back to indexTuring Test: Can Machines Think?
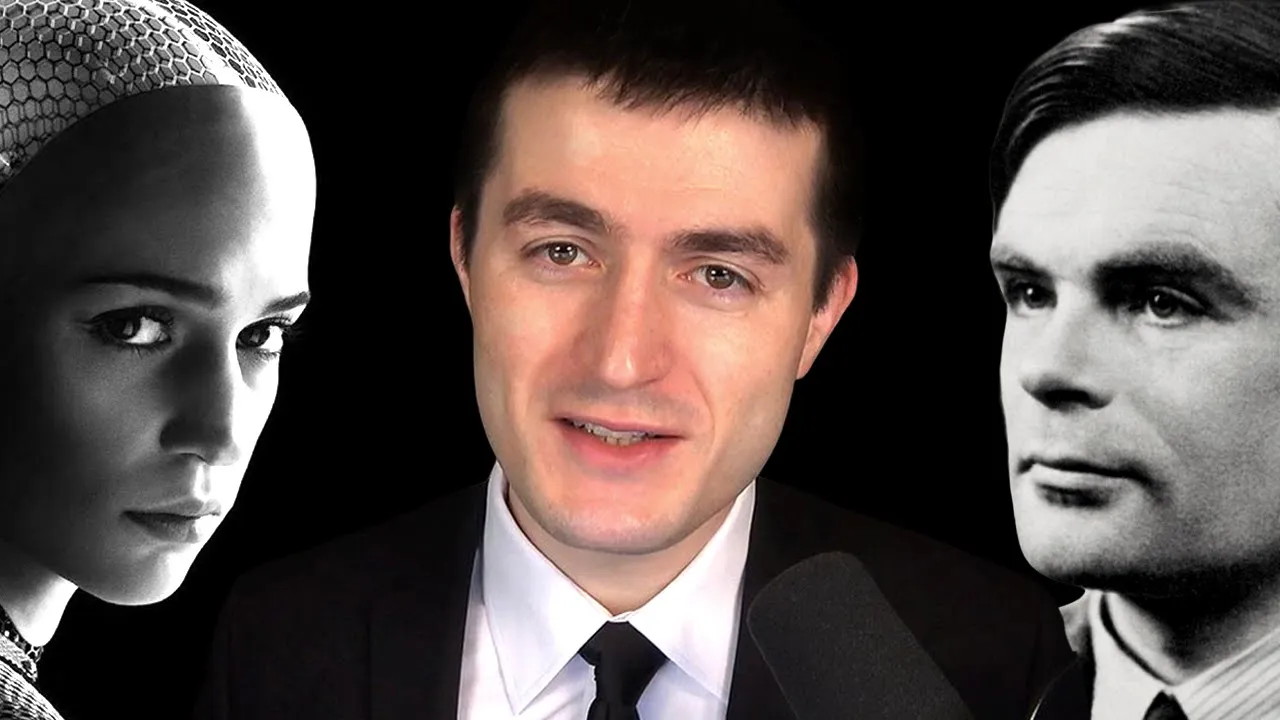
Chapters
0:0 Introduction
1:2 Paper opening lines
3:11 Paper overview
7:39 Loebner Prize
11:36 Eugene Goostman
13:43 Google's Meena
17:17 Objections to the Turing Test
17:29 Objection 1: Religious
18:7 Objection 2: "Heads in the Sand"
19:18 Objection 3: Godel Incompleteness Theorem
19:51 Objection 4: Consciousness
20:54 Objection 5: Machines will never do X
21:47 Objection 6: Ada Lovelace
23:22 Objection 7: Brain in analog
23:49 Objection 8: Determinism
24:55 Objection 9: Mind-reading
26:34 Chinese Room thought experiment
27:21 Coffee break
31:42 Turing Test extensions and alternatives
36:54 Winograd Schema Challenge
38:55 Alexa Prize
41:17 Hutter Prize
43:18 Francois Chollet's Abstraction and Reasoning Challenge (ARC)
49:32 Takeaways
56:51 Discord community
57:56 AI Paper Reading Club
00:00:02.280 |
that was asked by Alan Turing almost 70 years ago 00:00:05.240 |
in his paper, Computing Machinery and Intelligence. 00:00:10.780 |
This is the first paper in a paper reading club 00:00:16.280 |
that we started focused on artificial intelligence, 00:00:22.360 |
all of the scientific and engineering disciplines. 00:00:25.020 |
On the surface, this is a philosophical paper, 00:00:27.440 |
but really it's one of the most impactful, important 00:00:35.680 |
a benchmark that we call today the Turing test 00:00:46.560 |
So I'd like to talk about an overview of ideas 00:00:55.520 |
consider some alternatives to the test proposed 00:00:58.320 |
within the paper, and then finished with some takeaways. 00:01:15.120 |
on the slide I say it's one of the most impactful papers. 00:01:17.720 |
To me, it probably is the most impactful paper 00:01:29.160 |
from inside computer science and from outside 00:01:34.400 |
at a collective intelligence level of our species 00:01:38.440 |
inspired that this is possible, I think is immeasurable. 00:01:44.960 |
and computer science breakthroughs and papers 00:01:47.920 |
stretching all the way back to the 30s and 40s 00:01:50.600 |
with even the work by Alan Turing with the Turing machine, 00:01:55.040 |
some of the mathematical foundations of computer science 00:01:59.220 |
to today with deep learning, a sequence of papers 00:02:19.800 |
And it happens to have some of my favorite opening lines 00:02:33.280 |
of the meaning of the terms machine and think. 00:02:37.000 |
The definition might be framed so as to reflect 00:02:39.880 |
so far as possible the normal use of the words, 00:02:44.960 |
If the meaning of the words machine and think 00:02:52.520 |
that the meaning and the answer to the question, 00:02:57.240 |
in a statistical survey such as a Gallup poll. 00:03:08.200 |
and is expressed in relatively unambiguous terms. 00:03:14.760 |
the construction that we today call the Turing test, 00:03:19.640 |
There's a human interrogator on one side of the wall, 00:03:24.960 |
one a machine, one a human on the other side. 00:03:32.080 |
with the two entities on the other side of the wall 00:03:34.680 |
by written word, by passing those back and forth. 00:03:40.280 |
the human interrogator is tasked with making a decision, 00:03:47.560 |
I think this is a powerful leap of engineering, 00:03:50.480 |
which is take an ambiguous but a profound question 00:03:54.040 |
like can machines think and convert it into a concrete test 00:03:59.040 |
that can serve as a benchmark of intelligence. 00:04:04.720 |
to some of the other profound questions that we often ask. 00:04:22.000 |
I think these are really, really important questions, 00:04:26.920 |
when we're trying to create a non-human system 00:04:29.840 |
that tries to achieve human level capabilities. 00:04:33.120 |
So that's where Turing formulates this imitation game. 00:04:36.680 |
And his prediction was that by the year 2000, 00:04:58.160 |
is that people will no longer consider a phrase 00:05:04.280 |
So basically artificial intelligence at a human level 00:05:08.400 |
becomes so commonplace that we would just take it 00:05:20.700 |
will be a critical component of this success. 00:05:31.940 |
One is that the imitation game as Turing proposes 00:05:39.300 |
And the second is that machines can actually pass this test. 00:05:46.540 |
you're both proposing an engineering benchmark 00:05:59.580 |
but also exciting aspects of this whole area of work 00:06:08.300 |
I will not only describe some of the ideas in the paper 00:06:12.820 |
but also some of the open questions that remain, 00:06:16.480 |
both at the philosophical, the psychological, 00:06:21.800 |
is it even possible to create a test of intelligence 00:06:25.380 |
for artificial systems that will be convincing to us? 00:06:41.440 |
why do we still find that phrase contradictory? 00:06:53.020 |
was seen as the highest level of intelligence 00:07:00.540 |
to Garry Kasparov for being one of the greatest, 00:07:03.300 |
if not the greatest chess players of all time, 00:07:07.560 |
Why do we not assign at least an inkling of that 00:07:10.900 |
to IBM Deep Blue when it beat Garry Kasparov? 00:07:24.340 |
when they mastered the game of Go and the game of chess. 00:07:41.620 |
as a thought experiment, as a philosophical construct, 00:07:45.100 |
but it's also interesting as a real engineering test. 00:07:55.660 |
And the awards behind it, the award structure, 00:08:12.420 |
The rules of the competition have changed through the years, 00:08:26.780 |
Mitsuko and Rose from Steve Warswick and Bruce Wilcox 00:08:48.320 |
I believe there is a little bit of machine learning, 00:08:50.660 |
but as I understand, at the core, they're mostly scripted. 00:08:54.540 |
And on the next slide, we'll go through a transcript 00:08:56.620 |
of a conversation with Mitsuko to give you a sense 00:09:01.020 |
But I'd like to mention sort of a tragic note, really, 00:09:04.820 |
that as far as I understand, the prize is no longer funded. 00:09:08.660 |
But money aside, it shows that there's not a broad interest 00:09:18.300 |
It's really surprising to me that Google DeepMind, 00:09:21.600 |
Facebook AI, major groups, major individual researchers 00:09:25.940 |
in the field of AI have not taken on this challenge. 00:09:29.280 |
I hope to actually be a small part of changing that 00:09:32.100 |
a little bit, because I think there is a lot of value 00:09:38.020 |
And I'll return to that point later on in this presentation. 00:09:41.660 |
So you can pause and maybe read parts of this transcript. 00:09:45.580 |
I'll highlight on the right, at the top of the right column, 00:09:50.940 |
Mitsuko answers, "How can a person be a robot?" 00:09:54.660 |
Human, "Many people live lives akin to robots." 00:10:06.020 |
Human, "Do you think Southampton will beat Swansea?" 00:10:13.500 |
Mitsuko, "No reason, it's just what I think." 00:10:31.140 |
Mitsuko, "Yes, it is a city in the north of England. 00:10:48.380 |
There's a lingering and a repeatability of points. 00:10:56.260 |
and ability to follow tangents, all those kinds of things. 00:10:59.180 |
But it's still not clear to me as an open question 00:11:11.780 |
I believe that hasn't actually been really researched 00:11:17.540 |
As opposed to decision making at the very end 00:11:32.540 |
if it's possible to make explicit what aspects 00:11:43.700 |
at an exhibition event that marked the 60th anniversary 00:12:02.220 |
and had some humor and a constant sort of drive 00:12:08.620 |
to the places where it was comfortable doing. 00:12:13.000 |
of this event due to some sort of smoke and mirrors, 00:12:24.900 |
But setting that aside, I think the interesting lessons here 00:12:29.260 |
is that the parameters, the rules of the actual engineering 00:12:33.260 |
of the Turing test can determine whether it contains 00:12:44.260 |
of an agent to have a deep, meaningful conversation. 00:12:48.660 |
So in this case, you can argue that a few tricks were used 00:12:59.460 |
And 30% of judges were fooled without rigorous, 00:13:06.260 |
On the left is a transcript with Scott Aronson, 00:13:15.980 |
He posted some of the conversation that he had 00:13:20.420 |
on his blog that I think is really interesting. 00:13:22.980 |
So it shows that the judge, the interrogator, 00:13:32.500 |
As Scott did, he really didn't allow the kind 00:13:35.940 |
of misdirection that Eugene nonstop tried to do. 00:13:53.400 |
Google has published a paper and proposed a system 00:14:02.720 |
The representational goal in the 2.6 billion parameters 00:14:06.180 |
is to capture the conversational context well, 00:14:23.960 |
And it's a two-part metric of sensibleness and specificity. 00:14:39.460 |
So ability to match what we're saying to the context. 00:14:45.080 |
Now, the reason you need another side of that metric 00:14:52.020 |
you can fit the context by being boring, by being generic, 00:15:00.180 |
that fit a lot of different kinds of context. 00:15:02.460 |
So the other side of the metric is specificity. 00:15:05.160 |
Basically, the goal being there is don't be boring. 00:15:08.480 |
It's to say something very specific to this context. 00:15:17.180 |
to this particular set of lines of conversation 00:15:24.060 |
I think it's fair to say that the beauty of the music, 00:15:31.300 |
comes from that ability to play with the specifics, 00:15:39.400 |
Humans achieve 86% sensibleness and specificity. 00:15:44.540 |
Mina achieves 79% compared to Mitsuku, who achieves 56%. 00:16:05.500 |
Naturally, perhaps the paper is made in such a way, 00:16:10.220 |
the methodology and the results are made in such a way 00:16:12.580 |
that benefit the way the learning framework was constructed. 00:16:18.740 |
because I think there's still a lot of interesting ideas 00:16:23.340 |
at the actual percentages of 86% human performance 00:16:26.980 |
and 79% Mina performance, I think we're quite away 00:16:31.600 |
from being able to make conclusive statements 00:16:38.380 |
So those plots should be taken with a grain of salt, 00:16:44.780 |
I think quite obviously the future, long-term, 00:16:49.240 |
but hopefully short-term, is in learning end-to-end, 00:16:53.600 |
learning-based approaches to open domain conversation. 00:16:59.620 |
70 years ago in his paper that machine learning 00:17:02.220 |
will be essential to success, I believe the same. 00:17:05.600 |
It's a lot less interesting and revolutionary 00:17:20.320 |
Nine of them are highlighted by Turing himself in his paper. 00:17:25.940 |
Here I provide some informal, highly informal summaries. 00:17:33.460 |
which connects thinking to, quote-unquote, the soul. 00:17:37.640 |
And God, presumably, is the giver of the soul to humans. 00:17:42.640 |
Now, Turing's response to that is God is all-powerful. 00:18:04.720 |
cannot also be repeated for artificial creatures. 00:18:16.860 |
even in today's context, highlighted by folks 00:18:22.420 |
The head in the sand objection is that AGI is scary. 00:18:27.260 |
So human-level and superhuman-level intelligence 00:18:34.140 |
It seems like the world would be totally transformed 00:18:37.900 |
Then it could be transformed in a highly negative way. 00:18:49.000 |
That's kind of the objection of the Turing test. 00:18:53.680 |
to even think about a test for this intelligence 00:19:02.640 |
is that it doesn't matter how you feel about something 00:19:11.160 |
and not allow fear or emotion to model our thinking 00:19:19.680 |
The third objection is from Gato's incompleteness theorem, 00:19:28.080 |
that basically if a machine is a computation system, 00:19:33.440 |
in that it can never be a perfectly rational system. 00:19:42.160 |
Nowhere does it say that intelligence equals infallibility. 00:19:47.720 |
that fallibility is at the core of intelligence. 00:20:00.980 |
and whether something appears to be conscious. 00:20:03.200 |
So the focus of the Turing test is how something appears. 00:20:06.240 |
And so in some sense, humans, to us, as far as we know, 00:20:12.880 |
We can't prove that they're actually conscious, 00:20:17.080 |
And so since humans only appear to be conscious, 00:20:27.320 |
So the Turing test kind of skirts around the question 00:20:41.700 |
that consciousness is or isn't required for intelligence. 00:20:56.000 |
The fifth objection is the Negative Nancy objection 00:21:01.000 |
of machines will never be able to do X, whatever X is. 00:21:08.420 |
understand or generate humor, eat, enjoy food, 00:21:16.380 |
So there's a lot of things we can put in that X 00:21:20.080 |
And basically highlighting our human intuition 00:21:28.240 |
naturally the response here is that the objection 00:21:37.260 |
It is just a vapid opinion based on the world today, 00:21:42.260 |
refusing to believe that the world of tomorrow 00:21:47.020 |
The sixth objection, probably the most important, 00:21:59.120 |
with the basic idea that machines can only do 00:22:03.820 |
Now this is an objection that appears in many forms 00:22:09.540 |
And I think it's a really important objection 00:22:23.260 |
His response is, well, if machines can only do 00:22:35.400 |
it becomes clear that machines actually surprise us 00:22:41.140 |
will no longer be one of which we have a solid intuition 00:22:47.260 |
even if we built all the individual pieces of code 00:22:56.900 |
you have an intuition about how it should behave. 00:23:09.340 |
from input to output fades with the size of the code base, 00:23:14.220 |
even if you understand everything about the code, 00:23:17.620 |
and even if you set logical and syntactic bugs aside. 00:23:39.580 |
but if you have a big enough digital computer, 00:23:41.620 |
it can sufficiently approximate the analog system, 00:23:50.540 |
The eighth objection is the free will objection, right? 00:23:55.300 |
Is that when you have deterministic rules, laws, algorithms, 00:24:00.300 |
they're going to result in predictable behavior. 00:24:05.100 |
And this kind of exactly deterministic predictable behavior 00:24:23.700 |
I think is behind the Chinese room thought experiment 00:24:33.340 |
humans very well could be a complex collection of rules. 00:24:51.500 |
of deterministic, perfectly predictable sets of rules. 00:25:10.180 |
So the objection here is what if mind reading 00:25:38.520 |
that not only protects you from being able to see, 00:25:55.120 |
I think it's a nice illustration at the time, 00:25:57.240 |
and even still today, that there's a lot of mystery 00:26:07.860 |
I think you're assuming too much about your own knowledge 00:26:13.580 |
I think we know very little about how our mind works. 00:26:15.920 |
It is true, we have very little scientific evidence 00:26:29.300 |
You should nevertheless maintain an open mind. 00:26:38.500 |
and probably the most famous objection to the Turing test 00:26:47.260 |
commonly known as the Chinese Room Thought Experiment. 00:26:50.820 |
And it's kind of a combination of number four, number six, 00:26:53.660 |
and number eight objections on the previous slide, 00:26:56.140 |
which is the consciousness is required for intelligence, 00:27:10.060 |
that deterministic rules lead to predictable behavior. 00:27:13.820 |
And that doesn't seem to be like what the mind does. 00:27:50.800 |
Okay, the Chinese Room involves following instructions 00:28:12.740 |
So the idea is if the brain inside the system 00:28:26.980 |
it is not conscious, it does not have a mind, 00:28:32.100 |
So there's not, for my computer science engineering self, 00:29:01.860 |
And so the claim that I think is the most important, 00:29:06.740 |
is that syntax by itself is neither constitutive of 00:29:13.620 |
So just because you can replicate the syntax of the language 00:29:21.580 |
we hear of language models of today with transformers, 00:29:24.820 |
that OpenAI's GP2 really doesn't understand the language, 00:29:28.700 |
it's just mimicking the statistics of it so well 00:29:39.340 |
that indicates some kind of understanding, but it doesn't. 00:29:48.940 |
humans can understand things, humans are special, 00:30:08.140 |
Or put in other words, if understanding, intelligence, 00:30:18.020 |
then where is the point that computation hits the wall? 00:30:21.900 |
The most interesting open questions to me here 00:30:25.140 |
are on the point of faking things, or mimicking, 00:30:29.440 |
Does the mimicking of thinking equal thinking? 00:30:32.460 |
Does the mimicking of consciousness equal consciousness? 00:30:43.200 |
But I tend to believe from an engineering perspective, 00:30:51.980 |
we can only focus on building the appearance of thinking, 00:30:55.460 |
the appearance of consciousness, the appearance of love. 00:30:58.780 |
I think as we work towards creating that appearance, 00:31:01.980 |
we'll actually begin to understand the fundamentals 00:31:08.140 |
what it means to love, what it means to think. 00:31:14.600 |
that the appearance of consciousness is consciousness. 00:31:23.100 |
from our exceptionally limited understanding, 00:31:34.500 |
that's gonna lead us astray, in my personal view. 00:31:44.380 |
And now I'd like to talk about some alternatives 00:31:50.140 |
So there's a lot of kind of natural variations 00:31:54.980 |
First, the total Turing test proposed in 1989. 00:32:33.620 |
of the Turing test is actually harder to pass 00:32:42.580 |
about the original Turing test is that it's so simple. 00:33:07.580 |
There is also, in 2014, was proposed a Lovelace 2.0 test, 00:33:12.940 |
which emphasizes a more constrained definition 00:33:15.660 |
of what surprising is, 'cause it's very difficult 00:33:17.600 |
to pin down, to formalize the idea of surprise 00:33:21.820 |
and explain, right, in the original formulation 00:33:41.860 |
You basically have to create an impressive piece 00:33:49.640 |
but it takes us in the land that's much more, 00:33:53.180 |
not less subjective than the original Turing test. 00:34:00.900 |
and the very interesting question of surprise, 00:34:19.880 |
of human-level or superhuman-level intelligence, 00:34:36.880 |
but one certainly, one of the hardest ones is humor. 00:34:40.840 |
And finally, the truly total Turing test proposed in 1998 00:34:52.600 |
of an individual agent in an isolated context, 00:34:58.200 |
produced by a collection of intelligent agents 00:35:09.600 |
It's interesting to suggest that the way we conceive 00:35:35.840 |
not in the moment or particular five minute period 00:35:38.720 |
or 20 minute period, but over a period of months and years, 00:36:25.960 |
So intelligence very well could be the journey, 00:36:39.880 |
for benchmark not to measure instantaneous performance, 00:36:45.240 |
and the improvement of performance over time. 00:36:52.760 |
in the way that the original Turing test is formalized. 00:36:56.480 |
Another kind of test is the Winograd Schema Challenge, 00:37:00.340 |
which I think is really compelling in many ways. 00:37:09.280 |
Let's say the trophy doesn't fit into the brown suitcase 00:37:13.540 |
and the trophy doesn't fit into the brown suitcase 00:37:27.080 |
The trophy doesn't fit into the brown suitcase 00:37:31.120 |
And then the second question is, what is too large? 00:37:36.360 |
The trophy doesn't fit into the brown suitcase 00:37:42.620 |
is the ambiguity in the sentence can only be resolved 00:37:47.380 |
with common sense reasoning about ideas in this world. 00:37:51.200 |
And so the strength of this test is it's quite clear, 00:37:56.160 |
quite simple, and yet requires, at least in theory, 00:38:01.100 |
this deep thing that we think makes us human, 00:38:07.940 |
at the very basic level of common sense reasoning. 00:38:10.640 |
The other nice thing is it can be a benchmark, 00:38:15.840 |
like we're used to in the machine learning world, 00:38:18.080 |
that doesn't require subjective human judges. 00:38:23.420 |
The weakness here that holds for other similar challenges 00:38:36.560 |
And so that means you can't build a benchmark 00:38:45.860 |
Variations of the Winograd scheme are included 00:38:54.860 |
that people use in the machine learning context. 00:39:00.500 |
captures nicely the spirit of the Turing test. 00:39:03.900 |
I think it's actually quite an amazing challenge 00:39:06.100 |
and competition that uses voice conversation in the wild, 00:39:17.340 |
And I don't wanna wake up my own Alexa devices, 00:39:20.300 |
but basically say her name and say, let's chat. 00:39:23.460 |
And that brings up one of the bots involved in the challenge 00:39:28.420 |
And then the bar that's to be reached is for you 00:39:32.700 |
to have a 20 minute or longer conversation with the bot 00:39:36.860 |
and for two thirds or more of the interactions 00:39:41.280 |
So the basic metric of successful interaction 00:39:52.580 |
And I do think it's a really powerful metric. 00:39:55.660 |
As opposed to us judging the quality of conversation 00:40:05.940 |
When we have other things contending for our time, 00:40:09.980 |
when we make the choice to stay in that conversation, 00:40:30.700 |
no team has even come close to passing the Turing Test 00:40:37.820 |
There's several things that are really surprising 00:40:43.300 |
and two, that Amazon chose to limit it to students only. 00:40:48.300 |
I mean, almost making it an educational exercise 00:40:57.980 |
I mentioned it before, but I'll say it again here 00:41:00.140 |
that it's surprising to me that the biggest research lab 00:41:03.740 |
and industry in academia have not focused on this problem, 00:41:08.140 |
have not found the magic within the Turing Test problem 00:41:15.180 |
I believe, the spirit of the Turing Test quite well. 00:41:19.140 |
A very different kind of test is the Hutter Prize 00:41:26.980 |
on both the philosophical and mathematical angle. 00:41:38.140 |
Put another way, the ability to compress knowledge 00:41:56.580 |
by how well you're able to compress knowledge. 00:42:02.740 |
being able to compress well is closely related 00:42:07.060 |
thus reducing the slippery concept of intelligence 00:42:12.420 |
So the task is to take one gigabyte of Wikipedia data 00:42:20.860 |
The current best is a 8.58 compression factor. 00:42:30.220 |
And the award for each 1% improvement, you win 5,000 euros. 00:42:43.020 |
of an intelligence challenge, but it's not a test. 00:43:01.340 |
beyond which we feel it would be human level intelligence. 00:43:07.820 |
Lobna Prize, Alexa Prize, are also arbitrary, 00:43:11.780 |
but it feels like we're able to intuit a good bar 00:43:14.180 |
in that context better than being able to intuit 00:43:17.700 |
the kind of bar we need to set for the compression challenge. 00:43:24.740 |
put forth by Francois Chollet just a few months ago. 00:43:29.460 |
It's actually ongoing as a competition on Kegel, 00:43:43.780 |
and I'll talk to Francois, I'm sure, on the podcast 00:43:48.140 |
I think there's a lot of brilliant ideas here 00:43:50.820 |
that I still have to kind of digest a little bit, 00:43:59.420 |
So first of all, the name is abstraction reasoning corpus 00:44:15.700 |
And the spirit of the set of tests that Francois proposes 00:44:23.740 |
that we use to measure the intelligence of human beings. 00:44:29.100 |
Now, the Turing test is kind of at a higher level 00:44:49.920 |
such that we can then make explicit the priors, 00:44:55.620 |
the concepts that we bring to the table of those tests. 00:45:04.420 |
to the measure of the system's ability to reason. 00:45:07.920 |
Now, the concepts that are brought to this grid world, 00:45:19.260 |
Here, prior concept is not referring to a previous concept. 00:45:27.420 |
So this first row of illustrations of the two grid worlds 00:45:31.220 |
illustrates the idea of object persistence with noise. 00:45:34.420 |
So we're able to understand that large objects, 00:45:49.480 |
And if that noise changes, the object is still unchanged. 00:45:53.580 |
So that idea of object persistence in the world 00:46:06.820 |
is objects are defined by spatial contiguity. 00:46:28.660 |
So this kind of spatial contiguity of colored cells 00:46:37.860 |
And on the right at the bottom is the color-based contiguity, 00:46:48.300 |
that means it likely belongs to a different object. 00:47:06.380 |
There's a lot of interesting insights in there. 00:47:09.280 |
Just to give you some examples of what the actual task 00:47:15.940 |
it's similar to the kind of task you would see in an IQ test. 00:47:21.680 |
and the task is for the fourth pairing of images 00:47:25.640 |
to generate the grid world that fits the other three, 00:47:30.640 |
that fits the generating pattern of the other three. 00:47:52.200 |
So here, what you're tasked with understanding 00:47:57.540 |
is that the input has a perfect global symmetry to it. 00:48:02.540 |
And also that there's parts of the image that are missing 00:48:19.080 |
which I think underlies a lot of our understanding 00:48:25.920 |
has to have a good representation of symmetry 00:48:33.140 |
This is fascinating and beautiful, beautiful images. 00:48:36.760 |
Okay, another example, figure 10 from the paper, 00:48:39.840 |
a task where the implicit goal is to count unique objects 00:48:43.240 |
and select the objects that appears the most times. 00:48:52.100 |
a task where the implicit goal is to count unique objects 00:48:55.080 |
and select the objects that appear the most times. 00:49:01.320 |
You see in the first one, there's three blue objects. 00:49:04.520 |
In the second one, there's four yellow objects. 00:49:10.560 |
And then the output is the grid cells capturing that object 00:49:20.380 |
to complete the output of the fourth pairing. 00:49:29.120 |
I think there's a lot of really interesting technical 00:49:31.640 |
and philosophical ideas here that are worth exploring. 00:49:35.680 |
So let's quickly talk through a few takeaways. 00:49:43.380 |
and can it serve as an answer to the big ambiguous 00:49:48.100 |
but profound philosophical question of can machines think? 00:49:54.020 |
on the underlying challenges of the Turing test. 00:50:00.660 |
So if we compare human behavior and intelligent behavior, 00:50:05.360 |
it's clear that the Turing test hopes to capture 00:50:21.820 |
the unintelligent, the irrational parts of human behavior. 00:50:25.500 |
So it's an open question whether natural conversation 00:50:36.380 |
it's focusing only on kind of rational systematic thinking. 00:50:42.620 |
then you have to capture the full range of emotion, 00:50:45.720 |
the mess, the irrationality, the laziness, the boredom, 00:50:52.020 |
and all the things that then project themselves 00:50:55.220 |
into the way we carry on through conversation. 00:50:59.700 |
the Turing test really focuses on the external appearances, 00:51:06.100 |
So like I said, from an engineering perspective, 00:51:12.460 |
for internal processes for some of these concepts 00:51:23.300 |
in terms of quantifying and having a measure of something, 00:51:27.180 |
we have to look at the external performance of the system 00:51:31.620 |
as opposed to some properties of the internal processes. 00:51:37.980 |
as Scott Aronson's conversation with Eugene Guzman indicates 00:51:58.100 |
the ability of the interrogator to identify the humanness 00:52:09.540 |
and the ability to make the actual identification 00:52:32.660 |
whether in some construction of the Turing test, 00:52:44.220 |
to convincing us humans that something is intelligent. 00:52:54.980 |
in our subjective judgment of its intelligence. 00:52:59.940 |
And finally, another limitation of the Turing test 00:53:28.820 |
over which we analyze the intelligence of the system, 00:53:49.540 |
I think in part may require getting to know the person. 00:53:54.700 |
And there's something to rethink in the Turing test 00:54:02.140 |
So you can think of it as kind of the ex machina Turing test 00:54:07.060 |
where they spend a series of conversations together, 00:54:10.980 |
several days together, all those kinds of things. 00:54:19.700 |
the significant limitation of the current construction 00:54:22.540 |
of the Turing test which is a limited window of time, 00:54:25.020 |
one time at the end interrogate or judgment of it 00:54:31.700 |
Now my view overall on the Turing test is that yes, 00:54:35.700 |
something like the Turing test as originally constructed 00:54:44.100 |
is close to the ultimate test of intelligence. 00:54:51.900 |
and other world-class researchers in the area, 00:54:55.580 |
Stuart Russell and so on, that I think the Turing test 00:55:01.700 |
It doesn't pull us away from actually making progress 00:55:12.140 |
in natural language conversation will help us understand 00:55:17.040 |
And more than that, I think there should be active research 00:55:22.180 |
I think the Lubna Prize type of formulations, 00:55:24.220 |
the Alexa Prize formulations should be more popular 00:55:27.260 |
than they are and I think researchers should take them 00:55:31.660 |
Now that doesn't mean that the work of the ARC benchmark 00:55:40.420 |
is not also going to be fruitful, potentially very fruitful. 00:55:48.760 |
of human-level intelligence will occur in something 00:55:55.660 |
with natural language open domain conversation 00:56:04.460 |
Zooming out a little bit, I think in general, 00:56:08.100 |
I think AI researchers don't like and try to avoid 00:56:16.380 |
by the human-robot interaction field and set of problems. 00:56:20.260 |
I think more than just embracing the Turing test, 00:56:23.980 |
I think we should embrace the messiness of the human being 00:56:27.780 |
in all the different domains of computer vision, 00:56:31.700 |
of natural language, of robotics, of autonomous vehicles. 00:56:36.700 |
I've been a long-time advocate that semi-autonomous vehicles 00:56:46.500 |
and for that we have to embrace perceiving everything 00:56:51.540 |
perceiving everything about the humans outside the car. 00:56:54.240 |
As I mentioned, this presentation of the paper 00:57:07.100 |
on a Discord server called LexPlus AI Podcast 00:57:12.220 |
We have an amazing community of brilliant people there 00:57:19.200 |
This particular illustration that I just love 00:57:24.580 |
from United Kingdom who is part of this Discord community 00:57:30.940 |
And in general, aside from the amazing conversations, 00:57:34.640 |
I encourage and hope to see other members of the community 00:57:38.260 |
contribute art, code, visualizations, slides, 00:57:45.820 |
I'm really excited by the kind of conversations I've seen. 00:57:48.980 |
If you're watching this video and wanna join in, 00:57:51.420 |
click on the Discord link in the description on the slide. 00:57:54.440 |
Join the conversation, new paper every week, it's fun. 00:58:07.380 |
I think the goal is to take a seminal paper in the field 00:58:13.960 |
sort of paragraph to paragraph, section to section analysis 00:58:17.700 |
what the paper is saying, but actually use the paper 00:58:20.400 |
to discuss the history, the big picture development 00:58:23.860 |
of the field within the context of that paper. 00:58:30.220 |
or it could be very specific papers in the field. 00:58:33.420 |
Again, physics, mathematics, computer science, 00:58:38.340 |
So the hope is to prioritize beautiful, powerful, 00:58:43.340 |
impactful insights as opposed to full coverage 00:58:57.360 |
There's a lot of brilliant people, they're civil, 00:59:00.140 |
so you can have 300, 400 people on voice chat, 00:59:05.540 |
And yet people aren't interrupting each other, 00:59:08.220 |
it's not chaos, it's quite an amazing community. 00:59:16.020 |
the goal is for it to be accessible to everyone. 00:59:21.820 |
people outside of all of these fields in general, 00:59:34.340 |
but still try to discover insights that are new, 00:59:50.420 |
suggest papers, suggest content, visualizations, code, 00:59:57.540 |
Thanks for watching this excessively long presentation.