 00:20:06.520 |
how tight the supply chains for data centers are. 00:20:11.360 |
they're longer time supply chains, unfortunately, right? 00:20:14.360 |
Which is why you see things like what Elon's doing. 00:20:27.120 |
put GPUs in them like they're doing in Texas. 00:20:30.160 |
Or you can do what some of these other folks are doing, 00:20:32.860 |
which is, hey, well, my depreciation for CPU servers 00:20:41.360 |
Because Intel's progress has been this, right? 00:20:46.120 |
But all of a sudden over the last couple of years, 00:20:57.100 |
the plurality of Amazon CPUs in their data centers 00:21:17.720 |
And well, if I just replace like six servers with one, 00:21:21.600 |
I've basically invented power out of thin air, right? 00:21:25.680 |
because these old servers, which are six plus years old, 00:21:28.520 |
or even, you know, they can just be deprecated and put. 00:21:37.960 |
I can throw another AI server in there, right? 00:21:39.760 |
So this is sort of the, yes, there is some replacement. 00:21:44.160 |
but that total capacity can be served by fewer machines, 00:21:49.560 |
And generally the market is not gonna shrink, 00:21:51.160 |
it's still gonna grow, just nowhere close to what AI is. 00:21:58.320 |
- Okay, Bill, this reminds me of a point Satya made 00:22:03.400 |
a bunch of times, and I think is fairly misunderstood. 00:22:08.720 |
that he was power and data center constrained, 00:22:16.560 |
on the real bottleneck, which is data centers and power, 00:22:20.480 |
as opposed to GPUs, because GPUs have come online. 00:22:29.920 |
- Well, before we dive into the alternatives to NVIDIA, 00:22:33.400 |
I thought we would hit on this pre-training scaling debate 00:22:38.400 |
that you wrote about in your last piece, Dylan, 00:22:55.440 |
and then it got repeated and cross-analyzed quite a bit. 00:23:02.000 |
I think Ilya said, data's the fossil fuel of AI, 00:23:11.840 |
And so the huge gains we got from pre-training 00:23:31.240 |
- So pre-training scaling laws are pretty simple, right? 00:23:34.760 |
You get more compute, and then I throw it at a model, 00:23:42.280 |
The bigger the model, the more data, the better. 00:23:44.320 |
And there's actually an optimal ratio, right? 00:23:46.120 |
So Google published a paper called Chinchilla, 00:23:48.840 |
which says the optimal ratio of data to parameter, 00:24:06.320 |
We have barely, barely, barely tapped video data, right? 00:24:10.800 |
So there is a significant amount of data that's not tapped. 00:24:13.000 |
It's just video data is so much more information 00:24:24.960 |
But more importantly, text is the most efficient domain, 00:24:29.040 |
Humans generally, yes, a picture paints a thousand words, 00:24:33.920 |
I can probably, you can tell, figure out faster, right? 00:24:35.760 |
- And the transcripts of most of those videos were already. 00:24:38.760 |
- Yeah, the transcripts of many of those videos 00:24:47.040 |
Now, the problem is this is only pre-training, right? 00:24:51.920 |
Training a model is more than just the pre-training, right? 00:25:03.240 |
and recursively be like, oh, that's not right. 00:25:14.920 |
And then they come back and bring something to you. 00:25:46.720 |
What are, you know, all these companies focused on? 00:25:53.040 |
who's like sort of one of the big reasoning people 00:25:54.880 |
on roadshows, just going and speaking everywhere, 00:25:59.880 |
They're saying, hey, we can still improve these models. 00:26:02.480 |
Yes, spending compute at inference time is important, 00:26:19.720 |
ask many people what's the square root of 81, 00:26:25.680 |
like almost, you know, a lot more people, right? 00:26:28.840 |
But you say, hey, let's have the existing model do that. 00:26:38.200 |
and then anytime it's unsure branch into multiple. 00:26:41.440 |
you have hundreds of quote unquote rollouts or trajectories 00:26:49.640 |
hey, only these paths got to the right answer. 00:26:52.080 |
Okay, now I feed that and that is now new training data. 00:27:02.560 |
Hey, this unit test that I have in my code base, 00:27:17.320 |
you throw away the vast, vast majority of it, 00:27:24.160 |
which then it will learn how to do that more effectively, 00:27:34.640 |
is kind of not proven yet, by the way, right? 00:27:52.800 |
but when Sam wants to go from 3 billion to 30 billion, 00:27:56.320 |
it's a little difficult to raise that money, right? 00:28:04.520 |
And so the question is, well, that's just one axis. 00:28:11.720 |
We've spent tens of millions of dollars, maybe, 00:28:22.560 |
it also had a qualifier like that in certain domains. 00:28:33.600 |
- Yeah, I think one of the interesting things about AI 00:28:41.720 |
with the release of text models, people were like, 00:28:43.040 |
oh, wow, artists are the one that are the most, 00:28:47.840 |
Actually, these things suck at technical jobs. 00:28:55.520 |
actually, where are the areas where we can teach the model? 00:29:03.640 |
We can teach it to write really good software. 00:29:06.360 |
We can teach it how to do mathematical proofs. 00:29:11.080 |
because there are, while there are trade-offs, 00:29:13.400 |
and this is not like, it's not just a one-zero thing, 00:29:17.440 |
this is something you can functionally verify. 00:29:35.320 |
'cause you could traverse it and run synthetically. 00:29:39.280 |
You could just let it create and create and create. 00:29:42.000 |
- Putting on my investor hat, public investor hat here, 00:30:03.240 |
then do people really need to buy bigger clusters? 00:30:09.480 |
that, no, the 90% benefit of pre-training is gone. 00:30:13.520 |
But then I look at the comments out of Hock Tan this week, 00:30:27.560 |
that they're gonna build 200 or 300,000 GPU clusters, 00:30:31.960 |
Meta reportedly building much bigger clusters, 00:30:39.440 |
If everybody's right and pre-training's dead, 00:30:42.080 |
then why is everybody building much bigger clusters? 00:30:49.240 |
What's the, how do we continue to grow, right? 00:30:59.720 |
And then there's also the access if it's a log chart, right? 00:31:01.720 |
You need 10X more to get the next job, right? 00:31:15.240 |
- So the point is I'm still gonna squeak out enough gain 00:31:20.840 |
particularly when you look at the competitive dynamic, 00:31:24.480 |
you know, our models versus our competitor models. 00:31:32.040 |
even if, you know, the kind of big one-time gain 00:31:38.560 |
it's logarithmically more expensive to do that gain. 00:31:43.120 |
but like the sort of whole, like Orion has failed 00:31:50.280 |
They released O1, which is sort of a different axis. 00:31:53.360 |
It's partially because, hey, this is, you know, 00:31:57.040 |
but it's partially because they did not scale 10X, right? 00:31:59.920 |
'Cause scaling 10X from four to this is actually was like- 00:32:05.520 |
- Well, I would also, let's go to Gavin a second. 00:32:07.760 |
One of the reasons this became controversial, I think, 00:32:22.800 |
made it sound like they were just gonna build 00:32:25.400 |
the next biggest thing and get the same amount of gain. 00:32:31.320 |
And so we get to this place, as you described it, 00:32:35.320 |
And then people go, "Oh, what does that mean?" 00:32:38.600 |
- I think they have never said the chinchilla scaling laws 00:32:56.680 |
you're spending a ton of compute at train time, right? 00:33:07.880 |
Because there's this new axis of synthetic data generation 00:33:11.960 |
and the amount of compute we can throw at it is, 00:33:14.640 |
we're still right here in the scaling law, right? 00:33:24.600 |
We've only spent millions, tens of millions of dollars, 00:33:31.720 |
And then there's, of course, test time compute as well, 00:33:33.560 |
i.e. spending time at inference to get better and better. 00:33:37.440 |
And in fact, many people at these labs believe 00:33:40.800 |
or the next six months of gains will be faster 00:33:49.160 |
Because this requires stupendous amounts of compute. 00:33:51.880 |
You're generating so much more data than exist on the web, 00:33:57.920 |
that you have to run the model constantly, right? 00:34:00.760 |
- What domains do you think are most applicable 00:34:06.840 |
Like where were synthetic data be most effective? 00:34:16.200 |
like a scenario where it's gonna be really good 00:34:20.240 |
- Yeah, so I think that goes back to the point around 00:34:23.280 |
what can we functionally verify is true or not? 00:34:34.920 |
Or you're like, "Dang, I messed that up," right? 00:34:37.400 |
- There's like a determinism of grading the output. 00:34:43.080 |
So if it can be functionally verified, amazing. 00:34:47.720 |
So there's sort of two ways to judge an output, right? 00:35:01.880 |
But now, humans don't scale for this level of data, right? 00:35:11.360 |
- So these are domains where, hey, in Google, 00:35:14.840 |
when they push data to any of their services, 00:35:19.360 |
These unit tests make sure everything's working. 00:35:25.160 |
and then use those unit tests to grade those outputs, right? 00:35:30.040 |
And then you can also grade these outputs in other ways. 00:35:34.960 |
there's other areas such as like, hey, image generation. 00:35:39.160 |
which image looks more beautiful to you versus me. 00:35:55.840 |
so where do we have objective grading, right? 00:36:04.400 |
engineering is not just, this is the best solution. 00:36:11.040 |
That's usually what engineering ends up being. 00:36:13.080 |
Well, I can still look at all these axes, right? 00:36:19.560 |
Like, hey, what's the best way to write this email 00:36:22.000 |
or what's the best way to negotiate with this person? 00:36:26.920 |
- What are you hearing from the hyperscalers? 00:36:45.080 |
So when we track every data center in the world 00:36:46.960 |
and it's insane how much, especially Microsoft 00:36:51.120 |
and now Meta and Amazon and, you know, and many others, 00:36:54.800 |
right, but those guys specifically are spending 00:37:00.920 |
if you look at all of the different regulatory filings 00:37:03.840 |
and use satellite imagery, all these things that we do, 00:37:12.760 |
- What are you going to fill in there, right? 00:37:14.480 |
It turns out you have to fill, to fill it up, you know, 00:37:17.080 |
you can make some estimates around how much power 00:37:21.240 |
Satya said he's going to slow down that a little bit, 00:37:22.960 |
but they've signed deals for next year rentals, right? 00:37:27.040 |
- And it's part of the reason he said is he expects 00:37:29.440 |
his cloud revenue in the first half of next year 00:37:31.160 |
to accelerate, because he said we're going to have 00:37:36.000 |
So, you know, what they're, you know, like again, 00:37:49.480 |
plus buying billions and billions of dollars of fiber 00:37:56.520 |
So let me just connect all the data centers together 00:37:59.240 |
So then I can make them act like one data center, right? 00:38:08.080 |
when you see what the people who know the best 00:38:28.040 |
but they might have thought they were gonna go 00:38:44.240 |
- So when you think about training a neural network, right, 00:38:47.160 |
it is doing a forwards pass and a backwards pass, right? 00:38:49.560 |
Forwards pass is generating the data, basically, 00:38:52.240 |
and it's half as much compute as the backwards pass, 00:39:00.640 |
grading the outputs, and then training the model, 00:39:03.400 |
you are going to do many, many, many forward passes 00:39:09.600 |
So it turns out that there is a lot of inference 00:39:18.480 |
because you have to generate hundreds of possibilities 00:39:21.800 |
and then, oh, you only train on a couple of them, right? 00:39:27.560 |
The other paradigm I would say that is very relevant 00:39:40.560 |
- The answer is, depends on what you're doing. 00:39:54.760 |
And why are they building multiple similar-sized 00:39:57.920 |
data centers in Wisconsin and Atlanta and Texas 00:40:03.920 |
Because they already see the research is there 00:40:06.400 |
for being able to split the workload more appropriately, 00:40:08.920 |
which is, hey, this data center, it's not serving users. 00:40:20.320 |
while they're also updating the model in other areas. 00:40:27.640 |
It's just, it's logarithmically more expensive 00:40:30.480 |
for each generation, for each incremental improvement. 00:40:33.840 |
- But there's other ways to not just continue this, 00:40:36.840 |
but hey, I don't need a logarithmic increase in spend 00:40:42.440 |
In fact, through this reasoning, training, and inference, 00:40:46.560 |
I can get that logarithmic improvement in the model 00:40:57.080 |
- I mean, the, you know, the thing that I think 00:40:59.840 |
so interesting, you know, I hear Kramer on CNBC 00:41:02.440 |
this morning, you know, and they're talking about, 00:41:06.840 |
I was in Omaha, Bill, Sunday night for dinner. 00:41:10.200 |
You know, they're obviously big investors and utilities 00:41:18.400 |
So I had my team pull up a chart for Cisco, you know, 2000, 00:41:24.120 |
But, you know, they peaked at like 120 PE, right? 00:41:31.760 |
that occurred in revenue and in EBITDA, you know, 00:41:39.000 |
So the price to earnings multiple went from 120 00:41:43.480 |
And so I said to, you know, in this dinner conversation, 00:41:47.120 |
I said, well, NVIDIA's, you know, PE today is 30. 00:41:53.400 |
So you would have to think that there would be 70% PE 00:42:05.280 |
we all have post-traumatic stress about that. 00:42:07.200 |
I mean, hell, you know, I lived through that too. 00:42:15.640 |
It's not to say that there can't be a pullback, 00:42:21.120 |
given what you told us about scaling laws continuing, 00:42:23.960 |
you know, what do you think when you hear, you know, 00:42:27.520 |
the Cisco comparison when people are talking about NVIDIA? 00:42:30.840 |
- Yeah, so I think there's a couple of things 00:42:41.040 |
into building out telecom infrastructure, right? 00:42:53.520 |
But CoreWeave is just backstopped by Microsoft. 00:42:55.360 |
There is significant amounts of, like, difference 00:42:57.480 |
in, like, what is the source of the capital, right? 00:42:59.680 |
The other thing is, at the peak of the dot-com, 00:43:02.160 |
you know, especially once you inflation-adjust it, 00:43:11.720 |
As much as people say the venture markets are going crazy, 00:43:16.400 |
all these companies, and we were just talking about this 00:43:18.560 |
before the show, but, like, hey, the venture markets, 00:43:21.960 |
the private markets, have not even tapped in, right? 00:43:25.120 |
Private market money, like in the Middle East, 00:43:27.080 |
in these sovereign wealth funds, it's not coming in yet. 00:43:37.800 |
the difference of capital, the source is positive cash flows 00:43:41.000 |
of the most profitable companies that have ever lived 00:43:55.760 |
- I think corporate America is investing more in AI 00:44:04.800 |
You've mentioned inference time reasoning a few times now. 00:44:08.560 |
It's clearly a new vector of scaling intelligence. 00:44:20.120 |
Than simply pre-train, you know, scaling pre-training. 00:44:26.680 |
about why that's the case that we'll post as well. 00:44:32.320 |
just kind of what inference time reasoning is 00:44:42.360 |
that if this is in fact going to continue to scale 00:44:50.240 |
it looks like it's gonna be even more compute intensive 00:44:57.560 |
but there's these other aspects of synthetic data generation 00:45:17.840 |
GPT-4 was trained with hundreds of billions of dollars 00:45:21.280 |
and it's generating billions of dollars of revenue. 00:45:25.600 |
- Hundreds of millions of dollars to train GPT-4. 00:45:27.720 |
And it's generating billions of dollars of revenue. 00:45:29.800 |
So when you say like, "Hey, Microsoft's CapEx is nuts." 00:45:32.560 |
Sure, but their spend on GPT-4 was very reasonable 00:45:37.320 |
relative to the ROI they're getting out of it, right? 00:45:39.880 |
Now, when you say, "Hey, I want the next gain." 00:45:42.440 |
If I just spend sort of a large amount of capital 00:45:48.760 |
But if I don't have to spend that large amount of capital 00:45:56.560 |
rather than ahead of time when I'm training the model, 00:46:00.360 |
But this comes with this big trade-off, right? 00:46:09.320 |
And then the answer is only a portion of that, right? 00:46:11.400 |
Today, when you open up chat GPT, use GPT-4, 4.0, 00:46:17.440 |
You send something, you get a response, whatever it is, right? 00:46:23.640 |
Now you're having this reasoning phase, right? 00:46:33.000 |
which are not quite as good as OpenAI, of course, 00:46:34.480 |
but they show you what that reasoning looks like 00:46:53.280 |
I didn't have to spend any more on R&D or capital, right? 00:47:05.320 |
they don't have this R&D ahead of time, right? 00:47:13.400 |
one simple thing that we've done a lot of tests on is, 00:47:21.880 |
I describe the function and a few hundred words. 00:47:24.660 |
I get back a response that's a thousand words. 00:47:30.560 |
When I do this with O1 or any other reasoning model, 00:47:37.500 |
I'm getting the same response, roughly a thousand tokens. 00:47:40.060 |
But in the middle, there was 10,000 tokens of it thinking. 00:47:43.180 |
Now, what does that 10,000 tokens of thinking actually mean? 00:47:59.820 |
depending on how you count the OpenAI profit share. 00:48:03.040 |
You know, anywhere from 50 to 70% gross margins. 00:48:32.720 |
despite it being the same model architecture as GPD 4.0, 00:48:37.080 |
it actually costs significantly more per token as well. 00:48:41.040 |
sort of this chart that we're looking at here, right? 00:48:43.860 |
- And this chart shows, hey, what is GPD 4.0, right? 00:48:46.300 |
If I'm generating, you know, call it a thousand tokens, 00:48:48.680 |
right, and that's what GPD 4.0 on the bottom right is, 00:49:03.240 |
i.e. the number of tokens they're getting at the speed, 00:49:07.400 |
it generates the unit, it generates the code, 00:49:10.040 |
whatever it is, I can group together many users' requests. 00:49:14.640 |
I can group together over 256 users' requests 00:49:18.160 |
on one server for LLAMA 405B of NVIDIA server, right? 00:49:26.080 |
because it's doing that thinking phase of 10,000, right, 00:49:28.920 |
this is basically the whole context length thing. 00:49:36.280 |
the attention mechanism, i.e. it spends a lot of memory 00:49:42.660 |
Now the maximum batch size, i.e. concurrent users I can have 00:49:46.880 |
is a fraction of that, one-fourth to one-fifth 00:49:50.800 |
the number of users can currently use the server. 00:49:52.960 |
So not only do I need to generate 10X as many tokens, 00:49:55.860 |
each token that's generated is four to five X less users. 00:50:17.240 |
- I know it's a 10X 'cause it was on the original O1 release 00:50:24.840 |
again, to service the same number of customers, 00:50:30.560 |
- Well, there's good news and bad news here, Brad, 00:50:47.360 |
unless they can pass it on to the end consumer 00:50:51.520 |
And the thing is you can pass it on to the end consumer 00:51:10.280 |
Remember, they're also paying for 10X as many tokens, right? 00:51:20.560 |
it can pass certain benchmarks like SWEbench, right? 00:51:40.800 |
but they're nothing close to the human, right? 00:51:42.840 |
And so each level of productivity gain I get, 00:51:47.320 |
is a whole new class of tasks that it can do, right? 00:51:54.600 |
yes, I spend a lot more to get the same output, 00:51:57.200 |
but you're not getting the same output with this model. 00:52:02.560 |
end-demand enterprise-level demand for the O1 model? 00:52:07.760 |
- So I would say the O1 style model is so early days, 00:52:12.920 |
O1 is like, they just crack the code and they're doing it, 00:52:16.720 |
Right now on, you know, some of the anonymous benchmarks, 00:52:24.120 |
get to, like, compete, sort of, and people vote on them. 00:52:27.360 |
There's a Google model that is doing reasoning right now, 00:52:31.920 |
but it's going to be released soon enough, right? 00:52:34.240 |
Anthropic is going to release a reasoning model. 00:52:39.880 |
on reasoning right now in terms of training time. 00:52:42.320 |
And they see a very clear path to spending a lot more, 00:52:50.760 |
two to three logarithms in scaling like that, 00:52:55.720 |
You know, I can go from $10 million to $100 billion 00:52:57.840 |
to $10 billion on reasoning in such a quick succession. 00:53:03.760 |
we'll get out of these models is humongous, right? 00:53:06.720 |
In the coming, you know, six months to a year 00:53:09.320 |
in certain benchmarks where you have functional verifiers. 00:53:17.660 |
But if you go back, we've used this internet wave 00:53:30.900 |
And five years later, they weren't on Oracle or Sun. 00:53:34.140 |
And some have argued it went from a development sandbox 00:54:21.100 |
- So you think those are market-clearing prices? 00:54:41.620 |
I can sort of run this model on a single chip. 00:54:44.100 |
This is a very easy, I won't say very easy problem, 00:54:46.340 |
it's still hard, but it's quite a bit easier problem 00:54:56.420 |
there's literally 15 different companies out there 00:55:02.780 |
on Lama, and Alibaba, and DeepSeek, and Mistral, 00:55:08.220 |
and, you know, Fireworks, and all these others. 00:55:12.740 |
all the companies that aren't using their own hardware. 00:55:15.780 |
are doing their own hardware and doing this as well. 00:55:17.540 |
But the market, the margins here are bad, right? 00:55:27.540 |
which was like very revolutionary, sort of late last year, 00:55:41.100 |
What am I, as an API provider, providing you, 00:55:43.900 |
like, why don't you switch from mine to his, why? 00:55:46.220 |
Because, well, there's no, it's pretty fungible, right? 00:55:48.820 |
I'm still getting the same tokens on the same model. 00:55:50.580 |
And so, the margins for these guys is much lower. 00:55:52.820 |
So, Microsoft's earning 50 to 70% gross margins 00:55:55.860 |
on OpenAI models, and that's with the profit share 00:55:58.060 |
they get to get, or the share that they give OpenAI, right? 00:56:07.900 |
You step down to here, no one uses this model from, 00:56:12.580 |
you know, a lot less people use it from OpenAI or Anthropic, 00:56:15.260 |
because they can just, like, take the weights from Llama, 00:56:21.060 |
Go to one of the many competitive API providers, 00:56:24.900 |
some of them, you know, and losing money, right? 00:56:28.780 |
So, not only are you saying, I'm taking a step back, 00:56:31.300 |
and it's an easier problem, and so, therefore, 00:56:37.220 |
On top of that, I'm removing that gross margin. 00:56:47.140 |
No, but, like, there is a huge chase to, like, 00:56:56.620 |
or you're no one if you're one of the labs, right? 00:56:58.580 |
And so, you see a lot of struggles for the companies 00:57:00.980 |
that were trying to build the best models, but failing. 00:57:03.060 |
- And arguably, not only do you have to have the best model, 00:57:05.420 |
you actually have to have an enterprise or a consumer 00:57:12.540 |
the best model implies that somebody's willing to pay you 00:57:16.460 |
And that's either an enterprise or a consumer. 00:57:18.340 |
So, I think, you know, you're quickly narrowing down 00:57:21.860 |
to just a handful of folks who will be able to compete, 00:57:27.340 |
I think on the who's willing to pay for these models is, 00:57:31.180 |
I think a lot more people will pay for the best model, right? 00:57:36.060 |
We have language models go through every regulatory, 00:57:39.060 |
filing, and permit to look at data center stuff 00:57:43.540 |
And we just use the best model because it's so cheap, right? 00:57:48.900 |
the value I'm getting out of it is so much higher. 00:57:52.140 |
- We're using Anthropic, actually, right now, 00:57:56.660 |
And so, just because O1 is a lot better on certain tasks, 00:58:00.220 |
but not necessarily regulatory filings and permitting 00:58:03.780 |
because the cost of errors is so much higher, right? 00:58:09.180 |
who makes $300,000 a year here in the Bay by 20%, 00:58:21.380 |
this is so worth using the most expensive model 00:58:23.700 |
because O1, as expensive it is relative to 4.0, 00:58:29.380 |
The cost for intelligence is so high in society, right? 00:58:32.860 |
That's why intelligent jobs are the most high-paying jobs. 00:58:36.380 |
White-collar jobs, right, are the most high-paying jobs. 00:58:38.220 |
If you can bring down the cost of intelligence 00:58:42.140 |
then there's a high market clearing price for that, 00:58:49.820 |
what's the cheapest thing at a certain level of intelligence, 00:58:52.060 |
but each time we break a new level of intelligence, 00:58:54.100 |
it's not just, oh, we've got a few more tasks we can do. 00:59:00.700 |
Very few people could use GPT-2 and 3, right? 00:59:13.420 |
and therefore the amount of sort of white-collar jobs 00:59:15.740 |
that it can augment increased productivity on will grow, 00:59:18.220 |
and therefore the market clearing price for that token 00:59:25.380 |
you know, just replacing tons of customer service calls 00:59:28.660 |
or whatever might be tempted to minimize the spend-- 00:59:47.700 |
and you said all of them are gonna inference LLAMA 7B, 01:00:04.180 |
if we're just deploying LLAMA 7B quality models, 01:00:10.500 |
Now, if we're deploying things that can like augment 01:00:16.780 |
and help us build robotics or AV or whatever else faster, 01:00:21.020 |
then that's a very different like calculation, right? 01:00:27.860 |
- And it may just, both these things may be true. 01:00:30.700 |
- Right, we're gonna have tons of small models 01:00:32.100 |
running everywhere, but the compute cost of them is so low. 01:00:49.860 |
You don't own them anywhere, you know, in between. 01:00:55.300 |
I'm talking about Hynex and I'm talking about, 01:00:58.740 |
As you think about the shift toward inference time compute, 01:01:01.820 |
it seems that the memory demanded of these chips, 01:01:11.060 |
Because if they're doing these passes, you know, 01:01:23.020 |
So, you know, talk to us a little bit about, you know, 01:01:25.820 |
kind of how you think about the memory market. 01:01:27.620 |
- Yeah, so, you know, to sort of like set the stage 01:01:40.540 |
attention, right, like holy grail of transformers, 01:01:43.460 |
i.e. how it like understands the entire context 01:01:57.780 |
And therefore, if I go from a context length of 10 to 100, 01:02:13.980 |
where they're thinking for hundreds of thousands of tokens. 01:02:24.020 |
- You're saying memory could grow faster than GPU cache. 01:02:32.100 |
their highest cost of goods sold is not TSMC, 01:02:36.620 |
It's actually HBM memory, primarily SK Hynix. 01:02:40.980 |
- Yeah, so there's three memory companies out there, right? 01:02:48.900 |
And this is like a big shift in the memory market as a whole 01:02:51.540 |
'cause historically it has been a commodity, right? 01:02:55.980 |
Whether I buy from Samsung or SK Hynix or Micron or. 01:03:04.180 |
because there's a Chinese memory maker, CXMT, 01:03:07.980 |
and their memory is not as good as the last, but it's fine. 01:03:15.940 |
- In HBM, Samsung has almost no share, right? 01:03:21.460 |
And so this is hitting Samsung really hard, right? 01:03:25.060 |
Despite them being the largest memory maker in the world, 01:03:29.500 |
it's like, yeah, Samsung's a little bit ahead in tech 01:03:35.020 |
because on the low end, they're getting a little bit hit. 01:03:40.960 |
On the flip side, you have companies like SK Hynix 01:03:43.500 |
and Micron who are converting significant amounts 01:03:47.380 |
of their capacity of sort of commodity DRAM to HBM. 01:03:52.960 |
In that if someone hits a certain level of technology, 01:04:01.300 |
but because reasoning requires so much more memory 01:04:04.980 |
and the cost of goods sold of an H100 to Blackwell, 01:04:08.000 |
the percentage of costs to HBM has grown faster 01:04:11.860 |
than the percentage of costs to leading edge silicon. 01:04:15.640 |
You've got this big shift or dynamic going on. 01:04:20.900 |
but it applies to the hyperscalers GPUs as well, right? 01:04:23.740 |
Or accelerators like the TPU, Amazon Tranium, et cetera. 01:04:32.140 |
If you listen to Jensen at least describe it, 01:04:41.100 |
there's more software associated with the product today, 01:04:43.460 |
but it's also how it's integrated into the overall system, 01:04:56.860 |
We know the secular curve is up and to the right. 01:05:00.740 |
It may be differentiated enough to not be a commodity. 01:05:12.100 |
- They've been good, but they haven't been fantastic. 01:05:13.580 |
Actually, regular memory, high-end like server memory 01:05:16.540 |
that is not HBM is actually higher gross margin than HBM. 01:05:20.780 |
NVIDIA is pushing the memory makers so hard, right? 01:05:23.660 |
They want the faster, newer generation of memory, 01:05:28.100 |
but not necessarily like everyone else for servers. 01:05:32.140 |
Is that, hey, even though Samsung may achieve level four, 01:05:35.580 |
right, or level three or whatever that they had previously, 01:05:48.980 |
They give you more memory and more memory bandwidth. 01:06:06.260 |
and our whole post about it and our analysis of it 01:06:17.060 |
And B, it gives you the most HBM capacity per dollar 01:06:29.660 |
Like, hey, we maybe can't design as well as NVIDIA, 01:06:33.080 |
but we can put more memory on the package, right? 01:06:38.300 |
They don't have the networking nearly as good. 01:06:41.260 |
Their compute elements are not nearly as good. 01:06:43.640 |
By golly, they've got more memory bandwidth per dollar. 01:06:55.540 |
why no one would seemingly wanna pick a fight with NVIDIA, 01:07:05.480 |
Like OpenAI is constantly talking about their own chip. 01:07:12.920 |
Let's start with AMD just because they're a standalone 01:07:15.920 |
company, and then we'll go to some of the internal program. 01:07:20.840 |
because silicon engineering-wise, they're amazing, right? 01:07:28.080 |
but that's like, you know, stealing candy from a baby. 01:07:32.700 |
Over a 20-year period, it was pretty (beep) amazing. 01:07:35.600 |
- So AMD is really good, but they're missing software. 01:07:42.780 |
They won't spend the money to build a GPU cluster 01:07:46.340 |
for themselves so that they can develop software, right? 01:07:51.540 |
Like NVIDIA, you know, the top 500 supercomputer list 01:07:54.860 |
is not relevant because most of the biggest supercomputers 01:07:57.420 |
like Elon's and Microsoft's and so on and so forth, 01:08:09.180 |
whether it be network software or compute software, 01:08:19.660 |
software's not working, NVIDIA will push it the next day 01:08:24.840 |
Because there's tons of things that break constantly 01:08:30.020 |
And I don't know why they won't spend the money 01:08:39.060 |
so if I make a better chip than Intel, then I'm great. 01:08:41.480 |
Because software, x86, it's x86, everything's fungible. 01:08:50.260 |
- Yeah, and so they bought this systems company 01:08:53.180 |
But they're, you know, the whole rack scale architecture, 01:08:57.180 |
which Google deployed in 2018 with the TPU v3. 01:09:00.420 |
- Are there any hyperscalers that are so interested 01:09:04.680 |
in AMD being successful that they're co-developing 01:09:08.300 |
- So the hyperscalers all have their own custom 01:09:11.100 |
silicon efforts, but they also are helping AMD 01:09:15.060 |
So Meta and Microsoft are helping them with software, right? 01:09:26.820 |
if I have the best engineering team in the world, 01:09:28.620 |
that doesn't tell me what the problem is, right? 01:09:36.540 |
It doesn't know what inference economics look like. 01:09:39.260 |
And so how do they know what trade-offs to make? 01:09:41.340 |
Do I push this lever on the chip a bit harder, 01:09:43.500 |
which then makes me have to back off on this? 01:09:48.700 |
but not enough that AMD is on the same timelines as NVIDIA. 01:09:52.580 |
- How successful will AMD be in the next year on AI revenue 01:09:56.980 |
and what kind of sockets might they succeed in? 01:10:00.620 |
- Yes, I think they'll have a lot less success 01:10:05.520 |
And they'll have less success than they did with Meta 01:10:11.500 |
And it's because like the regulations make it so 01:10:13.860 |
actually AMD's GPU is like quite good for China 01:10:23.500 |
They just won't like go gangbusters like people are hoping. 01:10:28.620 |
their share of total revenue will fall next year. 01:10:33.620 |
Billions of dollars of revenue is not nothing to stop at. 01:10:37.260 |
You earlier stated that it's got the second most workloads. 01:10:42.260 |
It seems like by a lot, like it's firmly in second place. 01:10:57.420 |
It's got good, you know, architecture, et cetera. 01:11:03.540 |
But when you say, hey, if I'm spending X amount of money 01:11:07.280 |
and then what's my system, Google's TPU looks amazing, right? 01:11:11.560 |
that Nvidia maybe has not focused on as much, right? 01:11:14.800 |
So actually their interconnects between chips 01:11:17.240 |
is arguably competitive, if not better in certain aspects, 01:11:22.000 |
Because they've been doing this with Broadcom, 01:11:27.640 |
And since 2018, they've had this scale up, right? 01:11:36.640 |
And while it's not a switch, it's a point to point, 01:11:44.120 |
are not all you should look at, but this is important. 01:11:47.800 |
Google's brought in water cooling for years, right? 01:11:51.760 |
they needed water cooling on this generation. 01:11:53.560 |
And Google's brought in a level of reliability 01:12:00.360 |
You know, the dirty secret is to go ask people 01:12:02.120 |
what the reliability rate of GPUs is in the cloud 01:12:05.800 |
It's like, oh God, it's not, they're reliable-ish, 01:12:12.560 |
- Why has TPU not been more commercially successful 01:12:16.360 |
- I think Google keeps a lot of their software internal 01:12:26.840 |
You know, there's a lot of software that DeepMind uses 01:12:32.840 |
- Even their Google Cloud offering relative to AWS 01:12:46.480 |
like list price of a GPU at Google Cloud is also egregious. 01:12:51.160 |
But you as a person know when I go rent a GPU, 01:12:55.040 |
you know, I tell Google like, hey, like, you know, 01:12:57.760 |
you can get around the first round of negotiations, 01:13:00.280 |
But then you're like, well, look at this offer from Oracle 01:13:02.320 |
or from Microsoft or from Amazon or from CoreWeave 01:13:07.880 |
And Google might not match like many of these companies, 01:13:10.200 |
but like, they'll go down because they, you know, 01:13:20.600 |
- A little bit over versus like the $4 quoted, right? 01:13:26.200 |
And so people see the list price and they're like, eh. 01:13:34.400 |
Google is better off using all of their TPUs internally. 01:13:37.280 |
Microsoft rents very few GPUs by the way, right? 01:13:46.160 |
because the gross margin on selling tokens is 50 to 70%. 01:13:56.680 |
- And they've said out of the 10 billion that they've quoted, 01:13:59.000 |
none of that's coming from external renting of GPUs. 01:14:02.360 |
- If Gemini becomes hyper competitive as an API, 01:14:20.200 |
So it's not that like, you know, that you're not using, 01:14:22.640 |
every YouTube video you upload is going through a TPU, right? 01:14:25.200 |
Like, you know, it goes through other chips as well 01:14:27.480 |
that they've made themselves custom chips for YouTube. 01:14:29.160 |
But like, there's so much that touches a TPU, 01:14:31.600 |
but you indirectly would never rent it, right? 01:14:38.800 |
there's only one company accounts for over 70% 01:14:41.720 |
of Google's revenue from TPUs as far as I understand, 01:14:48.000 |
But, you know, that may be a story for another time, but-- 01:14:51.920 |
- You just did a super deep piece on Tranium. 01:14:59.960 |
- Yeah, so funnily enough, Amazon's chip is the Amazon, 01:15:08.800 |
yes, it uses more silicon, yes, it uses more memory, 01:15:11.760 |
yes, the network is like somewhat comparable to TPUs, 01:15:18.160 |
They just do it in a less efficient way in terms of, 01:15:27.120 |
Because they're working with Marvell and L-chip 01:15:30.200 |
on their own chips versus working with Broadcom, 01:15:32.320 |
the leader in networking, who then can use passive cables, 01:15:37.000 |
Like there's other things here, their SERTI speed is lower, 01:15:40.280 |
they spend more silicon area, like there's all these things 01:15:47.000 |
this would suck if it was a merchant silicon thing, 01:15:58.080 |
they're paying lower margins in general, right? 01:16:01.040 |
They're paying the margins to Marvell on HPM. 01:16:03.240 |
You know, there's all these different things they do 01:16:04.720 |
to crush the price down to where their AmazonBasics TPU, 01:16:08.920 |
the Tranium 2, right, is very, very cost-effective 01:16:14.040 |
in terms of HBM per dollar, memory bandwidth per dollar, 01:16:21.040 |
it actually requires them two racks to do 64, 01:16:26.480 |
and their memory per chip is lower than Nvidia's, 01:16:29.920 |
and their memory bandwidth per chip is lower than Nvidia, 01:16:32.040 |
but you're not paying north of $40,000 per chip 01:16:37.040 |
for the server, you're paying significantly less, right? 01:16:41.840 |
Like, you know, it's like such a gulf, right, for Amazon, 01:16:44.440 |
and then they pass that on to the customer, right, 01:16:50.400 |
and because of this, right, Amazon and Anthropic 01:16:53.000 |
have decided to make a 400,000 Tranium supercomputer, right? 01:16:58.200 |
400,000 chips, right, going back to the whole 01:17:14.040 |
You want your inference to be more distributed than that. 01:17:20.600 |
and while technically it's not that impressive, 01:17:36.880 |
to kind of what you see happening in 25 and 26, right? 01:17:43.940 |
we've seen Broadcom, you know, explode higher, 01:17:53.120 |
with Broadcom being this play on custom ASICs, 01:17:57.760 |
Nvidia's got a lot of new competition, pre-training, 01:18:01.400 |
you know, not improving at the rate that it was before. 01:18:13.440 |
kind of the things that are most misunderstood, 01:18:15.520 |
best ideas, you know, in the spaces that you cover? 01:18:20.320 |
- So I think a couple of the things are, you know, 01:18:23.200 |
hey, Broadcom does have multiple custom ASIC wins, right? 01:18:27.760 |
Meta's ramping up mostly still for recommendation systems, 01:18:38.840 |
You know, there's Apple who are not quite making 01:18:42.720 |
but a small portion of it will be made with Broadcom, right? 01:18:45.440 |
You know, there's a lot of wins they have, right? 01:18:53.360 |
so like it could be a failure and not be good, 01:19:00.480 |
or at least, you know, good price to performance 01:19:03.320 |
like Amazon's and it could ramp a lot, right? 01:19:07.000 |
but Broadcom has that custom ASIC business, one. 01:19:11.880 |
the networking side is so, so important, right? 01:19:14.240 |
Yes, NVIDIA is selling a lot of networking equipment, 01:19:23.600 |
but they could also, they also need to network 01:19:29.760 |
They could go to Marvell or many other competitors 01:19:43.080 |
biggest competitive advantages on a hardware basis 01:19:53.360 |
Not just, AMD will be using that competitor to NVSwitch, 01:19:59.640 |
They're going to Broadcom to get it made, right? 01:20:16.200 |
Who's best positioned from current levels to do well? 01:20:30.200 |
there is a bit of a slowdown in Google TPU purchases 01:20:34.840 |
They just literally have no data center space to put them. 01:20:36.760 |
So we actually like, you know, can see how they're like, 01:20:39.600 |
there's a bit of a pause, but people may look past that. 01:20:42.880 |
Beyond that, right, it's the question is like, 01:20:52.840 |
Are the hyperscalers going to be able to internalize 01:20:56.040 |
Like it's no secret Google's trying to leave Broadcom. 01:20:58.920 |
They could succeed or they could fail, right? 01:21:06.520 |
Like, you know, we've had these two massive years, right, 01:21:15.680 |
Do you think it's another year that the sector does well? 01:21:24.640 |
they're going to spend a crapload more next year, right? 01:21:26.760 |
And therefore the ecosystem of networking players, 01:21:29.560 |
of ASIC vendors, of systems vendors is going to do well, 01:21:33.560 |
whether it be NVIDIA or Marvell or Broadcom or AMD, 01:21:36.320 |
or, you know, generally, you know, some better than others. 01:21:39.020 |
The real question that people should be looking out to 01:21:48.560 |
And that's going to drag the entire component supply chain up. 01:21:51.000 |
It's going to bring so many people with them. 01:21:52.840 |
But 2026 is like where the reckoning comes, right? 01:21:55.800 |
You know, will people keep spending like this? 01:22:03.560 |
Because if they don't continue to get better, 01:22:05.200 |
in my opinion, we'll get better faster, in fact, next year, 01:22:14.660 |
is there is consolidation in the Neo cloud market, right? 01:22:19.580 |
that we talk to, that we see how many GPUs they have, right? 01:22:31.060 |
Where you can, you used to have to pay, you know, 01:22:35.420 |
You'd sign a venture ground and you'd buy a cluster 01:22:40.400 |
Nowadays, you can get three-month, six-month deals 01:22:43.320 |
at way better pricing than even the four-month 01:22:49.160 |
And on top of that, it's not just through the Neo clouds, 01:22:51.520 |
Amazon's pricing for, you know, on-demand GPUs is falling. 01:22:54.480 |
Now it's still over, it's like still really expensive, 01:22:56.480 |
relatively, but like pricing is falling really fast. 01:23:04.160 |
And that's because five of those are sovereign, right? 01:23:09.700 |
- What percentage of the industry AI revenues 01:23:13.260 |
have come from those Neo clouds that may not survive? 01:23:26.360 |
because enterprises purchases of GPU clusters 01:23:28.860 |
is still quite low and it ends up being better for them 01:23:42.400 |
where you see industry volumes actually down versus 2025 01:23:47.400 |
or Nvidia volumes actually down meaningfully from 2025? 01:23:55.200 |
- So when you look at custom ASIC designs that are coming, 01:24:01.960 |
the revenue, the content in each chip is exploding. 01:24:13.560 |
obviously they're cutting margins a little bit, 01:24:20.920 |
is there a scenario where industry revenues are down in '26 01:24:36.720 |
are they okay with taking their free cash flow to zero? 01:24:42.920 |
their free cash flows close to zero and just spent. 01:24:46.700 |
But then that's only if models continue to get better, 01:24:50.160 |
And then B, are we going to have this huge influx 01:24:52.400 |
of capital from people we haven't had it yet from? 01:24:55.000 |
The Middle East, the sovereign wealth funds in Singapore 01:24:57.960 |
and Nordics and Canadian pension fund and all these folks, 01:25:09.060 |
I truly do believe that OpenAI and XAI and Anthropic 01:25:19.960 |
Well, it's 8 billion and it might double or whatever, 01:25:31.040 |
Elon is forcing everyone to spend more actually, right? 01:25:36.680 |
because everybody's like, "Well, we can't get outscaled 01:25:41.240 |
And so there's sort of a game of chicken there too. 01:25:47.360 |
So, you know, in sort of Pascal's wager sense, right? 01:25:50.760 |
If I underspend, that's just the worst scenario ever. 01:25:56.200 |
But if I overspend, yeah, shareholders will be mad, 01:26:03.760 |
'cause if that becomes the reasoning for doing it, 01:26:06.320 |
you're more, the probability of overshooting goes up. 01:26:22.600 |
and go back to what Satya said to us last week. 01:26:31.240 |
who are making the purchases of the GPUs, right? 01:26:36.360 |
I'm gonna buy a certain amount every single year, 01:26:58.520 |
and they're making, he and Amy are making some forecast 01:27:07.000 |
And so if you assume they're acting rationally, 01:27:11.840 |
it's also the rate of adoption of the underlying, 01:27:15.080 |
you know, enterprises who are using their services. 01:27:26.520 |
So, you know, if you think that infrastructure expenses 01:27:33.880 |
that the underlying inference revenues, right, 01:27:36.600 |
both on the consumer side and the enterprise side 01:27:38.880 |
are gonna grow somewhere in that range as well. 01:27:41.760 |
- There is definitely an element of spend ahead though, 01:27:44.480 |
- And it's point in time spend versus, you know, 01:27:47.800 |
for the next five years for the server, right? 01:27:49.640 |
So I think there is an element of that for sure, 01:27:53.080 |
Models, the whole point is models getting better 01:28:00.880 |
but people are definitely spending ahead of what's charted. 01:28:13.840 |
Congratulations on the success of your business. 01:28:17.120 |
You know, I think you add a lot of important information 01:28:32.880 |
But, you know, as both an investor and an analyst, 01:28:39.120 |
there are definitely people out there who are spending 01:28:41.920 |
who don't have commensurate revenues, to your point. 01:28:55.480 |
I haven't heard that from everybody else, right? 01:29:04.080 |
I think you already see some of these smaller 01:29:06.720 |
second and third tier models, changing business model, 01:29:09.800 |
falling aside, no longer engaged in the arms race, 01:29:16.120 |
I think that's part of the creative destructive process,