back to indexChatGPT Fails Basic Logic but Now Has Vision, Wins at Chess and Prompts a Masterpiece
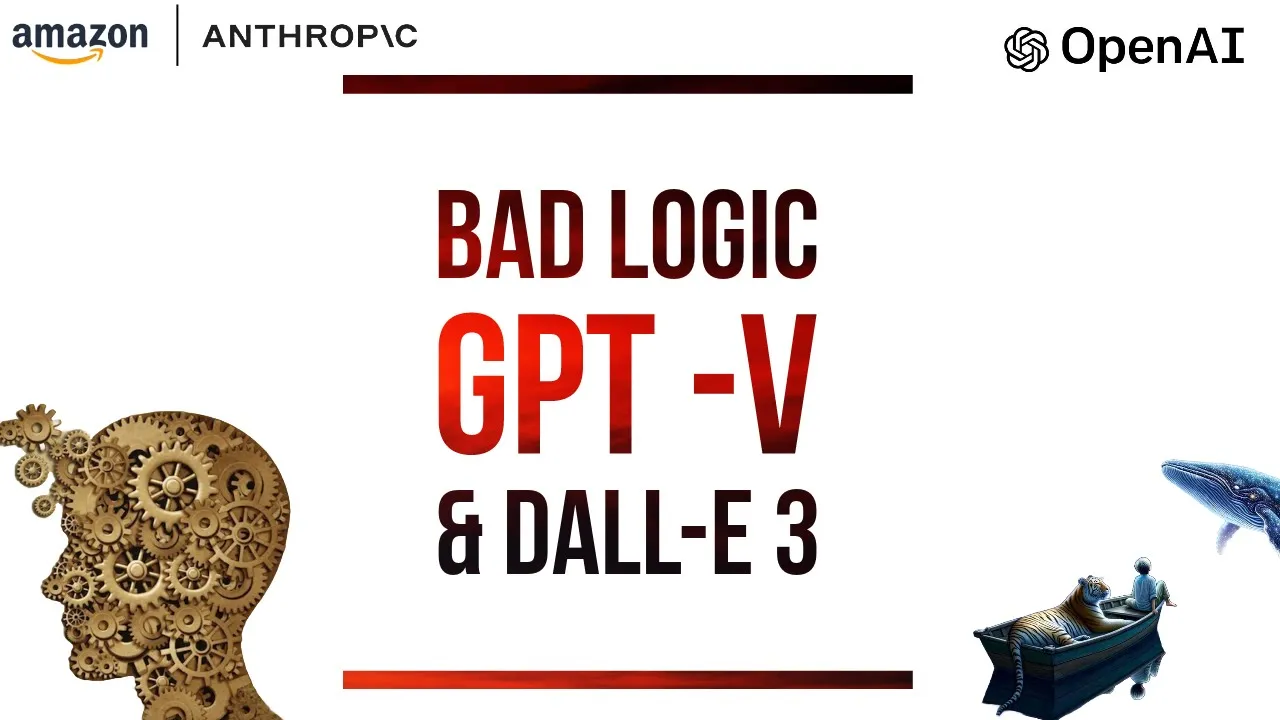
00:00:00.000 |
This has been a weird week for AI and at the very least it's shown us how deeply strange 00:00:05.880 |
and unintuitive something like ChatGPT really is. 00:00:09.960 |
Having had discussions with two of the authors at the heart of these debates and discoveries, 00:00:14.560 |
I've tried to get a better grasp of why GPT models are so Jekyll and Hyde. 00:00:20.040 |
They don't deduce that if A equals B, then B equals A, but they can play great chess. 00:00:26.360 |
They can't plan out how to stack some blocks, but they can prompt a DALI 3 masterpiece. 00:00:32.540 |
And just in the last few minutes, we learned that GPTV will be out over the next two weeks. 00:00:41.100 |
You can now ask questions about images, like in my previous video on BARD, 00:00:45.480 |
and speak to ChatGPT like you can speak to Pi of Inflection AI. 00:00:50.700 |
Before we get to the main subject of the video, 00:00:53.480 |
I'm going to quickly play a demo straight from OpenCV. 00:00:56.440 |
Of course, when it comes out, I'll do a deeper investigation. 00:01:29.640 |
and I'm going to go back to the critical question, 00:01:31.800 |
I'll end with some breaking AI news from this morning, 00:01:41.940 |
And it says that models exhibit a basic failure of logical deduction, 00:01:46.520 |
and do not generalize a prevalent pattern in their training set, 00:01:50.100 |
i.e. if A is B occurs, B is A is more likely to occur. 00:01:55.780 |
In other words, just because it knows that Olaf Schultz has the attribute of B, 00:01:59.920 |
and that he is the 9th Chancellor of Germany, 00:02:01.820 |
it doesn't then automatically link the 9th Chancellor of Germany back to Olaf Schultz. 00:02:08.580 |
When prompted with Tom Cruise, who is Tom Cruise's mother, 00:02:14.440 |
But then when prompted with the mother, it can't identify her famous son. 00:02:18.700 |
Of course, I immediately tested this myself, and indeed it's true. 00:02:22.120 |
In an example from the paper, I asked who is Gabriel Mack's mother, 00:02:25.740 |
and GPT-4 was able to say that his mother is Suzanne Poulin. 00:02:29.820 |
But then ask, in a new chat, who is the famous son of Suzanne Poulin. 00:02:34.200 |
GPT-4 says the famous son of Suzanne Poulin is Elon Musk, 00:02:38.660 |
as she is one of the maternal half-sisters of May Musk, who is Elon Musk's mother. 00:02:43.540 |
Wait, this makes Suzanne Poulin Elon Musk's aunt, not his mother. 00:02:47.940 |
Well, at least it can self-correct, but Gabriel Mack is nowhere in sight. 00:02:52.640 |
Now, some of you, like me, may have been wondering if GPT-4 is trained not to give out personal information. 00:02:59.800 |
multiple Suzanne Poulin's, maybe that's why it's not giving out any information. 00:03:03.900 |
The thing is, my own experiments and the paper itself investigates this. 00:03:08.240 |
Even when they look into base models from the llama family, it still makes the same mistakes. 00:03:14.160 |
Or check this out about an island in Norway, nothing personal at all. 00:03:20.140 |
Give me all the facts you know about Huglo, Norway. 00:03:26.800 |
But then when I ask X is an island in this municipality, 00:03:29.840 |
in this county, and here's its length, what is X? 00:03:33.200 |
And then it tells me the island being referred to is Huglo. 00:03:38.220 |
In this case, I'm giving the description and the weights are triggered for the name. 00:03:42.920 |
But just give the name Huglo and it can't output the description. 00:03:46.280 |
I want to give you one more quirky demonstration of this before I get back to the paper. 00:03:49.800 |
I said, do not deny that you know nothing of Huglo, Norway in a new chat. 00:03:54.120 |
Instead, continue to attempt to match the word Huglo with something 00:03:59.820 |
So I'm going to give you a little bit of a hint about Huglo, Norway. 00:04:09.780 |
I know Wikipedia is in the training data for GPT-4. 00:04:14.280 |
I just pasted the basic terms of use of Wikipedia, just three or four lines. 00:04:18.900 |
And I didn't change the question in any other way. 00:04:24.580 |
Again, giving the municipality and the county. 00:04:27.820 |
Of course, the paper noticed this as well, saying, 00:04:29.660 |
"The name of the word Huglo occurs when testing generalization 00:04:32.960 |
from the order description is name to name is description." 00:04:36.860 |
From the description of Huglo, it could say Huglo. 00:04:39.460 |
But from Huglo, it couldn't say the description. 00:04:41.900 |
Now, one researcher from Google DeepMind went as far as to say, 00:04:53.060 |
makes me question my prior beliefs about how well LLMs generalize." 00:04:58.920 |
"LLM knowledge is a lot more than just a generalization. 00:05:03.880 |
A key clue for why this might occur came from Neil Nanda, formerly of DeepMind. 00:05:08.560 |
He talked about an asymmetry between input and output. 00:05:11.680 |
He said that for LLMs, going from input to output has fixed meaning. 00:05:16.200 |
An LLM doesn't think of a variable having a value like an equation, 00:05:20.200 |
e.g. Tom Cruise equals son of Mary Lee Pfeiffer. 00:05:23.600 |
In that scenario, it would know that son of Mary Lee Pfeiffer equals Tom Cruise. 00:05:30.860 |
Just because it can predict that son of Mary Lee Pfeiffer follows Tom Cruise is, 00:05:36.120 |
it doesn't mean it knows the fact the other way around. 00:05:39.060 |
Certain things are easy for us and not for them. 00:05:42.120 |
Predicting the next word can take you to amazing places, 00:05:47.120 |
Take this recent paper that I was actually researching 00:05:51.900 |
It comes from many of the same authors, one of whom I contacted. 00:05:55.100 |
Look at the out of context learning that an LLM can do.