back to indexA practical summary of the covid-19 situation
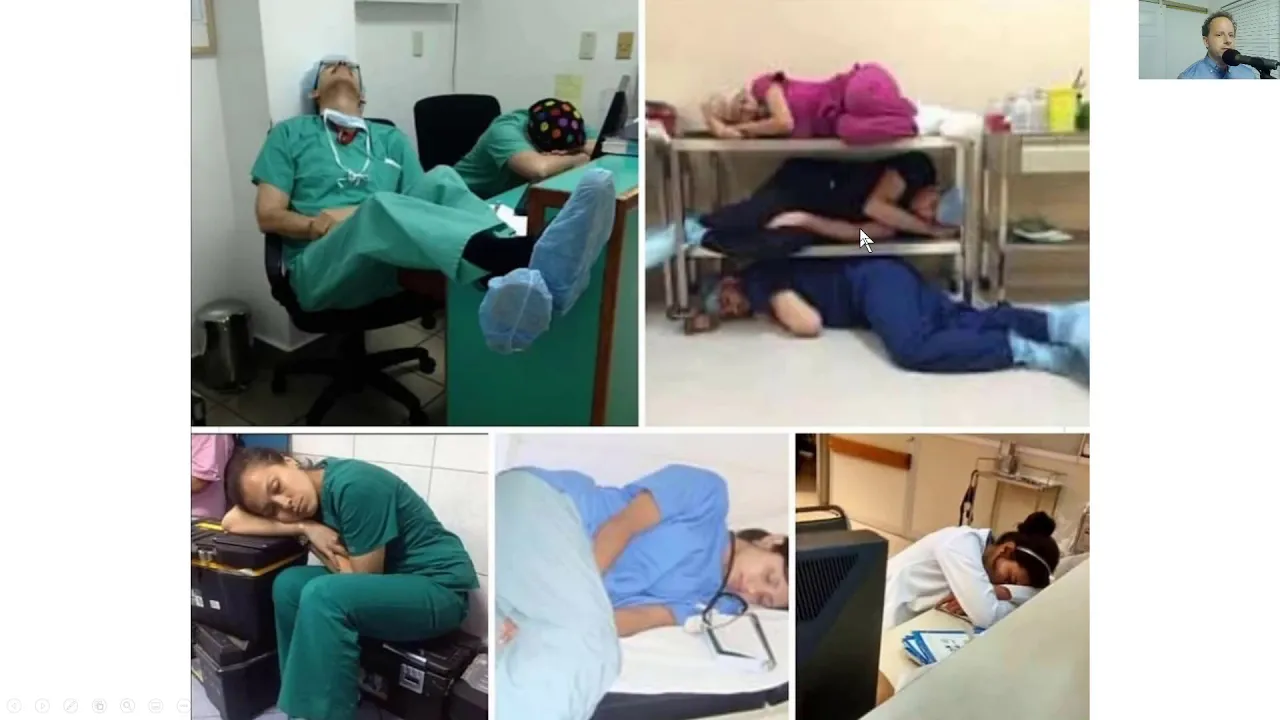
Chapters
0:0 Intro
7:2 Italy
9:58 UK
12:27 US
18:29 Early response
19:57 Disinformation
20:57 Masks
23:53 Trust situation
25:4 Research on masks
26:1 Spreading the disease
26:52 Young people
28:7 The 1918 flu
31:1 Supply chain
32:11 Hand sanitizer
32:59 Code Red game
35:45 Data scientists
36:55 Data testing
00:00:00.000 |
So we kind of spent the weekend putting together some thoughts about some of the problems we 00:00:06.680 |
saw and how people were thinking about this and tackling this and I was, I can't believe 00:00:14.040 |
As you see over half a million people read this thing, which we've never reached that 00:00:22.080 |
So clearly there's kind of orders of magnitude more interest in what we have to say about 00:00:26.560 |
COVID-19 in the community than deep learning, so should probably talk about it briefly. 00:00:33.560 |
You know, one of the things we talked about in this, this post is now translated into 00:00:38.800 |
like 15 languages, thanks to amazing volunteers. 00:00:42.080 |
I did a little Twitter summary of it, I'll mention a couple of the things we said. 00:00:48.440 |
Now that this is eight days old, a lot of this is pretty well understood, but for those 00:00:52.560 |
people that aren't yet locked down like San Francisco is, you should probably be thinking, 00:00:57.360 |
acting as if you are, certainly canceling events, getting together with more than 10 00:01:02.440 |
people, is giving an opportunity to basically kill them and their families, because you 00:01:09.800 |
So work from home if you can, not everybody can, we'll talk a bit about sanitation, it's 00:01:20.960 |
Most importantly, a lot of people watching this are in a position of authority, and so 00:01:24.440 |
I particularly want to talk to you and say, you know, give, provide sick leave, make meetings 00:01:32.160 |
You're the folks who can save the most lives, perhaps other than the medical people. 00:01:38.240 |
There are still people saying this is just like the flu, eight days ago, most people 00:01:42.880 |
seem to be saying that, but the flu has a death rate of about 0.1%. 00:01:49.560 |
The director of the Center for Communicable Diseases at Harvard estimates that for COVID-19 00:01:59.440 |
So 10 or 20 times more infectious, we don't know that, but this is a guess from somebody 00:02:04.800 |
who knows what he's talking about, and it's not, you know, it's somewhat widely accepted 00:02:10.440 |
amongst epidemiologists, some think it's higher, some think it's lower. 00:02:15.560 |
Other modeling suggested it was 1.6% in China in February. 00:02:22.080 |
So it's much, much more infectious, sorry, much, much more deadly than the flu. 00:02:29.920 |
One of the really terrifying things also is it spreads incredibly quickly. 00:02:36.660 |
So it's, there's nothing, you know, from an epidemiological point of view there's nothing 00:02:43.980 |
that suggests anything you understand about the flu is going to help you understand COVID-19. 00:02:51.140 |
A lot of people say they're basically, their responses don't panic. 00:03:00.280 |
It can tend to lead to kind of apathy or hubris. 00:03:04.320 |
In China, by the time they were where we US was when we wrote this eight days ago, tens 00:03:11.780 |
of millions of people were already on lockdown and two from all these hospitals were built. 00:03:17.160 |
China did this amazing, I mean, they did plenty of wrong things at the start in terms of not 00:03:22.640 |
actually listening to the whistleblowers and in fact arresting them. 00:03:25.920 |
But then they did an astonishing response, Italy, not so much. 00:03:32.880 |
And at the time we were writing this, they had to go to the point of locking down 16 00:03:38.400 |
million people too late, we'll talk about more about that in a moment. 00:03:45.440 |
And for scientists, one of the challenges here is we tend to not like to do things when 00:03:52.120 |
We demand high levels of evidence and, you know, this is not peer review level stuff. 00:04:04.480 |
What's the kind of, from a Bayesian point of view, figure out the priors. 00:04:12.720 |
What would be the utility impact of doing these two things? 00:04:18.820 |
So we need to learn to behave in the, you know, in a kind of an optimal way under uncertainty, 00:04:27.880 |
which is not easy and not very compatible with kind of usual scientific approaches. 00:04:36.000 |
But we can see empirically, you know, what's actually happening in Italy, for example. 00:04:44.780 |
So we can, we don't have to guess totally about where things will be in 10 days time, 00:04:50.280 |
because we can see what's happening in countries that are 10 days ahead of the US or the UK. 00:04:57.460 |
One of the horrific things about this we pointed out in our post is that in the US in particular, 00:05:04.880 |
those impacted are those who can least afford to be. 00:05:09.240 |
So the lowest 10% of wage earners in the US of that group only a third have paid sick leave. 00:05:16.880 |
So one of the things that happens in a country like the US that doesn't have very strong, 00:05:20.600 |
to say the least, social safety nets is we force people to go to work and, you know, financially. 00:05:27.560 |
And as a result, we force them to spread their possible infection to everybody else at work, 00:05:34.080 |
which is terrible for the economy and terrible for society. 00:05:46.360 |
It seems like it's something that people have trouble taking on board emotionally. 00:05:52.280 |
Like this was a, and I'm definitely not hassling this person. 00:05:54.720 |
I want to, you know, this person's been super brave, but I want to point out this guy's 00:05:58.120 |
a doctor and he was tweeting just at the start of March, "Hey, don't worry about this. 00:06:21.200 |
You know, look at all these other things which are much worse than COVID-19." 00:06:27.000 |
He said, "Ah, my hospital asked physicians to form a response team. 00:06:33.960 |
And then, you know, this is a terrifying bit. 00:06:41.760 |
And I can tell you in 18 years of medical practice, I've never seen anything like this. 00:06:45.800 |
And if you now look at this, look at this guy's Twitter account, it's full of messages 00:06:53.320 |
So the different, you know, when you see people on the ground, how they're reacting, I find 00:07:03.880 |
So, you know, the data, and I'm focusing on the US because I know most of our people watching 00:07:12.480 |
this are in the US, but if you look at somewhere like Italy and you say, "What was Italy looking 00:07:20.060 |
like in terms of the number of cases on the 23rd of February?" 00:07:25.080 |
United States on the 5th of March had about the same number of cases. 00:07:30.240 |
And so then if you go a day forward, Italy and United States a day later have a similar 00:07:34.760 |
number of cases, and another day later they have a similar number of cases, and so forth. 00:07:39.480 |
So this is just for Italy and the US, but actually if you draw this graph for most Western nations 00:07:47.120 |
in kind of cold or temperate climates, it looks exactly the same. 00:07:52.120 |
Everybody is following Italy just by a different number of days. 00:07:55.440 |
And so that means that we know that if we respond in the same way as Italy, we're basically 00:08:03.000 |
So Italy is a very useful role model to understand. 00:08:07.440 |
So you know, as data scientists, here's some great data. 00:08:13.960 |
So turning that data into reality, here's what people were saying on the 28th of February. 00:08:20.880 |
So the 28th of February is here, okay? Italy had 888 cases, the equivalent of the US on 00:08:30.080 |
So you know, tourists are hanging out, people are chilling. 00:08:37.440 |
And then by yesterday, Italy was losing 368 lives in a single day. 00:08:53.440 |
Many of those lives being lost in, you know, a fairly small number of locations. 00:09:02.640 |
You see it as, oh, hi folks, I'm sorry, but our president of the medical group just died. 00:09:11.320 |
The director of our training school just died. 00:09:18.440 |
These are pictures from Italy, it's, you know, everybody is just working all the time. 00:09:26.240 |
There is not nearly enough beds and doctors are having to make horrific decisions where 00:09:33.080 |
they have to make a judgment call about who is it worth them treating. 00:09:38.480 |
Who is too old that it's going to die anyway, they just let them die. 00:09:44.160 |
Who is, anybody under 65, last time I heard was basically not being looked at on the assumption 00:09:49.600 |
that they should be, you know, that they might be fine. 00:10:02.080 |
There are countries that look particularly scary, perhaps the most scary outside of Italy 00:10:10.320 |
The Imperial College just released their latest modeling, which actually had some, looks like 00:10:17.640 |
some errors that might have underestimated the number of fatalities. 00:10:22.520 |
But even with that, this red bar here represents the total hospital capacity in the UK. 00:10:32.680 |
And these different lines represent the number of people that need hospitalization under 00:10:37.160 |
the assumption of UK does nothing versus UK closes schools and universities versus UK isolates 00:10:45.680 |
cases versus UK isolates cases and quarantines households versus UK isolates cases, quarantines 00:10:57.240 |
In every case the difference between the number of people required to be in hospital versus 00:11:03.320 |
the amount of people that can be in hospital is vast. 00:11:11.760 |
This is basically showing what percentage of symptomatic cases require hospitalization. 00:11:19.760 |
And in the over 50 group, it's well, you know, 1 in 10 to 1 in 4. 00:11:27.240 |
And of that group, how many require critical care goes from 1 in 10 to 70%. 00:11:34.000 |
Critical care basically means ventilation, oxygenation. 00:11:37.640 |
If you don't get it, I don't know exactly what happens, but the answer seems to be very 00:11:49.120 |
So this is scary stuff and the UK obviously did nothing for too long. 00:11:58.040 |
And countries like the Netherlands and Sweden have explicitly made a decision at this point 00:12:03.880 |
to follow the UK's example, even though the UK is now, it was actually the Imperial College 00:12:11.120 |
that did the original incorrect, totally incorrect modeling that caused the incorrect response 00:12:19.960 |
And so people who are still saying, let's do what the UK did heading in this direction 00:12:25.560 |
and basing things off totally wrong information. 00:12:29.400 |
Talking of information, it's very hard to be data scientists in this environment because 00:12:36.740 |
In the United States, the number of tests we're doing per million people, 23 compared 00:12:42.360 |
to South Korea, 3,700, things are starting to ramp up now, but it's a huge shortage. 00:12:49.840 |
And just to orient on this graph, the blue circles are showing the number of tests and 00:12:54.560 |
the per capita per million and the red circles are showing the population size. 00:13:02.900 |
So one of the things that's really interesting is to look at South Korea and China because 00:13:11.020 |
these are countries that have, you know, a very different experience as you'll see to 00:13:25.620 |
This is from today and you can see we start basically at 0.0 for each country is when 00:13:35.320 |
they have 10 deaths and then this is the cumulative number of deaths. 00:13:39.520 |
So this is a very lagging indicator from a data point of view. 00:13:44.740 |
This is something that happens along, you know, you've been infected, you've got sick, 00:13:49.480 |
you've gone to hospital, there's hospital beds and eventually if things go badly you 00:13:55.640 |
So on this very lagging indicator, you can see there's very, very different shapes, right? 00:14:01.480 |
There's the Spain and UK shape, which is still early, but it's just, you can see these shapes 00:14:07.520 |
once they are on a certain direction, they tend to kind of stick in that direction for 00:14:13.880 |
And then there's the, where's Italy, here we are, there's the Italy shape, which is pretty 00:14:22.740 |
And we've already talked about where that shape's ended up. 00:14:29.760 |
The US is a pretty big country, pretty spread out. 00:14:36.320 |
Oh, your cursor is showing offset from the curves you're talking about. 00:14:42.640 |
Yeah, it kind of just, it just goes a little slowly, okay. 00:14:50.840 |
So maybe just focus on the color of the curves you're highlighting? 00:14:59.960 |
Okay, so the pink one, US, I mean you guys can read which one it is, thanks Rachel. 00:15:05.760 |
It's a very different geography, so it looks a little different. 00:15:17.300 |
On the other hand, look at South Korea and Japan. 00:15:23.320 |
Now South Korea is super interesting because they haven't had a lockdown. 00:15:35.120 |
So economically, they're keeping their economy going. 00:15:40.880 |
And you know, for society, they're keeping their society going. 00:15:48.480 |
And the things they're doing are very different to at least what the US is doing. 00:15:52.980 |
In South Korea, they have massively invested in tests, in testing and in tests. 00:16:00.180 |
So the US is good at investing in things and making things, but currently the US is not 00:16:13.560 |
Everybody is wearing masks in South Korea and anybody who, you know, everybody's getting 00:16:19.280 |
tested regularly and that anybody who's tested with the virus is getting quarantined. 00:16:24.760 |
So South Korea is, you know, it's great to look at data, see outliers and figure out 00:16:36.000 |
China has taken their curve and flattened it. 00:16:41.340 |
And they did this by a very early lockdown and also what many would consider draconian 00:16:51.740 |
So there seems to be a couple of different models we can follow. 00:16:54.280 |
There's the lots of masks, lots of tests model of South Korea or there's the kind of major 00:17:01.800 |
But China is now at the point where they are, the disease is not really spreading anymore 00:17:09.800 |
and they're starting to remove the lockdowns and wind the economy back up again. 00:17:18.440 |
So the impact of these interventions is huge. 00:17:23.980 |
R0, which is the measure of each person, how many people do they infect on average, was 00:17:33.040 |
And after the interventions in Wuhan, it went down to 0.3. 00:17:37.820 |
So when you go down from one person infecting 4 to one person infecting 0.3, you're going 00:17:45.700 |
from a disease which is growing to a disease which is shrinking. 00:17:49.200 |
But we had a question about the previous graph. 00:17:54.440 |
What's the significance of the 33% daily increase line? 00:18:00.520 |
I think my understanding is it was just like a kind of an estimate, it's kind of like an 00:18:05.160 |
average of what seems to be happening in places that aren't really doing much around response. 00:18:11.100 |
Somehow Spain and UK are even worse than that. 00:18:13.320 |
But at the time they were adding this, it was before Spain and UK were appearing much. 00:18:18.000 |
So it's kind of like, oh Iran, early China, Italy are all kind of, that seemed to be a 00:18:22.680 |
kind of a default I guess that people were following. 00:18:38.800 |
And one region, Lodi, the green one, did a very early lockdown. 00:18:46.920 |
And you can see what happened as a result, their number of cases has not increased exponentially. 00:19:00.300 |
And the reason this matters is going back to the graph we saw earlier. 00:19:06.840 |
If you can keep this going up slowly enough, you can try to keep it under the red line, 00:19:12.280 |
which means people that need to be hospitalized get hospitalized. 00:19:16.480 |
So it makes a huge difference to the number of deaths. 00:19:19.500 |
And we saw that in the 1918 flu, where regions like, it was St. Louis, right, that responded 00:19:34.240 |
Responded early and did on the whole manage to get people into hospitals and ended up 00:19:38.640 |
with a much lower death rate than places like Philadelphia that literally put on a parade 00:19:43.840 |
for 250,000 people, just as the pandemic was spreading. 00:19:53.560 |
So it's not like we don't have data or history to learn from, we do. 00:19:59.000 |
One of the challenges is it's kind of, you know, in Rachel's work as the founding director 00:20:04.920 |
of the Center for Applied Data Ethics, she talks a lot about disinformation and trust. 00:20:10.280 |
It's very hard at the moment because we are in a low trust environment, for good reason. 00:20:16.440 |
For example, the big UK newspaper, The Daily Mail, internally writes, "Official government 00:20:24.680 |
advice is no longer adequate enough to safeguard our staff." 00:20:28.760 |
At the same time that they write in the newspaper regarding Boris and his boffins, "We must 00:20:38.760 |
So we're seeing, you know, this low trust environment being created where very clearly 00:20:46.040 |
media and governments are not always telling the truth. 00:20:51.920 |
It makes it very difficult to know where we get, you know, good information from. 00:21:01.280 |
I mentioned that kind of from an empirical point of view, we can see that countries that 00:21:06.200 |
are using masks widely are doing much better than those that aren't. 00:21:12.960 |
Correlation is not causation, but as I mentioned at the start of this discussion, we are not 00:21:22.360 |
We're looking for like, what makes sense, right? 00:21:24.560 |
And compare the cost of a mask or a bunch of masks to the cost of a lockdown. 00:21:33.960 |
If we can be South Korea, our economy is going to be much better, and of course our society 00:21:41.480 |
is going to be much better than if we have a lockdown and even that turns out not to 00:21:49.080 |
So we kind of, we have to go on the information we have. 00:21:52.920 |
We can't look for there is no peer-reviewed, definitive answer, right? 00:21:58.840 |
The fact is, when people cough, there's a lot of coughing involved in this disease. 00:22:05.720 |
It's one of the most common symptoms as a dry cough. 00:22:10.840 |
Droplets head out there somewhere up to eight meters. 00:22:14.040 |
And if you've got a mask on it, it's your mask rather than, you know, hitting your, 00:22:18.440 |
well, particularly if you've also got glasses or goggles rather than hitting your eyes and 00:22:23.640 |
So you know, that on the other hand, a lot of governments are saying they're not effective. 00:22:30.520 |
Now we don't know that, as I mentioned, but there's a good reason for them to say this, 00:22:38.000 |
I was just going to know, St. Upto Effect, she wrote an excellent op-ed on this in the 00:22:42.120 |
New York Times today called "Why Telling People They Don't Need Mass Backfired?" 00:22:46.960 |
Yeah, it's not great for all kinds of reasons. 00:22:50.000 |
I mean, you know, she mentioned the obvious things like, hey, you're saying that we don't 00:22:53.240 |
need masks, so you should stop buying them so that then doctors can have them because 00:22:59.840 |
You know, and she's kind of mentioning, oh, it's because you can't possibly know how to 00:23:04.680 |
So come on, you know, it's a five minute YouTube video showing how to put on a mask. 00:23:10.920 |
It's basically a government response where they don't want to do the stuff that's been 00:23:14.680 |
happening in Southeast Asia, which is in Southeast Asia, they basically say, okay, the government 00:23:20.800 |
has decided that this many masks have to be given to hospitals, so they've allocated them. 00:23:27.120 |
And for whatever reason, we're not doing that in the West, on the whole. 00:23:31.480 |
And also in China, they bought 38 million masks right at the start of this. 00:23:37.800 |
The US is not investing in increasing capacity. 00:23:41.680 |
So when you're not doing those things, it can seem like the right response is to tell 00:23:47.200 |
people not to buy them, tell people they don't need them, but probably not a good long-term 00:23:55.040 |
So as I say, that the trust situation is such that doctors are saying they're learning more 00:24:03.720 |
from Facebook and Twitter than peer-reviewed and even pre-pub medical research literature 00:24:10.040 |
at the moment, which is, it's not a great situation, but let's accept that this is what 00:24:14.960 |
it is, right? And it partly is because there's a great community response, you know, of doctors 00:24:21.400 |
and virologists and epidemiologists saying like, okay, I don't have time to write a paper, 00:24:27.040 |
but let me tell you, I just went to this place, I did this study, I found this thing, I talked 00:24:30.640 |
to this person, you know, it's fast-moving information, you know, for, but we do have 00:24:38.000 |
information about masks, not for COVID-19, but for other things that involve coughing 00:24:44.040 |
and droplets moving around, so there is some research, not perfect, but can tell us a bit 00:24:52.760 |
about what kind of masks work. And interestingly, this particular example showed that just simple 00:24:57.840 |
surgical masks were just as useful as N95 masks for influenza virus. Virologists are 00:25:06.640 |
telling us exposure dose matters and therefore masks help. And because when you have a mask 00:25:15.640 |
it means, you know, less of those droplets end up in your nose and mouth and if that 00:25:19.920 |
happens it keeps the peak viral load lower and so it does less damage, the immune system 00:25:24.480 |
starts responding earlier and can flatten the curve. So, you know, there's a big difference 00:25:29.640 |
between, at least in the West, the official information that's generally being passed 00:25:34.880 |
on and the information that we can see in research papers and from, from experts. Having 00:25:41.400 |
said all that, it's certainly true that there isn't enough masks for doctors. And so one 00:25:46.800 |
of the things, you know, if people can find ways to acquire more masks, create more masks, 00:25:55.120 |
figure out better ways to reuse masks, I don't know, like this is something everybody is 00:25:58.760 |
trying to figure out right now because it could make a big difference, particularly 00:26:02.920 |
because it's becoming increasingly clear that people who are infected, so most people who 00:26:11.040 |
are infected don't have symptoms. But the problem is that it's, it's seeming pretty 00:26:15.560 |
clear that those people are spreading the disease. So again, there's a lot of official 00:26:19.480 |
advice saying, you know, if you've got symptoms, stay home, if you've got symptoms, self isolate, 00:26:26.640 |
so forth. But the kind of the data we currently have does not show that to make sense. It 00:26:34.360 |
seems actually like regardless of whether you have symptoms, if you have the virus, 00:26:40.480 |
you need to be not spreading it. So that means again, more testing, more masks, more self 00:26:47.600 |
isolation, particularly if we have testing like super wide testing. Another thing I wanted 00:26:55.680 |
to talk about was young people. People under 50 probably won't die. Some do, the kinds 00:27:05.360 |
of people who might, you probably know if it's you because they're kind of immunocompromised 00:27:09.880 |
people and so forth. But you know, let's, let's be honest, you probably won't die if 00:27:15.040 |
you're under 50. You might pass it on to your grandparents or parents and kill them, or 00:27:21.680 |
your colleagues at work. But the other thing to mention is these kinds of diseases have 00:27:28.160 |
tend to shown long term impacts for people when they get older. So less lung function, 00:27:37.080 |
neurological symptoms, depression, you know, there's tended to be for this kind of class 00:27:43.040 |
of viruses, there's tended to be pretty debilitating lifetime impacts even for young people. So 00:27:53.360 |
you know, if it's not enough to say, hey, stay home so that you don't kill your community 00:27:57.960 |
and family, then you know, maybe okay, we'll stay home so that you don't make your rest 00:28:03.680 |
of your life much crappier than it otherwise would have been. 00:28:08.720 |
I was also just going to add that you probably have more coworkers and acquaintances who 00:28:13.080 |
have chronic illnesses that you're not aware of, because many people don't share this information 00:28:17.040 |
out of a very reasonable fear of discrimination. 00:28:20.200 |
Okay, there is something which is terrifyingly complicated, which is in the 1918 flu, the 00:28:29.720 |
second and third seasons were much worse than the first. That's what a lot of people are 00:28:35.080 |
worried might happen. I don't think anybody has a good idea really of what to do about 00:28:40.080 |
this. It was because of this that the UK did the original disastrous response of, let's 00:28:51.600 |
not close down events, let's not close down schools, and now there as we saw there in 00:28:56.600 |
a lot of trouble. And indeed that same group, Imperial College, is now saying in the U.S. 00:29:06.360 |
there's a chance that if you isolate cases and household quarantine and social distancing, 00:29:12.280 |
we might be able to, for the initial period, we might be able to keep things under the 00:29:18.080 |
red line. This is a super concerning, dangerous kind of approach, because if we don't close 00:29:31.840 |
schools and universities and we don't calculate the exponentials right and nobody actually 00:29:36.880 |
knows, then we won't find out that we failed to get this right until we're well up along 00:29:43.760 |
kind of the black line, you know, particularly because we can't really do case isolation 00:29:49.120 |
because we don't have testing capacity. So this kind of modeling that's being done to 00:29:53.120 |
say like maybe we don't have to do too much right now is not based on reality of what 00:29:59.920 |
we can do, and it's also kind of assuming data we don't have. And most importantly from 00:30:04.640 |
a Bayesian point of view, we don't have confidence intervals here. So if you actually do this 00:30:09.400 |
modeling with confidence intervals, you'll realize that kind of probability that each 00:30:13.680 |
of these things is successful, the number of cases that we actually find through testing, 00:30:18.780 |
the bounds we have on our understanding of what the death rate is and so forth, basically 00:30:24.000 |
mean that the kind of confidence interval of this brown and green line kind of go from 00:30:29.000 |
down here to way up here. And of course the utility of each direction varies a lot. So 00:30:37.680 |
I kind of just wanted to mention that when you look at this kind of modeling, I haven't 00:30:44.000 |
seen any done yet with that kind of full confidence interval approach and considering the utility 00:30:53.040 |
of each of the possibilities. And so I'd say be very, very careful of this and that's what 00:30:58.320 |
the UK got so very wrong so far. Finally, I wanted to talk about stuff that you can do. 00:31:07.240 |
One thing you can do is not stress about the supply chain. There's plenty of food. There's 00:31:12.720 |
plenty of toilet paper. The supply chain is not threatened. And by the way, I'm using a 00:31:20.880 |
lot of slides here from other people without credit, which I normally wouldn't do, but 00:31:25.760 |
I wanted to put together something that's very up-to-date and very quick and I apologize 00:31:31.120 |
but I'm trying to put people's names at least including them where they were already on 00:31:35.960 |
the slide. So this is from Michael Lin. So over the next few weeks as people settle down, 00:31:46.080 |
you should see your shops. I mean, I'm still already starting to see shops starting to 00:31:51.120 |
see goods again. This is an opportunity to take advantage of some casual racism. You'll 00:31:57.080 |
find that Chinese Asian grocery shops are absolutely stacked right now. So Rachel and 00:32:02.880 |
I went shopping at one the other day and got everything that we wanted straight away. So 00:32:07.920 |
yeah, don't worry about that. It is hard to find hand sanitizer, which is super important. 00:32:16.520 |
If you can find something with at least 60 or 70 percent, sorry, with something with 00:32:20.400 |
at least 90 percent alcohol, you can mix it up with glycerin or aloe vera and create your 00:32:24.600 |
own. There's actually an official WHO sanitizer recipe. Here's another one. It is a bit difficult 00:32:35.640 |
to kind of handle things properly without hand sanitizer. I did post on Twitter a list 00:32:41.760 |
of Singapore's official list of chemicals which kill the virus that you can find in 00:32:48.060 |
basically household cleaners. And I found we had like five household cleaners at home 00:32:52.160 |
of which four turned out to have the ingredients recommended. So you might be surprised at 00:32:57.720 |
how many things you can kind of use. The tricky thing here is thinking about how to stay safe 00:33:09.240 |
is Rachel and I kind of play this game. I think of this computer, think of it like a 00:33:14.800 |
computer game. We call it the Code Red game, where somebody else has sat at a table and 00:33:20.440 |
they were infected with COVID-19. And so you then sit at that table. So that table, we 00:33:27.400 |
would say it's red, right? And you have to assume that everything that you haven't personally 00:33:33.320 |
cleaned thoroughly is red because you don't know if it is or it isn't, right? So that's 00:33:38.720 |
your red. So you have to assume that's red. So you go to a table at a restaurant, you 00:33:42.760 |
sit down and your hand touches it. So now think of a computer game where like every 00:33:47.240 |
time something touches a red thing, it becomes red as well. So your hand touches the table, 00:33:51.960 |
it's now red, by which I mean potentially infected. You pick up your phone, bing, that's 00:33:59.080 |
now red. You take the phone home, you put it on your desk, bing, that's now red. You 00:34:05.360 |
pick it up off the desk and then you put your computer where it was sitting, bing, that's 00:34:09.240 |
now red. Right? So that's kind of like how you have to think about this. And so the thing 00:34:13.120 |
is now you then say like, oh, forgot to wash my hands after I went out to the restaurant. 00:34:20.440 |
So you clean them and hands are now green. But this kind of now whole chain of things 00:34:26.160 |
which touch things, which touch things, which touch things, they're all red. Right? So this 00:34:30.040 |
is one of the reasons that kind of everything's taking us a long time at the moment to just 00:34:35.600 |
get around the place is we have to make sure that everything red becomes green in a way 00:34:44.160 |
that we don't make other things red in the process. And you know, sometimes we forget 00:34:48.200 |
and then we realize there's a whole chain of things that happened and then we have to 00:34:51.040 |
go and do all of those over again. But if you get good at this, then you can kind of 00:34:57.720 |
like, as long as you stay distance from people, you can kind of go about your day to day life, 00:35:02.360 |
you know, you can go and get takeout or whatever. But for this to work, you need hand sanitizer. 00:35:09.920 |
It helps to have proper, what are they called? Night trial gloves. Night trial gloves. And 00:35:18.600 |
of course, you know, cleaning fluids that you can just spray on a piece of paper on 00:35:22.400 |
a piece of, you know, cleaning paper and wipe things down. So like when we get shopping, 00:35:29.720 |
we just pop it on the front deck, wipe down everything with alcohol or bleach before we 00:35:35.600 |
bring it in and so forth. So it's a hassle, but you know, it's, you can totally handle 00:35:44.640 |
the situation this way. There's a lot of opportunities for data scientists to help. People have been 00:35:51.840 |
reaching out to me and so this gentleman said like, hey Lombardi is, I mean Lombardi's really 00:35:59.080 |
the place is nearly struggling the most. We founded a nonprofit group of data scientists 00:36:03.080 |
where we're trying to help. So you can go to defeat covid19.org to find out what kind 00:36:09.760 |
of help they need. There are ways to deal with, you know, a little bit some of the lack 00:36:16.680 |
of testing to at least figure out a bit of what's going on, for example, by, so if you 00:36:23.040 |
don't follow Eric Fagel-Ding, he has lots of great information about this. Folks like 00:36:29.840 |
him have been looking at what's the kind of reporting of flu like symptoms based on just 00:36:35.720 |
the regular reporting that we have coming out of hospitals and comparing that to the 00:36:40.320 |
amount of flu diagnoses. And you can kind of get creative like that to figure out like, 00:36:45.720 |
oh maybe the difference is because of covid19. And the bad news is based on that kind of 00:36:51.320 |
back of the envelope analysis, things look much worse than the official figures. Pete 00:36:56.760 |
Skomrock kind of had the idea, you know, as other people have had similar ideas, hey maybe 00:37:01.480 |
things like the Google business system that tells you when it's busy or not could be kind 00:37:06.920 |
of reused to try and help do some kind of pandemic social distance measurement stuff. 00:37:14.280 |
This kind of stuff we've got to be super careful of creating some kind of dystopian surveillance 00:37:19.040 |
society that we then can't untangle again at the end. But you know there's opportunities 00:37:25.120 |
for data scientists and kind of software developers to think creatively about ways to help fill 00:37:31.560 |
in these gaps, fill in the gaps around testing, around keeping data available. 00:37:48.720 |
So one of the things to think about is how can we help improve testing. The data that's 00:37:55.920 |
available is suggesting that, so this is from science, that the vast majority of infections 00:38:02.020 |
were undocumented. And that's led folks like Jeff Dean to ask what's been the economic 00:38:08.760 |
cost of delaying testing in the US. I reckon it might be hundreds of billions or trillions 00:38:13.240 |
of dollars. You can see the difference between South Korea and United States that both saw 00:38:18.000 |
their first cases within a day of each other. South Korea tested massively. United States 00:38:25.800 |
just starting to test super late. So you know if you can help us find ways to do more tests 00:38:32.960 |
that would be super helpful. Also I mean you know we're data scientists but there's people 00:38:43.600 |
with all kinds of backgrounds here. If you've got a background in hardware or 3D printing 00:38:47.720 |
people are saving lives here. These folks bought a 3D printer to a hospital in Italy 00:38:53.360 |
and there are people now breathing who previously couldn't thanks to that 3D printing. The world 00:39:01.920 |
being what it is these people have now been sued by a patent troll which I guess was probably 00:39:06.480 |
predictable but yeah. I guess a lot of people are trying to get creative and sometimes working 00:39:12.760 |
around regulations as necessary to save lives which hopefully will turn out to be a good 00:39:19.680 |
thing not a bad thing. Ventilators is one thing a lot of people are trying to build 00:39:24.800 |
at the moment so maybe you can help. So the director of the Johns Hopkins Center for Health 00:39:32.960 |
Security is saying we need a wartime mobilization to make mass number of ventilators and to 00:39:39.560 |
get enough oxygen. So kind of wartime mobilization means a lot of creativity and a lot of you 00:39:49.000 |
know not being quite as precise about things as we might have used to have been but just 00:39:55.120 |
trying things out and seeing what works. Mind you the first thing we could do in the US 00:40:01.000 |
is just buy the capacity we already have. There's actually an opportunity to ramp up 00:40:05.560 |
production fivefold right now but the US just hasn't actually ordered the product. So make 00:40:11.440 |
of that what you will. Some of these folks reached out to me and asked for help finding 00:40:17.440 |
people to help with open source medical supplies. They're sharing CAD files and there's now 00:40:23.800 |
been responses all around the world to that and that's going pretty well. Okay so that 00:40:35.880 |
is my little spiel about COVID-19. Rachel is there anything you wanted to add? I was 00:40:45.720 |
planning to yeah. Okay so for those of you that aren't interested in that topic I apologize 00:40:52.160 |
for taking up your time hopefully some of you found that useful. For those of you that 00:40:57.480 |
did and are interested in contributing I have created a forum category for this. Here it 00:41:10.200 |
is COVID-19. Now I think there's a lot of opportunities to matter I wouldn't say I think 00:41:17.440 |
maybe there's opportunities to apply some of the stuff we're learning in this course 00:41:21.320 |
to do projects that are related to COVID-19. It would be nice wouldn't it to spend these 00:41:26.680 |
two months learning a new thing but also meeting other people working together on something 00:41:32.080 |
that seems important. So if you're interested in doing that this is the place to put it 00:41:40.440 |
because it's not a closed category like our course category is. If you do of course don't 00:41:45.840 |
like say hey I've just learned this thing in the course to people who aren't in the 00:41:50.400 |
course because they're going to feel jealous or whatever. So just remember you're talking 00:41:54.400 |
to folks who aren't necessarily part of the course but yeah this is a great place to help 00:42:02.620 |
write screen scrapers to put data sets together or set up automation of things or you know 00:42:10.520 |
do a survival analysis that incorporates uncertainty in the way that I don't think I've seen done 00:42:15.360 |
yet or you know so on and so forth. So hopefully that turns out to be useful to some people. 00:42:29.240 |
So I'm back again and I just had a quick huddle with Rachel and we decided to put this thing 00:42:34.080 |
online publicly not just for our course. So I figured I'd better just pop in again to 00:42:41.000 |
folks that aren't part of the course and are watching this to fill in some gaps here. When 00:42:48.720 |
I was talking about the forums I'm talking about this website here forums.fast.ai we normally 00:42:58.120 |
use it to talk about deep learning not to talk about COVID-19 but we can talk about 00:43:03.720 |
COVID-19 as well we've got a category for it there. If you're somebody who's not a data 00:43:09.920 |
scientist but is interested in having a conversation about COVID-19 particularly if it's something 00:43:14.480 |
that's more on the technical side or data driven or practical which is kind of our things. 00:43:22.840 |
We would love to hear from you even if it's not a data science perspective if you're a 00:43:26.560 |
3D printing person if you're a chemist who knows good ways to create oxygen if you're 00:43:31.640 |
somebody who knows a good source of reagents for testing whatever we would love to hear 00:43:39.600 |
from you there. So thanks very much for listening to this extremely non-deep learning related 00:43:46.440 |
video about COVID-19 and I guess the other thing to mention is you may have no idea who 00:43:53.760 |
I am. I'm Jeremy Howard. This is my Twitter @jeremyphoward and Rachel is @mathratial_ratial. 00:44:06.480 |
Don't forget the P in Jeremy P Howard. I'm going to be pretty busy trying to create this 00:44:12.360 |
deep learning course over the next few days so I don't promise I'll be putting much on 00:44:16.200 |
Twitter but I will do my best to share things which I think are of interest so thanks a