back to indexProgramming Meme Review with George Hotz
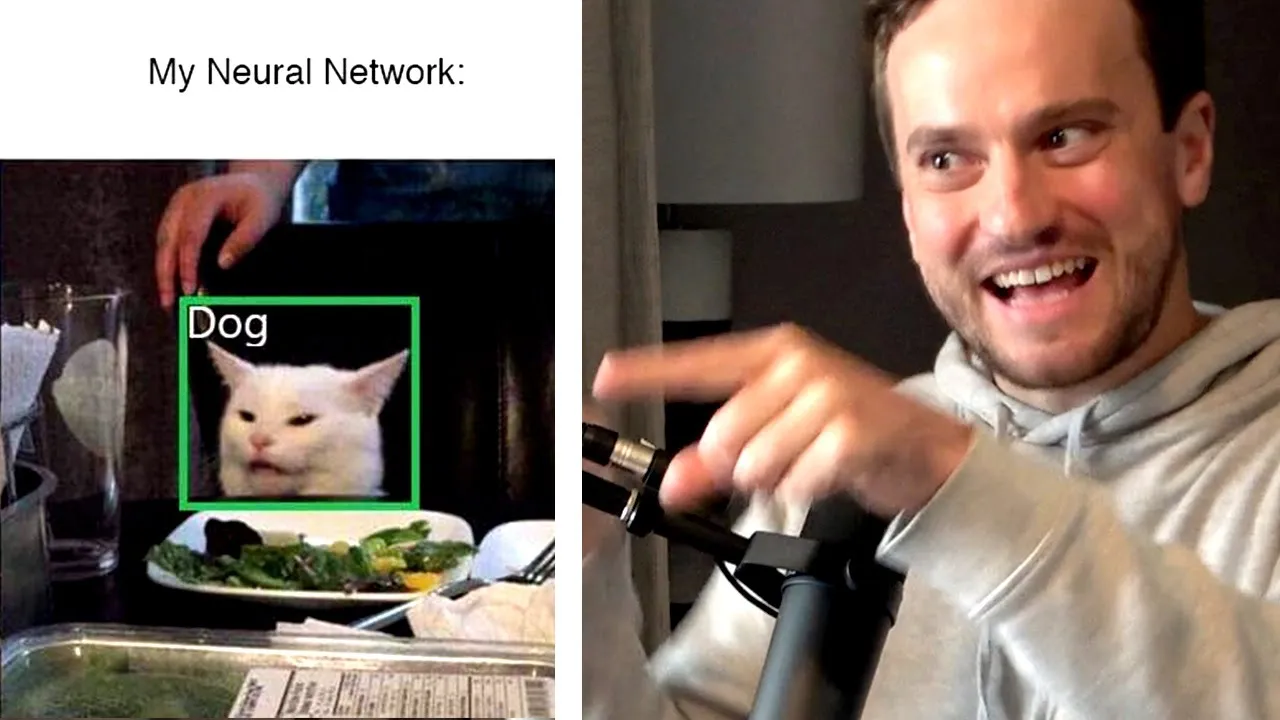
00:00:00.000 |
This is a review of programming memes with George Hotz. 00:00:06.200 |
Four Sigmatic, the maker of delicious mushroom coffee 00:00:12.940 |
for minimalist household products and basic healthy food. 00:00:16.880 |
Please check out these sponsors in the description 00:00:19.240 |
to get a discount and to support this channel. 00:00:26.040 |
Let me ask you to do a meme review or inspect 00:00:33.800 |
and maybe give a rating of pass or fail, like binary, 00:00:40.840 |
These are mostly from the programming subreddit. 00:00:47.360 |
- I mean, you were like, I could do it straight up 00:01:18.040 |
- No, I think, like, now this one, this meme's much better. 00:01:23.080 |
- You can spend six minutes doing something by hand 00:01:25.120 |
when you can spend six hours failing to automate it. 00:01:38.360 |
I mean, but you learn so much trying to automate. 00:01:40.320 |
You know, you could spend six minutes just driving there 00:01:42.280 |
instead of spending, you know, 10 years of your life 00:01:50.240 |
- Of course, you know, it's like you're trying 00:01:54.080 |
to overcoming laziness than just overcoming it. 00:02:03.400 |
This just, yeah, this is why I trust programmers 00:02:12.480 |
- Doctors think they have some, like, divine wisdom 00:02:16.120 |
because they went to med school for a few years. 00:02:26.100 |
I remember once when I was, let's say, drug shopping 00:02:40.360 |
But, you know, like, doctor, you don't know that? 00:02:45.040 |
and you don't know about the drug scheduling system? 00:02:54.080 |
If you outsource the entirety of your knowledge 00:03:09.000 |
- Well, the integral of E to the X is E to the X. 00:03:39.480 |
We have, our regression testing's gotten a ton better 00:03:43.840 |
Now we're pretty confident, if the tests pass, 00:03:46.640 |
that at least it's gonna go into someone's car 00:03:52.120 |
Versus in the past, like sometimes we'd merge stuff in 00:03:54.040 |
and then one of the processes wouldn't start. 00:03:57.280 |
is still really useful for Autonomous Vehicles? 00:04:08.480 |
- Yeah, that it runs, and that it runs in CI, 00:04:13.960 |
called Process Replay, which just runs the processes 00:04:16.520 |
and checks that they give the exact same outputs. 00:04:23.040 |
Well, yeah, then we update the process replay hash. 00:04:25.280 |
This prevents you from, if you're doing something 00:04:27.520 |
that you claim is a refactor, this will pretty much, 00:04:31.520 |
it will check that it gives the exact same output 00:04:34.760 |
And we run that over like 60 minutes of driving. 00:04:37.600 |
- But that one's a win for you, you get past the 10, 00:04:54.640 |
this is why I don't use IDEs, that's just true. 00:04:58.800 |
- But I gotta say, I mean, to me, it's kind of funny, 00:05:01.280 |
'cause it's so annoying, it is so annoying with IDEs. 00:05:11.400 |
I always still wanna, this is a company idea I wanna do, 00:05:18.200 |
You can train these big language models on Python, 00:05:23.920 |
- So you hope for that, like machine learning approaches 00:05:30.040 |
- Yeah, I think machine learning approaches for improved, 00:05:43.400 |
of GPT-3 language model that might be kind of cool. 00:05:47.360 |
- I think that the idea that you're gonna somehow 00:05:48.720 |
get GPT-3s to replace customer service agents, 00:05:53.440 |
on customer service agents, and why don't you just 00:06:17.600 |
- No, I'd rather you mine cryptocurrency in my browser 00:06:29.640 |
The only thing I do want to autoplay is like YouTube. 00:06:32.160 |
- Why do news sites start autoplaying their news? 00:06:43.160 |
I still hear about the news 'cause people talk about it. 00:06:51.880 |
And in fact, even on Hacker News, I'll always avoid it 00:07:04.840 |
That's at the core why journalism is broken, actually, 00:07:07.120 |
just 'cause like the revenue model is broken, 00:07:14.400 |
I want to pay the New York Times and the Wall Street Journal. 00:07:16.880 |
I want to pay them money, but like they make it 00:07:20.560 |
like to where I have to click like so many times 00:07:28.480 |
- Yes, and also they're doing the gym membership thing 00:07:37.520 |
Like just be up front and let it be five bucks. 00:07:42.960 |
which is like just make it cheap, accessible to everyone. 00:07:55.160 |
is ad block that also blocks all the recommendeds. 00:08:02.400 |
These trending topics have zero relevance in my life. 00:08:08.880 |
- You know, one interesting thing I've used on Twitter, 00:08:12.120 |
I had to turn it back on 'cause it was tough, 00:08:14.600 |
is there's Chrome extensions people could try, 00:08:23.680 |
It's such a different, people should definitely try this. 00:08:27.560 |
'Cause I've realized that I judge the quality 00:08:33.440 |
Like it's not, you know, basically if it has no likes, 00:08:42.720 |
And when you turn those numbers off, I'm lost. 00:08:45.720 |
At first I'm like, what am I supposed to think? 00:08:49.960 |
- That's right, but then how much is it manipulated? 00:08:54.000 |
But it's an interesting experience, worth thinking about. 00:09:01.060 |
Anything that's global and applicable to everybody, 00:09:07.480 |
It's always some like political agitprop or like some, 00:09:13.200 |
- But at the same time, like to push back slightly, 00:09:15.680 |
for me, trending, like if there's a nuclear war 00:09:20.880 |
- That's how they get you, that's how they get you. 00:09:22.600 |
And I just trust that if nuclear war breaks out, 00:09:36.720 |
but I realized that they do provide value to me. 00:09:42.680 |
- As opposed to a general one, like a trending one, yeah. 00:09:48.200 |
So this one is about how Stack Overflow can be really rough. 00:09:51.000 |
Asking a question on Stack Overflow, be like. 00:09:55.960 |
No, I've never asked a question on Stack Overflow. 00:10:06.840 |
- Well, I mean, there is some truth to that too. 00:10:14.800 |
is it's Eric Raymond and it's how to ask smart questions. 00:10:21.200 |
the quality of the answer you get depends a lot 00:10:25.160 |
To be fair, I think, yeah, the auto keyword is not, 00:10:45.160 |
- I do this in interviews, I ask ambiguous questions. 00:10:47.640 |
And I just see how, I found that that's so much more useful 00:11:00.880 |
- What do you, by the way, just as a quick aside, 00:11:03.840 |
is there any magic formula to a good candidate? 00:11:12.060 |
And you can see it through just conversation? 00:11:16.500 |
I always wish there was a test that you could just online, 00:11:23.360 |
testing for intelligence is easy because, okay, 00:11:27.080 |
but dumb people can't really lie and say they're smart. 00:11:37.880 |
to come work on something real in our code base. 00:11:43.240 |
are you just doing this as like a job interview? 00:11:53.300 |
and see like if there's a, is there a fire there like that? 00:11:56.960 |
And we see, and like now we hire a lot of people, 00:12:03.960 |
you're kind of expected to have read the open pilot code. 00:12:06.880 |
All right, like the best candidates are the ones 00:12:08.320 |
who like read the code and ask questions about it. 00:12:12.200 |
The beauty of Kama is you don't need to be hired by us 00:12:24.260 |
- Yeah, are you obsessed about resource constraints? 00:12:27.480 |
- Some, not size, I don't care about bytes, but CPU. 00:12:39.860 |
People complain about the Kama 2's overheating. 00:12:51.900 |
- Awesome, that's, yeah, that's one way to go. 00:13:01.100 |
- My code doesn't work, let's change nothing. 00:13:03.860 |
- That's the weird thing, and it often works. 00:13:09.980 |
I don't get how this world, this universe is programmed, 00:13:14.060 |
Just like restarting a computer, restarting the system. 00:13:16.820 |
Like this audio setup, sometimes it's just like not working, 00:13:20.700 |
and then I'll just shut it off and turn it back on, 00:13:27.020 |
You interpreted it as in like, this is a good idea. 00:13:30.700 |
I interpreted it as in, I find myself doing this all the time. 00:13:37.260 |
and I'm not gonna be happy with it, but like, you know. 00:13:39.500 |
I found this, I did Advent of Code last December. 00:13:53.540 |
Yeah, I found myself doing that all the time. 00:14:04.140 |
You're able to, I mean, at least I've seen you 00:14:14.100 |
I don't know, some of them, like people don't realize 00:14:19.980 |
To be fair, I did write that slam from scratch on there. 00:14:24.220 |
but I also spent like the previous six months studying Slam. 00:14:31.860 |
- Going into it, whereas like with these other things, 00:14:36.700 |
And people are like, why isn't it like Twitch Slam? 00:14:39.960 |
- So this one is just, you find this to be true? 00:15:05.340 |
I get this, I mean, sometimes this meme pisses me off 00:15:13.500 |
and they're struggling with like object detection, 00:15:17.420 |
and they think how is this supposed to achieve 00:15:20.920 |
- That's 'cause you're just doing a first intro 00:15:26.680 |
when you scale things, I mean, you can achieve 00:15:29.240 |
incredible things that would be very surprising. 00:15:32.680 |
- I mean, it's the same thing with software 1.0. 00:15:34.520 |
You know, they sit there and they write "Hello World," 00:15:37.040 |
and they're like, how is this thing supposed to fly rockets? 00:15:42.360 |
- Just takes a whole lot more effort than you think. 00:16:05.060 |
what's the complexity of matrix multiplication? 00:16:07.420 |
And I was explaining this, like why fundamentally 00:16:12.660 |
this is true, and then I went a little further with it. 00:16:14.220 |
I'm like, you know, neural networks is just like 00:16:16.180 |
matrix multiplications, interspersed with nonlinearities. 00:16:21.600 |
the fact that that's such an expressive thing, 00:16:25.300 |
and it's also differentiable, it's also quasi-convex, 00:16:27.580 |
like it's convex-y, which means convex optimizers 00:16:30.140 |
work on it, like, yeah, well this isn't going anywhere. 00:16:39.620 |
- I think that we haven't seen the loss function yet. 00:16:47.820 |
And I haven't seen these things really applied. 00:16:51.140 |
Maybe there was that one paper where they tried, 00:16:55.520 |
- Yeah, there's a lot of fun stuff in RL that