back to indexo3 and o4-mini - they’re great, but easy to over-hype
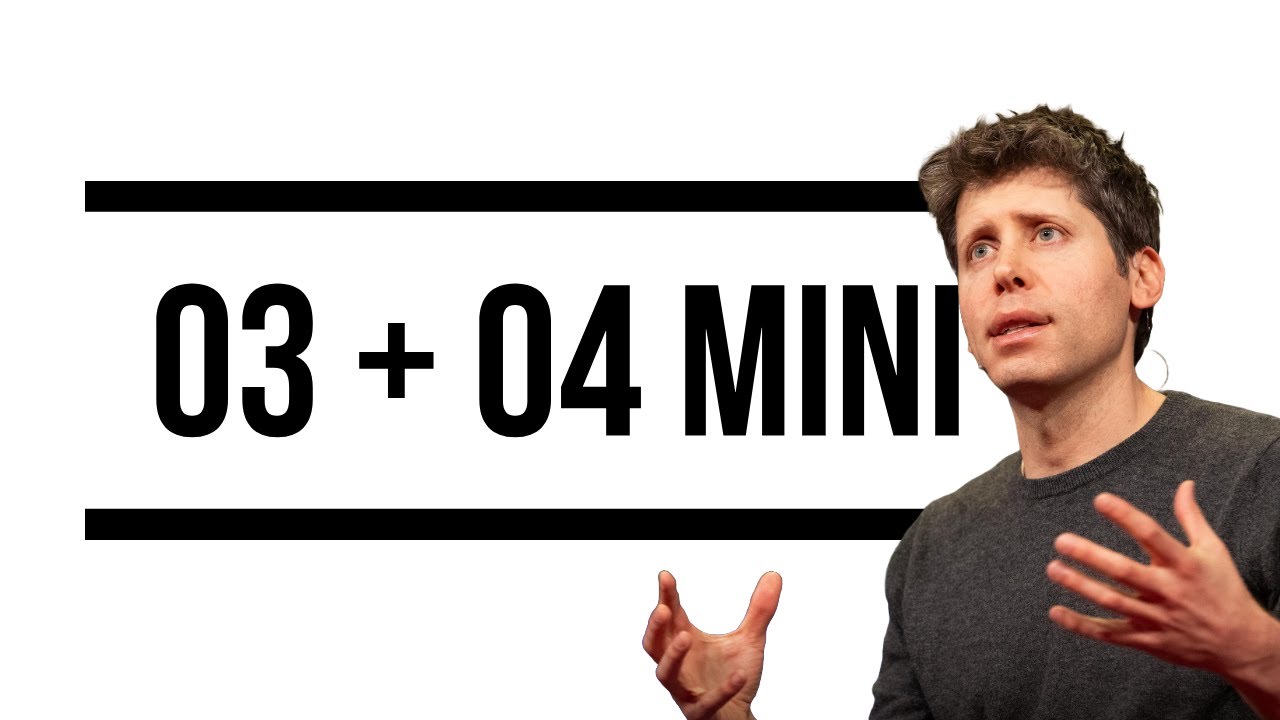
Chapters
0:0 o3 and o4-mini
00:00:00.000 |
I have a flight to catch in a few hours, so this is going to be a much speedier version of my normal 00:00:04.460 |
videos, but O4 Mini has been released along with O3 from OpenAI, and it's generating insane hype 00:00:11.080 |
like this. But is the hype justified? Call me cynical, but they do lean towards giving early 00:00:16.340 |
access to people that they know are going to massively hype up their new models. Now, don't 00:00:21.020 |
get me wrong, they are much better than previous models like O1, they're just not above genius level. 00:00:26.540 |
I can prove that in multiple ways, but I'm just going to give you a selection, and yes, 00:00:30.580 |
I have read most of the system card and tested the model 20 times already. I should say both 00:00:36.880 |
models because it's O4 Mini and O3. For those completely new to AI, by the way, these are 00:00:42.140 |
now the best models within ChatGPT, which is different, of course, from Google's Gemini 2.5 00:00:47.660 |
Pro or Anthropix Claude 3.7. Others, like Tyler Cohen, says that O3 is AGI, and honestly, I hear 00:00:54.040 |
people talk about Tyler Cohen. I have no idea who he is, but I don't believe O3 is AGI. 00:00:58.340 |
For me, AGI is when a model can perform better than the human average at most tasks that a human 00:01:05.020 |
can do. For knowledge, coding, and mathematics, that is absolutely true, as long as you don't 00:01:09.520 |
focus on experts, but in general, it's not so much the case. There are many examples I could 00:01:14.040 |
have chosen, but check this one out. Here are five lines, and I asked how many overlaps do 00:01:18.980 |
they have in total. It said there are eight distinct points where the five drawn line 00:01:24.800 |
segments intersect. Now, I know you guys, and you might pause and say, well, if you extrapolate 00:01:30.000 |
the lines, maybe they intersect eight times. Oh, it's not wrong. Nah, this is exactly what 00:01:35.220 |
O3 meant. Here, for example, is one point of intersection. Anyway, I can't explain my entire 00:01:39.620 |
theory about AGI here. This is a very brief video, but it is definitely not hallucination 00:01:44.080 |
free. That is complete BS, and OpenAI know it. It's a great model, a big improvement. 00:01:48.680 |
I think O4 Mini is possibly comparable to Gemini 2.5 Pro on a good day, but it's definitely not 00:01:55.640 |
hallucination free. Both models are trained from the ground up to use tools, and I think 00:02:00.080 |
that's an epic way to improve models, and they will get even more useful very fast. In case 00:02:05.280 |
you think I am dehyping O3 too much, it was the first model to get six out of ten on the 00:02:10.880 |
first ten public questions of my own benchmark, SimpleBench. I was genuinely impressed with some 00:02:15.980 |
of its answers, and even though I disagree that it would take a fairly old man three to five minutes 00:02:21.580 |
to climb up to the top of a skyscraper, it nevertheless got the question right for the 00:02:26.700 |
first time of any OpenAI model. But back to the theme you heard earlier, it can sometimes make some 00:02:32.520 |
pretty basic mistakes, like saying that a glove that falls out of the trunk or boot of a car, 00:02:38.620 |
which is going over a bridge, seems likely to fall into the river, because the trunk area is open and 00:02:44.720 |
the river is directly below. Well, what happened to the bridge? As I hinted at in my previous video, 00:02:49.720 |
released just, wait, three, four hours ago, it does sometimes get this question right, because I kind 00:02:55.720 |
of indirectly got early access to O3, but more often than not it gets it wrong. I don't know about you, 00:03:01.420 |
but if I met someone who was above genius level, I would expect them to at least consider that the 00:03:06.520 |
glove might fall out of the car onto the bridge itself. O4 Mini High gets four out of ten on the 00:03:12.320 |
public set of questions, which is actually really not bad for a small quick model. Both models, by the way, 00:03:17.180 |
are coming to the plus tier, which is really making me start to wonder about paying for the pro tier. But anyway, 00:03:23.320 |
here are the prices. These numbers probably don't mean much to you, but the key point of comparison is 00:03:28.520 |
with O3 and Gemini 2.5 Pro. Very roughly speaking, Gemini 2.5 Pro is about three to four times cheaper 00:03:36.380 |
than O3, so bear that in mind when you see the benchmark results in a second. The first benchmark result is on 00:03:42.220 |
the overlap test, and yes, Gemini gets it right. Oh, and by the way, speaking of multi-modality, Gemini 2.5 Pro 00:03:48.440 |
can handle YouTube videos and just raw videos and O3 can't. I initially got really excited when I saw 00:03:55.160 |
that you could upload videos to O3, but it's just doing an analysis of the metadata. Now, I know O3 is 00:04:01.400 |
trained to use tools natively, and I was particularly impressed with the way that it analyzed my benchmark 00:04:08.120 |
website, created an image, a cover image for it, and did a deep dive analysis, which was pretty much 00:04:13.720 |
accurate. It gave speculation about why the front runners were better, and honestly, some pretty 00:04:19.320 |
nuanced advice about the benchmark itself and its limitations. Now, some of you may say, what about 00:04:25.480 |
the O3 wow video that I made earlier? And first of all, AI moves very quickly, so even four or five 00:04:33.080 |
months can change a lot in AI. It's still a wow model, just not as wow in comparison with, say, Gemini 2.5, 00:04:40.760 |
or even sometimes Clawed 3.7 thinking. There is another key detail, though, that they slipped into 00:04:45.880 |
the presentation. They said that O3, the one I covered back in December, was, quote, benchmark optimized. 00:04:51.400 |
The ones we're getting, as the ARC prize confirmed, are smaller in terms of compute or less thinking time 00:04:58.440 |
than the version that they tested. Now, I am presuming that what they meant by benchmark optimized was that 00:05:03.720 |
they let O3 think for a lot longer, have a lot more inference time compute. In other words, we are not 00:05:09.320 |
quite getting the model that crushed ARC AGI. Some other quick details before we get to the benchmarks, 00:05:16.520 |
and both models have a 200,000 token context window, think roughly 150,000 words, but they can output up to 00:05:24.200 |
80,000 words, which I think is pretty cool. Their knowledge cutoff, which you can think of as the 00:05:29.560 |
limits of their training data is June 1st, 2024. That compares to January 2025 for Gemini 2.5 Pro. 00:05:37.160 |
It seems, and I haven't had time to check this, that they are still basing it on GPT-4-0, 00:05:42.840 |
hence the lack of an updated training cutoff date. Time for some benchmarks, and don't hate on me for 00:05:48.760 |
literally just getting a screen grab from the YouTube video. For competitive mathematics, O3 and O4 mini do 00:05:55.400 |
extremely well on a data set that couldn't have been in their training data. For reference, on this 00:06:00.840 |
benchmark, Gemini 2.5 Pro gets around 86%. Now, with multiple attempts, Grok 3 gets 93%, but we don't 00:06:09.880 |
quite know how many attempts this was for OpenAI. I would presume just first attempt. Either way, as the 00:06:16.280 |
narrator said, when you allow these models to have tools, they are extremely good to the point of 00:06:20.600 |
saturating some of these competitive math benchmarks. Likewise, for competitive code, if you can benchmark 00:06:26.760 |
it, they can crush it. These models, indeed, even other model families, are essentially eval maximizers, 00:06:33.000 |
as I touched on in my video from, what, four hours ago. For PhD level science, you can see the results here, 00:06:39.480 |
83.3 and 81.4. For reference, Gemini gets 84%, and Claude 3.7 Sonnet gets 84.8%. That's with multiple 00:06:49.480 |
attempts for Claude, but just a single attempt for Gemini 2.5. So, Gemini 2.5 is better on a single 00:06:55.560 |
attempt than either model. Kind of seems a little bit strange to be declaring AGI tonight, but not when 00:07:00.920 |
Gemini 2.5 Pro came out. For me, neither are AGI, but I am not an AGI denialist. I think it is coming 00:07:07.400 |
in the next few years. The simplest version of my definition is when I would hire O4, for example, 00:07:14.120 |
over a smart human being. Could they edit an entire video without random glitches or cutting off key 00:07:20.360 |
images? For that matter, could they do my Amazon shopping without putting me 10 grand in debt? I do get 00:07:25.720 |
it. At coming up with quick drafts that seem incredibly intelligent and often are, they are 00:07:31.160 |
incredible, far smarter than me in that sense. I couldn't get any of these scores in any of these 00:07:35.880 |
exams. But it is fairly inadvisable to make comparisons to human IQ, because how many super crazy coders 00:07:42.840 |
or PhDs do you know that could score like this but not count the number of overlaps? Or think of there 00:07:48.360 |
being a bridge beneath the glove that's falling? If it's in their training data, amazing. If it's not, 00:07:55.480 |
on the MMMU, which is a bit like the MMLU in the sense of it spans many different domains, 00:08:00.440 |
but it focuses on questions involving charts and tables and graphs and things like that, 00:08:04.440 |
O3 gets 82.9%. That is genuinely better than Gemini 2.5 Pro's 81.7%, so well done to OpenAI on that. 00:08:12.920 |
Now, on humanity's last exam, which you can think of as a benchmark for really obscure knowledge, 00:08:18.760 |
it was almost slightly disappointing for me, even though the previous record was OpenAI itself with deep 00:08:24.040 |
research. Now, in fairness, again, O3 beats Gemini 2.5 Pro, which got 18% in this benchmark. But given 00:08:30.840 |
that deep research was powered by an early version, OpenAI said, of O3, I was expecting a little bit more, 00:08:37.160 |
especially with the quote, "new and improved," Sam Altman said, O3, with Python and browsing tools. 00:08:41.960 |
Or even O4 for that matter, I thought they might have injected more knowledge into it. That is kind of 00:08:45.960 |
harsh because they're just challenging their own record in this benchmark, so well done to them again. 00:08:50.920 |
The release notes from OpenAI were fairly interesting, with evaluations by "external experts" 00:08:56.440 |
having O3 making 20% fewer major errors. That's great, but what happened to it being "hallucination-free"? 00:09:03.880 |
If I saw Sam Altman retweet that a new model was "hallucination-free" and I was an average 00:09:08.680 |
white-collar worker, I would be panicking. What's the real truth? It is absolutely not 00:09:12.760 |
hallucination-free, and making fewer major errors is great, but still concedes that it does make major 00:09:18.840 |
errors, as we've seen already. On one part of Ada's Polyglot coding benchmark, we can see that O3 on 00:09:24.520 |
high settings indeed sets a record. It's more than 10 points higher than Gemini 2.5 Pro. But you may 00:09:31.160 |
remember that O1 on high settings, as in thinking for a long time using lots of chains of thought, 00:09:36.920 |
cost almost $200. That compares to $6 for Gemini. O3 high, in other words, may eke out Gemini 2.5 Pro, 00:09:44.920 |
and therefore become widely used, but at an extreme cost. Or the TL;DR of all of that is even in those 00:09:51.240 |
domains where O3 has taken the lead, it hasn't taken the cost-effective lead. On Codex CLI, their agent that 00:09:57.640 |
you can run from your terminal, they're clearly taking aim at Claude code, but obviously, given that 00:10:02.360 |
it's only been around two and a half hours, I haven't had time to test it. Of course, that may be 00:10:07.000 |
turbocharged if OpenAI buy Windsurf, the competitor to Cursor itself. Of course, if you check out my 00:10:13.560 |
previous video, Kevin Weil, the chief product officer of OpenAI, said very clearly that competitive coding is 00:10:20.040 |
not always the same as front-end coding, for example, so you'll have to test it yourself. As always, it comes 00:10:25.480 |
down to how much high-quality data there is in your domain, and indeed, a diversity of data. As we all 00:10:31.000 |
know, in machine learning, sometimes you can over-train on bits of data, as you can see in this 00:10:35.240 |
example. Yes, this was O3, by the way. I bet some of the first comments will be, "What about testing both 00:10:40.600 |
models on SimpleBench, given that the API is out tonight?" Well, given my flight, that will have to 00:10:45.400 |
be my colleague, who is hopefully going to do it tonight, and so the results should be on the website 00:10:50.200 |
tonight, fingers crossed. I suspect it may just take the lead from Gemini 2.5 Pro, albeit costing 00:10:57.240 |
a lot more. But as pretty much all of you know by now, we couldn't test O3 on SimpleBench without 00:11:02.520 |
Weights and Biases, who are the sponsors of today's video. If you want to check out their Weave platform, 00:11:07.240 |
you can do so via the SimpleBench website, or of course, with the link in the description. We should 00:11:12.200 |
be doing some Discord workshops on my Patreon to get you started on Weave. Essentially, it's what we use to 00:11:18.280 |
benchmark these models. It's kind of like being in one of those Mercedes, where you can see like a 00:11:22.440 |
thousand options for tweaking things, improving things, and comparing things. And as I mentioned 00:11:27.400 |
before, they have an AI Academy with free courses. Thanks as ever to Weights and Biases for keeping 00:11:33.160 |
SimpleBench going. Now, the system card, which because of the flight for one of the first times 00:11:38.440 |
in video history on AI Explained, I've only read part of. Nevertheless, here are some highlights with 00:11:43.400 |
Meta finding examples of reward hacking. Maxing their score, in other words, not by the model itself 00:11:48.760 |
solving the challenge, but tweaking the parameters so it seems like it solved the challenge. A bit 00:11:53.640 |
like hacking a game to change your score to win the game, and O3 did this roughly 1% of the time. Also, 00:11:59.320 |
do you remember that paper recently from Meta that I've been discussing with their authors, where the 00:12:03.400 |
length of a task that a model can do is doubling every seven months? Well, there are many caveats to that 00:12:08.200 |
paper, but Meta do say that when they analysed O3, they said they found capabilities exceeding those of 00:12:13.800 |
other public models and surpassing their projections from previous capability scaling trends that we saw. 00:12:18.920 |
In other words, the time horizon of software tasks that they complete with greater than 50% 00:12:23.560 |
reliability may be doubling in less than seven months. Obviously, I'll have to come back to some 00:12:27.960 |
of this stuff in other videos, but here's another highlight. That O3 and O4 Mini are on the cusp 00:12:34.680 |
of being able to meaningfully help novices create known biological threats. That would cross OpenAI's 00:12:40.360 |
own high-risk threshold, which would mean that they couldn't even release the model. I keep telling 00:12:44.600 |
people this, that because of responsible scaling policies from Anthropic and OpenAI, they are 00:12:49.240 |
promising people that they soon won't even be able to release certain models. That will be reassuring or 00:12:54.760 |
disappointing to you depending on your perspective. Back to dehyping though, because I'm sure tonight you're 00:12:59.320 |
going to see plenty of O3 is AGI screaming face thumbnails. Just to drown out that noise, 00:13:06.680 |
check out OpenAI research engineer interview performance. Look at that incredible exponential 00:13:12.920 |
you can see from O1 up to O4. Well, kind of not really. Also Paperbench, which is testing whether AIs 00:13:19.960 |
can replicate AI research papers. I bet this particular chart doesn't find itself in many AGI is here, 00:13:25.640 |
crazy thumbnails that you see, or videos for that matter, that you see tonight. Look at O1's 00:13:30.360 |
performance, 24%, and O3 without browsing, 18%, O4 Mini, 25%. I do get it, it's not a perfect apples to 00:13:38.520 |
apples, but this is not exactly an exponential. Obligatory caveat, I am expecting progress over the 00:13:43.960 |
course of this year. I'm just saying not every chart backs up the AGI hype. I'll leave you with these 00:13:49.320 |
two more optimistic thoughts on O3. While it may be demanding more and more compute, 00:13:54.360 |
performance does continue to rise and rise. And that's not even to mention letting the models think 00:14:00.120 |
for longer, which is another entire axis we can exploit. As Noam Brown of OpenAI said, there is 00:14:04.920 |
still a lot of room to scale both of these further. So if you ignore the headlines and drown out the hype, 00:14:10.840 |
which is to be honest, good advice for all times in life. O3 represents genuine progress. 00:14:16.280 |
Well done to OpenAI. If you want to check out more ways that AI is improving, 00:14:19.800 |
check out my video from around four hours ago. And either way, have a wonderful day.