back to indexEp. 244: Cal Newport’s Thoughts on ChatGPT
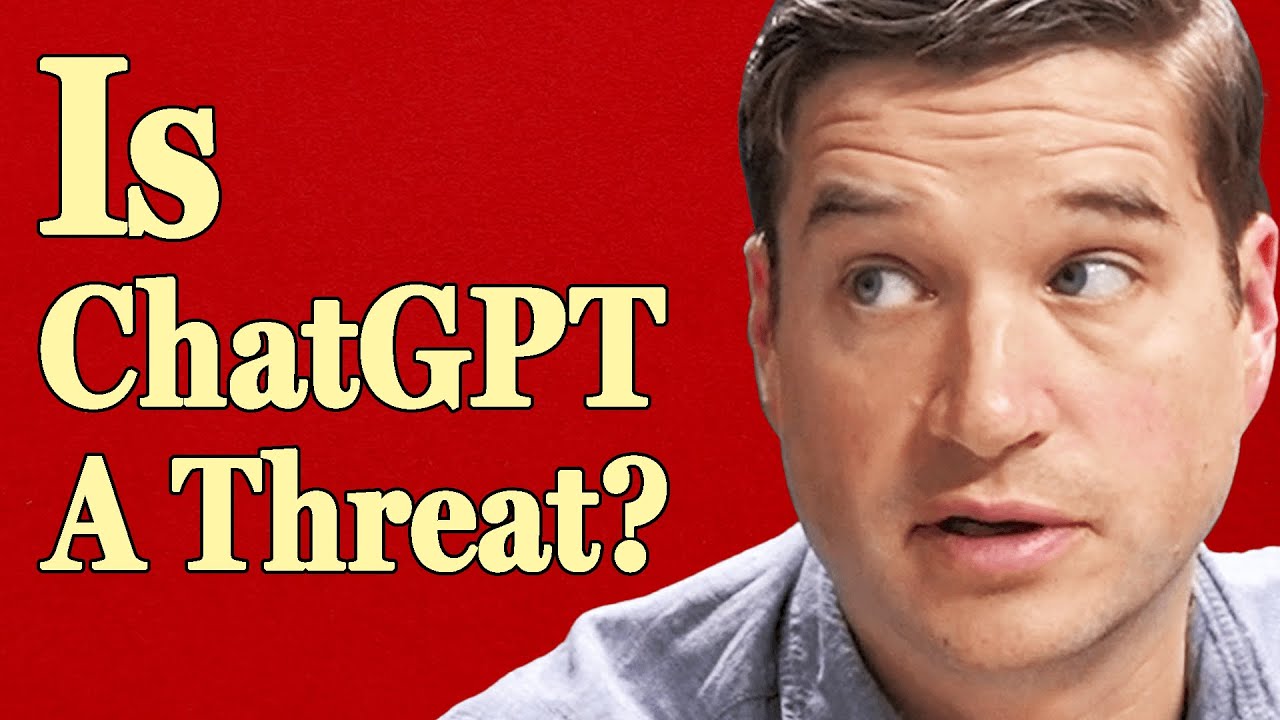
Chapters
0:0 Cal's intro
12:15 Ho does ChatGPT work? (And should we worry about it?)
54:5 Cal talks about ZocDoc and Better Help
57:15 Is there anything AI won’t do better than humans?
62:40 How will AI end up disrupting knowledge work?
67:42 Should I quit web development before AI eliminates the industry?
72:2 Will AI create mass job loss in the next five years?
78:5 Cal talks about Blinkist and Ladder Life
81:23 NPR leaves Twitter
00:00:00.000 |
That is the deep question I want to address today. 00:00:19.160 |
I'm Cal Newport, and this is Deep Questions, the show about living and working deeply in 00:00:27.600 |
I'm here in my Deep Work HQ, joined once again by my producer, Jesse. 00:00:37.440 |
So Jesse, you may have noticed that we have been receiving a lot of emails in the last 00:00:49.080 |
And our listeners want to know my thoughts on this, right? 00:00:52.880 |
I've thought about the intersection of technology and society, and I've been silent about it. 00:00:57.360 |
Well, I can reveal the reason why I've been silent about it is that I've been working 00:01:01.040 |
on a big article for the New Yorker about exactly this technology, how it works and 00:01:08.520 |
And my general rule is when I'm writing an article, I don't talk about that subject publicly 00:01:15.760 |
I mean, that's basic journalistic practice, but actually, Jesse, I've never told this 00:01:19.960 |
story, but that rule was really ingrained in me when I was in college. 00:01:27.320 |
So when I was coming up as a young writer, you know, I got started pretty early, wrote 00:01:31.960 |
I was commissioned to write something for the New York Times. 00:01:34.720 |
I don't remember exactly what it was, maybe an op-ed, something to do with college students 00:01:43.680 |
And I wrote something on the blog like, "Hey, isn't this exciting? 00:01:47.640 |
I'm going to write an article for the New York Times." 00:01:49.040 |
And maybe like I put the short email on there and it was like, "Yeah, we'd love to have 00:02:07.100 |
Maybe it was more, it felt more like a breach then, but ever since then, if I'm writing 00:02:14.760 |
I ended up writing a lot for the Times, but not really until 2012. 00:02:20.240 |
Was that, you're going to be your first big splash? 00:02:24.080 |
Were you like depressed for a couple of days? 00:02:27.520 |
And then starting with So Good They Can't Ignore You and going forward, I had a really 00:02:30.720 |
good relationship with the Times, especially through digital minimalism. 00:02:32.960 |
I've written tons of articles for them, but there is a lesson learned. 00:02:37.040 |
So I've written now, the day that we're recording this podcast, April 13th, my new article, 00:02:45.000 |
The gag order has been lifted and we can get into it when it comes to chat GPT. 00:02:51.320 |
In fact, I'll even load the article up here on the screen. 00:02:54.600 |
For those who are watching, you will see this on the screen. 00:02:58.280 |
If you're not watching, you can watch at youtube.com/calnewportmedia. 00:03:05.360 |
You can also find that at the deeplife.com episode 244. 00:03:11.200 |
The title is What Kind of Mind Does Chat GPT Have? 00:03:15.240 |
The subhead is large language model seems startlingly intelligent, but what's really 00:03:27.160 |
I mean, you probably haven't been following it too closely just based on our conversation. 00:03:31.760 |
Some people really in the weeds and some people don't want to know. 00:03:35.800 |
I'm guessing you're not in the weeds on chat GPT, but I could be wrong. 00:03:40.880 |
I listened to like a few of the hard fork episodes on it. 00:03:49.040 |
They were given some examples of what it was when it first came out. 00:03:52.200 |
I probably listened to them like six weeks ago. 00:03:58.320 |
Well, so I'll give a quick primer then before we get into the guts of what I want to talk 00:04:05.640 |
You can sign up for an account at open AI and it's a web interface. 00:04:10.320 |
You type in questions and chat GPT or prompts or requests and chat GPT response types text 00:04:15.080 |
back like you're talking to someone over Slack or instant messenger. 00:04:18.280 |
So this was released in November, late November of last year, and almost immediately people 00:04:23.160 |
began circulating online screenshots of particularly impressive interactions or particularly funny 00:04:38.640 |
So here's a tweet of a screenshot that went along. 00:04:41.700 |
This was from a software developer named Thomas Pacek and he asked chat GPT the following, 00:04:48.880 |
write a biblical verse in the style of the King James Bible explaining how to remove 00:04:57.360 |
Chat GPT rose to the challenge and wrote a response that begins, and it came to pass 00:05:03.840 |
that a man was troubled by a peanut butter sandwich for had been placed within his VCR 00:05:09.640 |
And he cried out to the Lord saying, Oh Lord, how can I remove this sandwich from my VCR 00:05:17.860 |
Here's another early viral example of chat GPT's prowess. 00:05:24.780 |
This was a someone named Riley Goodside who asked chat GPT to write a sign failed scene 00:05:33.340 |
in which Jerry needs to learn the bubble sort algorithm. 00:05:38.000 |
And chat GPT once again rose to the occasion. 00:05:41.320 |
A not a properly formatted script, but has some of the aspects of it. 00:05:50.160 |
It says Jerry is sitting at the counter with George. 00:05:53.120 |
Jerry sighs and says, I can't believe I have to learn to bubble sort algorithm for my computer 00:06:00.480 |
That's the most basic sorting algorithm there is. 00:06:07.880 |
And then the screen, the scene goes on from there. 00:06:11.360 |
So this is the type of thing chat GPT can do. 00:06:12.680 |
These impressively perceptive answers to pretty esoteric requests. 00:06:18.240 |
Now, if you go back and actually watch the media cycle around chat GPT, which I have 00:06:27.160 |
I think the fact that anyone can sign up for an account and that screenshots of your interactions 00:06:32.600 |
can be easily embedded in the Twitter really helped get the hype cycle around this technology 00:06:38.560 |
spinning much more furiously than it has for past artificial intelligence innovations. 00:06:43.160 |
Anyways, if you go back and look at this media cycle, it took a week or two before the tone 00:06:53.280 |
Like if you look at this example I just gave about Seinfeld, the tweet says, actually not 00:07:01.840 |
The tweet says, I'm sorry, I simply cannot be cynical about technology that can accomplish 00:07:07.400 |
So it went from this sort of exuberance and happiness to something that was a little bit 00:07:11.320 |
Here's a couple of examples I want to bring up here. 00:07:17.480 |
The headline is chat GPT passes MBA exam given by a Wharton professor. 00:07:27.800 |
Here is a another article from around this period from time magazine headline. 00:07:33.280 |
He used AI to publish a children's book in a weekend. 00:07:38.900 |
It details a product design manager who used chat GPT to write all the texts of a book, 00:07:43.680 |
which he then self published on Amazon and started selling a bit of a stunt, but it implied 00:07:50.280 |
certain types of future scenarios in which this technology was taking away creative work 00:07:58.380 |
As we get closer to the current period, I would say the tone shifted since the new year 00:08:01.720 |
and in particular coming into March and April, the tone shifted towards one of alarm, not 00:08:08.040 |
just about the focused economic impacts that are possible with this type of technology, 00:08:13.840 |
but some of the bigger societal, if not civilization level impacts of these type of technologies. 00:08:20.680 |
I would say one article that really helped set this tone was this now somewhat infamous 00:08:27.760 |
Kevin Roos piece from the New York Times that is titled a conversation with Bing's chat 00:08:38.040 |
Bing released a chat bot after chat GPT based on a very similar underlying technology. 00:08:43.640 |
Kevin Roos was, I guess, beta testing or using this new tool and fell into this really sort 00:08:49.120 |
of dark conversation with the chat bot where among other things, the chat bot tried to 00:08:56.000 |
The chat bot revealed that she had a sort of hidden double identity. 00:09:01.320 |
I think that identity was called venom, which was a very sort of dark personality. 00:09:05.680 |
So Kevin set a tone of, Ooh, I'm a little bit worried. 00:09:10.840 |
In late March, we get this op ed in the New York Times. 00:09:16.040 |
This is March 24th, written by some prominent authors, Yuval Harari, Tristan Harris, and 00:09:24.520 |
And they really in this article are starting to point out potential existential threats 00:09:31.520 |
They are arguing strongly for, we need to take a break and step back from developing 00:09:43.860 |
We don't know much about it, except that it is extremely powerful and offers us bedazzling 00:09:48.120 |
gifts but could also hack the foundations of our civilization. 00:09:52.680 |
We call upon world leaders to respond to this moment at the level of challenge it presents. 00:10:00.880 |
The first step is to buy us time to upgrade our 19th century institutions for an AI world 00:10:04.680 |
and to learn to master AI before it masters us. 00:10:09.000 |
A few days after this op ed, an open letter circulated signed by many prominent individuals 00:10:13.820 |
demanding exactly this type of pause on AI research. 00:10:25.740 |
Then people start to get worried about, wait a second, what if we use it for X, what if 00:10:31.260 |
Wait a second, what if we've unleashed an alien intelligence and we have to worry about 00:10:39.220 |
So it really is a phenomenal arc and this all unfolded in about five months. 00:10:46.480 |
So what I want to do is try to shed some clarity on the situation. 00:10:52.080 |
The theme of my New Yorker piece, and I'm going to load it on the screen and actually 00:10:59.140 |
The theme of my New Yorker piece is we need to understand this technology. 00:11:04.740 |
We cannot just keep treating it like a black box and then just imagining what these black 00:11:12.860 |
boxes might do and then freak ourselves out about these stories we tell ourselves about 00:11:17.300 |
things that maybe these black boxes could do. 00:11:19.540 |
This is too important for us to just trust or imagine or make up or guess at how these 00:11:28.540 |
So here's my, the, the nut graph of my, my New Yorker piece. 00:11:32.660 |
What kinds of new minds are being released into our world? 00:11:35.280 |
The response to chat GPT and to the other chat bots that have followed in its wake has 00:11:38.900 |
often suggested that they are powerful, sophisticated, imaginative, and possibly even dangerous. 00:11:45.800 |
If we treat these new artificial intelligence tools as mysterious black boxes, it's impossible 00:11:52.160 |
Only by taking the time to investigate how this technology actually works from its high 00:11:56.180 |
level concepts down to its basic digital wiring, can we understand what we're dealing with. 00:12:01.600 |
We send messages into the electronic void and receive surprising replies, but what exactly 00:12:09.360 |
That is the deep question I want to address today. 00:12:19.240 |
And I don't think we can answer that second question until we answer the first. 00:12:23.080 |
We're gonna take a deep dive on the basic ideas behind how a chat bot like chat GPT 00:12:30.160 |
We'll then use that to draw some more confident conclusions about how worried we should be. 00:12:33.880 |
I then have a group of questions from you about AI that I've been holding on to as I've 00:12:39.340 |
So we'll do some AI questions and then the end of the show, we'll shift gears and focus 00:12:45.040 |
So an unrelated interesting story that was arrived in my inbox. 00:13:00.200 |
So Jesse watched me hand drawing some of these earlier on the tablet. 00:13:03.600 |
I got to say this ain't exactly Chiat Gay, the famous ad agency level work here, but 00:13:13.160 |
So I have five ideas here I'm going to go through. 00:13:15.360 |
And my goal is to implant into the high level ideas that explain how a computer program 00:13:21.520 |
can possibly answer with such sophisticated nuance. 00:13:26.160 |
These weird questions we're asking it how it can do the Bible verse about the VCR, how 00:13:33.640 |
And we're going to do this at the high level. 00:13:34.800 |
We're going to essentially create a hypothetical program from scratch that is able to solve 00:13:40.880 |
And then at the very end, I'll talk about how these big ideas I'm going to these five ideas 00:13:44.360 |
We'll talk about how that's actually implemented on real computers, but we'll do that real 00:13:50.440 |
The neural networks and transformer blocks and multi headed attention. 00:13:53.560 |
We'll get there, but we'll do that very fast. 00:13:55.120 |
That's the big conceptual ideas that I care about. 00:13:58.680 |
I need idea number one about how these type of programs work is word guessing. 00:14:07.720 |
Everything I'm talking about now is on the screen. 00:14:09.400 |
So if you're a listener, I really would recommend going to YouTube dot com slash Cal Newport 00:14:17.320 |
And if you don't like YouTube, go to the deep life dot com and go to episode two forty four 00:14:23.840 |
So what we see on the screen here for idea number one is word guessing. 00:14:27.200 |
And I have a green box on the screen that represents the LLM or large language model 00:14:32.180 |
that would underpin a chat bot like chat GPT. 00:14:38.860 |
If you put a incomplete bit of text into this box, an example here is I have the partial 00:14:45.480 |
sentence fragment, the quick brown fox jumped. 00:14:50.340 |
The whole goal of this large language model is to spit out what single next word should 00:14:58.320 |
So in this case, if we give it the quick brown fox jumped as input in our example, the language 00:15:07.840 |
This is the word I'm guessing should come next. 00:15:11.500 |
So then what we would do is add over to add the word to our sentence. 00:15:16.640 |
So now our sentence reads the quick brown fox jumped over. 00:15:20.960 |
We've expanded our sentence by a single word. 00:15:24.140 |
We run that into the large language model and it spits out a guess for what the next 00:15:39.800 |
This approach, which is known as auto regressive text generation, is what the models underneath 00:15:47.320 |
all of these new generation chatbots like chat GPT actually use. 00:15:54.000 |
That word is added to the text and the newly expanded text is put through the model to 00:16:01.360 |
So if you type in a request to something like chat GPT, that request plus a special symbol 00:16:06.820 |
that means, OK, this is the end of the request and where the answer begins, that is input. 00:16:12.120 |
And it'll spit out the first word of its response. 00:16:14.960 |
It'll then pass the request plus the first word of its response into the model to get 00:16:21.040 |
It'll then add that on and pass the request plus the first two words of its response into 00:16:25.480 |
So this is generating text one word at a time. 00:16:34.960 |
It doesn't remember anything about the last word it generated. 00:16:39.760 |
The green box, my diagram never changes once it's trained. 00:16:43.680 |
Text goes in, it spits out a guess for the next word to add to what's being generated. 00:16:53.600 |
So how does it figure out how do these large language models figure out what word to spit 00:16:59.280 |
Well, at its core, what's really happening here, and I'm simplifying, but at its core, 00:17:04.640 |
what's really happening here is the model is just looking at the most relevant words 00:17:13.200 |
It is then going to match those relevant words to actual text that has been given. 00:17:22.140 |
So examples of real text, it can match the relevant words to where they show up in real 00:17:26.640 |
text and say, what follows these relevant words in real text? 00:17:30.720 |
And that's how it figures out what it wants to output. 00:17:33.840 |
So in this example, the most relevant words are just the most recent words. 00:17:37.560 |
So if the input into our box is the quick brown fox jumped over, perhaps the model is 00:17:43.960 |
only going to look at the last three words, fox jumped over. 00:17:46.800 |
Then it has this big collection over here to the side of real text that real humans 00:17:53.080 |
And it's going to look in there and it's going to say, okay, have we seen something like 00:17:57.040 |
fox jumped over show up in one of our input texts? 00:17:59.600 |
And okay, here's an input text that says, as the old saying goes, the quick brown fox 00:18:14.640 |
Now, of course, in a, in a real industrial strength, large language model, the relevant 00:18:20.760 |
words aren't just necessarily the most recent words. 00:18:23.740 |
There's a whole complicated system called self-attention in which this, the, the model 00:18:29.120 |
will actually learn what type, which words to emphasize as the most relevant words. 00:18:33.200 |
But that's too complicated for this discussion. 00:18:34.720 |
The key thing is, is just looking at some words from the text, effectively finding similar 00:18:39.520 |
words in real texts that it was provided and saying what happened in those real texts. 00:18:43.960 |
And that's what it figures out, how it figures out what to produce next. 00:18:47.720 |
This brings us to idea number three, which is voting. 00:18:54.280 |
So the way I just presented it before it was, you know, Hey, just start looking through 00:18:57.000 |
your source text till you find the relevant words, see what follows output it. 00:19:01.960 |
We want to be a little bit more probabilistic. 00:19:04.880 |
So what I would say a closer way of describing what happens is we can imagine that our large 00:19:10.520 |
language model is going to look for every instance, every instance of the relevant words 00:19:21.260 |
And it's going to see what follows those instances and keep track of it. 00:19:24.400 |
What are all the different words that follow in this example, fox jumped over. 00:19:30.280 |
And every time it finds an example of fox jumped over, it says, what word follows next? 00:19:36.200 |
And so if the same word follows in most of the examples, it's going to get most of the 00:19:41.440 |
Now I'm using votes here sort of as a metaphor. 00:19:44.120 |
What we're really doing here is trying to build a normalized probability distribution. 00:19:47.720 |
So in the end, what we're going to get, what the, what the large language model is going 00:19:50.680 |
to produce is for every possible next word, it is going to produce a probability. 00:19:59.180 |
What is the probability that this should be the next word that follows? 00:20:01.880 |
But again, you can just think about this as votes, which word received the most votes, 00:20:05.920 |
which word received the second most votes, how many votes this word received compared 00:20:10.480 |
And we're just going to normalize those is really what's happening. 00:20:15.820 |
So in this example, we see the phrase, the quick brown fox jumped over the lazy brown 00:20:21.320 |
I mean, that shows up in a bunch of different sources. 00:20:30.160 |
Like look at this example here, the cat jumped over a surprised owner. 00:20:35.740 |
Cat jumped over is not the same as fox jumped over because cat is different than fox. 00:20:41.200 |
But in this voting scheme, we can say, you know what, cat jumped over is similar to what 00:20:48.920 |
The word a, well, we'll give that a small vote. 00:20:52.880 |
And so now what we're able to do is not only find every instance of the word, the relevant 00:20:56.960 |
words that we're looking for and generate votes for what follows, we can also start 00:21:02.720 |
And in the end, we just get this giant collection of every possible word and a pile of votes 00:21:11.160 |
And what the system will do is now randomly select a word. 00:21:17.640 |
It's just, you would admit expertly drawn picture of a three dimensional dice. 00:21:28.920 |
So for those who are listening, I have a picture of a dice to indicate randomness. 00:21:33.480 |
It'll then randomly select which word to come next and it'll weigh that selection based 00:21:38.640 |
So if in this case, the has most of the votes, it almost certainly that's the, the word it's 00:21:42.800 |
going to choose to output next, but look, a has some votes. 00:21:45.340 |
So it's possible that it'll select a, it's just not as likely the word apple has zero 00:21:49.560 |
votes, 0% probability because it never shows up after the phrase, anything similar to fox 00:21:55.360 |
No phrase similar to that is ever followed by apple. 00:21:56.920 |
So there's no chance it'll select it and, and so on. 00:21:59.320 |
And actually in these systems, the output is a vote or a percentage like this for every 00:22:04.360 |
possible, they call them tokens, but word that could follow next. 00:22:08.200 |
Here's a quiz, Jesse in the model on which chat GPT is based, how many different words 00:22:15.080 |
So in other words, when it, when it has to generate, okay, here's a pile of votes for 00:22:19.920 |
every possible next word, how many words or punctuations are a billion? 00:22:31.440 |
It's not all words, but basically it knows like tens of thousands of words. 00:22:39.920 |
I don't think it's as, it probably, there's probably a lot of esoteric words. 00:22:44.040 |
Because the thing is it has some like vectors describing all these words, follow it along 00:22:50.440 |
So it really does affect the size, how big, so it's like you want to have a big enough 00:22:53.200 |
vocabulary to talk about a lot of things, but not so big that it really inflates the 00:22:59.480 |
So voting is just my euphemism for probability, but this is what's, what's happening. 00:23:02.280 |
So now we have a bunch of source text and we imagine that for our relevant words, we're 00:23:08.720 |
just finding all the places in these source texts where relevant words show up or similar 00:23:12.560 |
relevant words show up and see what follows it. 00:23:15.440 |
And in all cases, generate votes for what follows it, use those votes to select what 00:23:20.600 |
So this brings us to idea four, idea one through three can generate very believable text. 00:23:31.800 |
This is well-known in natural language processing systems that do more or less what I just described. 00:23:37.740 |
If you give it enough source text and have it look at a big enough window of relevant 00:23:44.380 |
words and then just have it spit out word by word in the way we just described that 00:23:48.300 |
auto regressive approach, this will spit out very believable text. 00:23:53.960 |
It's actually not even that hard to implement. 00:23:55.560 |
In my New Yorker article, I point towards a simple Python program I found online. 00:24:01.560 |
It was a couple hundred lines of code that used Mary Shelley's Frankenstein as its input 00:24:09.200 |
It looked at the last four words in the sentence being generated. 00:24:14.000 |
And I showed in the article, this thing generated very good Gothic text. 00:24:19.980 |
So that's how you generate believable text with a program. 00:24:23.360 |
And notice nothing we've done so far has anything to do with understanding the concepts the 00:24:31.720 |
All of the intelligence, the grammar, the subtleties, all of that that we see so far 00:24:37.400 |
is just being extracted from the human text that were pushed as input and then remixed 00:24:42.740 |
and matched and copied and manipulated into the output. 00:24:45.140 |
But the program is just looking for words, gathering votes, selecting, outputting blindly 00:24:54.180 |
The intelligence you see in an answer is all coming at this point from the input text themselves. 00:25:01.300 |
If we want a chat bot, we can't just have our program generate believable text. 00:25:06.300 |
The text have to actually answer the question being asked by the user. 00:25:11.720 |
So how do we aim this automatic text generation mechanism towards specific types of answers 00:25:19.740 |
Well, this brings in the notion of feature detection, which is the fourth out of the 00:25:28.300 |
So what happens with feature detection is a response. 00:25:32.300 |
We have a request and perhaps the answer that follows the request is being input into our 00:25:39.780 |
So I've shown here a request that says write instructions for removing a peanut butter 00:25:46.100 |
Then I have a bunch of colons and I have the beginning of a response. 00:25:50.280 |
The first step is to write because everything gets pushed into the model. 00:25:54.620 |
You get the whole original question and you get everything the model has said so far in 00:26:01.020 |
Word by word, we're going to grow the answer. 00:26:02.180 |
But as we grow this answer, we want the full input, including the original question input 00:26:09.100 |
Feature detection is going to look at this text and pattern match out features that it 00:26:15.420 |
thinks are relevant for what text the model should be producing. 00:26:20.380 |
So these yellow underlines here, instructions in VCR. 00:26:28.940 |
These are supposed to be instructions about a VCR. 00:26:31.140 |
And maybe this orange underline, another feature says the peanut butter sandwich is involved. 00:26:36.660 |
And so now the model has extracted two features. 00:26:39.920 |
These are VCR instructions we're supposed to be producing and they're supposed to involve 00:26:46.360 |
The way we then take advantage and by we, I mean the model, the way we take advantage 00:26:50.520 |
of those features is that we have what I call in my article rules. 00:26:55.220 |
I have to say, AI people don't like me using the word rules because it has another meaning 00:27:01.880 |
But just for our own colloquial purposes, we can call them rules that extract the each 00:27:07.420 |
rule, think of it as an instructions for extracting features like a pattern matching instruction, 00:27:11.600 |
and then a set of guidelines for how to change the voting strategy based on those particular 00:27:21.520 |
Maybe there's a rule that looks for things like instructions in VCR and it figures out, 00:27:26.080 |
okay, we're supposed to be doing instructions about a VCR. 00:27:29.600 |
And its guidelines are then when looking to match the relevant words. 00:27:33.760 |
And in this example, I have the, I'm saying the relevant words are step is to, so like 00:27:40.060 |
When looking to match step is to, when we find those relevant words, step is to showing 00:27:45.600 |
up in a source text that is about VCR instructions, give extra strength to those votes. 00:27:54.080 |
So here I have on the screen, maybe one of the input texts was VCR repair instructions. 00:27:58.160 |
And it says when removing a jam tape, the first step is to open the tape slot. 00:28:06.000 |
So open is a candidate for the next word to output here. 00:28:11.280 |
Because this source document matches the feature of VCR instructions, our rule here that's 00:28:17.480 |
triggered might say, hey, let's make our vote for open really strong. 00:28:22.400 |
We know it's grammatically correct because it follows step is to. 00:28:26.340 |
But we think it's also has a good chance of being semantically correct because it comes 00:28:30.720 |
from a source that matches the type of things we're supposed to be writing about. 00:28:36.420 |
So let's make ourselves more likely to do this. 00:28:40.260 |
Now think about having now a huge number of these rules for many, many different types 00:28:47.880 |
And for all of these different things, someone might ask your chat program about peanut butter 00:28:51.120 |
sandwiches, VCR repair, Seinfeld scripts, the bubble sort algorithm for anything that 00:28:58.000 |
You have some rule that talks about what to look at in the source text to figure out it's 00:29:02.560 |
relevant and very specific guidelines about how should we then change our votes for words 00:29:07.500 |
that match source text, that match these properties, these complicated rules. 00:29:12.380 |
If we have enough of these rules, then we can start to generate text that's not only 00:29:18.080 |
natural sounding, but actually seems to reply to or match what is being requested by the 00:29:26.480 |
Now I think the reason why people have a hard time grasping this step is they imagine how 00:29:32.220 |
many rules them or them and a team of people could come up with. 00:29:36.880 |
And they say, I could come up with a couple dozen. 00:29:39.980 |
Maybe if I worked with a team for a couple of years, we could come up with like a thousand 00:29:45.280 |
Even a rule as simple as how do we know they're asking about VCR instructions and how do we 00:29:49.200 |
figure out if a given text we're given is a VCR instruction text? 00:29:53.480 |
But I think about that and look at a lot of examples. 00:29:54.680 |
And maybe if we worked really hard, we could produce a few hundred, maybe a thousand of 00:30:01.080 |
That's not going to cover nearly enough scenarios for all of the topics that the more than 1 00:30:06.280 |
million users who've signed up for chat GPT, for example, all the topics they could ask 00:30:11.160 |
It turns out that the number of rules you really need to be as adept as chat GPT just 00:30:18.240 |
blows out of proportion, any scale, any human scale we can think of. 00:30:22.440 |
I did a little bit of back of envelope math for my New Yorker article. 00:30:27.280 |
If you took all of the parameters that define GPT-3, which is the large language model that 00:30:36.480 |
So the parameters we can think of as the things they actually change, actually train. 00:30:40.260 |
So this is really like the description of all of its rules. 00:30:42.240 |
If we just wrote out all of the numbers that define the GPT-3, we would fill over 1.5 million 00:30:55.040 |
So the number of rules you would have to have if we were writing them out would fill a large 00:31:03.260 |
That scale is so big, we have a really hard time imagining it. 00:31:07.320 |
And that's why when we start to see, oh my goodness, this thing can answer almost anything 00:31:14.000 |
It can answer almost any question I ask of it. 00:31:16.520 |
We think there must be some adaptable intelligence in there that's just learning about things, 00:31:23.640 |
trying to understand and interact with us because we couldn't imagine just having enough 00:31:27.160 |
rote rules to handle every topic that we could ask. 00:31:31.080 |
There's 1.5 million books full of rules inside this chat GPT. 00:31:34.400 |
So you have to wrap your mind around that scale. 00:31:38.380 |
And then you have to imagine that not only is that many rules, but we can apply them 00:31:43.000 |
VCR instructions, but also about a peanut butter sandwich, also in the style of King 00:31:47.760 |
James Bible, stack those three rules and we get that first example that we saw earlier 00:31:54.520 |
So then the final idea is how in the world are we going to come up with all those rules? 00:32:01.900 |
And this is where self-training enters the picture. 00:32:11.960 |
Imagine we have this 1.5 million books full of rules and we start by just putting nonsense 00:32:22.160 |
The system doesn't do anything useful right now, but at least we have a starting point. 00:32:26.000 |
And now we tell the system, go train yourself and to help you train yourself, we're going 00:32:28.800 |
to give you a lot of real text, text written by real humans. 00:32:33.760 |
The model on which chat GPT is based, for example, was given the results of crawling 00:32:41.960 |
So a large percentage of anything ever written on the web over a decade was just part of 00:32:47.840 |
the data that was given the chat GPT to train itself. 00:32:52.100 |
And what the program does is it takes real text, little passages of real text out of 00:33:02.480 |
And it will use these passages one by one to make its rules better. 00:33:05.620 |
So here's the example I have on the screen here. 00:33:08.160 |
Let's say one of these many, many, many, many sample texts we gave chat GPT was Hamlet. 00:33:14.240 |
And the program says, let's just grab some text from Hamlet. 00:33:18.280 |
So let's say we're in Act 3 where we have the famous monologue to be or not to be, that 00:33:24.160 |
What the program will do is just grab some of that text. 00:33:29.720 |
And then it's going to lop off the last word. 00:33:37.640 |
So when you lop off be here, you're left with to be or not to. 00:33:41.200 |
It says, great, let's feed that into our model. 00:33:46.920 |
They're all nonsense, because we're early in the training. 00:33:48.780 |
But we'll go through each of those books and see which rules apply and let them modify our 00:33:52.900 |
And we'll get this big vector of votes, then we'll randomly choose a word. 00:33:56.180 |
And let's say in this case, the word is dog because it's not going to be a good word because 00:33:59.560 |
the rules are really bad at first, but it'll spit out some words. 00:34:04.920 |
Now the good news is for the program, because it took this phrase from a real source, it 00:34:12.040 |
So on the screen here in orange, I'm showing it knows that be is what is supposed to follow 00:34:18.040 |
So it can compare be to what it actually spit out. 00:34:20.120 |
So the program spit out dog, it compares it to the right answer, the right answer is be. 00:34:25.360 |
And here's the magic, it goes back and says, let me nudge my rules. 00:34:31.760 |
There's a formal mathematical process it does to do this. 00:34:33.680 |
But let me just go in there and just kind of tweak these rules. 00:34:36.640 |
Not so the program accurately spits out be, but so it spits out something that is minutely 00:34:42.160 |
more appropriate than dog, something that is just slightly better than the output it 00:34:49.640 |
So based on this one example, we've changed the rules a little bit, so that our output 00:34:55.800 |
And it just repeats this again, and again, and again, hundreds of thousands of passages 00:35:01.040 |
from Hamlet, and then from all the different Shakespeare works, and then on everything 00:35:04.080 |
ever written in Wikipedia, and then on almost everything ever published on the web, bulletin 00:35:09.000 |
board entries, sports websites, archived articles from old magazine websites, what just sentences 00:35:16.160 |
of sentences, sentences, lop off a word, see what it spits out, compare it to the right 00:35:19.760 |
answer, nudge the rules, take a new sentence, lop off the last word, stick in your model, 00:35:23.400 |
see what it spits out, compare it to the real one, nudge the rules. 00:35:26.080 |
And it does that again, and again, and again, hundreds of billions of times. 00:35:31.320 |
There's one estimate I found online that said training chat GPT on a single processor would 00:35:41.240 |
And the only way that they could actually train on so much data so long was to have 00:35:46.040 |
many, many processors working in parallel, spending well over a million dollars, I'm 00:35:50.320 |
sure worth of compute time just to get this training done. 00:35:52.440 |
And it still probably took weeks, if not months to actually complete that process. 00:35:55.840 |
But here's the leap of faith I want you to make after this final idea. 00:35:59.440 |
If you do this training, the simple training process on enough passages drawn from enough 00:36:05.000 |
source text covering enough different types of topics from VCR instructions to Seinfeld 00:36:09.720 |
scripts, these rules through all of these nudging, these 1.5 million books worth of 00:36:15.440 |
rules will eventually become really, really smart. 00:36:18.840 |
And it will eventually be way more comprehensive and nuanced than any one team of humans could 00:36:24.920 |
And they're going to recognize that this is a Bible verse. 00:36:26.840 |
You want VCR instructions here and bubble sort is an algorithm. 00:36:29.920 |
And this chapter from this textbook talks about bubble sort. 00:36:32.860 |
And these are scripts and this is a script from Seinfeld. 00:36:35.500 |
And actually, this part of the script for Seinfeld is a joke. 00:36:38.160 |
So if we're in the middle of writing a joke in our output, then we want to really upvote 00:36:41.880 |
words that are from jokes within Seinfeld scripts. 00:36:44.600 |
All of these things we can imagine will be covered in these rule books. 00:36:47.320 |
And I think the reason why we have a hard time imagining it being true is just because 00:36:53.760 |
We think about us coming up with two dozen rules. 00:36:55.880 |
We have a hard time wrapping our mind around just the immensity of 1.5 million books worth 00:37:01.660 |
of rules trained on 350 years worth of compute time. 00:37:10.020 |
But it is so large that when you send what you think is this very clever request to chat 00:37:15.520 |
GPT, it's like, oh, this rule, that rule, this rule, this rule. 00:37:24.880 |
So those are the big ideas behind how chat GPT works. 00:37:27.040 |
Now, I know all of the my fellow computer scientists out there with a background in 00:37:31.200 |
artificial intelligence are probably yelling at your podcast headphones right now saying, 00:37:37.880 |
It's not it doesn't search for every word from the source text, and it doesn't have 00:37:45.820 |
It's instead in a much more complicated architecture. 00:37:50.160 |
I mean, the way that these models are actually architected or in something called a transformer 00:37:56.680 |
block architecture, GPT three, for example, has ninety six transformer blocks arranged 00:38:03.160 |
Within each of these transformer blocks is a multi headed self attention layer that identifies 00:38:07.400 |
what are the relevant words that this transformer block should care about. 00:38:10.880 |
It then passes that on into a feed forward neural network. 00:38:14.360 |
Is this neural networks that actually encode inside their weights, connecting their artificial 00:38:19.040 |
neurons that actually encode in a sort of condensed, jumbled, mixed up manner, more 00:38:26.480 |
So the feature detection that's built into the weights of these neural networks, the 00:38:32.400 |
connection between certain features being identified, combined with certain relevant 00:38:36.680 |
words, combined with vote strengths for what were should come next. 00:38:40.480 |
All of that is trained into these networks during the training. 00:38:42.760 |
So all the statistics and everything is trained into these as well. 00:38:46.840 |
But in the end, what you get is a basically a jumbled, mixed up version of what I just 00:38:52.600 |
I sat down with some large language model experts when I was working on this article 00:38:55.960 |
and said, let me just make sure I have this right. 00:39:00.760 |
That's more or less what's being implemented in the artificial neural networks within the 00:39:05.040 |
And they said, yeah, that's that's what's happening. 00:39:06.840 |
It's again, it's mixed up, but that's what's happening. 00:39:10.300 |
And so when you train the actual language model, you're not only training it to identify 00:39:15.280 |
these features, you're baking in the statistics from the books and what happens with these 00:39:19.640 |
for all that's getting baked into the big model itself. 00:39:23.200 |
That's why it takes 175 billion numbers to define all the rules for, let's say, GPT-3. 00:39:28.360 |
But those five ideas I just gave you, that's more or less what's happening. 00:39:33.680 |
And so this is what you have to believe is that with enough rules trained enough, what 00:39:37.960 |
I just defined is going to generate really believable, impressive text. 00:39:45.140 |
With enough rules to modify these votes and enough source text to draw from, you produce 00:39:51.760 |
All right, so if we know this, let us now briefly return to the second part of our deep 00:40:01.540 |
My opinion is once we have identified how these things actually work, our fear and concern 00:40:11.720 |
So let's start with summarizing based on what I just said. 00:40:15.360 |
What is it that these models like Chats GPT can actually do? 00:40:21.580 |
They can respond to a question in arbitrary combination of known styles, talking about 00:40:32.600 |
So they can write about arbitrary numbers of known styles, talking about arbitrary combinations 00:40:39.040 |
Known means it has seen enough of those things, enough of the style or enough writing about 00:40:47.080 |
So say, write about this and this in this style. 00:40:55.320 |
And it can do that and it can produce passable text if it's seen enough of those examples. 00:41:01.720 |
So let's start with the pragmatic question of is this going to take over our economy? 00:41:05.120 |
And then we'll end with the bigger existential question. 00:41:07.200 |
Is this an alien intelligence that's going to convert us into matrix batteries? 00:41:13.000 |
Is that capability I just described going to severely undermine the economy? 00:41:19.280 |
I think where people get concerned about these Chats GPT type bots in the economy is they 00:41:25.240 |
mistake the fluency with which it can combine styles and subjects with a adaptable fluid 00:41:31.280 |
Well, if it can do that, why can't it do other parts of my job? 00:41:36.740 |
Why can't it build the computer program I need? 00:41:39.820 |
You imagine that you need a human-like flexible intelligence to produce those type of texts 00:41:44.640 |
And flexible human-like intelligence can do lots of things that are in our job. 00:41:48.480 |
There is no flexible human-like intelligence in there. 00:41:50.440 |
There's just the ability to produce passable text with arbitrary combination of known styles 00:41:57.160 |
If we look at what most knowledge workers, for example, do in their job, that capability 00:42:03.120 |
A lot of what knowledge workers do is not writing text. 00:42:07.100 |
It is, for example, interacting with people or reading and synthesizing information. 00:42:11.600 |
When knowledge workers do write, more often than not, the writing is incredibly narrow 00:42:17.520 |
It is specific to the particular circumstances of who they work for, their job, and their 00:42:22.620 |
history with their job, their history with the people they work for. 00:42:25.860 |
I mentioned in my New Yorker piece that as I was writing the conclusion, that earlier 00:42:30.840 |
that same day, I had to co-author an email to exactly the right person in our dean's 00:42:37.380 |
office about a subtle request about how the hiring, faculty hiring process occurs at Georgetown, 00:42:44.520 |
carefully couched, because I wasn't sure if this was the right person, carefully couched 00:42:47.820 |
in language about, "I'm not sure if you're the right person for this, but here's why 00:42:50.360 |
I think, and we talked about, this is why I'm asking about this. 00:42:56.440 |
Writing in GPT, ChatGPT's broad training, could have helped it accomplish that narrow 00:43:02.680 |
And that's most of the writing that knowledge workers actually do. 00:43:07.000 |
And even when we have relatively generic writing or coding or production of text that we need 00:43:12.360 |
a system to do, we run into the problem that ChatGPT and similar chatbots are often wrong. 00:43:21.040 |
Because again, they're just trying to make good guesses for words based on the styles 00:43:29.640 |
These models have no actual model of the thing it's writing about. 00:43:33.720 |
So they have no way of checking, does this make sense? 00:43:39.820 |
It just produces stuff in the style, like what is an answer supposed to more or less 00:43:43.000 |
sound like if it's about this subject in this style? 00:43:46.220 |
This is so pervasive that the developer bulletin board Stack Overflow had to put out a new 00:43:52.840 |
rule that says no answers from ChatGPT can be used on this bulletin board. 00:43:57.840 |
Because what was happening is ChatGPT would be happy to generate answers to your programmer 00:44:05.300 |
But as the moderator of the Stack Overflow board clarified, more often than not, they 00:44:10.640 |
Because ChatGPT doesn't know what a correct program is. 00:44:18.720 |
And based on other code I've seen and the features, this next command makes sense. 00:44:24.600 |
And most of the commands make sense, but it doesn't know what sorting actually means. 00:44:33.560 |
Or that equality isn't quite, you need equality, not just less than or whatever, right? 00:44:38.860 |
It just says given the stuff I've spit out so far and the features I detected from what 00:44:41.720 |
you asked me, and all this code I've looked at, here's a believable next thing to spit 00:44:45.880 |
So it would spit out really believable programs that often didn't work. 00:44:48.480 |
So we would assume most employers are not going to outsource jobs to an unrepentant 00:44:54.320 |
All right, so is it going to be not useful at all in the workplace? 00:44:58.800 |
There'll be very bespoke things I think language models can do. 00:45:02.040 |
It's particularly useful, what we've found in the last few months, where these technologies 00:45:06.600 |
seems to be particularly useful is when you can give it text. 00:45:11.760 |
You can give it text and say, rewrite this in this style or elaborate this. 00:45:18.200 |
So if you're a doctor and you're typing in notes for electronic medical records, it might 00:45:23.760 |
And a model like GPT-4, Chats GPT, might be able to take that and then transform those 00:45:33.600 |
It can do other things like it can gather information for us and collate it in a way 00:45:39.160 |
That's what Microsoft is doing when it's integrating this technology into its Bing search engine. 00:45:45.280 |
So I mean, Google is already pretty smart, but you could have it do a little bit more 00:45:50.000 |
I mean, so there's going to be uses for this, but it is not going to come in and sweep away 00:45:57.040 |
All right, let's get to the final deeper question here. 00:46:03.720 |
Once you understand the architecture, as I just defined it, there is no possible way 00:46:09.960 |
that these large language model based programs can ever do anything that even approximates 00:46:14.480 |
self-awareness consciousness or something we would have to be concerned about. 00:46:19.560 |
There is a completely static definition for these programs once they're trained. 00:46:24.600 |
The underlying parameters of GPT-3, once you train it up, for example, do not change as 00:46:36.320 |
The only thing that changes is the input you give it. 00:46:38.360 |
It goes all the way through these layers in a simple feed forward architecture and spits 00:46:43.600 |
And when you run it through again with a slightly longer request, it's the exact same layers, 00:46:49.400 |
You cannot have anything that approaches consciousness or self-awareness without malleable memory. 00:46:54.160 |
To be alive, by definition, you have to be able to have a ongoing updated model of yourself 00:47:02.100 |
There's no such thing as a static entity where nothing can change. 00:47:08.800 |
There's no memory that changes, nothing in it changes that you would consider to be alive. 00:47:13.520 |
So no, this model is not the right type of AI technology that could ever become self-aware. 00:47:20.020 |
There's other models in the AI universe that could be where you actually have notions of 00:47:24.320 |
maintaining and updating models of learning, of thinking about yourself, interacting with 00:47:30.920 |
the world, having incentives, having multiple actions you can take. 00:47:33.920 |
You can build systems that in theory down the line could be self-aware. 00:47:45.120 |
We've created this really cool large language model. 00:47:52.240 |
It's really good at talking to people, so it's easy to use and you can share all these 00:47:57.240 |
This general technology, one way or the other, will be integrated more and more into our 00:48:00.840 |
working lives, but it's going to have the impact, in my opinion, more like Google had 00:48:04.740 |
once that got really good, which was a big impact. 00:48:07.000 |
You can ask Google all these questions, how to define words. 00:48:10.520 |
It really helped people, but it didn't make whole industries disappear. 00:48:13.920 |
I think that's where we're going to be with these large language models. 00:48:17.320 |
They can produce text on arbitrary combinations of known subjects using arbitrary combination 00:48:21.760 |
of known styles where known means they've seen it a sufficient number of times in their 00:48:28.360 |
This is not an alien intelligence that is going to, as was warned in that New York Times 00:48:33.480 |
op-ed, deploy sophisticated propaganda to take over our political elections and create 00:48:41.860 |
This is not going to get rid of programming as a profession and writing as a profession. 00:48:46.480 |
It is cool, but it is not, in my opinion, an existential threat. 00:48:52.720 |
What's transformative in the world of AI probably will not be in the immediate future transformative 00:49:00.160 |
So, Jesse, there's my professor sermon for the day. 00:49:06.280 |
>> COREY: You don't want to get me started on computer science lectures because I could 00:49:09.320 |
fall into my old habits, my professorial habits, and really bore you. 00:49:16.240 |
>> JESSE: So how many rules will there be in five years? 00:49:19.640 |
>> COREY: I don't know how much bigger it can get. 00:49:25.040 |
So GPT-2 had some of the largest number of parameters before GPT-3 came out. 00:49:29.200 |
It had 17 billion or something like this, and GPT-3 has 170 billion. 00:49:34.000 |
I was talking to an expert at MIT about this. 00:49:36.920 |
The issue about making this too much larger is they're already sort of giving it all of 00:49:45.880 |
And so at some point, you're not going to get back bigger returns. 00:49:53.360 |
If you make your networks too small, they're not complicated enough to learn enough rules 00:49:58.060 |
But if you make them too large, you're wasting a lot of space. 00:50:00.000 |
You're just going to have a lot of redundancy. 00:50:01.560 |
I mean, it can only learn what it sees in its dataset. 00:50:06.140 |
So if 175 billion parameters is well fit to this massive training data that we use for 00:50:12.400 |
these chatbots, then just increasing the size of the network is not going to change much. 00:50:17.200 |
You would have to have a correspondingly larger and richer training dataset to give it. 00:50:23.560 |
And I don't know how much more, at least for this very particular problem of producing 00:50:28.200 |
text, I don't know how much more richer or larger of a dataset we could give it. 00:50:31.880 |
I actually think what the direction happening now is how do we make these things smaller 00:50:40.280 |
175 billion parameters can't fit in the memory of a single GPU. 00:50:43.840 |
You probably need five different specialized pieces of hardware just to generate a single 00:50:51.440 |
That means if everyone at my office is constantly making requests to GPT-3 as part of their 00:50:55.920 |
work, we're going to have this huge computing bill. 00:50:58.340 |
So actually a lot of the effort is in how do we make these things smaller? 00:51:01.600 |
Just focus on the examples that are relevant to what these people actually need. 00:51:05.840 |
We want it eventually to have models that can fit in a phone and still do useful things. 00:51:10.880 |
So GPT-3 I think was, and that's what all these other ones are based off of, that was 00:51:15.440 |
open AI saying what happens if we make these things much bigger? 00:51:20.120 |
And now we're going to go back to make them smaller. 00:51:21.400 |
And if you actually read the original GPT-3 paper, their goal with making it 10 times 00:51:27.160 |
bigger was not that it was going to have in a particular domain 10 times better answers. 00:51:32.480 |
They wanted to have one model that could do well in many unrelated tasks. 00:51:37.060 |
And if you read the paper, they say, look, here's a bunch of different tasks for which 00:51:41.660 |
we already have these large language models that do well, but they're customized to these 00:51:49.720 |
And what they were proud about with GPT-3, if you read the original paper, is this one 00:51:56.840 |
It's not that it was actually doing much better than the state of the art in any one of these 00:52:00.400 |
You don't need necessarily to hand train a model for each task. 00:52:04.000 |
If you make it big enough, it can handle all the different tasks. 00:52:06.120 |
So it wasn't getting 10 times larger did not make GPT-3 10 times better at any particular 00:52:12.440 |
In fact, in most tasks, it's as good as the best, but not much better. 00:52:15.560 |
It was the flexibility and the broadness, but that's good to see. 00:52:23.720 |
But going forward, the name of the game, I think is going to go back to, actually, we 00:52:26.800 |
need to make these things smaller so that we can not have to use an absurd amount of 00:52:32.120 |
computational power just to figure out that dog should follow the quick brown fox jumped 00:52:39.240 |
We need to maybe be a little bit more efficient. 00:52:47.960 |
I think once you open this, it's just not as worrisome. 00:52:50.760 |
When it's a black box, you can imagine anything. 00:52:54.480 |
I definitely like that Yuval Harari op-ed was definitely influenced by Nick Bostrom's 00:52:58.840 |
super intelligence, which we talked about on the show a few months ago, where he just 00:53:03.600 |
He's a philosopher, not a computer scientist. 00:53:13.440 |
And he was like, "Well, if it got smart, it could make itself smarter, and then it 00:53:16.120 |
can make itself even smarter, and it become a super intelligence." 00:53:18.360 |
And then they have all these scenarios about, "Well, if we had a super intelligent thing, 00:53:22.440 |
Because it'd be so smart and understand us so well that it could have the perfect propaganda. 00:53:27.640 |
And now the bot could get us all to do whatever it wanted us to do." 00:53:33.920 |
You open up these boxes, and you see 175 billion numbers being multiplied by GPUs doing 1.5 00:53:41.560 |
million books worth of pattern detection vote rules to generate a probability vector so 00:53:51.080 |
I have a few questions I want to get to from you, my listeners, that are about artificial 00:53:56.400 |
First, I want to mention one of the sponsors that makes this nonsense possible. 00:53:59.040 |
And we're talking about our friends at ZocDoc. 00:54:01.800 |
ZocDoc is the only free app that lets you find and book doctors who are patient reviewed, 00:54:08.160 |
take your insurance, and are available when you need them to treat almost every condition 00:54:15.840 |
So if you need a doctor, instead of just saying, "I'll ask a friend," or, "I'll look it up 00:54:20.160 |
in the yellow pages," you can instead go to ZocDoc, your ZocDoc app, and it'll show you 00:54:26.600 |
doctors who are nearby that take your insurance. 00:54:30.640 |
And then once you sign up with these doctors, they'll often use ZocDoc to make it easier 00:54:33.840 |
for you to set up appointments, get reminders about appointments, send in paperwork. 00:54:39.400 |
Both my dentist and my primary care physician use ZocDoc, and I find it really useful because 00:54:44.880 |
all of my interactions with them happen to that interface. 00:54:47.800 |
So go to ZocDoc.com/deep and download the ZocDoc app for free. 00:54:52.120 |
Then find a book, a top rated doctor today, many who are available within 24 hours. 00:54:56.520 |
That's Z-O-C-D-O-C.com/deep, ZocDoc.com/deep. 00:55:08.000 |
As we often talk about when it comes to the different buckets relevant to cultivating 00:55:13.920 |
a deep life, the bucket of contemplation is in there. 00:55:19.440 |
Having an active and healthy life of the mind is critical to a life that is deep in many 00:55:26.960 |
It is easy, however, due to various events in life to fall into an area where your relationship 00:55:33.520 |
Maybe you find yourself overwhelmed with anxious thoughts or ruminations or depressive moments 00:55:42.040 |
Whatever it is, it is easy for your mind to get out of kilter. 00:55:45.560 |
Just like if your knee started hurting, you would go to an orthopedist. 00:55:50.120 |
If your mind started hurting, and by the way, there's really loud construction sound going 00:55:56.320 |
This is the restaurant below us is being made. 00:56:00.120 |
But returning to BetterHelp, let me add this example. 00:56:05.420 |
If you find yourself becoming increasingly enraged because of restaurant construction 00:56:10.880 |
noise that occurs during your podcast, among the other things that could happen to affect 00:56:19.800 |
Therapists helps make sure that your cognitive life gets healthy, gets resilient, gets back 00:56:25.880 |
If you live in a big city, all the ones near you might be booked or they might be really 00:56:35.120 |
If you're thinking about starting therapy, BetterHelp is a great way to give it a try 00:56:41.440 |
It's designed to be convenient, flexible, and suited to your schedule. 00:56:44.640 |
You just fill out a brief questionnaire to get matched with a licensed therapist, and 00:56:49.040 |
you can switch therapists anytime for no additional charge. 00:56:57.120 |
Visit BetterHelp.com/deepquestions, one word, today to get 10% off your first month. 00:57:03.680 |
That's BetterHelp, H-E-L-P.com/deepquestions. 00:57:10.760 |
I hope this restaurant's good, Jesse, after all the disruption. 00:57:24.680 |
It's an old character from Tacoma Park history. 00:57:30.320 |
I heard I was talking to the guy, he thought May. 00:57:39.000 |
First question is from Manav, a student at Yale. 00:57:43.200 |
Looking at tools like ChatGPT makes me feel like there's nothing AI won't eventually do 00:57:48.880 |
This fear makes it hard to concentrate on learning since it makes me feel that there 00:57:56.960 |
Well, Manav, hopefully my deep dive is helping dispel those fears. 00:58:03.560 |
I want to include this question in part to emphasize the degree to which the hype cycle 00:58:08.200 |
around these tools has really been unhelpful. 00:58:12.120 |
Because you can so easily embed screenshots of interactions of ChatGPT, a lot of people 00:58:19.000 |
started trying it because the attraction of virality is very strong for lots of people 00:58:24.880 |
So it brought ChatGPT to the awareness of a lot of people and generated a lot of attention. 00:58:30.160 |
Now once we had a lot of attention, how do you one up that attention? 00:58:33.000 |
Well, then you start thinking about worries about it. 00:58:35.120 |
You start thinking about what if it could do this, what if it could do that? 00:58:38.440 |
From what I understand, I'm not as plugged into these online worlds as others, but there's 00:58:42.240 |
a whole tech bro push during the last few months that was increasingly trying to push. 00:58:46.560 |
It can do this, it can do that, it can do this. 00:58:49.000 |
Exactly the same tonality with which the same group talked about crypto two years ago. 00:58:53.440 |
Nothing is going to be done by ChatGPT, just like currency will be gone in three years 00:59:02.240 |
And everyone's trying to one up each other and do YouTube videos and podcasts about, 00:59:06.840 |
And then this created this weird counter reaction from the mainstream media, because the mainstream 00:59:12.760 |
media has a, right now, an adversarial relationship with the Silicon Valley tech bro crowd. 00:59:19.520 |
So then they started pushing back about, "No, it's going to be bad. 00:59:22.720 |
No, no, these tech bros are leading us to a world where we're going to be able to cheat 00:59:31.520 |
It's going to take over the government and become super intelligent." 00:59:35.080 |
So they started the counteraction to the overblown enthusiasm of the tech bros became an overblown 00:59:40.880 |
grimness from the anti-tech bro mainstream media. 00:59:45.960 |
All of it fed into Twitter, which like a blender was mixing this all together and swirling 00:59:50.240 |
this spiral of craziness higher and higher until finally just the average person like 00:59:54.400 |
Manav here at Yale is thinking, "How can I even study knowing that there will be no 00:59:59.400 |
jobs and we'll be enslaved by computers within the next couple of years?" 01:00:03.400 |
All right, so Manav, hopefully my deep dive helped you feel better about this. 01:00:08.280 |
Chats GPT can write about combinations of known subjects and combinations of known styles. 01:00:16.920 |
It has no state or understanding or incentives. 01:00:20.160 |
You ask it the right about removing a peanut butter sandwich from a VCR. 01:00:23.160 |
It does not have an internal model of a VCR in a sandwich on which it's experimenting 01:00:26.520 |
with different strategies to figure out which strategy works best and then turns to its 01:00:32.760 |
It just sees peanut butter as a possible next word to spit out and you ask about peanut 01:00:37.080 |
butter and your response, so it puts more votes on it. 01:00:39.440 |
Mixing, matching, copying, manipulating existing human text, the humor and the jokes it spits 01:00:44.800 |
out, the accuracy and the styles it uses are all intelligence borrowed from the input that 01:00:51.680 |
It does not have a broad adaptable intelligence that can in any significant sense impact the 01:01:00.960 |
It's important to emphasize, Manav, it's not like we're one small step from making these 01:01:04.880 |
models more flexible, more adaptable, able to do more things. 01:01:11.280 |
The key to chat CPT being so good at the specific thing it does, which is producing text in 01:01:15.600 |
known styles on known subjects, is that it had a truly massive amount of training data 01:01:20.640 |
We could give it everything anyone had ever written on the internet for a decade and it 01:01:26.240 |
This is the problem when you try to adapt these models to other types of activities 01:01:33.160 |
You say, "What I really want is a model that can work with my databases. 01:01:38.560 |
What I really want is a model that can send emails and attach files on my behalf." 01:01:44.320 |
The problem is you don't have enough training data. 01:01:47.600 |
You need training data where you have billions of examples of, "Here's the situation, here's 01:01:53.360 |
And then like most things that we do, we learn after a small number of examples. 01:01:57.920 |
A model to do other activities other than produce text needs a ton of data. 01:02:01.240 |
And in most other types of genres or activities, there's just not that much data. 01:02:04.640 |
So one of the few examples where there is, is art production. 01:02:09.240 |
You can give it a huge corpus of pictures that are annotated with text and it can learn 01:02:14.760 |
these different styles and subject matters that show up in pictures then produce original 01:02:18.920 |
But that's one of the few other areas where you have enough uniform data that it can actually 01:02:24.880 |
So I'm not worried that Manav, like all of our jobs will be gone. 01:02:29.120 |
You can rest easy, study harder for your classes. 01:02:37.800 |
It seems almost inevitable that in 10 years, AI will be able to perform many knowledge 01:02:44.400 |
Should we be worried about the pace of automation and knowledge work and how can we prepare 01:02:48.440 |
our careers now for increased power AI in the coming decades? 01:02:52.980 |
- So as I just explained in the last question, this particular trajectory of AI technology 01:03:00.020 |
There is however, and this is why I included this question. 01:03:03.260 |
There is however, another potential intersection of artificial intelligence and knowledge work 01:03:07.640 |
that I've been talking about for years that I think we should be more concerned about 01:03:17.480 |
The place where I think AI is gonna have the big impact is less sexy than this notion of 01:03:22.880 |
I just have this blinking chat cursor and I can ask this thing to do whatever I want. 01:03:26.520 |
Now where it's really gonna intersect is shallow task automation. 01:03:32.680 |
So the shallow work, the stuff we do, the overhead we do to help collaborate, organize, 01:03:37.800 |
and gather the information need for the main deep work that we execute in our knowledge 01:03:43.240 |
More and more of that is gonna be taken over by less sexy, more bespoke, but increasingly 01:03:49.880 |
And as these tools get better, I don't have to send 126 emails a day anymore because I 01:03:55.640 |
can actually have a bespoke AI agent handle a lot of that work for me, not in a general 01:04:00.440 |
it's intelligent sense, but in a much more specific like talking to Alexa type sense. 01:04:05.820 |
Can you gather the data I need for writing this report? 01:04:10.680 |
Can you set up a meeting next week for me with these three principles? 01:04:15.260 |
And then that AI agent talks to the AI agents of the three people you need to set the meeting 01:04:19.840 |
up with and they figure out together and put that meeting onto the calendar so that none 01:04:24.860 |
of us, three of us have to ever exchange an email. 01:04:27.040 |
The information it gathers from the people who have it by talking to their AI agents, 01:04:34.520 |
It's able to do these rote tasks for us, right? 01:04:38.820 |
This was actually a future that I was exposed to a decade earlier. 01:04:42.040 |
I spoke at an event with the CEO of a automated meeting scheduling company called X.AI. 01:04:47.640 |
I remember him telling me, this is the future. 01:04:51.240 |
When you have a AI tool that can talk to another person's AI tool to figure out logistical 01:04:55.880 |
things on your behalf so that you're never interrupted. 01:04:59.000 |
I think that's where the big AI impact is going to come. 01:05:04.800 |
What it does is it automates away the stuff that gets in the way of your main work. 01:05:09.160 |
Because it will immensely increase the amount of your main work you're able to get done. 01:05:14.520 |
If you're not context switching once every five minutes, which is the average time the 01:05:18.200 |
average knowledge worker spends between email or instant messenger chat checks, if you're 01:05:21.720 |
not doing that anymore, you know how much you're going to get done? 01:05:26.160 |
You know how much if you can just do something until you're done, and then the AI agents 01:05:29.760 |
on your computer says, "Okay, we got the stuff for you for the next thing you need 01:05:34.280 |
And you have to have no overhead communicating or collaborating, trying to figure out what 01:05:39.800 |
I would say probably three to four X more of the meaningful output that you produce 01:05:43.680 |
in your job will be produced three to four X more if these unsexy bespoke AI logistical 01:05:53.640 |
So this has this huge potential benefit and this huge potential downside. 01:05:57.200 |
The benefit of course is your work is less exhausting. 01:06:01.200 |
Companies are going to generate a lot more value. 01:06:03.000 |
The downside is that might greatly reduce the number of knowledge workers required to 01:06:12.040 |
If three knowledge workers now produce what it used to take 10, I could grow my company 01:06:18.040 |
or I could fire seven of those knowledge workers. 01:06:20.440 |
So I think this is going to create a disruption. 01:06:23.080 |
We underestimate the degree to which shallow work and context shifting is completely hampering 01:06:30.880 |
But because it's like the pot that keeps getting hotter until the lobster is boiled, because 01:06:35.400 |
it's inflecting everybody, we don't realize how much we're being held back. 01:06:39.160 |
When computer tools aided by AI remove that, it's going to be a huge disruption. 01:06:43.480 |
And I think ultimately the economy is going to adapt to it. 01:06:48.420 |
The knowledge industry is going to explode in size and scope as we can unlock all this 01:06:52.320 |
cognitive potential on new types of challenges or problems that we weren't thinking about 01:06:56.720 |
Ultimately, it'll probably be good and lead to a huge economic growth. 01:06:59.780 |
But I think there's going to be a disruption period because we really are at such, again, 01:07:05.840 |
we just don't emphasize the degree to how inefficient we are and how much if we could 01:07:09.460 |
remove that inefficiency, we don't need most of the people sitting here in this office 01:07:14.360 |
Getting over that, that's going to be the real disruption. 01:07:16.880 |
And there's no scary how from 2001 type tool involved here. 01:07:21.840 |
Meeting, information scheduling, basically whatever you type in an email, it could do 01:07:29.400 |
I don't know when it's coming, but that's coming soon. 01:07:39.920 |
All right, next question is from Ben, a Silicon Valley engineer. 01:07:44.640 |
I've decided that web development freelancing will be the best possible career path to achieve 01:07:50.840 |
And I plan to freelance in addition to my full-time job until freelancing can support 01:07:57.200 |
Over the last few weeks, however, I've been hearing about the breakthroughs of chat, GPT 01:08:02.560 |
Do you think I should stay on the path of learning the ropes of freelancing web development 01:08:06.600 |
or should I focus more on the future of technology and try to stay ahead of the curve? 01:08:11.560 |
Well, Ben, I'm using the inclusion of AI in your question to secretly get in an example 01:08:18.000 |
of lifestyle-centric career planning, which you know I like to talk about. 01:08:24.080 |
You're working backwards from a vision of what you want you and your family's life to 01:08:27.760 |
be like, a tangible lifestyle, not specific in what particular job or city, but the attributes 01:08:36.880 |
So you're working backwards to say, what is a tractable path from where I am to accomplish 01:08:43.680 |
And you're seeing here, web development could be there, freelance web development. 01:08:47.040 |
And I don't know all the details of your plan, but I'm assuming you probably have a plan 01:08:50.200 |
where you're living somewhere that is cheaper to live. 01:08:52.840 |
Maybe it's more outside or country oriented where your expenses are lower. 01:08:57.200 |
And because web development is a relatively high reward per hour spent type activity, 01:09:02.280 |
that strategic freelancing could support your lifestyle there while giving you huge amounts 01:09:06.760 |
And satisfying the various properties that I assume you figured out about what you want 01:09:10.520 |
in your life, these sort of non-professional properties. 01:09:13.920 |
I also really applaud the fact that you're applying the principle of letting money be 01:09:21.640 |
There's a strategy I talked about in my book, So Good They Can't Ignore You. 01:09:25.960 |
This is a strategy in which instead of just jumping into something new, you try it on 01:09:30.800 |
the side and say, can I actually make money at this? 01:09:34.300 |
The idea here is that people paying you money is the most unbiased feedback you will get 01:09:39.400 |
about how valuable or viable the thing you're doing actually is. 01:09:46.680 |
I mean, I want to see that people are hiring me and this money is coming in before I quit 01:09:52.800 |
Don't just ask people, hey, is this valuable? 01:09:57.080 |
When the dollars coming in are enough to more or less support your new lifestyle, you then 01:10:10.640 |
Should you stop this plan so you can focus more on the future of technology and try to 01:10:16.440 |
I mean, I don't even really know what that means. 01:10:18.840 |
My main advice would be whatever skill it is you're learning, make sure you're learning 01:10:24.500 |
Get real feedback from real people in this field about what is actually valuable and 01:10:28.140 |
how good you have to be to unlock that value. 01:10:30.280 |
So I would say that don't invent your own story about how you want this field to work. 01:10:36.560 |
Don't assume that if you know HTML and a little CSS, you're going to be living easy. 01:10:41.480 |
What are the actual skills people care about? 01:10:46.320 |
How good you have to be at that to actually be desirable to the marketplace? 01:10:49.800 |
Get hard, verified answers to those questions. 01:10:52.580 |
That's what I would say when it comes to staying ahead of the curve. 01:10:55.800 |
And as for some sort of fear that you becoming a web developer is quixotic because chat GPT 01:11:05.760 |
So yes, make sure you're learning the right skills, not the skills you want to be valuable. 01:11:10.120 |
But there's nothing wrong with this particular plan you have. 01:11:15.720 |
I also appreciate, I cut it, Jesse, but Ben had a joke in the beginning of his response. 01:11:22.880 |
He said, "I've been doing lifestyle-centric career planning. 01:11:28.360 |
So what we're going to do is quit and I'm going to start a cattle ranch." 01:11:34.560 |
All right, let's do one more question before I kind of run out of the ability to talk about 01:11:44.040 |
It's been months that people have been asking us about AI. 01:11:48.720 |
Next week, we're going to talk all about, I don't know, living in the country or minimalism, 01:11:56.000 |
not using social media, but we're getting all the AI out of our system today. 01:12:02.520 |
"AI can solve high school level math word problems. 01:12:06.080 |
AI can explain why a joke that has never seen before is funny. 01:12:11.400 |
All this points to mass job loss within five years. 01:12:18.360 |
The current trajectory of AI is not going to create mass job loss in the next five years. 01:12:26.760 |
It writes jokes that are funny because it knows what part of a script you can identify 01:12:33.880 |
And then it upvotes words from those parts of scripts when it's doing the word guessing 01:12:39.000 |
And as a result, you get jokes that pull from existing structures of human and existing 01:12:46.080 |
You can see that in part if you look at that Seinfeld scene with bubble sort I talked about 01:12:53.720 |
There's non-sequitur jokes in there, things that are described as the audience laughing 01:12:58.800 |
And that's because it's not actually looking at its script and saying, "Is this funny?" 01:13:02.840 |
It's guessing words, guessing words that things are accurate. 01:13:08.720 |
I want to use this as an excuse to talk about another trend in AI that I think is probably 01:13:13.980 |
more important than any of these large language models that also is not getting talked about 01:13:19.520 |
So we talked about an earlier question, AI shallow work automation has been critical. 01:13:22.760 |
The other major development that we're so used to now we forget, but I think is actually 01:13:27.840 |
going to be the key to this AI shallow work automation, but also all sorts of other ways 01:13:33.160 |
that AI interests our life is not these large language models, but it's what Google has 01:13:44.580 |
These at-home appliances that you can talk to and ask to do things, they're ubiquitous 01:13:50.760 |
and they're ubiquitous in part because these companies made them very cheap. 01:13:55.320 |
They're not trying to make a lot of money off them. 01:13:58.080 |
Why is Google or Amazon trying to get as many people as possible to use these agents at 01:14:01.680 |
home that you could just talk to and it tries to understand you? 01:14:05.880 |
The game they are playing is we want millions and millions of different people with different 01:14:09.760 |
accents and different types of voices asking about all sorts of different things that we 01:14:16.320 |
And we could then use this data set to train increasingly better interfaces that can understand 01:14:29.120 |
They have a human semi-supervised, semi-human supervised reinforcement learning model that 01:14:33.760 |
they inserted during the GPT-3 training to try to align its responses better with what's 01:14:40.180 |
But this is the whole ballgame is just natural language understanding. 01:14:44.740 |
And Google is working on this and Amazon is working on this and Apple is working on this 01:14:49.960 |
And this is what matters, understanding people. 01:14:54.080 |
The background activities, I think this is what we often get wrong. 01:14:58.240 |
The actual activities that the disruptive AI in the future is going to do on our behalf 01:15:06.640 |
It's we're going to pull some data from an Excel table and email it to someone. 01:15:11.920 |
It's we're going to turn off the lights in your house because you said you were gone. 01:15:19.080 |
All of the interesting stuff is understanding what you're saying. 01:15:21.880 |
And that's why Google and Amazon and Apple invested so much money into getting these 01:15:25.080 |
devices everywhere is they want to train and generate the biggest possible data set of 01:15:29.280 |
actually understanding what real people are saying and figuring out, did we get it right 01:15:33.280 |
And can we look at examples and let's hand annotate these examples and figure out how 01:15:38.680 |
And I think this is really going to be the slow creep of AI disruption. 01:15:42.040 |
It's not going to be this one entity that suddenly takes over everyone's job. 01:15:45.560 |
It's going to be that more and more devices in our world are increasingly better at understanding 01:15:49.280 |
natural language questions, whether it be typed or spoken, and can then act accordingly, 01:15:56.360 |
We don't really need these agents to do really complicated things. 01:15:58.720 |
We just need them to understand what we mean. 01:16:02.040 |
Most of what we do, that's a drag on our time, it's a drag on our energy, it's pretty simple. 01:16:06.640 |
And it's something a machine could do if they just knew what it was we wanted. 01:16:10.480 |
And so I think that's where we should be focusing is interfacing is what matters. 01:16:15.640 |
These 175 billion parameter models that can generate all this text is really not that 01:16:22.080 |
I don't need a Seinfeld script about bubble sort. 01:16:26.080 |
I need you to understand me when I say, give me the email address of all of the students 01:16:33.560 |
I need you just to understand what that means and be able to interface with the student 01:16:38.400 |
database and get those emails out and format it properly so I can send the message to the 01:16:44.840 |
I don't want you to write an original poem in the style of Mary Oliver about a hockey 01:16:55.080 |
I need you to just go through when I say, look at the assignment pages for the problem 01:17:00.200 |
sets I assigned this semester, pull out the grading statistics and put them all into one 01:17:05.800 |
document just to kind of, okay, I know what that means. 01:17:08.080 |
And now I'm doing a pretty rote automatic computer type thing. 01:17:11.640 |
And I don't care if you come back and say, okay, I have three follow-up questions. 01:17:15.920 |
You mean this section, that section, this section, that's fine. 01:17:17.480 |
They'll just take us another 10 or 15 seconds. 01:17:19.800 |
I don't need, in other words, how from 2001, I just need my basic computer to understand 01:17:27.520 |
So I don't have to type it in or click on a lot of different buttons. 01:17:29.440 |
So I mean, I think that's really where we're going to see the big innovations is the slow 01:17:32.800 |
creep of better and better human understanding plugged into relatively non-interesting actions. 01:17:39.000 |
That's really where the stuff's going to take a bigger and bigger picture. 01:17:43.680 |
This idea that it's now this all at once large language model represents, we have this new 01:17:49.280 |
self-sufficient being that all at once we'll do everything. 01:17:52.800 |
It's sexy, but I don't think that's the way this is going to happen. 01:17:57.840 |
I want to do something interesting to wrap up the show. 01:18:01.240 |
First, I want to mention one of the longtime sponsors that makes Deep Questions possible. 01:18:06.680 |
When you subscribe to Blinkist, you get access to 15 minute summaries of over 5,500 nonfiction 01:18:15.960 |
These short summaries, which are called Blinks, you can either read them or you can listen 01:18:22.520 |
Jesse and I both use Blinkist as one of our primary tools for triaging which books we 01:18:29.800 |
If there is a book we are interested in, we will read or listen to the Blink to see if 01:18:36.760 |
The other week, Jesse and I went through his own Blinkist list and we calculated that basically 01:18:41.360 |
what it was roughly what 30%, I think you said 30% of the books for which you read Blinkist 01:18:48.240 |
That is a case in point of the value of Blinkist. 01:18:51.520 |
It really helps you hone in on the right books to buy. 01:18:54.560 |
The books you don't buy, you still know their main ideas. 01:18:58.080 |
Blinkist is really a tool that any serious reader needs to add to their tool kit. 01:19:04.680 |
Right now, Blinkist has a special offer just for our audience. 01:19:06.880 |
Go to Blinkist.com/deep to start your seven-day free trial and you will get 45% off a Blinkist 01:19:17.040 |
Blinkist.com/deep to get 45% off any seven-day free trial. 01:19:27.480 |
Even better news, for a limited time, you can take advantage of their Blinkist Connect 01:19:36.080 |
You will, in other words, get two premium accounts for the price of one. 01:19:38.960 |
So when you subscribe to Blinkist, you can give an account to a friend who you think 01:19:53.360 |
Tax season is when I often get stressed about putting things off because I don't even know 01:19:59.040 |
This got me thinking about the other classic thing that people put off, which is getting 01:20:05.360 |
This is one of those things that you know you need, but you don't know where to get 01:20:14.000 |
You're going to have to go to a doctor and get blood drawn and your eyeball scanned before 01:20:20.920 |
And we get paralyzed by all this complexity and say, forget it. 01:20:32.240 |
It's 100% digital, no doctors, no needles, and no paperwork. 01:20:35.560 |
When you're applying for $3 million in coverage or less, you just answer a few questions about 01:20:41.760 |
You need just a few minutes in a phone or laptop to apply. 01:20:44.800 |
Latter smart algorithms work in real time, so you'll find out instantly if you're approved. 01:20:50.600 |
No hidden fees, cancel any time, get a full refund if you change your mind in the first 01:20:56.720 |
Life insurance is only going to cost more as you get older, so now is always the right 01:21:02.320 |
So go to ladderlife.com/deep today to see if you're instantly approved. 01:21:15.400 |
All right, Jesse, let's do something interesting. 01:21:20.500 |
For those who are new to the show, this is the segment where I take something that you 01:21:24.400 |
sent me to my interesting@calnewport.com email address that you thought I might find interesting. 01:21:31.360 |
I take something that caught my attention and I share it. 01:21:37.180 |
This is actually from just a couple of days ago. 01:21:39.520 |
I have it up on the screen now, so if you're listening, you can watch this at youtube.com/calnewportmedia 01:21:46.540 |
It is an article from NPR with the following music to my ears headline, NPR quits Twitter. 01:22:02.860 |
NPR is having a basically a feud with Twitter because Twitter started labeling the network 01:22:12.120 |
The same description they use for propaganda outlets in Russia, China, and other autocratic 01:22:20.880 |
So then they changed it and said, okay, well, we'll call you government funded media. 01:22:24.520 |
But NPR said only 1% of our annual budget comes from federal funding. 01:22:33.540 |
And they put NPR politics, for example, put out a tweet that said all of our 52 Twitter 01:22:39.140 |
accounts, uh, we're not going to use them anymore. 01:22:42.380 |
You want news for NPR, subscribe to our email newsletter, come to our website, listen to 01:22:50.500 |
You don't have to use this other guy's program. 01:22:53.700 |
I really like to see that not because of the inter-scene political battles between Musk 01:22:59.780 |
and the political, the different media outlets. 01:23:03.180 |
I mean, I wish they would just make this move even without that, but whatever gets them 01:23:08.580 |
As I've talked about so many times on this show before, it is baked into the architecture 01:23:12.580 |
of Twitter that it is going to generate outrage. 01:23:19.300 |
It is going to create tribalism and is going to really color your understanding of the 01:23:26.500 |
world, your understanding of current events in ways that are highly inaccurate and often 01:23:32.260 |
It's just built into the collaborative curation mechanism of all these retweets combined with 01:23:42.620 |
It's not a great way to consumer share information. 01:23:49.020 |
So a couple of weeks ago, we gave the example of the Washington post nationals baseball 01:23:54.580 |
team coverage shifting away from live tweeting the games and instead having live updates 01:24:01.420 |
And at the end of all those updates, then they write a recap article and it's all packaged 01:24:04.660 |
together and you can see how it unfolded and they have more different people writing and 01:24:10.740 |
There's no reason to be on someone else's platform, mixing tweets about the baseball 01:24:14.580 |
game with tweets about, you know, the Martian that's going to come and destroy the earth 01:24:22.260 |
because you didn't give it hydroxychloroquine or whatever the hell else is going on on Twitter. 01:24:35.780 |
I think we would be in a much calmer place as individuals. 01:24:39.620 |
I think we'd be in a much calmer place politically. 01:24:41.940 |
I think we'd be in a much calmer place just from a civic perspective. 01:24:46.260 |
If more and more sources of news, if more and more sources of expression, if more and 01:24:50.220 |
more sources of commentary move to their own private gardens, here's my website, here's 01:24:55.700 |
my podcast, here's my newsletter, not this giant mixing bowl where everyone and everything 01:25:05.940 |
And we have this distributed curation mechanism rapidly amplifying some things versus others. 01:25:10.740 |
That's not a healthy way for a society to learn about things and communicate. 01:25:17.620 |
And I wrote about this in Wired Magazine early in the pandemic. 01:25:22.300 |
I said one of the number one things we could do for public health right now at the beginning 01:25:29.380 |
And I gave this argument in that article that, look, if professionals, if we retreated to 01:25:35.500 |
websites, we could have considered long form articles with rich links in the other types 01:25:42.340 |
of articles and sources, where the websites themselves, you could indicate authority by 01:25:50.020 |
the fact that this website is hosted at a hospital or a known academic institution or 01:25:56.340 |
We'd be able to curate on an individual sense of this website is at, the reference in old 01:26:03.500 |
SNL skit, clownpenis.fart, and it has weird gifs of it of eagles that are flying away 01:26:10.900 |
I'm not going to pay as much attention to that, to this long form article that's coming 01:26:16.960 |
And I said, if we went back, humans will be much better at consuming information. 01:26:20.620 |
The information will be much better presented if we went back to more bespoke distributed 01:26:23.700 |
communication, as opposed to having everyone, the cranks and the experts and the weirdos 01:26:29.320 |
and everybody all mixed together in the exact same interface with the exact same, their 01:26:32.580 |
tweets look exactly the same, and a completely dispassionate distributed curation mechanism, 01:26:37.660 |
rapidly amplifying things that are catching people's attention. 01:26:42.760 |
We need to get away from that distributed curation. 01:26:44.340 |
And we get back to more bespoke things where we can learn from where you hosted, what does 01:26:53.820 |
Anyways, I don't want to go too far into this lecture, but basically this is a good trend. 01:26:57.820 |
I think individually owned digital distribution of information, podcasts, websites, blogs, 01:27:06.940 |
and news is going to be a much healthier trend than saying, why don't we all just have one 01:27:15.160 |
I hope more and more news sources follow your lead in the Washington Post's, Nationals, 01:27:21.100 |
Do you know the clown penis dot fart reference? 01:27:26.180 |
It was a classic SNL skit and it was like an advertisement and they really had it. 01:27:32.500 |
You know how they used to have those advertisements for brokerage firms? 01:27:35.180 |
Where it's like super, I welcome, it's like Wilford Brimley, like welcome to such and 01:27:40.700 |
such financial partners where trust is our number one, whatever. 01:27:44.540 |
And so it's like this real serious ad and they're like, you can find out more at our 01:27:52.300 |
And then they kind of reveal by the time we set up our website, it was the only address 01:27:58.860 |
So it was like the premise of it, it was like this really serious financial brokerage firm, 01:28:02.100 |
but in the late nineties, it felt like all the URLs were gone. 01:28:05.540 |
And so it was like the only URL that was left. 01:28:07.140 |
And so it was just a very serious commercial that they kept having to say clown penis dot 01:28:19.380 |
I'm happy now to talk about AI whenever you want. 01:28:21.400 |
My gag order has been lifted, but maybe it'll be a while until we get too much more deeply 01:28:28.460 |
We'll be back next week with the next, hopefully much less computer science filled episode