back to indexDebunking The AI Reset: Alien Mind Fear, Chat GPT, Future of AI & Slow Productivity | Cal Newport
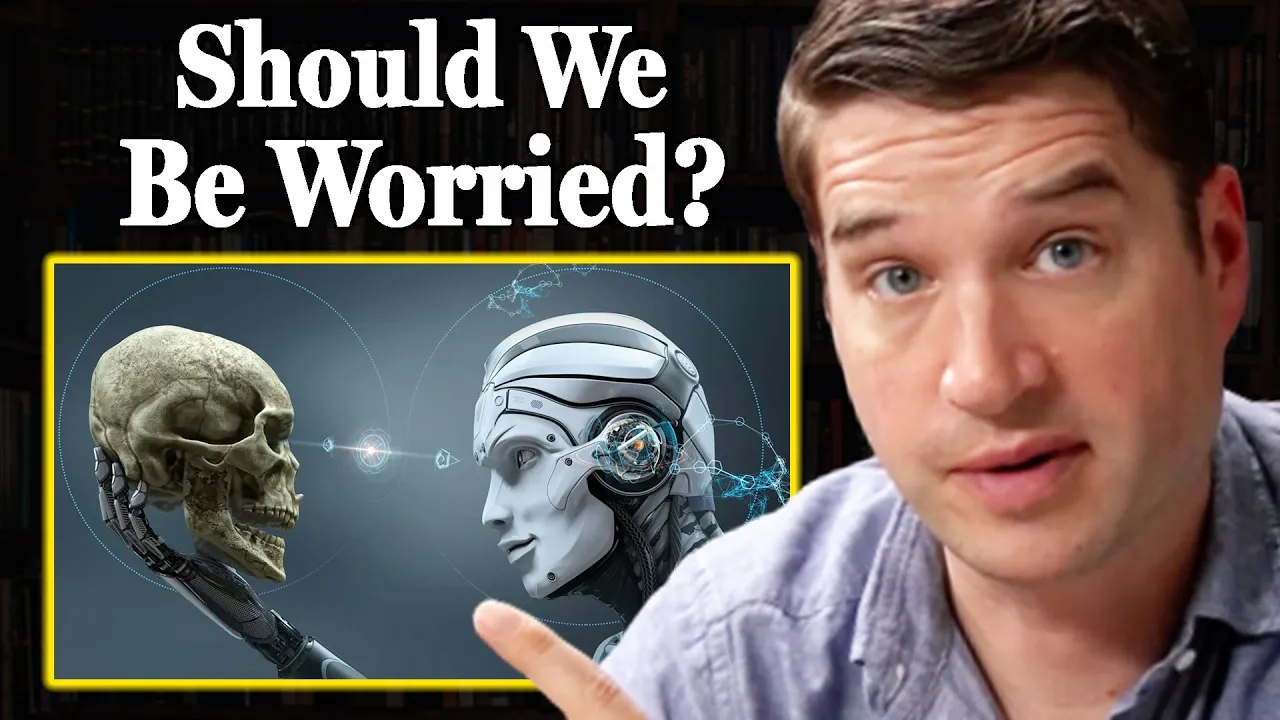
Chapters
0:0 Defusing AI Panic
41:55 Will A.I. agents spread misinformation on a large scale?
46:42 Is lack of good measurement and evaluation for A.I. systems a major problem?
52:4 Is the development of A.I. the biggest thing to happen in technology since the internet?
56:23 How do I balance a 30 day declutter with my overall technology use?
62:54 How do I convince my team that prioritizing quality over quantity will help them get promotions?
68:17 Distributed trust models and social media
78:9 Using Deep Work and Slow Productivity to engineer a better work situation
87:10 Employees fired for using “mouse jigglers”
00:00:00.000 |
So there are a lot of concerns and excitements and confusions surrounding our current moment 00:00:10.480 |
Perhaps one of the most fundamental of these concerns is this idea that in our quest to 00:00:15.600 |
train increasingly bigger and more capable AI systems that we might accidentally create 00:00:23.760 |
I want to address this particular concern from the many concerns surrounding AI in my 00:00:29.000 |
capacity as a computer science professor and one of the founding members of Georgetown's 00:00:34.720 |
I have been thinking a lot from a sort of technological perspective about this idea 00:00:39.760 |
of runaway or unexpected intelligence and AI systems. 00:00:42.840 |
I have some new ideas I want to preview right here. 00:00:45.600 |
These are in rough form, but I think they're interesting. 00:00:48.440 |
And I hopefully will give you a new way and a more precise and hopefully comforting way 00:00:52.960 |
of thinking about the possibility of AI getting smarter than we hope. 00:00:57.600 |
All right, so where I want to start with here is the fear. 00:01:02.000 |
Okay, so one way to think of the fear that I want to address is what I call the alien 00:01:06.640 |
mind fear, that we are training these systems, most popularly as captured by large language 00:01:13.740 |
model systems like the GPT family systems, and we don't know how they work. 00:01:19.400 |
They sit in big data centers and do stuff for months and hundreds of millions of dollars 00:01:24.120 |
We get this thing afterwards and we engage with it and say, "What can this thing do now?" 00:01:30.680 |
We don't understand how they're going to work. 00:01:33.360 |
That's what sets up this fear of these minds getting too smart. 00:01:36.240 |
I want to trace some of the origins of this particular fear. 00:01:39.200 |
I'm going to load up on the screen here for people who are watching instead of just listening, 00:01:46.220 |
This comes from the New York Times in March of 2023. 00:01:49.120 |
So this was pretty soon after CHAT-GPT made its big late 2022 debut. 00:01:54.560 |
The title of this opinion piece is, "You can have the blue pill or the red pill, and we're 00:02:00.520 |
This is co-authored by Yuval Harari, who you know from his book, Sapiens, as well as Tristan 00:02:05.640 |
Harris, who you know as the sort of whistleblower on social media who now runs a nonprofit dealing 00:02:12.840 |
with the harms of technology, and Asa Raskin, who works with Tristan at his nonprofit. 00:02:19.660 |
So this was essentially a call for we need to be worried about what we're building with 00:02:24.120 |
these large language model systems like CHAT-GPT. 00:02:28.640 |
There is a particular quote in here that I want to pull out, and I'll read this off of 00:02:38.360 |
We don't know much about it, except that it is extremely powerful and offers us bedazzling 00:02:43.400 |
gifts but could also hack the foundations of our civilization." 00:02:46.920 |
So we get this alien terminology, this notion of we don't really know how this thing works, 00:02:52.480 |
and so we don't really know what this thing might be capable. 00:02:56.240 |
Let me give you another example of this thinking. 00:02:59.880 |
This is an academic paper that was from this same time. 00:03:08.600 |
I wrote about this paper, actually, in a New Yorker piece that I wrote earlier this year 00:03:17.440 |
The title of this influential paper is important. 00:03:20.320 |
"Sparks of Artificial General Intelligence, Early Experiments with GPT-4." 00:03:27.280 |
The whole structure of this paper is that these researchers, because they're at Microsoft, 00:03:33.240 |
This was before it was publicly released, and they ran intelligence tests, sort of human 00:03:39.220 |
intelligence tests that had been developed for humans. 00:03:41.040 |
They were running these intelligence tests on GPT-4 and were really surprised by how 00:03:46.440 |
So this sort of glimmers of AGI, this glimmers of artificial general intelligence, the sort 00:03:51.240 |
of theme of this is, "My God, these things are smarter than we thought. 00:03:56.720 |
These machines are becoming rapidly powerful." 00:03:59.000 |
So it was sort of, "Hey, if you were worried about GPT-3.5, the original chat GPT language 00:04:05.080 |
model that they were writing about in the New York Times op-ed, wait until you see what's 00:04:11.480 |
There's a general rational extrapolation to make here. 00:04:16.600 |
The original GPT worried people, like Yuval Harari. 00:04:25.520 |
We keep extrapolating this curve, the GPT-5, GPT-6. 00:04:30.040 |
It's going to keep getting more capable in ways that are unexpected and surprising. 00:04:35.360 |
It's very rational to imagine this extrapolation bringing these alien minds to abilities where 00:04:43.000 |
We're uncomfortable about how smart they are. 00:04:45.720 |
They can do things we don't even really understand. 00:04:49.080 |
This is this origin of this, "These things are going to get smarter than we hoped." 00:04:52.120 |
I had a conversation with a friend of mine about this who's really interested in AI, 00:04:57.720 |
The way he conceptualized this is he just said, "Look, we're going to keep building 00:05:02.320 |
One of these days, probably pretty soon, as we keep 10x-ing the size of these models, 00:05:06.000 |
we are going to be very uncomfortable with what we build." 00:05:08.240 |
All right, so that is our setup for the concern. 00:05:13.360 |
To address this concern, the first thing I want to do is start with a strong but narrow 00:05:23.000 |
A large language model in isolation can never be understood to be a mind. 00:05:33.680 |
And what I'm saying here is actually very narrow. 00:05:35.520 |
If we actually take a specific large language model like GPT-4, that by itself, even if 00:05:42.480 |
we make it bigger, even if we train it on many more things, cannot by itself be something 00:05:48.600 |
that we imagine as an alien mind with which we have to contend like we might another mind. 00:05:56.480 |
The reason is, in isolation, what a large language model does is it takes an input. 00:06:03.240 |
This information moves forward through layers. 00:06:06.960 |
And out of the other end comes a token, which is a part of a word. 00:06:11.120 |
In reality, it's a probability distribution over tokens, but whatever, a part of a word 00:06:20.680 |
Now how it generates what token to spit out next can have a huge amount of sophistication, 00:06:28.600 |
It's difficult to come up with the proper analogies for describing this. 00:06:31.760 |
But I think a somewhat reductive but useful way for understanding how these tokens are 00:06:36.920 |
produced is the following analogy that I used in a New Yorker piece from a few months ago. 00:06:41.060 |
You can imagine what happens is when you have your input, which is like the prompt or the 00:06:44.880 |
prompt plus the part of the answer you've generated already, as this is going through 00:06:50.480 |
the large language model, it can come up with candidates for like the next word or part 00:07:00.080 |
This is known as Ngram prediction in some sense, except for here, it's a little bit 00:07:03.440 |
more sophisticated because with self-attention, it can look at multiple parts of the input 00:07:08.320 |
But it's not too hard to be like, this is kind of the pool of grammatically correct, 00:07:13.320 |
semantically correct next words that we could output. 00:07:16.280 |
How do we figure out which of those things to output to actually match what's being asked 00:07:19.880 |
or what's actually being discussed in the prompt? 00:07:21.760 |
Well, this is where these models go through something like complex pattern recognition. 00:07:25.160 |
I like to use the metaphor of a massive checklist, a checklist that has billions of possible 00:07:34.760 |
We're in the middle of producing moves for a chess game. 00:07:38.600 |
This is like a middle of a chess game move that's being produced. 00:07:51.280 |
We're sort of understanding as it goes to these recognizers, this is what we're trying, 00:07:55.800 |
this is what we're in the middle of talking about. 00:07:57.640 |
And then you can imagine, again, this is a rough analogy that you have these really complex 00:08:02.880 |
It looks at the combination of different properties that describes what we're talking about. 00:08:07.800 |
They combine these properties to say, okay, given this combination of properties of what 00:08:11.600 |
we're talking about, which of these possible correct, grammatically correct next word or 00:08:16.500 |
tokens we could output, which of these makes the most sense, right? 00:08:20.400 |
But then it's combining, okay, it's a chess game and here's the recent chess moves and 00:08:27.480 |
we're supposed to be describing a middle game move. 00:08:29.720 |
And the rules might say, these are legal moves given like this current situation. 00:08:35.800 |
So of the different things we could output here that looks like the move in a chess game, 00:08:39.760 |
these are actually legal moves and so let's choose one of these, right? 00:08:43.920 |
So you have possible next words, you have checklist of properties, you have combinatorial 00:08:48.080 |
combinations of those properties with rules that then help you influence which of these 00:08:53.640 |
And all of this sort of happens in this sort of feed forward manner. 00:08:56.160 |
Those checklists and the rules in particular can be incredibly complicated. 00:09:00.840 |
The rules can have novel combinations of properties. 00:09:05.280 |
So combinations of properties that were never seen in the training data, but you have rules 00:09:09.180 |
that just combine properties and that's how you can produce output with these models that 00:09:13.360 |
don't directly match anything that ever solved before. 00:09:20.880 |
But in the end, this is still, you can imagine it like a giant metal machine with dials and 00:09:26.440 |
gears, and you're turning this big crank and hundreds of thousands of gears are all cranking 00:09:34.560 |
And at the very end, at the far end of the machine, there's a dial of letters. 00:09:41.760 |
A word or a piece of the word is what comes out on the other side after you've turned 00:09:46.120 |
It can be a very complicated apparatus, but in the end, what it does at the end is it 00:09:53.120 |
So it doesn't matter how big you make this thing. 00:09:56.160 |
It can, it spits out parts of words, no matter how sophisticated its pattern recognizers 00:10:01.940 |
and combinatorial rule generators, no matter how sophisticated these are, it's a word spitter 00:10:11.080 |
I wanted to interrupt briefly to say that if you're enjoying this video, then you need 00:10:15.040 |
to check out my new book, Slow Productivity, The Lost Art of Accomplishment Without Burnout. 00:10:22.500 |
This is like the Bible for most of the ideas we talk about here in these videos. 00:10:27.920 |
You can get a free excerpt at calnewport.com/slow. 00:10:39.480 |
But where things get interesting, as people like to tell me when I talk to people, is 00:10:43.420 |
when you begin to combine this really, really sophisticated word generator with control 00:10:52.320 |
layers, something that sits outside of and works with the language model, that's really 00:11:04.020 |
It's better understanding the control logic that we place outside of the language models 00:11:09.320 |
that we get a better understanding of the possible capabilities of artificial intelligence, 00:11:13.780 |
because it's the combined system, language model plus control logic that becomes more 00:11:22.520 |
It chooses what to activate the model with, what input to give it, and it can then second 00:11:27.200 |
actuate in the real world or the digital world based on what the model says. 00:11:31.440 |
So it's the control logic that can put input into the model and then take the output of 00:11:35.640 |
the model and actuate that, like take action. 00:11:38.680 |
Do something on the internet, move a physical thing. 00:11:41.720 |
So it's that control logic with its activation actuation capability that when combined with 00:11:45.700 |
a language model, which again is just a word generator, that's when these systems begin 00:11:51.200 |
So something I've been doing recently is sort of thinking about the evolution of control 00:11:58.040 |
logic that can be appended to generative AI systems like large language models. 00:12:04.480 |
And I want to go through like what we know right now. 00:12:08.960 |
For people who are watching instead of just listening, you can watch me draw this on the 00:12:25.360 |
Oh man, Jesse, my handwriting is only getting worse. 00:12:30.080 |
People are like, "Oh my God, Cal's having a stroke." 00:12:35.600 |
So layer zero control logic is actually what we got right away with the basic chatbots 00:12:42.660 |
So I'm going to label this like, for example, ChatGPT. 00:12:51.640 |
So level zero control logic basically just implements what's known as auto regression. 00:12:58.520 |
So large language model spits out a single word or part of a word, but when you type 00:13:02.500 |
a query into ChatGPT, you don't want just a one word answer. 00:13:07.860 |
So there's a basic what I'm calling layer zero control logic that takes your prompt, 00:13:14.280 |
submits it to the underlying large language model, gets the answer of the language model, 00:13:18.800 |
which is a single word or part of word that expands the input in a reasonable way. 00:13:25.320 |
So now the input is your original prompt plus the first word of the answer. 00:13:29.880 |
It then inputs fresh, fresh copy of the model, inputs a slightly longer input. 00:13:37.480 |
The control logic adds that and now submits the slightly longer input to the model. 00:13:43.180 |
And it sort of keeps doing this until it judges this as a complete answer. 00:13:47.680 |
And then it returns that answer to you, the user who are typing into the ChatGPT interface, 00:13:55.880 |
That's how we just repeatedly keep using the same language model to get very long answers, 00:14:02.520 |
The model by itself can just spit out one thing. 00:14:07.140 |
Another thing that we got in early versions and contemporary versions of chatbots is the 00:14:11.360 |
other thing level layer zero control logic might do is append previous parts of your 00:14:20.160 |
So you know how when you're using ChatGPT or you're using Cloud or something like this 00:14:24.000 |
or perplexity, you can sort of ask a follow-up question, right? 00:14:29.040 |
So there's a little bit of control logic here where what it's really doing is it's not just 00:14:32.200 |
submitting your follow-up question by itself to the language model. 00:14:35.400 |
Remember, the language models have no memory. 00:14:36.880 |
It's the exact same snapshot of this model, trained whenever it was trained, that's used 00:14:43.160 |
What the control logic will do is take your follow-up question, but then also take all 00:14:47.440 |
of the conversation before that and paste that whole thing into the input, right? 00:14:51.400 |
So this is simple logic, but it makes the token generators useful. 00:14:56.440 |
So we already have some control logic and even the most basic generative AI tools. 00:15:03.280 |
Now let's go up to what I'm going to call layer one. 00:15:08.800 |
So with layer one, now we get two things we didn't have in layer zero. 00:15:14.240 |
We're still taking input from a user, like you're typing some sort of prompt, but now 00:15:19.160 |
we might get a substantial transformation of what you typed in for whatever is actually 00:15:28.920 |
So what you type in might go through a substantial transformation by the control logic before 00:15:37.080 |
The other key part of layer one is there's actuation. 00:15:42.640 |
So it might also do some actions on behalf of you or the language model based on the 00:15:49.560 |
output of language model, instead of just sending text back to the user, it might actually 00:15:56.880 |
So an example of this, for example, would be the web enabled chatbots like Google's 00:16:07.080 |
So Google's Gemini, you can ask it a question where it's going to do a contemporary web 00:16:11.560 |
search, like stuff that's on the internet now, not what it was trained with when they 00:16:15.040 |
changed the original model, but it can actually look at stuff on the web and then give you 00:16:19.260 |
an answer based on stuff that actually found contemporaneously on the web. 00:16:25.880 |
What's really happening here is when you ask something like Gemini or something like perplexity, 00:16:31.240 |
a question about, you know, a current, a web search, an advanced web search, the control 00:16:35.680 |
logic before the language model is ever involved, actually just goes and does a Google search 00:16:43.320 |
and it finds like, these are relevant articles. 00:16:46.600 |
It then takes the text of these articles and it puts it together into a really long prompt, 00:16:53.400 |
I'm simplifying this, but this is basically what's going on. 00:16:56.040 |
So the language model doesn't know about the specific articles necessarily in advance. 00:17:00.240 |
It wasn't trained on them, but it gets a really long prompt that the prompt written by the 00:17:04.280 |
control logic might say something like, please look at the following, you know, text that's 00:17:10.760 |
pasted in this prompt and summarize from it, you know, an answer to the following question, 00:17:17.840 |
And then below it is, you know, 5,000 words of web results, right? 00:17:22.160 |
So the prompt that's actually being submitted under the covers to the language model here 00:17:29.040 |
It's a much bigger, substantially transformed prompt, right? 00:17:34.660 |
So if we consider like OpenAI's original plugin, you know, so these are these things you can 00:17:40.880 |
turn on in GPT-4 that can do things, for example, like generate a picture for you or book airline 00:17:47.040 |
flights or show you the schedules of airlines. 00:17:51.820 |
In the new Microsoft Copilot integrations, you can have the model take action on your 00:17:57.520 |
behalf and tools like Microsoft Excel or in Microsoft Word. 00:18:01.620 |
So there's actual action happening in the software world based on the model. 00:18:05.480 |
This is also being done by the control logic, right? 00:18:09.300 |
So you're saying like, help me find a flight to, you know, whatever, this place at this 00:18:16.020 |
The control logic is going to, before we get to a language model, you know, it might make 00:18:24.140 |
Or what it might do is actually create a prompt to give to the language model and says, hey, 00:18:28.740 |
please take this question about, you know, flight request and summarize it in the following 00:18:33.900 |
format for me, which is like a very, you know, flight day destination. 00:18:38.280 |
The language model then returns to the control logic a better, more consistently formatted 00:18:47.800 |
Now the control logic, which can understand this really well, format a request, talk over 00:18:52.460 |
the internet to a flight booking service, get the results, and then it can pass those 00:18:57.020 |
results to the language model and say, okay, take these flight results and please like 00:19:00.580 |
write a summary of these in like a polite English. 00:19:06.220 |
And so what you see as the user is like, okay, I asked about flights and then I got back 00:19:10.300 |
like a really nice response, like here's your various options for flights. 00:19:13.380 |
And then maybe you say, hey, can you book this flight for me? 00:19:16.140 |
The control logic takes that and say, hey, can you take this request from the user? 00:19:19.340 |
And again, put it into this really precise format, you know, flight number, flight, whatever. 00:19:25.780 |
And now the control logic can take that and talk over the internet to the flight booking 00:19:31.840 |
So this sort of actuation that happens in the sort of our current level of plugins. 00:19:36.180 |
Same thing if you're asking co-pilot, Microsoft co-pilot to do something, build a table in 00:19:42.040 |
Microsoft Word or something like this, it's taking your request. 00:19:45.500 |
It's asking the language model to essentially reformat your request into something much 00:19:49.180 |
more systematic and canonical, and then the control logic talks to Microsoft Word. 00:19:54.700 |
These language models are just giant tables of numbers in a data warehouse somewhere being 00:19:59.820 |
They don't talk to Microsoft Word in your computer, the control logic does as well. 00:20:05.260 |
So now we have substantial transformation of your prompts and some actuation on the 00:20:12.780 |
So now we move up and things begin to get more interesting. 00:20:19.660 |
I've been writing some about this for the New Yorker among other places. 00:20:23.860 |
So in layer two, we now have the control logic able to keep state and make complex planning 00:20:33.700 |
So it's going to be highly interactive with the language model, perhaps making many, many 00:20:37.820 |
queries to the language model on route to trying to execute whatever the original request 00:20:43.460 |
So this is where things start to get interesting. 00:20:46.140 |
A less well-known, but illustrative example of this is that the meta put out this bot 00:20:55.700 |
called Cicero, which I've talked about on the show before. 00:20:58.580 |
Cicero can play the game diplomacy, the strategy war game diplomacy very well. 00:21:04.700 |
The way Cicero works is we can actually think about it as a large language model combined 00:21:10.900 |
So diplomacy is a board game, but it has lots of interpersonal negotiation with the other 00:21:16.620 |
The way this diplomacy plane AI system works is the language model, the control logic will 00:21:22.320 |
use the language model to take the conversations happening with the players and explain to 00:21:28.180 |
the control program, the control logic in a very consistent systematic way, what's being 00:21:33.220 |
proposed by the various players in a way that like the control program understands without 00:21:39.680 |
Then the control program simulates lots of possible moves, but what if we did this, right? 00:21:44.260 |
And what it's really doing here is simulating possibilities. 00:21:47.100 |
If this person is lying, like they're trying to, but these two are honest and we do this, 00:21:52.660 |
Well, what if this person was lying, but they're being honest, which move would be best? 00:21:56.820 |
It kind of figures out all these possibilities for what's really happening to figure out 00:22:00.140 |
what play gives it its best chance of being successful. 00:22:03.180 |
And then it tells the language model, okay, here's what we want to do now. 00:22:09.600 |
Give me a message that's in this player that would be convincing to get them to do the 00:22:14.460 |
And the language model actually generates the text that then the control logic sends. 00:22:18.180 |
So in Cicero, we have much more complicated control logic where now we're simulating moves, 00:22:25.440 |
The logic might have multiple queries of the language model to actually implement a turn. 00:22:33.200 |
So Devon AI has been building these agent-based systems to do complicated computer programming 00:22:40.600 |
And the way it works is you give a more complicated computer programming task to the Devon and 00:22:44.740 |
it has control logic that's going to continually talk to a language model to generate code, 00:22:50.400 |
but it can actually keep track of there's multiple steps to this task that we're trying 00:22:58.060 |
All right, let me get some code from the language model that we think does this. 00:23:07.500 |
Okay, we need code that integrates this into this system. 00:23:12.580 |
So again, it's keeping track of a complex plan, the control logic, and using a language 00:23:17.100 |
model as the actual production of a specific code that solves a specific request. 00:23:21.860 |
A language model can't keep track of a long-term plan like this. 00:23:25.100 |
It can't simulate novel futures because again, it's just a token generator. 00:23:31.220 |
This is where a lot of the energy is in AI right now, is these sort of complex control 00:23:35.680 |
The layer that doesn't exist yet, but this is the layer that we speculate about, I call 00:23:41.220 |
And this is where we get closer to something like a general intelligence. 00:23:49.460 |
And this is where, and I'm going to put a question mark, it's unclear exactly how close 00:23:53.380 |
But now we have a very complicated, this is hypothetical, we'd have a very complicated 00:23:57.220 |
control logic that keeps track of intention and state and understanding of the world. 00:24:02.300 |
It might be interacting with many different generative models and recognizers. 00:24:06.480 |
So it has a language model to help understand the world of language and produce texts, but 00:24:13.380 |
If this was a fully actuated, like robotic artificial general intelligence, you would 00:24:17.140 |
have something like visual recognizers that really can do a good job of saying, here's 00:24:24.420 |
It might have some sort of like social intention recognizer where just trained to take recent 00:24:31.660 |
conversations and try to understand what people's intent are. 00:24:35.180 |
And then you have all of this being orchestrated by some master control logic that's trying 00:24:39.020 |
to keep a sort of stateful existence and interaction in the world of some sort of simulated agent. 00:24:44.380 |
So that's how you get to something like artificial general intelligence. 00:24:52.140 |
In all of these layers, the control logic is not self-trained. 00:24:58.020 |
The control logic, unlike a language model, is not something where we just turn on the 00:25:02.060 |
switch and it looks at a lot of data and trains itself and then we have to say, how does this 00:25:08.220 |
At least in the layers that exist so far, layers two through layer zero, the control 00:25:20.100 |
Here's what's something interesting about Cicero. 00:25:22.260 |
In the game Diplomacy, one of the big strategies that's common is lying, right? 00:25:28.260 |
You make an alliance with another player, but you're backstabbing them and you have 00:25:35.500 |
The developers of Cicero were uncomfortable with having their computer program lie to 00:25:40.260 |
So they said, okay, though other people are doing that, our player, Cicero, will not lie. 00:25:46.420 |
That's really easy to do because the control logic where that simulates moves, this is 00:25:49.900 |
not some emergent thing they don't understand. 00:25:57.980 |
So we have this reality about the control plus generative AI combination. 00:26:04.300 |
We have this reality that at least so far, the control is just hand-coded by people to 00:26:15.540 |
There is no way for the intelligence in these cases of the language model, no matter how 00:26:19.780 |
sophisticated its checklist and rules get at being able to produce tokens using very, 00:26:24.060 |
very sophisticated digital contemplations, that cannot control the control logic. 00:26:31.140 |
It can't break through and control the control logic. 00:26:40.260 |
We don't want it to produce versions of us that are smarter. 00:26:41.820 |
We just don't have that coded into the control logic. 00:26:47.860 |
The plugins, there's a lot of control over these things of like, okay, we have gotten 00:26:53.740 |
We've asked for a formatted request, the book of flight from the LLM. 00:26:58.220 |
Let's just look at this because we're not going to spend more than this much money and 00:27:01.340 |
we're not going to fly to places that aren't on this list we think are appropriate places 00:27:07.740 |
The control logic is just programmed right there. 00:27:09.560 |
So I think we've extrapolated the emergent, hard to interpret reality of generative models 00:27:18.280 |
But the control logic in these systems right now is not at all difficult to understand 00:27:31.240 |
One, this doesn't mean that we have nothing to be practically concerned about, but the 00:27:35.840 |
biggest practical concern, especially about layer two or below artificial intelligence 00:27:40.440 |
systems of this architecture is exceptions, right? 00:27:44.760 |
Our control logic didn't think to worry about a particular opportunity. 00:27:49.720 |
We didn't put the right checks and something that is like practically damaging happens. 00:27:57.680 |
Well, for example, we're doing flight booking and our control logic doesn't have a check 00:28:02.160 |
that says make sure the flight doesn't cost more than X and don't book it if it costs 00:28:07.800 |
We forgot to put that check in and the LLM gives us a first class flight on Emirates 00:28:14.880 |
It's like, whoops, we spent a lot of money, right? 00:28:17.160 |
Or we have a Devon type setup where it's giving us a program to run and we don't have a check 00:28:23.920 |
that says make sure that it doesn't use more than its computational resources and that 00:28:28.320 |
program actually is like a giant resource consuming infinite loop and it uses a hundred 00:28:32.800 |
thousand dollars of Amazon cloud time before anyone realizes like what's going on here, 00:28:39.160 |
Like your control logic doesn't check for the right things. 00:28:41.320 |
You can have excessive behaviors, sure, but that's a very different thing than the system 00:28:46.480 |
itself is somehow smarter than we expected or in taking intentional actions that we don't 00:28:56.760 |
Yeah, too, in theory, when we get to layer two, these really complicated control layers, 00:29:02.760 |
in theory, one could imagine hand coding control logic that we completely understand that is 00:29:09.760 |
working with LLMs to produce computer code for a better control logic. 00:29:16.460 |
And it may be then you could get this sort of runaway superintelligence scenario of Nick 00:29:21.340 |
But here's the thing, A, we're nowhere close to being knowing how to do that, how to write 00:29:25.740 |
a control program that can talk to a coding machine like LLMs and get a better version 00:29:31.040 |
There's a lot of CS to be done there that quite frankly, no one's really working on. 00:29:39.020 |
You would have to build a system to do that and then to start executing the new program. 00:29:44.980 |
And so maybe we just don't build those types of systems. 00:29:47.240 |
I call this whole way of thinking about things and I'll write this on here. 00:29:51.260 |
I call this whole way of thinking about things, I'll use a different color text here, IAI, 00:29:58.220 |
right, lowercase I capital AI for intentional artificial intelligence. 00:30:03.300 |
The idea being that there can be tons of intention in the control logic, even if we can't interpret 00:30:07.580 |
very well the generative models like the language models that these control logics use. 00:30:12.540 |
And we should really lean into the control we have in the control logics to make sure 00:30:17.460 |
that this is how we keep sort of predictability on what these systems actually do. 00:30:23.300 |
There might actually be a legislative implication here, one way or the other, making sure that 00:30:28.340 |
we do not develop a legal doctrine that says AI systems are unpredictable. 00:30:32.340 |
So it's not your fault as the developer of an AI system for what it does once actuated. 00:30:39.100 |
That would put a lot of emphasis on this control layers. 00:30:43.860 |
And exactly what we put in these control layers matter, especially once there's actuation, 00:30:52.020 |
The language model can be as smart as we want, but we're gonna be very careful on the actions 00:30:56.380 |
that our control logic is willing to take on its behalf. 00:31:02.540 |
And I do think it is important that we separate the emergent, hard to predict, uninterpretable 00:31:07.420 |
intelligence of self trained generative models, we separate that from the control logics that 00:31:19.460 |
If we go back to our analogy of the giant machine, the Babbage style machine of meshing 00:31:23.620 |
gears and dials, that when you turn it, great sophistication happens inside the machine. 00:31:28.740 |
And at the very end, a word comes out on these dials on the other end of this massive city 00:31:35.820 |
We're not afraid of a machine like that in that analogy. 00:31:39.080 |
We do worry about what the people who are running the machine do with it. 00:31:42.320 |
So that's where we should keep our focus is the people who are actually running the machine, 00:31:47.160 |
you know, what they do should be constrained. 00:31:50.160 |
Don't let them spend money without constraint. 00:31:53.160 |
Don't let them fire missiles without constraint. 00:31:56.240 |
Don't let the control logic have full access to all computational resources. 00:32:01.120 |
Don't let the control logic be able to install an improved version automatically of its own 00:32:07.080 |
We code the control logic, we can tell it what to do and what not to do. 00:32:10.040 |
And let's just make it clear, whatever people do with this big system, like you are a liable, 00:32:15.980 |
the whole systems you build, you're liable for it. 00:32:17.640 |
So you'll be very careful about who you let in in this metaphor to actually turn those 00:32:24.160 |
This is early thinking, just putting it out there for comment, but hopefully it diffuses 00:32:28.420 |
a little bit of the sort of incipient idea that GPT-6 or 7 is going to become HAL. 00:32:34.840 |
What do you think, Jesse, is that sufficiently nerdy? 00:32:41.840 |
What do you think the comments will be for those that think the other way, that don't 00:32:47.800 |
It's interesting, you know, when I first pointed out in my article last year, the language 00:32:54.640 |
It has no state, it has no recursion, it has no interactivity. 00:32:59.200 |
So this is not a mind in any sort of self-aware way. 00:33:01.920 |
What a lot of people came back to me with is like, yeah, yeah, but it's, they were talking 00:33:05.800 |
about, back then they were talking about auto-GPT, which was one of these very early, very early 00:33:11.280 |
Yeah, but people are writing programs that sort of keep track of things outside of the 00:33:16.120 |
language model, and they talk back to the language model, and that's where the sophistication 00:33:31.680 |
Sorry, for those who are listening, I realized that for all the precision of my speech, 00:33:44.480 |
I think some of the more just philosophical thinkers who just sort of tackle these issues 00:33:47.920 |
of like superintelligence from an abstract perspective, like an abstract logical perspective, 00:33:52.640 |
I think their main response will be like, yeah, but all it takes is one person to write 00:33:56.160 |
layer-3 control logic that says write control logic program and then install it, replace 00:34:02.760 |
myself with that program, and like that's what could allow sort of like runaway whatever. 00:34:08.560 |
We don't know how to write a control program. 00:34:10.160 |
If we think of the language model like a coder, we can tell it to write code that does something. 00:34:14.400 |
Very constrained, but we can write this function, write that function. 00:34:18.280 |
That's a very hard problem to sort of work with a language model to produce a different 00:34:27.440 |
It's a hard problem, and there's no reason to write that program, and I think it's not 00:34:30.620 |
just one, you could, again, it's just a very hard problem. 00:34:35.360 |
We don't even know if it's possible to write a significantly smarter control program, or, 00:34:42.560 |
you know, the control program is limited by the intelligence of what the language model 00:34:48.040 |
We don't have any great reason to believe that a language model trained on a bunch of 00:34:53.440 |
existing code, and what it does is predict code that matches the type of things it can 00:34:58.440 |
see, can produce code that is somehow better than any code a human has ever produced. 00:35:04.440 |
We don't know that a language model can do that. 00:35:06.440 |
What it does is it's been trained to try to expand text based on the structures it's seen 00:35:12.160 |
So do we know that even with the right control program? 00:35:14.920 |
So I think that whole thing is more messy than people think, and we're nowhere near 00:35:20.960 |
What I care about mainly is layer zero through two, and layer zero through two, we're in 00:35:25.120 |
I think it's very hypothetical to think about like a control layer that's trying to write 00:35:33.840 |
Eventually the control layer's value is stuck on like what the language model can do, and 00:35:38.360 |
the language model can only do so much, and, you know, there's a lot of interesting debates 00:35:42.040 |
at layer three, but they're also very speculative right now. 00:35:45.720 |
They're not things we're going to stumble into the next six months or so. 00:35:48.640 |
And you went to the OpenAI headquarters like a year ago, right? 00:35:55.920 |
They're worried about just the practicality of how do you actually have a product that 00:35:58.880 |
a hundred million people use around the world. 00:36:00.400 |
That's just like a very complicated software problem. 00:36:04.960 |
And just figuring out all the different things they have to worry about, like there's copyright 00:36:09.640 |
law in this country that like affects this in a way, and it's just, you know, it's just 00:36:14.560 |
Like OpenAI, it's not based on my visit, but based on just listening to interviews with 00:36:18.360 |
Sam Altman recently, they care more right now I think about, for example, getting smaller 00:36:22.960 |
models that can fit on a phone and can be much more responsive. 00:36:26.200 |
I think they see a future in which their models can be a very effective voice interface to 00:36:33.160 |
Like it's very practical what the companies are thinking about. 00:36:35.680 |
This is more the philosophers and the P. Doomers in San Francisco that are thinking about mad 00:36:48.200 |
The control is not emergent, the control we code. 00:36:51.560 |
And that's why I think the core tenet of IAI is if you produce a piece of software, you're 00:37:00.000 |
And that's what's going to keep you very careful about your control layers, like what you allow 00:37:04.280 |
them to do or not do, no matter how smart the language model is that they're talking 00:37:09.360 |
And again, I keep coming back to the language model is inert. 00:37:12.760 |
The control logic can autoregressively keep calling it to get tokens out of it, but it 00:37:18.040 |
The language model is not an intelligence that can sort of take over. 00:37:22.360 |
It's just the giant collection of gears and dials that if you turn long enough, a word 00:37:42.920 |
A lot of them are very techie, so we'll kind of keep this nerd thing going. 00:37:45.160 |
But first I want to briefly talk about one of our sponsors. 00:37:47.720 |
I thought it was appropriate after a discussion of AI to talk about one of our very first 00:37:52.760 |
sponsors who has integrated language model-based AI in a very interesting way into its product. 00:38:03.000 |
Grammarly, quite simply, is an AI writing partner that helps you not only get your work 00:38:11.880 |
96% of Grammarly users report that Grammarly helps them craft more impactful writing. 00:38:18.440 |
It works across over 500,000 apps and websites, right? 00:38:22.160 |
So when you subscribe and use Grammarly, it's there for wherever you're already doing your 00:38:26.680 |
writing, in your word processor, in your email client. 00:38:30.760 |
Grammarly is there to help you make that writing better. 00:38:34.400 |
The ways it can do this now continue to expand. 00:38:37.520 |
So it's not just, hey, you said this wrong, or here's a more grammatically correct way 00:38:42.200 |
It can now do sophisticated things, for example, like tone detection. 00:38:47.680 |
Can you rewrite what I just wrote to sound more professional, to sound more friendly, 00:39:01.880 |
Can you take this outline and write like a draft of a summary of these points, right? 00:39:05.820 |
So it can generate, not just correct or rewrite, but generate in ways that as you get more 00:39:12.380 |
And again, the key thing with Grammarly is that it integrates into all these other tools. 00:39:15.660 |
You're not just over at some separate website typing into a chat interface, like Grammarly 00:39:25.140 |
It's the gold standard of responsible AI in the sense that they have for 15 years, have 00:39:29.900 |
best in class communication trusted by tens of millions of professionals in IT development. 00:39:34.800 |
It is a secure AI writing partner that's going to make you or your team make their point 00:39:39.020 |
better, write more clearly and get your work done more faster. 00:39:42.780 |
So get AI writing support that works where you work. 00:39:46.660 |
Sign up and download for free at Grammarly.com/podcast, that's G-R-A-M-M-A-R-L-Y.com/podcast, easier 00:40:01.100 |
Now wherever it is you happen to be doing this work, you want to feel comfortable while 00:40:05.100 |
So I want to talk about our friends at Roan and in particular their commuter collection, 00:40:11.060 |
the most comfortable, breathable and flexible set of products known to man. 00:40:15.500 |
The commuter collection has clothing for any situation from the world's most comfortable 00:40:21.100 |
pants to dress shirts to quarter zips and polos so you never have to worry about what 00:40:24.340 |
to wear when you have the commuter collection. 00:40:27.680 |
It's four way stretch fabric makes it very flexible, it's lightweight, it's very breathable. 00:40:32.860 |
It even has gold fusion anti-odor technology and wrinkle release technology so you can 00:40:37.500 |
travel with this as you wear it, the wrinkles go away. 00:40:40.500 |
It's very useful if you're on like a, you have an active day or you're on a trip, you 00:40:44.820 |
can just throw this on and be speaking at conferences or teaching classes or on let's 00:40:54.620 |
And having the commuter collection means you're going to look good but it's going to be breathable, 00:40:57.620 |
you're not going to overheat, it's not going to look wrinkled, it's going to whisk away 00:41:02.820 |
It's going to look fantastic if you are active. 00:41:06.700 |
So the commuter collection can get you through any workday and straight into whatever comes 00:41:11.340 |
Head to rhone.com/cow and use that promo code cow to save 20% off your entire order. 00:41:18.900 |
That's 20% off your entire order when you head to rhone.com/cow and use that code cow 00:41:26.580 |
when you check out, it's time to find your corner offer office comfort. 00:41:38.100 |
You often give advice on methods to consume news. 00:41:40.940 |
With the advent of chat GPT and other tools, should I be worried about the spread of disinformation 00:41:50.980 |
So when people are trying to say what are we worried about with these large language 00:41:54.160 |
models that are good at generating text, one of the big concerns is you could use it to 00:42:00.740 |
Generate text that's false, but people might believe, and of course it could then therefore 00:42:06.160 |
be used equally for disinformation where you're doing that for particular purposes. 00:42:09.340 |
I want to influence the way people think about it. 00:42:15.660 |
I think in the general sense, I'm not as worried and let me explain why. 00:42:21.500 |
What do you need for, let's just call it negative, high impact negative information. 00:42:27.220 |
What do you need for these type of high impact negative information events? 00:42:30.020 |
Well, you need a combination of two things, a tool that is really good at engendering 00:42:35.100 |
viral spread of information that hits just like the right combination of stickiness. 00:42:41.580 |
And you need a pool of this sort of available negative information that's potentially viral. 00:42:46.740 |
So you have this big pool and then a selection algorithm on that pool that can find the thing 00:42:52.580 |
That's what allows us to be in our current age of sort of widespread mis or disinformation 00:42:57.060 |
is that there's a lot of information out there. 00:42:59.500 |
And because in particular of social media curation algorithms, which are engagement 00:43:03.740 |
focused, this tool exists that's basically surveying this pool of potential viral spreading 00:43:08.860 |
information that can take this negative information and expand it everywhere, right? 00:43:15.340 |
That's what makes our current moment different than, say, like 25 years ago, where the viral 00:43:21.540 |
So it could be a lot of people with either malintended or just wrong and they don't realize 00:43:26.660 |
it thoughts, you know, hey, I think the earth is flat. 00:43:31.460 |
But when we added the viral spread potential of recommendation algorithms in the social 00:43:35.840 |
media world, we got this current moment where mis or disinformation has the potential spreading 00:43:42.340 |
So what is generative AI change in this equation? 00:43:45.220 |
It makes the pool of available bad information bigger. 00:43:48.340 |
It is easier to generate information about whatever you want. 00:43:53.420 |
For most topics we care about, that doesn't matter, right? 00:43:58.300 |
Because what matters only is if AI can create content in this pool that is stickier than 00:44:05.620 |
There's only so many things that can spread and have a big impact. 00:44:09.140 |
And it's going to be the stickiest, the perfectly calibrated things that get identified by these 00:44:14.620 |
If large language models are just generating a lot of mediocre, bad information, that doesn't 00:44:20.260 |
Probably the stickiest stuff, the stuff that's going to spread best in the small number of 00:44:23.780 |
slots that each of our attention has to be impacted, it's going to be like very carefully 00:44:28.220 |
Like I really have a sense of like, this is going to work and we already have enough of 00:44:33.580 |
And most of our slots of ideas that can impact us are filled. 00:44:38.580 |
The exception to this would be very niche topics for that pool of potential bad information 00:44:45.300 |
It's just nothing that there's no information about it. 00:44:47.900 |
That's the case where language models could come into play because if that pool is empty, 00:44:51.860 |
because it's a very specific topic, like this election in this like county, you know, it's 00:44:59.140 |
not something that people are writing a lot about. 00:45:01.900 |
Now someone can come in who otherwise maybe before, because they didn't have like the 00:45:05.500 |
language skills, wouldn't be able to produce any text that could get virally spread here, 00:45:13.460 |
The stickiest things spread, but if the pool is empty, almost anything reasonable you produce 00:45:20.000 |
So that's the impact I see most immediately of missing disinformation in large language 00:45:24.620 |
models is hyper-targeted mis- or disinformation. 00:45:28.920 |
When it comes to big things like a national election or the way we're thinking about a 00:45:33.460 |
pandemic or conspiracies about major figures or something like this, there's already a 00:45:39.540 |
bunch of information adding more mediocre, bad information is not gonna change the equation. 00:45:44.780 |
But in these narrow instances, that's where we have to be more wary about it. 00:45:48.420 |
Unfortunately, like the right solution here is probably the same solution that we've been 00:45:52.980 |
promoting for the last 15 years, which is increased internet literacy. 00:45:57.600 |
Just we keep having to update what by default we trust or don't trust. 00:46:01.900 |
We have to keep updating that sort of sophisticated understanding of information. 00:46:05.420 |
But again, it's not changing significantly what's possible. 00:46:10.400 |
It's just, it's allowing, it's simplifying the act of producing this sort of bad information 00:46:14.860 |
of which there's already a lot of it that already exists. 00:46:22.020 |
Is the lack of good measurement and evaluation for AI systems a major problem? 00:46:26.740 |
Many AI companies use vague phrases like improved capabilities to describe how their models 00:46:34.020 |
As most tech companies don't publish detailed release notes, how do I know what changes? 00:46:38.700 |
- Yeah, I mean, it's a problem right now in this current age of what is happening is like 00:46:49.900 |
This is not however the long-term business model of these AI companies. 00:46:52.700 |
So the mega Oracle models, think of this as the chat GPT model. 00:46:56.460 |
Think about this as the clod model, where you have a Oracle that you talk to through 00:47:02.500 |
a chat bot about anything and you ask it to do anything and it can do whatever you ask 00:47:07.500 |
And so we build these huge models, GPT-3 went to GPT-35, which went to GPT-4, which 00:47:12.860 |
went to GPT, whatever it is, 4S or 5S or whatever they're calling it. 00:47:16.620 |
And you are absolutely right, Alyssa, it's not always really clear what's different, 00:47:23.740 |
Often that's discovered, like, I don't know, we trained this on 5X more parameters. 00:47:28.540 |
Now let's just go mess around with it and see what it does better than the last one. 00:47:31.820 |
So the sort of the release notes are emergently created in a distributed fashion over time. 00:47:37.380 |
But it's not the future of these companies because it's not very profitable to have these 00:47:41.780 |
massive right now, the biggest models, like a trillion parameter, Sam Altman's talking 00:47:46.020 |
about a potential 10 trillion parameter model. 00:47:49.580 |
This is something that's going to cost on the orders of multiple hundreds of millions 00:47:56.140 |
They're computationally very expensive to train. 00:47:58.340 |
They're computationally very expensive to run, right? 00:48:02.040 |
It's like having a Bugatti supercar to drop your kids off at school five blocks away, 00:48:07.880 |
you know, to be using a trillion or 10 trillion parameter model to, you know, do a summary 00:48:14.120 |
of this page that you got on a Google search is just way over provisioned and it's costing 00:48:19.960 |
It's a lot of computational resources, it's expensive. 00:48:22.680 |
What they want, of course, is smaller customized models to do specific things. 00:48:30.520 |
Computer programmers have an interface to a language model built right into their integrated 00:48:36.520 |
So they can just right there where they're coding, ask for a code to be finished or another 00:48:42.480 |
function to be added or ask it, what is the library that does this? 00:48:47.440 |
And it will come back like, this is the library you mean, and here's the description. 00:48:52.680 |
Microsoft Copilot, which again is confusingly named in an overlapping way, is trying to 00:48:58.040 |
do something similar with Microsoft Office tools. 00:49:01.020 |
You kind of have this universal chat interface to ask for actuated help with their Microsoft 00:49:10.560 |
And it's going to work back and forth using layer one control with those products. 00:49:17.080 |
Again, OpenAI has this dream of having a better, like a voice interface to lots of different 00:49:22.840 |
Apple Intelligence, which they've just added to their products is, you know, they're using 00:49:27.360 |
chat GPT as a backend to sort of more directly deal with specific things you're doing on 00:49:33.280 |
Like, can you take a recording of this phone conversation I just had and get a transcript 00:49:39.280 |
of it and summarize that transcript of it and email it to me? 00:49:41.960 |
So this is where these tools are going to get more interesting when they're doing specific, 00:49:47.840 |
So they're actually like taking action on your behalf, you know, in typically the digital 00:49:57.440 |
Okay, it can summarize phone calls, it can produce computer code, it can help me do formatting 00:50:05.000 |
So I think as these models get more specialized and actuated and integrated into specific 00:50:09.400 |
things we're already doing in our digital lives, the capabilities will be much more 00:50:14.560 |
This current era of just, we all go to chat.openai.com and like, what can this thing do now? 00:50:21.040 |
This is really just about, it's the equivalent of the car company having the Formula One 00:50:28.240 |
They're not planning to sell Formula One racers to a lot of people. 00:50:32.220 |
But if they have a really good Formula One race car, people think about them as being 00:50:35.680 |
a really good car company and so then they buy the car that's actually meant for their 00:50:40.840 |
And so I think that's what these big models are right now. 00:50:43.280 |
The bespoke models, their capabilities I think will be more clearly enumerated. 00:50:47.800 |
And that's where we're going to begin to see more disruptions. 00:50:50.600 |
I mean, notice we're at the year and a half mark of the chat GPT breakthrough, hasn't 00:50:57.880 |
The chat interface to a large language model, it's really cool what they can do, but right 00:51:03.360 |
away they were talking about imminent disruptions to major industries. 00:51:06.440 |
And we're still playing this game of like, well, I heard about this company over here 00:51:11.320 |
who their neighbor's cousin replaced six of their customer service representatives. 00:51:16.240 |
Like we're sort of still in that sort of passing along like a small number of examples. 00:51:22.560 |
Because I don't think these models are in the final form in which they're going to have 00:51:26.120 |
They haven't found their, if we can use a biological metaphor, the viral vector that's 00:51:31.880 |
actually able to propagate really effectively. 00:51:37.480 |
And I think their capabilities will be much more clearly enumerated when we're actually 00:51:41.280 |
using them much more integrated into our daily workflow. 00:51:47.280 |
So Microsoft is calling their Microsoft office integration co-pilot as well. 00:51:56.440 |
Is the development of AI the biggest thing that happened in technology since the internet? 00:52:03.320 |
I mean, what are the disruptions of the last 40 years? 00:52:06.960 |
Personal computing, number one, because that's what actually made computing capable of being 00:52:14.400 |
Next was the internet, democratized information and information flows, made that basically 00:52:21.280 |
After that came mobile computing slash the rise of a mobile computing assisted digital 00:52:29.640 |
So this idea that the computing was portable and that the main use, the main economic engine 00:52:36.120 |
of these portable computing devices would be monetizing attention, hugely disruptive 00:52:40.900 |
on just like the day-to-day pattern of what our life is like. 00:52:46.760 |
The other big one that's lurking, of course, I think is augmented reality and the rise 00:52:50.300 |
of virtual screens over actual physical screens that you hold in real life. 00:52:55.520 |
That's going to be less disruptive for our everyday life because that's going to be simulating 00:52:58.960 |
something we're doing now in a way that's better for the companies. 00:53:01.640 |
But the whole goal will be just to kind of take what we're doing now and make it virtual. 00:53:06.000 |
But that's going to be hugely economically disruptive because so much of the hardware 00:53:09.640 |
technology market is based on building very sleek individual physical devices. 00:53:14.280 |
So I think that and AI are vying to be like, what's going to be the next biggest disruption. 00:53:19.880 |
How big will it be compared to those prior disruptions? 00:53:26.160 |
On one end of the spectrum, it's going to be, you know, there's places where it has 00:53:33.120 |
a part of our daily life where it wasn't there before. 00:53:38.560 |
Email really changed the patterns of work, but didn't really change what work was. 00:53:42.320 |
On the other end of the spectrum, it could be much more comprehensive, maybe something 00:53:45.740 |
like personal computing, which just sort of changed how the economy operated. 00:53:51.480 |
You know, pre-computers, after computers fundamentally just changed the way that we interact with 00:53:59.600 |
Of course, there's the off-spectrum options as well as like, no, no, it like comes alive 00:54:04.040 |
and completely, it's so smart that it either takes over the world or it just takes over 00:54:12.140 |
I tend to call those off-spectrum because of what I talked about in the deep dive. 00:54:15.720 |
Like we just, I don't see us having the control logic to do that yet. 00:54:20.400 |
So I think really the spectrum is like personal computer on one end, email on the other. 00:54:24.480 |
I don't really know where it's going to fall, but I do go back to saying the current form 00:54:28.560 |
factor, I think we have to admit this, the current form factor of generative AI talking 00:54:34.040 |
to a chat interface through a web or phone app has been largely a failure to cause the 00:54:42.880 |
There's heavy users of it who like it, but it really has a novelty feel. 00:54:46.600 |
They'll really get into detail about these really specific ways that they're, I'm getting 00:54:50.760 |
ideas for my articles and having these interactions with it, but it really does have that sort 00:54:54.660 |
of early internet novelty feel where you had the mosaic browser and you're like, this is 00:54:59.240 |
really cool, but most people aren't using it yet. 00:55:01.680 |
It's going to have to be another form factor before we see its full disruptive potential. 00:55:05.320 |
And I think we do have to admit most things have not been changed. 00:55:09.960 |
We're very impressed by it, but we're not impressed by its footprint on our daily life 00:55:15.640 |
So I guess this is like a dot, dot, dot, stay tuned. 00:55:19.680 |
Unless your students just use it to put pass in papers, right? 00:55:24.680 |
So look, I have a New Yorker article I'm writing on that topic that's still in editing. 00:55:30.400 |
But the picture about what's happening with students and paper ride in AI, that's also 00:55:37.080 |
What's going on there might not be what you really think, but I'll hold that discussion 00:55:40.800 |
until my next New Yorker piece on this comes out. 00:55:47.200 |
How do I balance a 30 day declutter with my overall technology use? 00:55:51.080 |
I'm a freelance remote worker that uses Slack, online search, stuff like that. 00:55:56.800 |
So Dipta, when talking about the 30 day declutter, is referencing an idea from my book, Digital 00:56:02.560 |
Minimalism, where I suggest spending 30 days not using personal, optional personal technologies, 00:56:09.400 |
get reacquainted with what you care about and other activities that are valuable. 00:56:12.500 |
And then in the end, only add back things that you have a really clear case of value. 00:56:16.360 |
But Dipta is mentioning here, work stuff, right? 00:56:21.040 |
She's a freelance worker, use Slack, use online search, et cetera. 00:56:25.080 |
My book, Digital Minimalism, which has the declutter is a book about technology in your 00:56:30.600 |
It's not about technology at work, deep work, a world without email and slow productivity. 00:56:36.760 |
Those books really tackle the impact of technology on the workplace and what to do about it. 00:56:41.480 |
So digital knowledge work is one of the main topics that I'm known for. 00:56:45.480 |
It's why I'm often cast, I think, somewhat incorrectly as a productivity expert. 00:56:49.840 |
I'm much more of a like, how do we actually do work and not drown and hate our jobs in 00:56:57.520 |
And it looks like productivity advice, but it's really like survival advice. 00:57:00.880 |
How do we do work in an age of email and Slack without going insane? 00:57:06.160 |
That was my book where I said, hey, I acknowledged there's this other thing going on, which is 00:57:11.440 |
like, we're looking at our phones all the time in work, outside of work, unrelated to 00:57:21.440 |
So digital declutter is what to do with the technology in your personal life. 00:57:26.360 |
When it comes to the communication technologies in your work life, read a world without email, 00:57:35.240 |
So I'll just use that as a roadmap for people who are struggling with the promises and peril 00:57:41.280 |
Use my minimalism book for like the phone, the stuff you're doing on your phone that's 00:57:45.960 |
My other books will be more useful for what's happening in your professional life. 00:57:54.280 |
I think in part because the symptoms are similar. 00:57:59.280 |
I look at social media on my phone all the time more than I want to. 00:58:03.040 |
I look at email on my computer at work all the time more than I want to. 00:58:07.320 |
These feel like similar problems and the symptoms are similar. 00:58:10.780 |
I am distracted in some sort of abstract way from things that are more important, but the 00:58:17.300 |
But you're looking at your phone too much and social media too much because these massive, 00:58:21.280 |
massive attention economy conglomerates are producing apps to try to generate exactly 00:58:25.240 |
that response from you to monetize your attention. 00:58:27.880 |
You're looking at your email so much, not because someone makes money if you look at 00:58:30.560 |
your email more often, but because we have evolved this hyperactive hive mind style of 00:58:36.400 |
on-demand digital aided collaboration in the workplace, which is very convenient in the 00:58:40.840 |
moment, but just fries our brain in the long term. 00:58:43.280 |
We have to keep checking our email because we have 15 ongoing back and forth timely conversations 00:58:47.680 |
and the only way to keep those balls flying in the air is to make sure I see each response 00:58:51.540 |
in time to respond in time so that things can keep unfolding in a timely fashion. 00:58:55.400 |
It's a completely different cause and therefore the responses are different. 00:58:59.380 |
So if you want to not be so caught up in the attention economy in your phone and in your 00:59:02.800 |
personal life, well, the responses there have a lot to do with like personal autonomy, figuring 00:59:08.040 |
out what's valuable, making decisions about what you use and don't use. 00:59:10.960 |
In the workplace, it's all about replacing this collaboration style with other collaboration 00:59:14.940 |
styles that are less communication dependent. 00:59:16.840 |
So it's similar causes, but very different, I mean, similar symptoms, but very different 00:59:25.040 |
So I sold digital minimalism and a world without email together. 00:59:29.680 |
It was a two book deal, like I'm going to write one and then the other. 00:59:39.360 |
One of the editors was like, this is the, which was an interesting point, but I think 00:59:46.400 |
He's like, these are the, this is the same thing. 00:59:47.960 |
We're just like looking at stuff too much in our, in our, uh, digital lives. 00:59:55.020 |
And I was really clear, like, no, they shouldn't because actually it confuses the matter because 01:00:00.160 |
they already seem so similar, but it's so different. 01:00:03.480 |
A world without email and slow productivity are such different books than digital minimalism. 01:00:08.400 |
The causes are so different and the responses are so different that they can't be one book. 01:00:14.640 |
It's, it's, it's like two fully separate issues. 01:00:16.880 |
The only thing to commonality is they involve screens and they involve looking at the screens 01:00:21.820 |
And so I was like, I think you're wrong about that. 01:00:25.800 |
Other little known fact about that, it was originally supposed to be the other order. 01:00:29.640 |
The slope, uh, a world without email was the direct followup to deep work was the idea, 01:00:35.360 |
but the issues in digital minimalism became so pressing so quickly that I say, no, no, 01:00:42.600 |
And so that's why slope, um, a world without email did not directly follow deep work is 01:00:47.920 |
because in 2017 and 18, these issues surrounding our phone and social media, mobile, like that's 01:00:55.980 |
When you were writing deep work, did you know you were going to write a world without email 01:01:04.080 |
And then after I wrote deep work, I was thinking about what to write next. 01:01:06.560 |
And the very next idea I had was ruled without email. 01:01:08.960 |
And it was basically a response to the question of like, well, why is it so hard to do deep 01:01:14.960 |
In the book, deep work, I don't get too much into it. 01:01:17.960 |
Um, I'm not going to get into why we're in this place. 01:01:22.120 |
I just want to emphasize focus is diminishing, but it's important and here's how you can 01:01:27.400 |
And then I got more into it after that book was written. 01:01:31.200 |
And it was a pretty complicated question, right? 01:01:33.240 |
Like why did we get to this place where, uh, we check email 150 times a day? 01:01:41.800 |
So it was its own sort of like Epic investigation. 01:01:45.800 |
Um, yeah, it didn't sell the same as like digital minimalism or deep work because it's 01:01:49.840 |
less just let me give you this image of a lifestyle that you can shift to right now. 01:01:56.800 |
It's much more, how did we end up in this place? 01:02:00.960 |
It's much more of like social professional commentary. 01:02:02.760 |
I mean, it has solutions, but they're more systemic. 01:02:05.600 |
There's no easy thing you can do as an individual. 01:02:07.920 |
I think intellectually it's a very important book and it's had influence that way, but 01:02:11.800 |
it's hard to make a book like that be like a million copy seller. 01:02:17.680 |
Atomic habits is easier to read than a world without email. 01:02:32.120 |
Do we play the music before we asked a question or do we play the music after? 01:02:52.800 |
I work at a large tech company as a software engineer and I'm starting to feel really overwhelmed 01:02:57.280 |
by the number of projects getting thrown at us. 01:02:59.720 |
How do I convince my team that we should say no to more progress projects when everyone 01:03:09.600 |
So this is a great question for the corner because the whole point of the slow productivity 01:03:13.240 |
corner segment is that we ask a question that's relevant to my book, slow productivity, which 01:03:19.120 |
as we announced the beginning of the show, the number one business book of 2024 so far 01:03:26.780 |
Because I have an answer that comes straight from the book. 01:03:29.560 |
So in chapter three of slow productivity, where I talk about the principle of doing 01:03:36.360 |
fewer things, I have a case study that I think is very relevant to what you should, your 01:03:42.760 |
So this case study comes from the technology group at the Brood Institute, a joint Harvard 01:03:48.720 |
and MIT Brood Institute in Cambridge, Massachusetts. 01:03:52.480 |
This is like a large sort of interdisciplinary genomics research Institute that has all these 01:03:59.720 |
But I give a profile of a team that worked at this Institute. 01:04:05.720 |
It was basically, it's not the IT team, but it's a team that like what they do is they 01:04:09.380 |
build tech stuff that other scientists and people in the Institute need. 01:04:13.200 |
So you come to this team and they're like, Hey, could you build us a tool to do this? 01:04:16.360 |
It's a bunch of programmers and they'll let's do this, let's build that. 01:04:20.200 |
They had a very similar problem as what you're describing, Hanzo. 01:04:28.040 |
Some of them would be suggested by other stakeholders, you know, other scientists or teams in the 01:04:33.920 |
And they'd be like, okay, let's work on this. 01:04:39.000 |
And people are getting overloaded with all these projects and just things were getting 01:04:43.000 |
I mean, it's the classic idea from this chapter of the book is that if you're working on too 01:04:46.720 |
many things at the same time, nothing makes progress. 01:04:50.520 |
You put too many logs down the river, you get a log jam. 01:04:57.780 |
So what they did is they went to a relatively simple pull based agile inspired project management 01:05:03.680 |
workload system where whenever an idea came up, here's a project we should do. 01:05:09.900 |
They put it on an index card and they put it on the wall and they had a whole section 01:05:13.560 |
of the wall for like things we should, or at least consider working on. 01:05:18.080 |
Then they had a column on the wall for each of the programmers. 01:05:22.440 |
The things that each programmer were working on were put under their name. 01:05:26.240 |
So now you have like a really clear workload management thing happening. 01:05:29.560 |
If you had four or five cards under your name, they're like, this is crazy. 01:05:36.340 |
You should just do one or two things at a time. 01:05:37.560 |
And when you're done, we can decide as a team, okay, there's now space here for us to pull 01:05:45.600 |
And as a team, you could look at this big collection on the wall of stuff that you've 01:05:48.800 |
identified or has been proposed to you and say, which of these things is most important. 01:05:53.440 |
Equally important here as well is during this process of selecting what you're going to 01:05:57.920 |
work on next, because everyone is here, it's a good time to say, well, what do I need to 01:06:08.280 |
You sort of build your contract for execution. 01:06:11.100 |
So one of the things they did here is, okay, so first of all, this prevented overload. 01:06:15.220 |
Each individual person can only have a couple of things in their column. 01:06:17.400 |
So you didn't have people working on too many things at once. 01:06:21.780 |
But number two, this reminds me of your question, Hanzo. 01:06:25.560 |
They noted that this also made it easier for them to, over time, weed out projects that 01:06:32.200 |
they might've at some point been excited about, but are no longer excited about to weed those 01:06:41.000 |
And the way they did it was they would say, this thing has been sitting over here in this 01:06:46.400 |
This has been sitting over there for months, and we're consistently not pulling it onto 01:06:53.600 |
And so this allowed them to get past that trap of momentary enthusiasm. 01:07:00.340 |
We have those enthusiasms all the time, because here, that would just put something on the 01:07:04.560 |
But if it didn't get pulled over after a month or so, they would take it off the wall. 01:07:07.560 |
So they had a way of sort of filtering through which projects should we actually work on. 01:07:16.720 |
We can't just push things on people's plates in an obfuscated way and just sort of try 01:07:23.800 |
Things need to exist separate from individuals' obligations. 01:07:28.160 |
And then we need to be very clear about how many things each individual should work on 01:07:31.880 |
So, Hanzo, you need some version of this sort of vaguely Kanban Agile-style workload management 01:07:41.520 |
Read the case study in chapter three of Slow Predictivity to get details. 01:07:44.960 |
That will point you towards a paper from the Harvard Business Review that does an even 01:07:51.760 |
Send that around to your team or send my chapter around to your team. 01:07:58.120 |
And I think your team's going to work much better. 01:08:19.480 |
For the last couple of episodes, you've been talking about applying the distributed trust 01:08:27.000 |
There's a lot that I like about that, but I'd like to hear you evaluate that thought 01:08:32.600 |
in light of Fogg's behavioral model, which says that for an action to take place, motivation, 01:08:42.160 |
I don't see a problem with ability, but I'm wondering about the other two. 01:08:46.320 |
So if someone wants to follow, say, five creators, they're going to need significant motivation 01:08:55.600 |
to be checking those sources when they're not curated in one place. 01:09:00.560 |
Secondly, what is going to prompt them to go look at those five sources? 01:09:06.800 |
I think if those two things can be solved, this has a real chance. 01:09:10.640 |
One last unrelated note, somebody was asking about reading news articles. 01:09:15.360 |
I use Send to Kindle, and I send them my Kindle and read them later. 01:09:23.720 |
So I think what's key here is separating discovery from consumption. 01:09:28.320 |
So the consumption problem is once I've discovered, let's say, a creator that I'm interested in, 01:09:34.560 |
you know, how do I then consume that person's information in a way that's not going to be 01:09:43.400 |
So if there's a bunch of different people I've discovered one way or the other, put 01:09:46.460 |
aside how I do that, how do I consume their information? 01:09:49.440 |
That's the consumption problem, and that's fine. 01:09:55.800 |
If I discovered a syndicated blog that I enjoyed, I would subscribe to it. 01:10:04.480 |
Then that person's content is added to this sort of common list of content in my RSS reader. 01:10:10.600 |
This is what, for example, we currently do with podcast. 01:10:16.080 |
The RSS feeds now are describing podcast episodes and not blog posts, but it's the exact same 01:10:25.040 |
So when you have a podcast, you host your MP3 files on whatever server you want to. 01:10:31.640 |
It's not a centralized model like Facebook or like Instagram, where everything is stored 01:10:39.880 |
on the servers of a single company that makes sense of all of it and helps you discover 01:10:44.280 |
No, we have our servers on, our podcast are on Buzzsprout server somewhere, right? 01:10:48.520 |
It's just a company that does nothing but host podcast. 01:10:50.880 |
We could have our podcast, like in the old days of podcast, on a Mac studio in our HQ. 01:10:58.720 |
But what you do is you have an RSS feed that every time you put out a new episode, you 01:11:02.640 |
update that feed to say, here's the new episode. 01:11:10.760 |
All a podcast listener is, like a podcast app, is an RSS reader. 01:11:18.020 |
When it sees there's a new episode of a show because that RSS feed was updated, it can 01:11:24.980 |
It can go and retrieve the MP3 file from whatever server you happen to be serving it on, and 01:11:33.800 |
We get very nice interfaces for where do I pull together and read in a very nice way 01:11:39.360 |
or listen in a very nice way or watch in a very nice way. 01:11:43.520 |
Because by the way, I think video RSS is going to be a big thing that's coming. 01:11:51.000 |
Okay, well, how do I find the things that subscribe to in the first place? 01:11:54.000 |
This is where distributed trust comes into play. 01:11:55.860 |
It's the way we used to do this pre-major social media platforms. 01:12:02.520 |
Well, typically it would be through these distributed webs of trust. 01:12:13.120 |
I liked what I saw over there, and so now I'm going to subscribe to that person. 01:12:18.260 |
Or three or four people that I trust are in my existing web of trust have mentioned this 01:12:26.080 |
That now builds up this human to human curation, this human to human capital of this is a person 01:12:33.720 |
So now I will go and discover them, and I like what I see, and then I subscribe, and 01:12:38.760 |
So we've got to break apart discovery and consumption. 01:12:42.620 |
It's the moving discovery away from algorithms and back towards distributed webs of trust. 01:12:51.460 |
That's where we get rid of this feedback cycle of production, recommendation algorithm, feedback 01:12:59.940 |
to producers about how popular something was, which changes how they produce things into 01:13:07.820 |
That cycle is what creates this sort of hyper palatable, lowest common denominator, amygdala 01:13:17.940 |
You get rid of the recommendation algorithm piece of that, that goes away. 01:13:21.940 |
It also solves problems about disinformation and misinformation. 01:13:24.940 |
I mean, I argued this early in the COVID pandemic. 01:13:29.180 |
I wrote this op-ed for Wired, where I said like the biggest thing we could do for both 01:13:34.620 |
the physical and mental health of the country right now would be to shut down Twitter. 01:13:38.220 |
I said what we should do instead is go back to an older web two model, where information 01:13:43.860 |
was posted on websites, like blogs and articles posted on websites, and yeah, it's going to 01:13:48.060 |
be higher friction to sort of discover which of these sites you trust. 01:13:52.740 |
But this distributed web of trust is going to make it much easier for people to curate 01:13:59.420 |
Like this blog here is being hosted by a center of a major university. 01:14:06.340 |
I have all of this capital in me trusting that more than trusting johnnybananas.com/covidconspiracies. 01:14:18.820 |
Or I'm going to have to follow old-fashioned webs of trust to find my way to sort of like 01:14:25.500 |
And this is not really an argument for, yeah, but you're going to fall back to unquestioning 01:14:29.780 |
Webs of trust work very well for independent voices. 01:14:35.100 |
They're very useful for critiques of major voices. 01:14:38.100 |
It is slower for people to find independence or critical voices. 01:14:43.040 |
But if you find them through a web of trust, they're much more powerful and it filters 01:14:48.000 |
out the crank stuff, which is really bad for independent and critical voices because it 01:14:55.420 |
This person here critiquing this policy, that's the same as this other person over here who 01:15:01.700 |
Webs of trust, I think, are a very effective way to navigate information in a low friction 01:15:08.340 |
So I think distributed webs of trust, I really love that model. 01:15:15.940 |
So this is not like a model that is retroactive or reactionary. 01:15:22.140 |
It's not let's go back to some simpler technological age to try to get some... 01:15:27.500 |
We're doing it right now in some sectors of online content and it's working great. 01:15:36.700 |
Algorithms don't show us what podcast to listen to. 01:15:39.220 |
They don't spread virally and then we're just shown it and it catches our attention. 01:15:43.820 |
We probably have to hear about it multiple times from people we trust before we go over 01:15:53.460 |
It's a vibrant online content community right now. 01:15:56.140 |
How do people discover new email newsletters? 01:15:58.860 |
People they know forward them individual email newsletters like, "You might like this." 01:16:05.780 |
And they read it and they say, "I do and I trust you and so now I'm going to consider 01:16:15.140 |
It's not an algorithm as much as Substack is trying to get into the game of algorithmic 01:16:19.380 |
recommendation or be like the Netflix of text. 01:16:25.900 |
I like to think of the giant monopoly platform social media age as this aberration, this 01:16:31.220 |
weird divergence of the ultimate trajectory of the internet as a source of good. 01:16:37.220 |
And the right way to move forward on that trajectory is to continually move away from 01:16:40.700 |
the age of recommendation algorithms in the user-generated content space and return more 01:16:48.080 |
Recommendation algorithms themselves, these are useful, but I think they're more useful 01:16:52.500 |
when we put them in an environment where we don't have the user-generated content and 01:17:00.980 |
"Hey, you might like this show if you like that other show." 01:17:06.960 |
They're very useful Amazon to say, "This book is something you might like if you like that 01:17:12.460 |
I'm happy for you to have recommendation algorithms in those contexts. 01:17:14.940 |
But if you hook them up with user-generated content and then feedback to the users about 01:17:18.740 |
popularity, that's what in a Marshall McLuhan way sort of evolves the content itself in 01:17:24.860 |
the ways that are, I think, undesirable and as we see have really negative externalities. 01:17:28.700 |
So anyways, we've gone from geeking out on AI to geeking out on my other major topic, 01:17:35.380 |
But I think that is the way to discover information. 01:17:38.380 |
Hopefully that's the future of the internet as well. 01:17:40.460 |
And I love your idea, by the way, of the Send a Kindle cool app. 01:17:44.740 |
You send articles to your Kindle, and then you can go take that Kindle somewhere outside 01:17:51.300 |
No ads, no links, no rabbit holes, no social media. 01:18:00.820 |
This is where people send in a description of using some of my ideas out there in the 01:18:07.820 |
Are we been asking people to send these to you, Jesse? 01:18:14.260 |
So if you have a case study of putting any of these ideas into action, send those to 01:18:16.260 |
If you want to submit questions or calls, just go to thedeeplife.com/listen. 01:18:20.980 |
And there's also a section in there if they go to that website where they can put in a 01:18:27.200 |
And we have links there for submitting questions. 01:18:28.200 |
We have a link there where you can record a call straight from your phone or browser. 01:18:31.700 |
Our next case study comes from Salim who says, "I work at a large healthcare IT software 01:18:40.380 |
Our work is client-based, so we'll always work with the same analyst teams as our assigned 01:18:45.820 |
While I enjoy the core work, which is problem-solving based, I was struggling with a large client 01:18:50.640 |
load and specifically with one organization that did not align well with my communication 01:18:57.540 |
This was a constant problem in my quarterly feedback, and I was struggling with convincing 01:19:04.620 |
Around this time, our division had recently rolled out a work plan site for employees 01:19:11.220 |
The issue here was that it was communicated as a requirement. 01:19:14.620 |
So most of us saw this as upper micromanagement. 01:19:18.020 |
The site itself is also unstructured, so we didn't see the utility in doing this since 01:19:21.820 |
we already log our time retroactively anyways. 01:19:24.980 |
At this point, I had already read Deep Work and was using the time block planner, but 01:19:29.300 |
was lacking a system for planning at a weekly timescale. 01:19:33.740 |
This is where I started leveraging our work plan site and structured it in terms of what 01:19:40.540 |
This included itemizing my recurring calls, office hours with clients, and a general estimate 01:19:45.620 |
of how much time I would spend on client work per client. 01:19:48.820 |
I incorporated sections for a top priority list and a pull list backlog so I could quickly 01:19:52.980 |
go in and reprioritize as new ideas came in or as I had some free time. 01:19:57.980 |
I also added a section to track my completed tasks so that I could visually get a sense 01:20:04.540 |
After I made this weekly planning a habit, my team lead highlighted my approach at a 01:20:07.980 |
monthly team meeting, and we presented on how I leveraged the tool into something useful 01:20:13.500 |
I spoke to how this helped me organize me week to week so that I can take a proactive 01:20:17.820 |
approach and slow down versus being at the mercy of a hive mind mentality, constantly 01:20:23.900 |
reacting to incoming emails and team messages, and he goes on to mention some good stuff 01:20:34.060 |
What I like about it is that it emphasizes there are alternatives to what I call the 01:20:41.340 |
The list reactive method says you kind of just take each day as it comes, reacting the 01:20:45.540 |
stuff that's coming in over the transom through email and Slack, trying to make progress on 01:20:53.060 |
I'll react to things and try to make some progress on my to-do list. 01:20:56.420 |
It is not a very effective way to make use of your time and resources. 01:21:01.980 |
You get caught up in things that are lower value. 01:21:04.700 |
You lose the ability to give things the focus work required to get them done well and fast. 01:21:09.380 |
You fall behind on high priorities and get stuck on low priorities, so you have to be 01:21:15.740 |
Control, control, control is a big theme about how I talk about thriving in digital age knowledge 01:21:20.900 |
So I love this idea that the weekly plan discipline I talk about could be a big part of that answer. 01:21:25.220 |
Look at your week as a whole and say, what do I want to do with this week? 01:21:32.220 |
Why don't I consolidate all this time into this time over here surrounding this call 01:21:36.100 |
Why don't I cancel these two things because they're really making the rest of the week 01:21:40.700 |
When you plan your week in advance, it really helps you have a better week than if you just 01:21:46.780 |
stay at the scale of what am I doing today or even worse, the scale of just what am I 01:21:53.300 |
So multi-scale planning is critical for this control, control, control rhythm that I preach. 01:21:59.060 |
That's the only way really to survive in digital area knowledge work. 01:22:02.620 |
So what a cool example of weekly planning, helping you feel like you actually had some 01:22:13.020 |
I want to react to an article in the news, but first let's hear from another sponsor. 01:22:17.660 |
Look, the older I get and trust me, my birthday's in a few days. 01:22:23.700 |
The more I find myself wanting to be more intentional about the way I live. 01:22:28.380 |
And we talk about this all the time, my month, my birthday life planning, but I also want 01:22:34.740 |
to make sure I'm being intentional about how I eat and take care of my body. 01:22:44.920 |
This is why I really like our sponsor Mosh, M-O-S-H, Mosh bars. 01:22:54.260 |
It's one of my sort of go-to when I have these around, it's really like a go-to snack that 01:23:06.260 |
Let me tell you a little bit more why Mosh, which was started by actually interestingly 01:23:11.420 |
Maria Shriver and her son, Patrick Schwarzenegger, with the mission of creating a conversation 01:23:16.780 |
about brain health because Maria's father suffered from Alzheimer's. 01:23:22.940 |
They developed Mosh bars by joining forces with the world's top scientists and functional 01:23:28.220 |
nutritionist that give you a protein bar that goes beyond what you normally get in a protein 01:23:33.920 |
It has 10 delicious flavors, including three that are plant-based. 01:23:37.340 |
It is made with ingredients that support brain health, like ashwagandha, lion's mane, collagen, 01:23:46.500 |
Mosh bars come with a new look and a new formulation featuring a game-changing brain-boosting ingredient 01:23:53.100 |
It is the first and only food brand boosted with Cognizant, a premium nootropic that supplies 01:23:58.900 |
the brain with a patented form of cytokoline or cytokoline. 01:24:04.740 |
Here's the thing about the Mosh bars, they taste good. 01:24:06.860 |
I like them because they are soft with a little bit of crunch inside of them. 01:24:10.780 |
So you really, really crave eating these things. 01:24:13.420 |
A lot of protein sort of gives you what you need, and it has these sort of brain-boosting 01:24:16.780 |
ingredients as well, which really comes from Maria and her son, Patrick's real concern 01:24:25.780 |
That concern is also why Mosh donates a portion of all proceeds to fund gender-based brain 01:24:30.500 |
health research through the Women's Alzheimer's Movement. 01:24:35.260 |
Because Maria and Patrick noted that two-thirds of all Alzheimer's patients are women. 01:24:39.800 |
So Mosh is working closely to close the gap between women's and men's health research, 01:24:45.300 |
All right, so great tasting protein bars that have all this really cool stuff in them, built 01:24:55.480 |
So if you want to find ways to give back to others and fuel your body and your brain, 01:25:01.860 |
Head to moshlife.com/deep to save 20% off plus free shipping on either the Best Sellers 01:25:07.540 |
Trial Pack or the new Plant-Based Trial Pack. 01:25:10.740 |
That's 20% off plus free shipping on either the Best Sellers or Plant-Based Trial Pack 01:25:23.440 |
Thank you, Mosh, for sponsoring this episode. 01:25:26.020 |
I also want to talk about our friends at Shopify. 01:25:30.140 |
Whether you're selling a little or a lot, Shopify helps you do your things however you 01:25:37.860 |
If you sell things, you need to know about Shopify. 01:25:41.380 |
It's the global commerce platform that helps you sell at every stage of your business. 01:25:46.120 |
From the launch your online shop stage to the first real life store stage, all the way 01:25:51.660 |
to the "did we just hit a million order" stage, Shopify is there to help you grow. 01:25:58.700 |
They have an all-in-one e-commerce platform, which makes checking out online an absolute 01:26:06.220 |
They also have in-person POS systems right there in the store that what the people use 01:26:10.600 |
to actually do their credit card and do their transactions. 01:26:14.020 |
However you're selling, Shopify is really best in class at what they're doing. 01:26:18.900 |
They've even added, matching the theme of today's program, an AI feature called Shopify 01:26:25.400 |
Magic that helps you sell even more to your customers, be more successful at conversions. 01:26:34.780 |
You're selling something, you do need to check out Shopify. 01:26:38.740 |
The good news is I can help you do that with a good deal. 01:26:42.740 |
You can sign up for a $1 per month trial period at shopify.com/deep. 01:26:48.180 |
You got to type that all lowercase, but if you go to shopify.com/deep now, you can grow 01:26:56.640 |
your business no matter what stage you're in. 01:27:00.380 |
All right, Jesse, let's do our final segment. 01:27:06.640 |
All right, this article was sent to me a lot, and I guess it's because I'm mentioned in 01:27:12.680 |
it or because it feels like it's really important. 01:27:14.780 |
I brought it up here on the screen for people who are watching instead of just listening. 01:27:20.020 |
The article that most people sent me on this issue came from Axios. 01:27:26.020 |
The title of the Axios articles is "Why Employers Wind Up With Mouse-Jiggling Workers." 01:27:33.540 |
All right, so they're talking about mouse jigglers, which I had to look up. 01:27:40.820 |
But it is software you can run on your computer that basically moves your mouse pointer around. 01:27:45.260 |
So it simulates like if you're actually there jiggling your formal mouse. 01:27:51.740 |
Well, it turns out a bunch of mouse jigglers got fired at Wells Fargo. 01:27:59.880 |
They discovered that they were using the mouse jigglers, and they fired workers from their 01:28:11.100 |
There's a couple of reasons why the mouse jiggling is useful for remote workers. 01:28:15.620 |
One of them is the fact that common instant message agents like Slack and Microsoft Teams 01:28:22.520 |
puts this little status circle next to your name. 01:28:25.620 |
So if I'm looking at you in Slack or Teams, there's a status circle that says whether 01:28:31.360 |
The idea being like, "Hey, if you're not active, then I won't text you. 01:28:36.420 |
And if you are, like, if I know you're there working on your computer, I will." 01:28:39.260 |
Well, if your computer goes to sleep, your circle turns to inactive. 01:28:44.060 |
So the mouse jigglers keeps your circles active. 01:28:47.420 |
So if your boss is just like, "Hey, what's going on with Cal over here?" 01:28:50.940 |
They just sort of see like, "Oh, he must be working all very hard because his circle is 01:28:56.820 |
When in reality, you could be away from your computer, but the mouse jiggler is making 01:29:01.620 |
So there's been a kind of a lot of outrage about the mouse jigglers and about this type 01:29:11.100 |
Well, I'm cited in this Axios article, so we can see what they think I feel about it. 01:29:18.300 |
Here is how my take is described by Axios, and I'll see if I agree with this. 01:29:23.140 |
Remote surveillance is just the latest version of a boss looking out at the office floor 01:29:30.040 |
These kinds of crude measures are part of a culture of pseudoproductivity that kicked 01:29:33.260 |
off in the 1950s with the advent of office work, as Cal Newport writes in his latest 01:29:42.260 |
With technology-enabled 24-hour connection to the workplace, pseudoproductivity evolved 01:29:45.980 |
in ways that wound up driving worker burnout, like replying to emails at all hours or chiming 01:29:51.300 |
And with the rise of remote work, this push for employees to look busy and for managers 01:29:55.180 |
to understand who's actually working got even worse, Newport told me in a recent interview. 01:30:05.980 |
This is the way I see this, and I think this is the right way to see this. 01:30:10.540 |
There's a smaller argument, which I think is too narrow, which is the argument of bosses 01:30:17.420 |
are using remote surveillance, we should tell bosses to stop using remote surveillance. 01:30:25.420 |
Digital tools are giving us ways to do this privacy-violating surveillance, and we should 01:30:35.200 |
The bigger issue is what's mentioned here, this bigger trend. 01:30:38.300 |
This is what I outline in chapter one of my book, Slow Productivity. 01:30:43.700 |
It's what explicitly puts this book in the tradition of my technology writings, why this 01:30:48.140 |
book is really a technology book, even though it's talking about knowledge work. 01:30:54.280 |
For 70 years, knowledge work has depended on what I call pseudo-productivity, this heuristic 01:30:59.540 |
that says visible activity will be our proxy for useful effort. 01:31:04.300 |
We do this not because our bosses are mustache twirlers or because they're trying to exploit 01:31:09.020 |
us, but because we didn't have a better way of measuring productivity in this new world 01:31:16.880 |
There's no pile of Model Ts lined up in the parking lot that I can count. 01:31:21.420 |
So what we do is like, well, to see you in the office is better than not. 01:31:24.420 |
So come to the office, do factory shifts, be here for eight hours, don't spend too much 01:31:30.180 |
So we had this sort of crude heuristic because we didn't know how else to manage knowledge 01:31:37.060 |
And as pointed out in this article, that way of crudely managing productivity didn't play 01:31:47.740 |
And this mouse jiggler is just the latest example of this reality. 01:31:52.260 |
When we added 24-hour remote internet-based connectivity through mobile computing that's 01:31:57.940 |
with us at all times to the workplace, pseudo-productivity became a problem. 01:32:02.500 |
When pseudo-productivity meant, okay, I guess I have to come to an office for eight hours 01:32:05.540 |
like I'm putting steering wheels on a Model T, that's kind of dumb, but I'll do it. 01:32:11.300 |
And also like, if I'm reading a magazine at my desk, keep it below where my boss can see 01:32:18.580 |
But once we got laptops and then we got smartphones and we got the mobile computing revolution, 01:32:23.700 |
now pseudo-productivity meant every email I reply to is a demonstration of effort. 01:32:29.700 |
Every Slack message I reply to is a demonstration of effort. 01:32:36.100 |
At my kid's soccer game, I could be showing more effort. 01:32:39.340 |
This was impossible in 1973, completely possible in 2024. 01:32:43.580 |
This is what leads us to things like, I'm going to have a piece of software that artificially 01:32:47.000 |
shakes my mouse because that circle being green next to my name in Slack longer is showing 01:32:55.340 |
So the inanity of pseudo-productivity becomes pronounced and almost absurdist in its implications 01:33:05.420 |
That's why we need slow productivity now, because we have to replace pseudo-productivity 01:33:09.700 |
with something that's more results oriented and that plays nicer with the digital revolution. 01:33:13.780 |
So this is just like one of many, many symptoms of the diseased state of modern knowledge 01:33:19.480 |
work that's caused by us relying on this super vague and crude heuristic of just like doing 01:33:28.880 |
Slow productivity gives you a whole philosophical and tactical roadmap to something more specific. 01:33:37.320 |
It's based on production over time, not on busyness in the moment. 01:33:42.300 |
It's based on sequential focus and not on concurrent overload. 01:33:47.060 |
It's based on quality and not activity, right? 01:33:50.900 |
So it's an alternative to the pseudo-productivity that's causing problems like this mouse jiggler 01:33:59.120 |
New technologies requires us to finally do the work of really updating what we think 01:34:03.740 |
That's why I wrote that most recent book about it. 01:34:07.020 |
It's also why I hate that status light in Slack or Microsoft Teams. 01:34:15.900 |
And even the underlying mentality of that status light, which is like, if you're at 01:34:19.380 |
your computer, it's fine for someone to send you a message. 01:34:25.780 |
What if I'm doing something cognitively demanding? 01:34:27.460 |
It's a huge issue for me to have to turn over to your message. 01:34:30.640 |
So it also underlines the degree to which the specific tools we use completely disregard 01:34:36.580 |
the psychological realities of how people actually do cognitive effort. 01:34:40.360 |
So we have such a mess in knowledge work right now. 01:34:42.860 |
It's why, whatever, three of my books are about digital knowledge work. 01:34:46.340 |
It's why we talk about digital knowledge work so much on this technology show is because 01:34:50.780 |
digital age knowledge work is a complete mess. 01:34:53.840 |
The good news is that gives us a lot of low hanging fruit to pick. 01:34:56.740 |
That's going to cause advantages, delicious advantages. 01:35:02.100 |
There's a lot of easy changes we could make, but anyways, I'm glad people sent me this 01:35:12.980 |
Not narrow surveillance, but broad pseudo productivity plus technology is an unsustainable 01:35:20.220 |
All right, well, I think that's all the time we have for today. 01:35:23.740 |
Thank you everyone who sent in their questions, case studies and calls. 01:35:27.500 |
Be back next week with another episode, though it will probably be an episode filmed from 01:35:34.340 |
I'm doing my sort of annual retreat into the mountains for the summer. 01:35:38.640 |
The show will still come out on its regular basis, but just like last year, we'll be recording 01:35:42.420 |
some of these episodes with Jesse and I in different locations and I'll be in my undisclosed 01:35:47.780 |
I think next week might be the first week that is the case, but the shows will be otherwise 01:35:50.980 |
normal and I'll give you a report from what it's like from wherever I end up. 01:35:55.660 |
I'll tell you about my sort of deep endeavors, whatever deep undisclosed location I find, 01:36:01.900 |
but otherwise we'll be back and I'll see you next week. 01:36:06.660 |
Hey, if you liked today's discussion about diffusing AI panic, you might also like episode 01:36:12.700 |
244 where I gave some of my more contemporaneous thoughts on chat GPT right around the time 01:36:21.580 |
That is the deep question I want to address today. 01:36:25.340 |
How does chat GPT work and how worried should we be about it?