back to indexSterling Anderson, Co-Founder, Aurora - MIT Self-Driving Cars
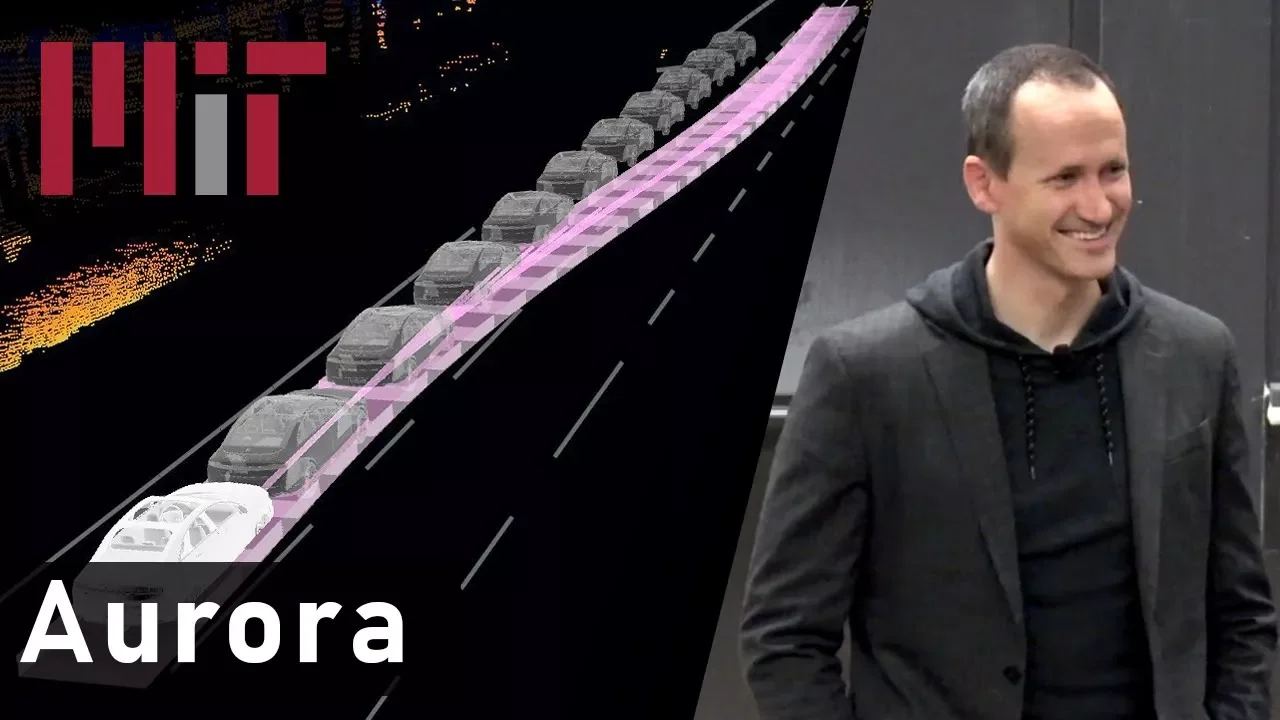
Chapters
0:0 Sterling Anderson
3:3 The Intelligent Co-Pilot
26:5 Technological Bottlenecks and Challenges
30:37 Go no-Go Tests
36:17 What's Your Opinion about Cooperation of Self-Driving Vehicles
00:00:07.600 |
Previously, he was the head of the Tesla Autopilot team 00:00:17.880 |
working on shared human machine control of ground vehicles, 00:00:45.840 |
I've just landed this afternoon from Korea via Germany, 00:00:52.400 |
And so I may speak a little slower than normal. 00:01:00.080 |
somebody flag it to me and we'll try to make corrections. 00:01:03.600 |
So tonight I thought I'd chat with you a little bit 00:01:08.080 |
It's been just over 10 years since I was at MIT. 00:01:19.680 |
And so I wanted to talk with you a little bit 00:01:28.000 |
where we go from here and what the next steps are, 00:01:32.660 |
but also for the company that we're building, 00:01:38.360 |
To start out with, there are a few sort of key phases 00:01:43.360 |
or transitions in my journey over the last 10 years. 00:01:49.600 |
I worked with Carl Ianniema, Emilio Fusoli, John Leonard, 00:01:53.900 |
a few others on some of these sort of shared, 00:02:23.380 |
enhanced convenience features from auto steer 00:02:27.340 |
to adaptive cruise control that we're able to refine 00:02:33.460 |
And then from there in December of last year, 00:02:42.420 |
So to start out with, when I came to MIT, it was 2007. 00:02:47.100 |
The DARPA urban challenges were well underway at that stage. 00:02:52.320 |
is find a way to address some of the safety issues 00:03:06.000 |
What you see here is a simulation of that operating. 00:03:09.280 |
I'll tell you a little bit more about that in just a second. 00:03:12.560 |
But to explain a little bit about the methodology, 00:03:15.240 |
the innovation, the key approach that we took 00:03:18.680 |
that was slightly different from what traditional 00:03:24.680 |
was instead of designing in path space for the robot, 00:03:27.920 |
we instead found a way to identify, plan, optimize, 00:03:32.920 |
and design a controller subject to a set of constraints 00:03:38.120 |
And so what we were doing is looking for homotopies 00:03:42.920 |
that's pockmarked by objects, by other vehicles, 00:03:53.200 |
you would have a set of each unique set of paths 00:04:00.180 |
that will take you from one location to another through it. 00:04:06.600 |
which is the Delaunay triangulation of said environment, 00:04:11.980 |
you can then tile those together rather trivially 00:04:14.260 |
to create a set of homotopies and transitions 00:04:22.160 |
sort of a given set of options for the human. 00:04:29.760 |
of imposing certain constraints on human operation 00:04:48.560 |
within a constraint-bounded n-dimensional tube 00:04:54.040 |
you imagine for a moment edges of the roadway 00:04:56.760 |
or circumventing various objects in the roadway. 00:05:05.420 |
tire friction imposed limits on side slip angles. 00:05:11.960 |
what we did is found a way to create those homotopies, 00:05:14.340 |
forward simulate the trajectory of the vehicle 00:05:22.700 |
that would optimize its stability through that. 00:05:24.980 |
We use model predictive control in that work. 00:05:27.700 |
And then taking that forward simulated trajectory, 00:05:34.860 |
For instance, if the objective function for that 00:05:41.220 |
or minimize some of these parameters like wheel side slip, 00:05:44.860 |
then wheel side slip is a fairly good indication 00:05:48.060 |
of how threatening that optimal maneuver is becoming. 00:05:54.340 |
in a modulation of control between the human and the car, 00:05:58.340 |
such that should the car ever find itself in a state 00:06:01.460 |
where that forward simulated optimal trajectory 00:06:16.140 |
And we played with a number of different methods 00:06:22.020 |
to ensure that we didn't throw off the human mental model, 00:06:30.820 |
that we were able to arrest accidents before they happen. 00:06:37.480 |
that was fairly faithful to the behavior we saw 00:06:45.420 |
Ford provided us with a Jaguar S-Type to test this on. 00:06:51.740 |
is there's a blue vehicle and a gray vehicle. 00:06:53.340 |
Both in both cases, we have a poorly tuned driver model, 00:07:12.240 |
You'll notice that obviously the driver becomes unstable, 00:07:23.880 |
It doesn't care where the vehicle lands on this roadway, 00:07:37.800 |
You'll notice that as this scenario continues, 00:07:42.520 |
what you see here on the left is in this green bar 00:07:46.040 |
is the portion of available control authority 00:07:58.200 |
of what the human and what the automation are providing. 00:08:03.280 |
And what results is a path for the blue vehicle 00:08:07.680 |
that actually better tracks the human's intended trajectory 00:08:15.280 |
Again, the copilot is keeping the vehicle stable, 00:08:18.900 |
The human is hewing to the center line of that roadway. 00:08:34.560 |
a human's mental model by causing the vehicle's behaviors 00:08:48.320 |
that we had early on was if we couple the computer control 00:08:57.200 |
and allow the human to feel actually a backwards torque 00:09:09.100 |
Is that more confusing or less confusing to a human? 00:09:11.920 |
And it turns out it depends on how experienced 00:09:28.960 |
in response to seeing the wheel turning opposite 00:09:37.600 |
human interface challenges that we were dealing with here. 00:09:46.360 |
developing a number of sort of micro applications for it. 00:10:08.880 |
So in this case, what you see is a human driver 00:10:14.040 |
as one would when operating an unmanned vehicle, 00:10:23.160 |
is the top down view of what the vehicle sees. 00:10:32.880 |
And what we did is we set up about 20 drivers, 00:10:35.120 |
20 test subjects, looking at this control screen 00:10:40.120 |
and operating the vehicle through this track. 00:10:46.560 |
with prizes for the winners, as one would expect, 00:10:51.000 |
and penalized them for every barrel they hit. 00:10:57.720 |
If they brushed a barrel, they got a one second penalty. 00:11:00.400 |
And they were to cross the field as fast as possible. 00:11:03.200 |
And they had no line of sight connection to the vehicle. 00:11:05.240 |
And we played with some things on their interface. 00:11:10.640 |
We delayed it, as one would realistically expect 00:11:15.080 |
And then we either engaged or didn't engage the co-pilot 00:11:27.320 |
It declined by about 72% when the co-pilot was engaged 00:11:32.120 |
We also found that even with that 72% decline 00:11:39.800 |
I'm blanking on the amount, but it was 20 to 30 percentage. 00:11:52.000 |
They didn't know if the co-pilot was active or not. 00:11:53.560 |
And I would ask them, how much control did you feel 00:11:57.760 |
And I found that there was a statistically significant 00:12:01.680 |
increase of about 12% when the co-pilot was engaged. 00:12:05.360 |
That is to say, drivers reported feeling more control 00:12:11.200 |
when the co-pilot was engaged than when it wasn't. 00:12:15.400 |
It turns out they actually, the average level of control 00:12:20.540 |
So they were reporting that they felt more in control 00:12:26.260 |
which was interesting and I think bears a little bit 00:12:37.040 |
what I wanted it to do, maybe not what I told it to do, 00:12:42.540 |
And fun to, I think the most enjoyable part of this 00:12:49.100 |
at the end of the study and presenting some of this 00:12:53.020 |
So from there, we looked at a few other areas. 00:12:59.180 |
My, Carl Yanima and I looked at a few different opportunities 00:13:08.240 |
was in a very different place than it is today. 00:13:15.340 |
This is the logo, it may look familiar to you. 00:13:29.300 |
At the time, very few saw self-driving as a technology 00:13:34.300 |
that was really gonna impact their business going forward. 00:13:38.340 |
They were, in fact, even ride sharing at the time 00:14:08.380 |
Drew Bagnell is a professor at Carnegie Mellon University, 00:14:11.140 |
exceptional machine learning and applied machine learning, 00:14:28.980 |
into the full-on realization that self-driving, 00:14:31.740 |
and particularly self-driving and ride sharing 00:14:33.940 |
and vehicle electrification are three vectors 00:14:38.780 |
That was something that didn't exist 10 years ago. 00:14:44.980 |
in some of these machine learning techniques, 00:14:56.820 |
and the availability of low-power GPU and TPU options 00:15:04.460 |
In sensing technologies, in high-resolution radar, 00:15:09.700 |
So it's really a unique time in the self-driving world. 00:15:12.180 |
A lot of these things are really coming together now. 00:15:15.380 |
And we felt like by bringing together an experienced team, 00:15:29.260 |
in applied machine learning together with our experience 00:15:35.660 |
of where some of the pitfalls tend to be down the road 00:15:42.740 |
as you get into the long tail of corner cases 00:15:55.500 |
operating in both Palo Alto and Pennsylvania. 00:15:57.780 |
A couple of weeks ago, we announced that Volkswagen Group, 00:16:04.020 |
also one of the largest automakers in the world, 00:16:08.980 |
We will be developing and are developing with them 00:16:12.380 |
a set of platforms that ultimately will scale 00:16:15.380 |
that our technology on their vehicles across the world. 00:16:19.100 |
And one of the important elements of building Lex, 00:16:25.580 |
what this group would be most interested in hearing. 00:16:34.140 |
One of the things that we found very important 00:16:36.980 |
was a business model that was non-threatening to others. 00:16:45.940 |
in my case, a decade, in Chris's case, almost two, 00:16:48.460 |
really lies in the development of the self-driving systems. 00:17:02.100 |
is to get this technology to market as quickly, 00:17:05.860 |
that mission is best served by playing our position 00:17:10.380 |
and working well with others who can play theirs, 00:17:13.460 |
which is why you see the model that we've adopted 00:17:16.260 |
and is now, you'll start to see some of the fruits of that 00:17:19.180 |
through these partnerships with some of these automakers. 00:17:21.580 |
So at the end of the day, our aspiration and our hope 00:17:24.780 |
is that this technology that is so important in the world 00:17:28.740 |
in increasing safety, in improving access to transportation, 00:17:33.900 |
in the utilization of our roadways in our cities. 00:17:39.660 |
where I didn't start by rattling off statistics 00:17:44.500 |
If you haven't heard them yet, you should look them up. 00:17:48.900 |
The fact that most vehicles in the United States today 00:17:57.940 |
The amount of land that's taken up across the world 00:18:06.540 |
The number of people, I think in the United States, 00:18:28.780 |
It's a tremendously exciting technological challenge. 00:18:34.660 |
I think is a really unique opportunity for engineers 00:19:07.540 |
I wanted to know if you had any thoughts about that. 00:19:10.420 |
- Yeah, I don't wanna talk about Tesla too much 00:19:26.140 |
And you get to market most quickly and safely 00:19:27.860 |
if you leverage multiple modalities, including LiDAR. 00:19:30.860 |
These are all just to clarify what's running in the background 00:19:42.940 |
A lot of customers have visceral type connections 00:19:48.460 |
the car enthusiast market being affected by AVs 00:19:52.300 |
how the AVs will be designed around those type of customers. 00:20:00.540 |
I very much appreciate being able to drive a car 00:20:08.660 |
I very much don't appreciate driving in others. 00:20:16.140 |
I almost literally pounding my steering wheel 00:20:18.900 |
sitting in Boston traffic, on my way to somewhere. 00:20:27.340 |
I think the opportunity really is to turn that, 00:20:30.780 |
turn sort of personal vehicle ownership and driving 00:20:34.820 |
into more of a sport and something you do for leisure. 00:20:37.660 |
I see it, a gentleman sometime ago asked me to talk, 00:20:45.420 |
hey, don't you think this is a problem for the country? 00:20:55.060 |
that's just something a human should know how to do. 00:21:05.180 |
If you wanna know how to ride a horse, go ride a horse. 00:21:10.740 |
or go out to a mountain road that's been allocated for it. 00:21:14.540 |
Ultimately, I think there is an important place for that 00:21:21.300 |
but I think there is so much opportunity here 00:21:28.940 |
particularly in places where it's not fun to drive, 00:21:47.420 |
The first one is, so we heard last week from, 00:21:57.380 |
And you seem to have ramped up extremely fast. 00:22:00.420 |
So is there a licensing model that you've taken? 00:22:10.700 |
- So just to be clear, we're not actually commercializing. 00:22:20.380 |
be running pilots, as we announced a week or two ago 00:22:28.420 |
from broad commercialization of the technology. 00:22:31.020 |
And I don't wanna get too much into the nuances 00:22:41.140 |
in very close partnership with our automotive partners. 00:22:44.020 |
Because at the end of the day, they understand their cars, 00:22:54.460 |
are fairly well positioned, provided they have 00:22:57.780 |
the right support in developing the self-driving technology, 00:22:59.980 |
they're fairly well positioned to roll it out at scale. 00:23:11.540 |
do you see an open source model for autonomous cars 00:23:27.540 |
In the long run, it's not clear to me what will happen. 00:23:34.980 |
I think there will be a handful of successful 00:23:41.420 |
Nowhere near the number of self-driving companies today, 00:24:11.980 |
So I'd be interested to know what you would say 00:24:16.140 |
if this part of the architecture was 10 times better, 00:24:25.820 |
then, so I'd be interested to hear your perspective 00:24:36.140 |
The economics of operating a self-driving vehicle 00:24:45.180 |
that business case closes even with high cost of sensors. 00:24:51.140 |
And that's part of why the gentleman earlier who asked, 00:24:58.780 |
If your target is to initially deploy these in fleets, 00:25:03.060 |
you would be wise to start at the top end of the market, 00:25:06.180 |
develop and deploy a system that's as capable as possible, 00:25:09.300 |
as quickly as possible, and then cost it down over time. 00:25:23.300 |
of going to market, and we believe that the right model 00:25:31.780 |
you can cost down, you'll cost down the center. 00:25:34.380 |
Inevitably, there's no unobtainium in LiDAR units today. 00:25:37.940 |
There's no reason fundamentally that a shared cost 00:25:40.780 |
of a LiDAR unit will lead you to a $70,000 price point. 00:25:43.740 |
However, if you build anything in low enough volumes, 00:25:53.020 |
They'll work their way into tier one suppliers, 00:26:12.300 |
is and remains that of forecasting the intent 00:26:21.980 |
but also in response to your own decisions in motion. 00:26:28.500 |
but it's something more than a perception problem. 00:26:40.580 |
We're excited about some of the tools that we're using 00:26:43.980 |
in interleaving various modern machine learning techniques 00:26:48.340 |
throughout the system to do things like project 00:26:59.260 |
- Like an expert system kind of approach, right? 00:27:15.500 |
when you're no longer putting out demonstration videos, 00:27:20.780 |
but you're instead just putting your head down 00:27:25.540 |
that's the kind of problem you tend to deal with. 00:27:43.160 |
isn't there always the possibility for outside agents 00:27:46.180 |
with malicious intent to use it for their own gain? 00:27:52.500 |
how do you intend to protect against attacks like that? 00:28:09.940 |
And so I think it just requires a very good team 00:28:18.620 |
and I certainly wouldn't pretend to have a plan 00:28:23.940 |
We try to leverage best practices where we can 00:28:28.260 |
in the fundamental architecture of the system 00:28:38.260 |
But at the end of the day, it's just a constant, 00:28:51.980 |
Since driving has kind of been designed around 00:28:54.020 |
like a human being at the center since the beginning, 00:29:02.460 |
and maybe even within individual car differences 00:29:04.660 |
that open up, could cars go 150 miles an hour 00:29:12.380 |
doesn't need to be paying attention and stuff like that? 00:29:16.180 |
And that's something that's very exciting, right? 00:29:27.260 |
when self-driving technology gets incorporated 00:29:35.380 |
augmented reality services or location services, 00:29:49.940 |
And it allows them to change the very vehicles themselves. 00:29:58.100 |
As we validate some of these self-driving systems 00:30:03.020 |
and confirm that they do in fact reduce the collision, 00:30:08.580 |
you can start to pull out a lot of the extra mass 00:30:14.460 |
and other things that we've added to vehicles 00:30:26.820 |
where we don't crash, there is much less need 00:30:34.220 |
- Hi, I have a question about the go or no go test 00:30:45.420 |
assuming that the driver has pressed the wrong pedal. 00:30:48.580 |
When you test, when do you decide to launch that feature? 00:30:53.140 |
in all scenarios because your dataset might not be-- 00:30:55.300 |
- Oh, it's a statistical evaluation in every case, right? 00:31:00.420 |
This is part of the art of self-driving vehicle development 00:31:05.220 |
is you will never have comprehensively captured 00:31:22.780 |
And so you'll never actually have characterized everything. 00:31:26.980 |
What you will have done, hopefully, if you do it right, 00:31:29.980 |
is you will have established with a reasonable degree 00:31:33.060 |
of confidence that you can perform at a level of safety 00:31:39.140 |
and you're confident that you've reached that threshold, 00:32:06.880 |
So how do you respond to fear and negative effects 00:32:12.740 |
And specifically, what do you see as the positive 00:32:15.340 |
and negative implications of future day self-driving? 00:32:35.060 |
I do worry a little bit about the displacement of jobs. 00:32:45.580 |
I think it's incumbent on us to find a good way 00:32:58.220 |
There are a few opportunities that are interesting 00:33:03.220 |
in that regard, but I think it's an important thing 00:33:10.560 |
And by the time we've got these self-driving systems 00:33:14.420 |
on the roads, really starting to place that labor, 00:33:23.020 |
My question was more about your business model, 00:33:25.220 |
again, with partnering with both VW and Hyundai, 00:33:29.100 |
and your just perspective on how you were able 00:33:33.700 |
Did not one of them wanna go sort of exclusive with you? 00:33:37.540 |
And what was your sort of thought process about that? 00:33:53.020 |
that the right way to do that is by providing it 00:33:57.700 |
To every automaker who shares our vision and our approach, 00:34:02.340 |
we were pleased to see that both Volkswagen Group, 00:34:06.220 |
and I'm assuming you all know the scope of Volkswagen, right? 00:34:19.140 |
They both shared our vision of how we should do this, 00:34:28.980 |
making a difference at scale through their platforms. 00:34:34.780 |
Volkswagen has, I think, a very admirable set of initiatives 00:34:38.020 |
around vehicle electrification, a few other things. 00:34:42.460 |
And so, for us, it was important that we enable everyone, 00:34:47.220 |
and that was kind of what Aurora was started to do. 00:34:51.740 |
Now that I see a lot of companies are coming up 00:35:00.500 |
So would we see something like an open network 00:35:06.460 |
And would this, in any way, increase the safety 00:35:10.140 |
or the performance of vehicles and stuff like that? 00:35:12.060 |
- Yeah, I think you're getting it vehicle-to-vehicle, 00:35:15.220 |
vehicle-to-infrastructure type communication. 00:35:19.420 |
and it's certainly, it's only positive, right? 00:35:28.140 |
The challenge has historically been with vehicle-to-vehicle, 00:35:30.620 |
and particularly vehicle-to-infrastructure, or vice versa, 00:35:34.820 |
that it doesn't scale well, one, and two, it's been slow. 00:35:39.020 |
It's been much slower in coming than our development. 00:35:46.740 |
that those communication protocol are available to us. 00:35:52.540 |
and it will certainly be a benefit once they're here. 00:36:06.580 |
I would have certainly used those 10 years ago. 00:36:13.300 |
a lot of the problems that would have solved. 00:36:19.300 |
about the cooperation of self-driving vehicles? 00:36:28.660 |
you can achieve a lot of benefits to the traffic. 00:36:32.300 |
That is where a lot of the benefits come from 00:36:40.140 |
And specifically, the better we understand demand patterns, 00:36:48.460 |
the better we can sort of optimally allocate these vehicles 00:36:59.060 |
these three vectors of vehicle electrification, 00:37:06.460 |
really come together with a unique value proposition. 00:37:13.140 |
- Thank you so much for a great talk and being here.