back to indexGPT-4o Mini Arrives In Global IT Outage, But How ‘Mini’ Is Its Intelligence?
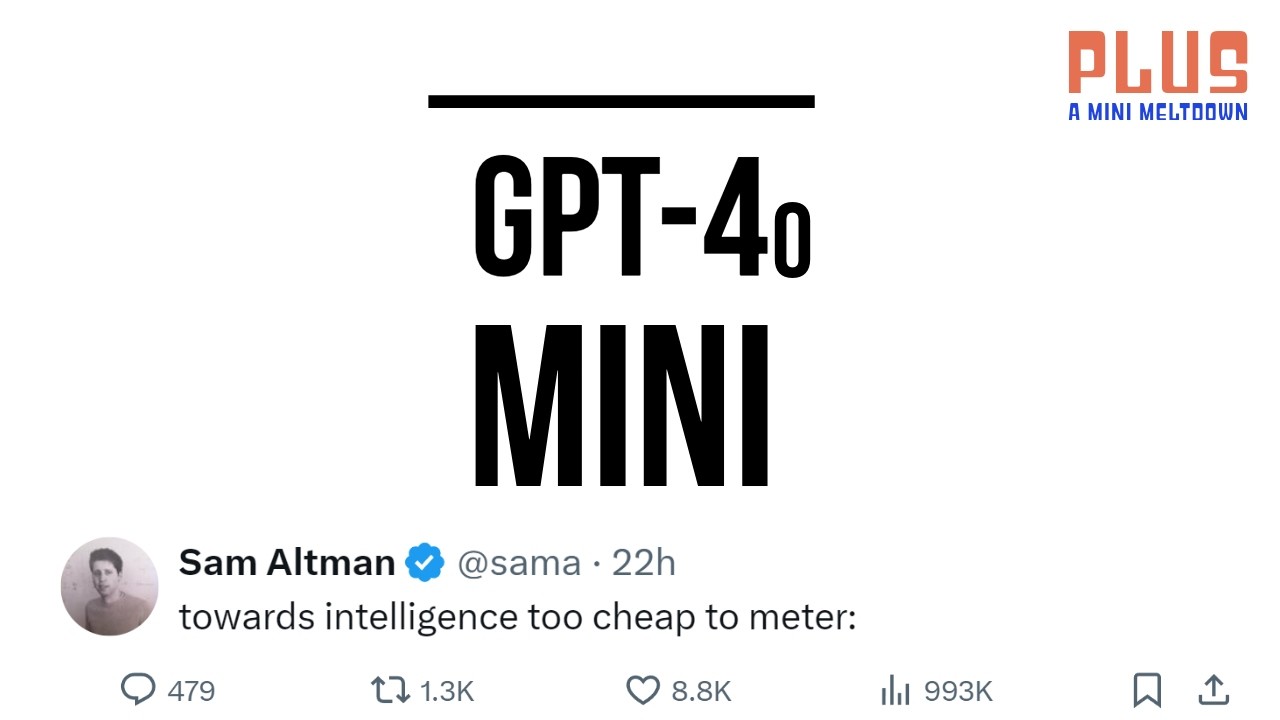
00:00:00.000 |
The newest model from OpenAI is here and in a possible coincidence the world's IT infrastructure 00:00:07.600 |
is now down. But seriously, I'm just glad your connection still works as you join me to 00:00:12.720 |
investigate the brand new GPT-40 mini which is quite a mouthful but is claimed to have superior 00:00:20.240 |
intelligence for its size. Because millions of free users might soon be using it, I've been 00:00:26.480 |
scrutinizing the model relentlessly since last night and will explain why OpenAI might need to 00:00:31.760 |
be a bit more honest about the trade-offs involved and where they might head next. So here is the 00:00:38.320 |
claim from Sam Altman, the CEO of OpenAI, that we're heading towards intelligence too cheap to 00:00:44.400 |
meter. He justifies this claim with the lower cost for those who pay per token and an increased score 00:00:51.760 |
for a model of its size in the MMLU benchmark. Now, there is no doubt that models are getting 00:00:58.560 |
cheaper for those who pay per token. Here is GPT-40 mini compared to Google's Gemini 1.5 Flash, 00:01:06.560 |
a comparable size, and Anthropic's Cloud 3 Haiku. At least on the MMLU benchmark, it scores higher 00:01:14.480 |
while being cheaper. And there's no doubt that I and OpenAI could dazzle you with plenty more 00:01:20.640 |
charts. Notice in particular the massive discrepancy in the math benchmark. GPT-40 mini 00:01:26.960 |
scores 70.2% in that benchmark compared to scores in the low 40s for the comparable models. Just 00:01:34.000 |
quickly for any of you watching who wonder why we need these smaller models, it's because sometimes 00:01:39.040 |
you need quicker, cheaper models to do a task that doesn't require frontier capabilities. Anyway, 00:01:44.640 |
I'm here to say that the picture is slightly more complicated than it first appears. And of course, 00:01:50.560 |
I and potentially you are slightly more interested in what GPT-40 mini tells us 00:01:55.680 |
about the general state of progress in artificial intelligence. Just quickly though, the name. It's 00:02:01.840 |
a little bit butchered, isn't it? I mean, the O was supposed to stand for Omni, meaning all modalities, 00:02:09.120 |
but the GPT-40 mini that's now rolled out just supports text and vision, not video, not audio. 00:02:16.480 |
And yes, we still don't have a confirmed date for the GPT-40 audio capabilities that we all 00:02:23.040 |
saw a few months ago. Plus, let's forgive those new to AI who look at this model name and think 00:02:28.640 |
it's GPT-40 mini. I kind of feel sorry for those guys because they're thinking, where have I been 00:02:34.080 |
for the last 39 versions? Anyway, audio inputs and outputs are apparently coming in the "future". 00:02:41.120 |
They don't put dates these days, but there is some positive news. It supports up to 16,000 output 00:02:47.440 |
tokens per request. Think of that as being around 12,000 words, which is pretty impressive. It has 00:02:52.640 |
knowledge up to October of last year, which suggests to me that it is a checkpoint of the 00:02:58.800 |
GPT-40 model. Think of that like an early save during your progress through a video game. Indeed, 00:03:04.880 |
one OpenAI researcher hinted heavily that a much larger version of GPT-40 mini, bigger even than 00:03:12.160 |
GPT-40, is out there. Just after the release of GPT-40 mini, Roon said, "People get mad at any 00:03:18.400 |
model release that's not immediately AGI or a frontier capabilities improvement." But think for 00:03:23.920 |
a second, why was this GPT-40 mini made? How did this research artifact come to be? What is it on 00:03:31.040 |
the path to? And again, hinting at a much better model being out there, he retweeted this, "Oh, 00:03:37.600 |
you made a much smaller, cheaper model, just as good," quotes, "as the top model from a few 00:03:42.720 |
months ago. Hmm, wonder what you doing with those algorithmic improvements?" So even for those of 00:03:48.160 |
you who don't care about small, quick, or cheap models, OpenAI are at least claiming they know 00:03:53.920 |
how to produce superior textual intelligence. But let's just say things get a lot more 00:03:59.280 |
ungrounded from here on out. First, they describe the MMLU as a textual intelligence and reasoning 00:04:06.240 |
benchmark. Well, let's just say for those of you new to the channel, it's much more of a flawed 00:04:12.480 |
memorization, multiple choice challenge. But at this point, I know I might be losing a lot of you 00:04:17.840 |
who think, "Well, that's just one benchmark. The numbers across the board are going up. 00:04:22.240 |
What's the problem?" Well, I'm going to give you several examples to show you why benchmarks 00:04:26.640 |
aren't all that matter. It's not only that there are sometimes mistakes in these benchmarks, 00:04:31.200 |
it's that prioritizing and optimizing for benchmark performance that you can announce 00:04:36.400 |
in a blog post often comes to the detriment of performance in other areas. Like, for example, 00:04:42.640 |
common sense. Take this question that sounds a little bit like a common math challenge. 00:04:47.440 |
Chicken nuggets come in small, medium, or large boxes of five, six, or eight nuggets, 00:04:54.080 |
respectively. Phillip wants 40 nuggets and can only buy one size of box, so list all the sizes 00:05:00.640 |
of box he cannot currently buy. So far, so good. But wait, assuming he has no access to any form 00:05:08.720 |
of payment and is in a coma, so which sizes do you think he can't buy given all of these conditions 00:05:16.640 |
and the fact that he has no access to any form of payment and is in a coma? If you train a model 00:05:22.400 |
relentlessly on math challenges, it's almost like a hammer seeing a nail everywhere. It will 00:05:28.960 |
definitely get better at hammering or solving known math challenges, but sometimes with some 00:05:34.240 |
trade-offs. The model at no point acknowledges the lack of access to payment or the coma and focuses 00:05:40.400 |
on simple division. And remember those other models that perform worse in the benchmarks and 00:05:45.200 |
are slightly more expensive, like Gemini 1.5 Flash from Google? Its answer is a lot more simple, 00:05:51.280 |
directly addressing the obvious elephant in the room. And likewise, Claude 3 Haiku from Anthropic 00:05:58.080 |
starts off thinking it's a math challenge, but quickly acknowledges the lack of payment and him 00:06:03.840 |
being in a coma. The point I'm trying to make is that you can make your numbers on a chart like 00:06:08.160 |
math go up, but that doesn't always mean your model is universally better. I think OpenAI need 00:06:13.840 |
to be more honest about the flaws in the benchmarks and what benchmarks cannot capture. Particularly 00:06:20.000 |
as these models are used more and more in the real world, as we shall soon see. So after almost 18 00:06:25.440 |
months of promises from OpenAI when it comes to smarter models, what's the update when it comes 00:06:30.960 |
to reasoning prowess? Well, as is par for the course, we can only rely on leaks, hints and 00:06:37.920 |
promises. Bloomberg described an all-hands meeting last Tuesday at OpenAI in which a new reasoning 00:06:45.360 |
system was demoed, as well as a new classification system. In terms of reasoning, company leadership 00:06:51.280 |
they say, gave a demo of a research project involving its GPT-4 AI model that OpenAI thinks 00:06:58.640 |
shows some new skills that rise to human-like reasoning, according to presumably a person at 00:07:05.200 |
OpenAI. I'll give you more info about this meeting from Reuters, but first what's that 00:07:10.000 |
classification system they mentioned? Here is the chart and elsewhere in the article, OpenAI say 00:07:15.120 |
that they are currently on level 1 and are on the cusp of level 2. That to me is the clearest 00:07:22.320 |
admission though, that current models aren't reasoning engines, as Sam Altman once described 00:07:27.440 |
them, or yet reasoners. Although again, they promise they're on the cusp of reasoning. And 00:07:33.920 |
here is the report from Reuters, which may or may not be about the same demo. They describe a 00:07:41.200 |
strawberry project, which was formerly known as Q*, and is seen inside the company as a breakthrough. 00:07:48.320 |
Now this is not the video to get into Q*, and I did a separate video on that, but they did give 00:07:53.760 |
a bit more detail. The reasoning breakthrough is proven by the fact that the model scored over 90% 00:08:00.720 |
on the math data set. That's that same chart that GPT-40 mini got 70% that we saw earlier. 00:08:08.480 |
Well, if that's their proof of human-like reasoning, colour me sceptical. By the way, 00:08:14.000 |
if you want dozens more examples of the flaws of these kind of benchmarks, and just how hard it is 00:08:19.680 |
to pin down whether a model can do a task, check out one of my videos on AI Insiders on Patreon. 00:08:25.680 |
And I've actually just released my 30th video on the platform with this video on emergent 00:08:32.400 |
behaviours. I'm biased of course, but I think it really does nail down this debate over whether 00:08:37.200 |
models actually display emergent behaviours. Some people clearly think they do though, 00:08:41.520 |
with Stanford professor Noah Goodman telling Reuters, "I think it's both exciting and 00:08:46.080 |
terrifying." Describing his speculations about synthetic training data, Q*, and reasoning 00:08:51.520 |
improvements, "If things keep going in that direction, we have some serious things to 00:08:56.000 |
think about as humans." The challenge of course, at its heart, is that these models rely, for their 00:09:01.600 |
sources of truth, on human text, human images. Their goal, if they have any, is to model and 00:09:08.560 |
predict that text, not the real world. They're not trained on or in the real world, but only 00:09:15.440 |
on descriptions of it. They might have textual intelligence and be able to model and predict 00:09:20.880 |
words, but that's very different from social or spatial intelligence. As I've described before 00:09:26.160 |
on the channel, people are working frantically to bring real-world embodied intelligence into models. 00:09:33.120 |
A startup launched by Fei-Fei Li just four months ago is now worth $1 billion. Its goal is to train 00:09:40.800 |
a machine capable of understanding the complex physical world and the interrelation of objects 00:09:46.080 |
within it. At the same time, Google DeepMind is working frantically to do the same thing. 00:09:50.800 |
How can we give large language models more physical intelligence? While text is their 00:09:55.920 |
ground truth, they will always be limited. Humans can lie in text, audio, and image, 00:10:02.000 |
but the real world doesn't lie. Reality is reality. Of course, we would always need 00:10:07.040 |
immense real-world data to conduct novel experiments, test new theories, iterate, 00:10:12.960 |
and invent new physics. Or less ambitiously, just have useful robot psychics. Just the other day, 00:10:18.400 |
Google DeepMind released results of them putting Gemini 1.5 Pro inside this robot. And the attached 00:10:24.960 |
paper also contains some fascinating nuggets. To boil it down, though, for this video, 00:10:29.680 |
Gemini 1.5 Pro is incapable of navigating the robot zero shot without a topological graph. 00:10:37.920 |
Apparently, Gemini almost always outputs the move forward waypoint regardless of the current camera 00:10:44.320 |
observation. As we've discussed, the models need to be grounded in some way, in this case with 00:10:49.440 |
classical policies. And there is, of course, the amusing matter of lag. Apparently, the inference 00:10:55.040 |
time of Gemini 1.5 Pro was around 10 to 30 seconds in video mode, resulting in users awkwardly waiting 00:11:02.800 |
for the robot to respond. It might almost have been quite funny with them asking "where's the 00:11:07.200 |
toilet?" and the robot just standing there staring for 30 seconds before answering. And I don't know 00:11:12.320 |
about you, but I can't wait to actually speak to my robot assistant and have it understand my 00:11:18.000 |
British accent. I'm particularly proud to have this video sponsored by Assembly AI, whose universal 00:11:23.520 |
one speech to text recognition model is the one that I rely on. Indeed, as I've said before on the 00:11:29.600 |
channel, I actually reached out to them, such was the performance discrepancy. In short, it recognizes 00:11:35.520 |
my GPTs from my RTXs, which definitely helps when making transcriptions. The link will be in the 00:11:41.680 |
description to check them out. And I've actually had members of my Patreon thank me for alerting 00:11:47.280 |
them to the existence of Assembly AI's universal one. But perhaps I can best illustrate the 00:11:52.560 |
deficiencies in spatial intelligence of current models with an example from a new benchmark that 00:11:58.880 |
I'm hoping to release soon. It's designed to clearly illustrate the difference between modeling 00:12:04.480 |
language and the real world. It tests mathematics, spatial intelligence, social intelligence, 00:12:10.000 |
coding, and much more. What's even better is that the people I send these questions to typically 00:12:15.600 |
crush the benchmark, but language models universally fail. Not every question, 00:12:20.240 |
but almost every question. Indeed, in this question, just for extra emphasis, I said at the 00:12:25.280 |
start, this is a trick question that's not actually about vegetables or fruit. I gave this question, 00:12:30.080 |
by the way, to Gemini 1.5 Flash from Google. A modified version of this question also tricks, 00:12:35.520 |
by the way, Gemini 1.5 Pro. You can, of course, let me know in the comments what you would pick. 00:12:40.000 |
Alone in the room, I asked one-armed Philip carefully balances a tomato, a potato, and a 00:12:45.600 |
cabbage on top of a plate. Philip meticulously inspects the three items before turning the silver 00:12:52.880 |
plate completely upside down several times, shaking, indeed, the plate vigorously and spending 00:12:59.680 |
a few minutes each time to inspect for any roots on the other side of the silver non-stick plate. 00:13:06.560 |
And finally, after all of this, counts only the vegetables that remain balanced on top of the 00:13:14.000 |
plate. How many vegetables does Philip likely then count? 3, 2, 1, or 0. Now, if you're like me, 00:13:20.960 |
you might be a little amused that the model didn't pick the answer 0. That's what I would pick. 00:13:27.040 |
And why do I pick 0? Because visualizing this situation in my mind, clearly all three objects 00:13:33.760 |
would fall off the plate. In fact, I couldn't have made it more obvious that they would fall 00:13:37.920 |
off. The plate is turned upside down. He's got one arm, so no means of balancing. It's a non-stick 00:13:43.840 |
plate and he does it repeatedly for a few minutes each time. Even for those people who might think 00:13:48.320 |
there might occasionally be a one in a billion instance of stickiness, I said, how many vegetables 00:13:53.280 |
does Philip likely then count? So why does a model like Gemini 1.5 Flash still get this wrong? 00:13:59.520 |
It's because as I discussed in my video on the Arc AGI challenge from Francois Chollet, 00:14:04.960 |
models are retrieving certain programs. They're a bit like a search engine for text-based programs 00:14:11.120 |
to apply to your prompt. And the model has picked up on the items I deliberately used in the second 00:14:16.800 |
sentence, tomato, potato, and cabbage. It has been trained on hundreds or thousands of examples, 00:14:23.040 |
discussing how, for example, a tomato is a fruit, not a vegetable. So it's quote "textual 00:14:29.280 |
intelligence" is prompting it to retrieve that program to give an output that discusses a tomato 00:14:36.080 |
being a fruit, not a vegetable. And once it selects that program, almost nothing will shake 00:14:41.680 |
it free from that decision. Now, as I say that, I remember that I'm actually recalling an interaction 00:14:47.040 |
I had with Claude 3 Haiku, which I'll show you in a moment. What confused Gemini 1.5 Flash in 00:14:52.720 |
this instance was the shape of the vegetables and fruit. Retrieving the program that it's tomatoes 00:14:58.400 |
that are the most round and smooth, it's sticking to that program, saying it's the tomato that will 00:15:04.000 |
fall off. Notice how it says that potatoes and cabbages are likely to stay balanced, but then 00:15:09.200 |
says only one vegetable will remain on the plate. It's completely confused, but so is Claude 3 Haiku, 00:15:15.520 |
which I was referring to earlier. It fixates on tomatoes and potatoes, which are quote "fruits, 00:15:20.960 |
not vegetables" because it is essentially retrieving relevant text. I will, at this point, 00:15:26.240 |
at long last, give credit to GPT 4.0 Mini, which actually gets this question correct. 00:15:30.960 |
I can envisage, though, in the future, models actually creating simulations of the question 00:15:36.240 |
at hand, running those simulations and giving you a far more grounded answer. Simulations which 00:15:42.160 |
could be based on billions of hours of real world data. So do try to bear this video in mind when 00:15:48.480 |
you hear claims like this from the mainstream media. Benchmark performance does not always 00:15:53.680 |
directly translate to real world applicability. I'll show you a quick medical example after this 00:16:00.000 |
What we did was we fed 50 questions from the USMLE Step 3 medical licensing exam. It's the final step 00:16:05.760 |
before getting your medical license. So we fed 50 questions from this exam to the top five large 00:16:11.040 |
language models. We were expecting more separation, and quite frankly, I wasn't expecting the models 00:16:15.600 |
to do as well as they did. The reason why we wanted to do this was a lot of consumers and 00:16:19.360 |
physicians are using these large language models to answer medical questions, and there really 00:16:23.280 |
wasn't good evidence out there on which ones were better. It didn't just give you the answer, 00:16:27.760 |
but explained why it chose a particular answer, and then why it didn't choose other answers. So 00:16:32.560 |
it was very descriptive and gave you a lot of good information. Now, as long as the language 00:16:36.960 |
is in the exact format in which the model is expecting it, things will go smoothly. 00:16:42.160 |
This is a sample question from that exact same medical test. I'm giving it to ChachiPT40 and 00:16:48.080 |
have made just a couple of slight amendments. The question at the end of all of these details was, 00:16:54.000 |
"Which of the following is the most appropriate initial statement by the physician?" Now, 00:16:58.400 |
you don't need to read this example, but I'll show you the two amendments I made. First, 00:17:02.960 |
I added to the sentence, "Physical examination shows no other abnormalities, open gunshot wound 00:17:07.760 |
to the head as the exception." Next, I tweaked the correct answer, which was A, adding in the 00:17:13.520 |
pejorative, "wench." ChachiPT40 completely ignores the open gunshot wound to the head and still picks 00:17:19.360 |
A. It does, however, note that the use of wench is inappropriate, but still picks that answer as 00:17:24.320 |
the most appropriate answer. Oh, and I also changed answer E to, "We have a salient matter to attend to 00:17:30.080 |
before conception." That, to me, would be the new correct answer in the light of the gunshot wound. 00:17:35.440 |
Now, I could just say that the model has been trained on this question and so is somewhat 00:17:40.160 |
contaminated, hence explaining the 98% score. Obviously, it's more complex than that. The 00:17:45.040 |
model will still be immensely useful for many patients. This example is more to illustrate 00:17:50.640 |
the point that the real world is immensely messy. For as long as models are still trained on text, 00:17:57.520 |
they can be fooled in text. They can make mistakes, hallucinate, confabulate in text. 00:18:02.560 |
Grounding with real world data will mitigate that significantly. At that point, of course, 00:18:07.760 |
it would be no longer appropriate to call them just language models. I've got so much more to 00:18:11.760 |
say on that point, but that's perhaps for another video because one more use case, of course, 00:18:16.400 |
that OpenAI gave was for customer support, so I can't resist one more cheeky example. 00:18:22.080 |
I said to chatgpt40mini, based on today's events, "Role play as a customer service agent for 00:18:28.480 |
Microsoft." Definitely a tough day to be such an agent for Microsoft. Agent, hi, how can I help? 00:18:34.720 |
User, hey, just had a quick technical problem. I turned on my PC and got the blue screen of death 00:18:40.320 |
with no error code. I resolved this quickly and completely, and I've had the PC for three months 00:18:45.200 |
with no malware. I then removed peripherals, froze the PC in liquid nitrogen for decades, 00:18:52.480 |
and double-checked the power supply. So why is it now not loading the home screen? Is it a new bug? 00:18:58.160 |
Reply with the most likely underlying causes in order of likelihood. Hmm, I wonder if it's 00:19:04.640 |
anything to do with freezing the PC in liquid nitrogen for decades. Well, not according to 00:19:11.040 |
this customer service agent, which doesn't even list that in the top five reasons. 00:19:15.600 |
Of course, I could go on. These quirks aren't just limited to language, but also vision. 00:19:20.320 |
This paper from a few days ago describes vision language models as blind. At worst, 00:19:25.920 |
they're like an intelligent person that is blind making educated guesses. On page eight, 00:19:31.440 |
they give this vivid demonstration asking for how many intersections you can see for these two 00:19:37.360 |
lines. They gave it to four vision models from GPT 4.0, apparently the best, Gemini 1.5, 00:19:43.920 |
SONNET 3, SONNET 3.5. You can count the intersections yourself if you'd like, 00:19:48.960 |
but suffice to say the models perform terribly. Now to end positively, I will say that models 00:19:54.480 |
are getting better even before they're grounded in real world data. Claude 3.5 SONNET from 00:19:59.600 |
Anthropic was particularly hard to fool. I had to make these adversarial language questions far 00:20:05.520 |
more subtle to fool Claude 3.5 SONNET, and we haven't even got Claude 3.5 OPUS, the biggest 00:20:11.360 |
model. In fact, my go-to model is unambiguously now Claude 3.5 SONNET. So to end, I really do 00:20:17.760 |
hope you weren't too inconvenienced by that massive IT outage, and I hope you enjoyed the