back to indexQ* - Clues to the Puzzle?
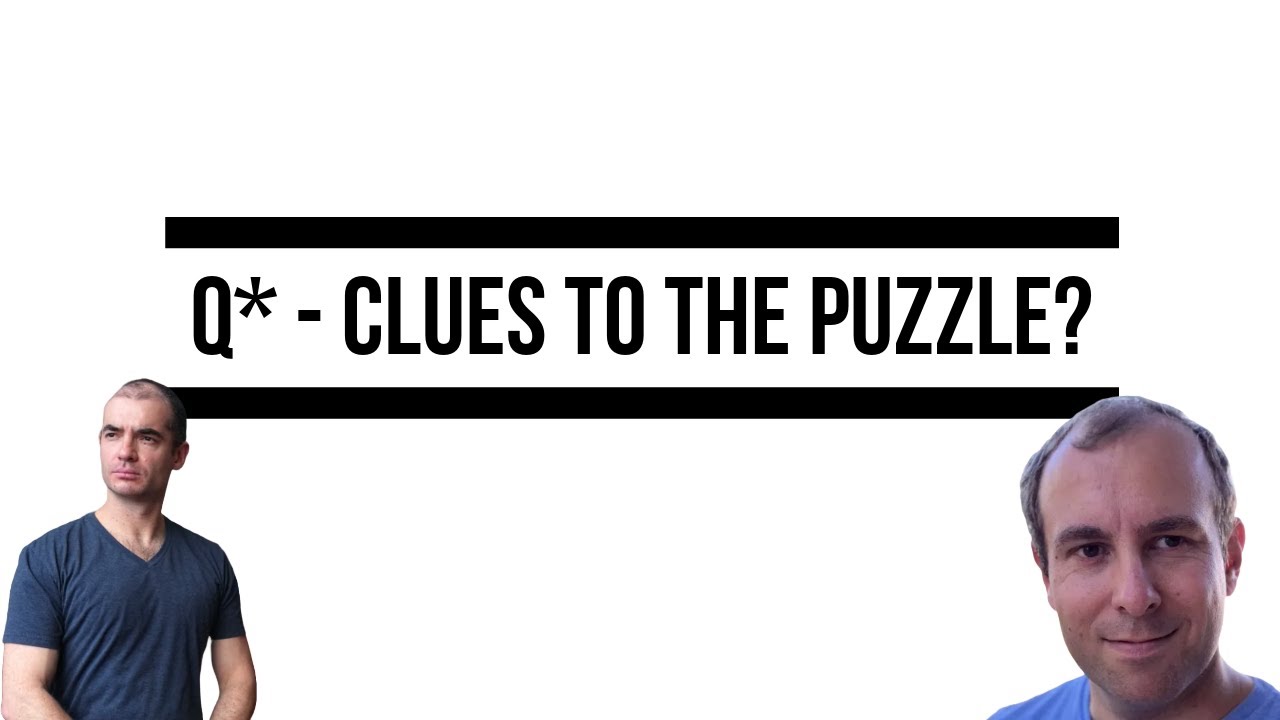
00:00:00.000 |
As you might expect, I have been researching nonstop about this apparent powerful AI discovery 00:00:06.200 |
that insiders at OpenAI said could threaten humanity. 00:00:10.000 |
I've spoken to every insider I know and done a ton of research and I am not claiming to 00:00:16.800 |
But I can provide some genuine clues that I think will be at least part of the answer. 00:00:22.600 |
Normally, I like to be a lot firmer than that, but this is the best I can do. 00:00:26.880 |
Now the first thing to note of course is that OpenAI have now denied that Sam Altman's 00:00:31.800 |
Alsthur was precipitated by this safety letter to the board. 00:00:35.440 |
As my previous three videos have shown, there was certainly a lot else going on, so it might 00:00:44.000 |
Second, I just want to quickly debunk this clip that's doing the rounds where people 00:00:48.440 |
are claiming that Sam Altman called this new creation a creature, not just a tool. 00:00:52.960 |
Actually, if you watch to the end, he's very much saying he's glad that people now think 00:00:58.960 |
So despite this frantic headline, I am not trying to overhype things, but I genuinely 00:01:06.600 |
Later in the article, these insiders, these researchers flagged up work by an AI scientist 00:01:11.760 |
team, the existence of which multiple sources confirmed. 00:01:14.800 |
This AI scientist team was formed by combining earlier co-gen and math gen teams at OpenAI. 00:01:21.720 |
Their work on exploring how to optimize existing AI models to improve their reasoning was flagged 00:01:28.120 |
Now there is very little public information about either the co-gen or math gen teams, 00:01:33.520 |
but I dredged up this old tweet from Sam Altman. 00:01:39.040 |
Anyway, Sam Altman said, "Really exciting process supervision result from our math gen 00:01:43.640 |
team," and he linked to a critical paper that I covered back in the spring. 00:01:52.960 |
That's what I think the former math gen, now AI scientist team were working on. 00:01:58.100 |
And take this tweet in September by Noam Brown at OpenAI. 00:02:01.720 |
"My teammates and I at OpenAI are hiring ML engineers for research on LLM multi-step 00:02:08.280 |
We recently hit a state of the art 78% on the math benchmark," which I'll get to in 00:02:12.120 |
a second, "Our new plans are even more ambitious. 00:02:15.520 |
I'm only just getting started, but you might already be glimpsing why I think this paper 00:02:27.880 |
That's apparently what they've achieved behind the scenes, at least according to Reuters. 00:02:33.160 |
Well, we have this exclusive report from the information. 00:02:36.280 |
Again, similar frantic headline, "OpenAI made an AI breakthrough before Altman firing, 00:02:44.240 |
The technical breakthrough, they say, was spearheaded by OpenAI chief scientist, Ilya 00:02:50.040 |
And the most recent paper on Archive listing Ilya Sutskov as an author is the "Let's 00:02:56.480 |
Now at this point, I know you want me to get to what it means, but with so many theories 00:03:00.720 |
floating out there, I want to give you yet more evidence that at least one of the breakthroughs 00:03:09.000 |
And I think I might even know what the other breakthrough is. 00:03:11.780 |
The same article in the information talks about Sutskov working on ways to allow language 00:03:16.560 |
models to solve tasks that involve reasoning, like math or science problems. 00:03:21.100 |
It talks about how he had this secret program called GPT-0. 00:03:26.400 |
The team hypothesized that giving language models more time and computing power to generate 00:03:31.480 |
responses to questions could allow them to develop new academic breakthroughs. 00:03:35.960 |
And Lucas Kaiser, who we will definitely be seeing more of in this video, indeed he appears 00:03:40.640 |
in the thumbnail, apparently held a key role in the GPT-0 project. 00:03:45.600 |
And look at this, among the techniques the team experimented with was an ML concept known 00:03:49.800 |
as "test-time computation" that's apparently meant to boost language models' problem-solving 00:03:55.880 |
And we have a hundred speculations online about what this might mean. 00:03:59.440 |
But I knew that name rang a bell and I dug up this 2021 paper which cites test-time compute. 00:04:06.620 |
And what is this random paper that Philip is talking about? 00:04:09.400 |
Well, it's from OpenAI and look at some of the co-authors, Carl Cobb of the MathGen 00:04:14.680 |
team and Lucas Kaiser cited in the information. 00:04:18.600 |
This was actually one of the precursor papers to Let's Verify Step-by-Step, which I will 00:04:24.920 |
It introduced the now famous in ML circles GSM 8K dataset. 00:04:33.120 |
More importantly though, it trialed this method of at test time, generating many candidates' 00:04:38.320 |
solutions and selecting the one ranked highest by a verifier. 00:04:43.120 |
And I'm going to massively oversimplify at this point and just say that a verifier 00:04:47.480 |
is a separate model trained only at this point in this paper to spot good solutions, solutions 00:04:55.120 |
And what the paper proposed was getting the base LLM to generate hundreds of solutions 00:05:00.400 |
and then getting this separate verifier to spot the ones that were likely the most correct. 00:05:05.400 |
And in a nutshell, the authors noticed that if they invested more computing power in generating 00:05:09.840 |
more solutions and taking a majority vote among the top verifier ranked solutions, that 00:05:19.280 |
And that's what it means by test time compute, investing your computing power while you're 00:05:27.480 |
You're not further training it or fine tuning it. 00:05:29.680 |
You're investing that computing power during test time, again, to generate potential solutions 00:05:35.200 |
and take majority votes amongst them, self-consistency. 00:05:38.120 |
They found that using a verifier in this way was better than fine tuning because verification 00:05:46.560 |
It's easier to check your answers than generate good ones. 00:05:49.680 |
So investing time in checking answers was more fruitful. 00:05:53.880 |
Well, the use of verifiers results in approximately the same performance boost as a 30 times model 00:06:00.660 |
And then it gets prophetic when it says, and verifiers scale significantly better with 00:06:06.640 |
And to hammer the point home, they said a 6 billion parameter model with verification 00:06:10.920 |
slightly outperforms a fine tuned 175 billion parameter model. 00:06:16.360 |
Again, thereby offering a boost approximately equivalent to a 30X model size increase. 00:06:21.520 |
And that team, by the way, with Lucas Kaiser was probably drawing on work by a single dude 00:06:30.920 |
While studying the board game Hex, the researcher, Andy Jones found an interesting result. 00:06:35.480 |
He said, along with our main result, we further show that the test time and train time compute 00:06:40.120 |
available to an agent can be traded off as we've seen while maintaining performance. 00:06:44.600 |
I read that paper in full and it was cited by no other than Noam Brown. 00:06:49.560 |
He's the guy we saw earlier who joined OpenAI in July of this year. 00:06:53.720 |
He said he's investigating how to make reasoning methods truly general. 00:06:57.720 |
If successful, we may one day see LLMs that are a thousand times better than GPT-4. 00:07:03.680 |
And later in that thread, he cites that same paper from Andy Jones. 00:07:07.360 |
And he concludes with this, all those prior methods are specific to the games they're 00:07:12.000 |
But if we can discover a general version, the benefits could be huge. 00:07:15.160 |
Yes, inference may be a thousand X slower and more costly, but what inference cost would 00:07:20.200 |
we pay for a new cancer drug or for a proof of the famous mathematical Riemann hypothesis? 00:07:25.720 |
And we'll come back to mathematical hypotheses in a moment. 00:07:28.480 |
Anyway, improved capabilities are always risky, he went on. 00:07:31.440 |
But if this research succeeds, it could be valuable for safety research as well. 00:07:35.880 |
Notice that point about capabilities being risky. 00:07:38.560 |
It does seem to me to be linking together into that Reuters story. 00:07:42.200 |
Imagine he says being able to spend $1 million on inference to see what a more capable future 00:07:48.400 |
It would give us a warning that we would otherwise lack. 00:07:51.840 |
Is that what OpenAI did when they recently pushed back the veil of ignorance? 00:07:56.520 |
Did they just spend a million dollars on inference and see what a more capable future model might 00:08:03.000 |
So that's test time compute, but what about let's verify step by step? 00:08:06.520 |
Well going back to that original 2021 verifier paper, they said this. 00:08:10.480 |
The problem that they noticed with their approach back in 2021 was that their models were rewarding 00:08:15.400 |
correct solutions, but sometimes there would be false positives. 00:08:19.240 |
Getting to the correct final answer using flawed reasoning. 00:08:22.520 |
They knew this was a problem and so they worked on it. 00:08:25.140 |
And then in May of this year, they came out with let's verify step by step. 00:08:30.040 |
In this paper, by getting a verifier or reward model to focus on the process, the P, instead 00:08:36.160 |
of the outcome, the O, results were far more dramatic. 00:08:40.160 |
Next, notice how the graph is continuing to rise. 00:08:43.700 |
If they just had more, let's say test time compute, this could continue rising higher. 00:08:49.640 |
And I actually speculated on that back on June the 1st. 00:08:53.240 |
That difference of about 10% is more than half of the difference between GPT-3 and GPT-4. 00:08:59.500 |
And also, is it me or is that line continuing to grow? 00:09:02.980 |
Suggesting that when more compute is available, the difference could be even more stark. 00:09:07.840 |
Imagine a future where GPT-4 or 5 can sample, say, a trillion 10 to the 12 solutions. 00:09:14.100 |
So you're beginning to see my hypothesis emerging. 00:09:16.420 |
A new and improved let's verify step by step called Q*, drawing upon enhanced inference 00:09:26.080 |
If you want more details on that process reward model, check out the video I did back then 00:09:32.500 |
But the very short version is that they trained a reward model to notice the individual steps 00:09:40.100 |
That reward model then got very good at spotting erroneous steps. 00:09:44.380 |
Furthermore, when that model concluded that there were no erroneous steps, as we've seen 00:09:48.520 |
from the graphs, that was highly indicative of a correct solution. 00:09:52.940 |
Notice also that sometimes it could pick out such a correct solution when the original 00:09:57.580 |
generator, GPT-4, only outputted that correct solution one time in a thousand. 00:10:03.260 |
Furthermore, the method somewhat generalized out of distribution, going beyond mathematics 00:10:08.320 |
to boost performance in chemistry, physics, and other subjects. 00:10:12.780 |
So was it potentially the million dollar inference run that spooked the researchers? 00:10:17.980 |
Or was it the potential to make radical breakthroughs in science? 00:10:22.060 |
And a further bit of supporting evidence for this theory comes again from the information. 00:10:26.980 |
It gives us a rough timeline in the months following the breakthrough. 00:10:30.740 |
Sutskve had reservations about the technology and in July he formed the super alignment 00:10:37.300 |
So the original breakthrough, whatever it was, had to have come way before July. 00:10:41.080 |
That would fit much more with it being associated with let's verify step by step. 00:10:45.540 |
Or again, maybe that combination of process reward modeling and inference time compute. 00:10:50.500 |
And in a genuinely fascinating recent conference call, this is what Ilya Sutskve said in reply 00:10:55.420 |
to a question asking about if models could produce mathematical conjectures. 00:11:00.380 |
How original or creative are the latest large language models? 00:11:07.480 |
Of course we know that for instance AlphaGo did some pretty creative moves when it won 00:11:20.800 |
But to be very concrete, do you think the existing models or some, you know, the next 00:11:28.920 |
GPT-4, say GPT-5 or so, would be able to state a new non-trivial mathematical conjecture? 00:11:44.120 |
Who thinks it's possible within the next five years? 00:11:49.280 |
Are you sure that the current model cannot do it? 00:11:59.720 |
I mean, let me give you an example of something creative that GPT-4 can already do. 00:12:06.840 |
Obviously we would have all loved to hear Ilya Sutskve's full answer to that question, 00:12:12.520 |
But here's where we get to arguably the strongest bit of evidence that I've got. 00:12:16.820 |
Remember that name again, Lucas Kaiser cited in the exclusive information report. 00:12:21.640 |
If you remember, he had that key role on the GPT-0 project. 00:12:25.720 |
He was a co-author in both of the papers that I've brought up, and he was focused on test 00:12:30.160 |
time computation to boost language models, problem solving abilities. 00:12:33.560 |
Well, as the article says, even more significantly, he was one of the co-authors of the original 00:12:38.680 |
attention is all you need transformers paper. 00:12:41.200 |
And so presumably with that much pedigree, it would take quite a lot to get him excited. 00:12:46.640 |
So I'm now going to show you some extracts from two YouTube videos, both of which have 00:12:50.980 |
hardly any views, despite him being mentioned in these exclusives about Q*. 00:12:56.140 |
And Lucas Kaiser will describe in these videos how significant he thinks a variation of let's 00:13:03.920 |
First he'll give you some further background on the breakthrough. 00:13:06.900 |
It needs to do this thinking inside its layers. 00:13:09.860 |
That may not be enough time and space to do it. 00:13:12.560 |
Like tell me what you're thinking and only then give the answer. 00:13:17.320 |
And if you do that, there is a recent paper that says the model can basically do any computation. 00:13:28.880 |
So on mathematics, you can tell the model, hey, do this thinking, but do it like number 00:13:35.560 |
each step, like one, two, three, four, five, six, seven, as you see here, and be very precise 00:13:42.760 |
And then you can try to verify each of these steps of thinking separately. 00:13:46.040 |
You can even ask the model, well, was step three correct? 00:13:50.340 |
And when you do that, like this MATH dataset, which is a little bit tougher math problems 00:13:55.340 |
than like the pure arithmetic, it was especially made to show like what the models cannot do. 00:14:02.240 |
If you add this thinking, you can get to like almost 80% just by allowing the model to think. 00:14:09.880 |
And at least on mathematical questions, this gives like insane gains. 00:14:19.080 |
Like if you-- a transformer has a limitation in running time. 00:14:24.400 |
It runs in n squared time for input of n, and that's it. 00:14:30.320 |
When you allow it to produce chains of thought, it's as computationally powerful as anything 00:14:37.880 |
But, okay, so these two ingredients give you something that generalizes. 00:14:45.080 |
And this is called chain of thought mostly, and chain of hindsight, and programs of thought 00:14:51.440 |
But I think this has turned out to be the method that makes transformers more powerful. 00:14:57.080 |
And it's not just mathematics where you can build this thinking. 00:15:02.080 |
He even goes as far as describing it as a major focus for deep learning in 2024. 00:15:08.480 |
If you think what is coming in the next years, I think there'll be a lot of focus on doing 00:15:16.240 |
this thinking thing with deep learning, with language models, probably with chains of thought, 00:15:22.600 |
but also these chains of thought currently, they're just prompted, but maybe you need 00:15:30.800 |
There'll be a lot of work there, but this is a very hard problem to solve. 00:15:35.200 |
And we'll start with much simpler exercises and probably move forward. 00:15:40.600 |
But I think this is something that the coming years will see a lot of work. 00:15:48.640 |
And in a sign of the significance he attributes to this method, he said it could even be revolutionary 00:15:55.960 |
I think you also need these chains of thought that, like you need to give the model the 00:16:09.000 |
So in the future, the models will have this knowledge of the world and this generation, 00:16:16.520 |
But multimodality, this means just it's a chain of frames of what's going to happen 00:16:21.240 |
to the world, which is basically how we sometimes think, you know, what will, if I go, what 00:16:29.240 |
And I think that will indeed be, so it will be multimodality and this ability to generate 00:16:35.400 |
sequences of things before you give an answer that will resemble much more what we call 00:16:44.720 |
He then described how this method will help models to generalize from much less data. 00:16:55.720 |
You can do GNNs, you can do recurrence in depth. 00:17:00.600 |
How do you see the next two years of deep learning? 00:17:04.440 |
Yeah, I think there'll be as interesting as any previous two years or even more. 00:17:11.080 |
I think there'll be a lot on the chain of thought, but very generally speaking. 00:17:16.720 |
So also on the agents, building libraries of knowledge, possibly multimodal where the 00:17:22.880 |
chain of thought is basically a simulation of the world. 00:17:28.240 |
So I think that will be like one big topic and I think this will make the models generalize 00:17:34.360 |
And that might remind you something, going back to Reuters and the information. 00:17:38.920 |
Sarscopha's breakthrough allowed OpenAI to overcome limitations on obtaining enough high 00:17:46.400 |
According to the insider with knowledge, a major obstacle for developing next generation 00:17:51.680 |
So according to my theory, this breakthrough is less about generating trillions and trillions 00:17:55.920 |
of tokens worth of synthetic data, but more about using the data you've got much more 00:18:01.880 |
But now, alas, we must get to the bits that my theory can't explain, namely the name. 00:18:07.120 |
The information sites two top researchers at OpenAI building on top of Sarscopha's 00:18:14.520 |
Now I've tested every link I could possibly find to the name Q* with my theory about let's 00:18:22.360 |
And while I do have some ideas, honestly, it's still an open question. 00:18:26.720 |
And of course, I like everyone has to admit that there's a chance that I'm entirely 00:18:32.000 |
When I put my idea to a senior ML researcher at a top AGI lab, he thought it had real legs. 00:18:39.400 |
And he said one link to the name Q* could be in a generic sense. 00:18:43.440 |
Without getting too technical, Q* refers to the optimal Q function or optimal policy. 00:18:48.800 |
Another possibility is that the Q references Q learning. 00:18:51.960 |
Generically, that's a reinforcement learning technique where an agent learns to make optimal 00:18:58.200 |
An agent chooses actions, see how they go, and then updates their policy. 00:19:02.520 |
Basically trial and error trading off exploration of new steps, new actions versus exploitation 00:19:10.660 |
And here's where the analogy gets a little bit tenuous. 00:19:13.600 |
Picking the reasoning steps in let's verify step by step could be like choosing an action. 00:19:18.520 |
After all, in the original paper, using test time compute in this way was described as 00:19:24.560 |
And in let's verify, they hinted at a step forward involving reinforcement learning. 00:19:29.080 |
They said we do not attempt to improve the generator, the model coming up with solutions, 00:19:35.280 |
We do not discuss any supervision the generator would receive from the reward model. 00:19:42.960 |
Although fine tuning the generator with reinforcement learning is a natural next step, it is intentionally 00:19:52.840 |
I mean, you can kind of think of Q learning for process supervision as minimizing the 00:19:58.280 |
cumulative probability of failure, which is the equivalent of maximizing the probability 00:20:04.400 |
And after all, maximizing a sum of rewards over multiple steps is exactly what Q learning 00:20:10.480 |
If any of you, though, have better guesses for the analogy, and I'm sure you do, do 00:20:19.400 |
Unlike the earlier parts of the video in which I am much more confident, this is much more 00:20:28.920 |
Remember the leak talked about acing math tests. 00:20:31.480 |
He said, "Sounds like OpenAI got some good numbers on GSM 8K." 00:20:36.200 |
Remember that's the set of questions made for that original Verifier paper back in 2021. 00:20:40.640 |
He said he's speculating, but there's a star in this paper, a technique that fine 00:20:48.520 |
In a nutshell, it involves fine tuning a model on the outputs it generated that happened 00:20:54.480 |
Keep going until you generate rationales that get the correct answer and then fine tune 00:21:01.100 |
And they say that we show that star significantly improves performance on multiple datasets 00:21:06.000 |
compared to a model fine tuned to directly predict final answers. 00:21:11.720 |
And performs comparably to fine tuning a 30X larger state-of-the-art language model. 00:21:17.360 |
He went on that GSM 8K and the math benchmark featured in Let's Verify are great testbeds 00:21:22.960 |
for self-improvement because model outputs can be evaluated for correctness more or less 00:21:28.680 |
This brings us on to another strand in what all of this actually means for us. 00:21:32.680 |
He said for more open-ended generation, humans often provide the feedback. 00:21:36.760 |
However, as LLMs have gotten more capable, an interesting emerging ability is that they're 00:21:41.080 |
getting better at evaluation for other things, not just math. 00:21:44.680 |
At some point, if self-evaluation or self-critique works reliably, you get general self-improvement 00:21:51.240 |
So this is a further possibility for what might have freaked out those researchers. 00:21:56.360 |
Generalizing beyond math though is hard, as Andrei Karpathy pointed out this week. 00:22:01.480 |
I think a lot of people are broadly inspired by what happened with AlphaGo. 00:22:06.440 |
In AlphaGo, this was a Go playing program developed by DeepMind. 00:22:10.880 |
AlphaGo actually had two major stages, the first release of it did. 00:22:14.680 |
In the first stage, you learn by imitating human expert players. 00:22:17.680 |
So you take lots of games that were played by humans, you kind of like just filter to 00:22:22.440 |
the games played by really good humans, and you learn by imitation. 00:22:26.320 |
You're getting the neural network to just imitate really good players. 00:22:29.280 |
This works and this gives you a pretty good Go playing program, but it can't surpass human. 00:22:35.160 |
It's only as good as the best human that gives you the training data. 00:22:39.140 |
So DeepMind figured out a way to actually surpass humans, and the way this was done 00:22:44.860 |
Now in the case of Go, this is a simple closed sandbox environment. 00:22:49.920 |
You have a game and you can play lots of games in the sandbox and you can have a very simple 00:22:54.440 |
reward function, which is just winning the game. 00:22:57.720 |
So you can query this reward function that tells you if whatever you've done was good 00:23:03.400 |
This is something that is available, very cheap to evaluate, and automatic. 00:23:07.840 |
And so because of that, you can play millions and millions of games and kind of perfect 00:23:11.340 |
the system just based on the probability of winning. 00:23:14.660 |
So there's no need to imitate, you can go beyond human. 00:23:17.500 |
And that's in fact what the system ended up doing. 00:23:19.960 |
So here on the right, we have the Elo rating and AlphaGo took 40 days in this case to overcome 00:23:25.820 |
some of the best human players by self-improvement. 00:23:29.920 |
So I think a lot of people are kind of interested in what is the equivalent of this step number 00:23:33.520 |
two for large language models, because today we're only doing step one. 00:23:39.000 |
As I mentioned, there are human labelers writing out these answers and we're imitating their 00:23:43.800 |
And we can have very good human labelers, but fundamentally, it would be hard to go 00:23:47.200 |
above sort of human response accuracy if we only train on the humans. 00:23:53.720 |
What is the step two equivalent in the domain of open language modeling? 00:23:58.920 |
And the main challenge here is that there's a lack of reward criterion in the general 00:24:03.520 |
So because we are in a space of language, everything is a lot more open and there's 00:24:08.420 |
And fundamentally, there's no like simple reward function you can access that just tells 00:24:11.880 |
you if whatever you did, whatever you sampled was good or bad. 00:24:15.480 |
There's no easy to evaluate fast criterion or reward function. 00:24:18.760 |
If models can get good at generalization using reinforcement learning with any of these techniques, 00:24:23.680 |
Ilya Satsykova has a slight warning that he put out earlier this year. 00:24:28.000 |
He compared the creative results we might get to outbursts from Bing Sydney. 00:24:33.920 |
Reinforcement learning has a much more significant challenge. 00:24:46.880 |
Every single stunning example of creativity in AI comes from a reinforcement learning 00:24:52.680 |
For example, AlphaZero has invented a whole new way of playing a game that humans have 00:25:01.080 |
It is reinforcement learning that can come up creative solutions to problems, solutions 00:25:05.880 |
which we might not be able to understand at all. 00:25:08.740 |
And so what happens if you do reinforcement learning on long or even medium time horizon 00:25:14.760 |
when your AI is interacting with the real world, trying to achieve some kind of a beneficial 00:25:21.240 |
outcome, let's say, as judged by us, but while being very, very, very creative. 00:25:27.000 |
This does not mean that this problem is unsolvable, but it means that it is a problem. 00:25:31.200 |
And it means that some of the more naive approaches will suffer from some unexpected creativity 00:25:36.120 |
that will make the antics of Sydney seem very modest. 00:25:45.200 |
I think the development is likely a big step forward for narrow domains like mathematics, 00:25:54.040 |
The world is still a bit too complex for this to work yet. 00:25:58.040 |
Anyway, time to move on to something more positive. 00:26:00.480 |
After all, even Sam Altman can now get along with Adam D'Angelo. 00:26:05.280 |
I'm going to end with some positive and amazing news about music generation. 00:26:09.640 |
But first I want to introduce you to the AI Explained bot. 00:26:13.400 |
If you're feeling bored, you may even want to discuss the contents of this video and 00:26:19.760 |
It has access to the transcripts of my videos, including this one. 00:26:23.320 |
I'm proud to announce that they're sponsoring this video and their playground is honestly 00:26:28.400 |
In fact, I reached out to them about sponsorship. 00:26:31.880 |
Their playground is super easy to use, even if you're not from a coding background. 00:26:35.680 |
And as they know, their speech to text model, Conforma 2, is state of the art and it is 00:26:42.820 |
A perfect example of that is how it can transcribe a GPT-4. 00:26:47.040 |
That is something in my transcripts that so many models struggled with. 00:26:50.480 |
Anyway, I have honestly thought that their playground is amazing for anyone to use for 00:26:55.760 |
And yes, it's literally just clicking to upload your audio file and then pressing transcribe. 00:27:00.960 |
Anyway, thanks to Assembly AI, you can now play about with the AI Explained bot. 00:27:05.920 |
After such a heavy video, I think it's only appropriate to end with a bit of music, but 00:27:10.880 |
not any music, music generated by Google DeepMind. 00:27:14.200 |
Their new Lyra model can convert your hums into an orchestra. 00:27:39.440 |
As always, thank you so much for watching and whatever happens, have a wonderful day.