back to index20 Years of Tech Startup Experiences in One Hour
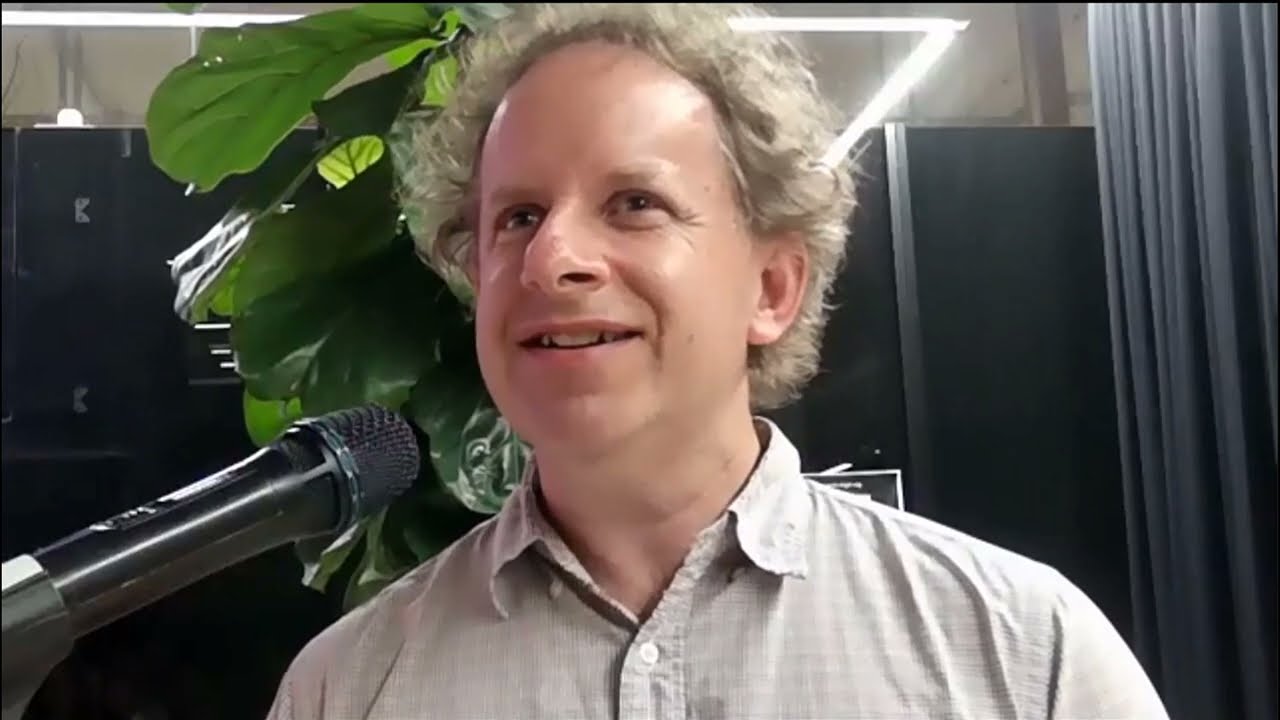
Chapters
0:0
0:27 Jeremy Howard
11:52 Octopus Deploy
12:48 Fast Ai
13:21 Kaggle
27:11 Deep Learning
37:0 Fast Ai
37:5 Faster Ai
43:25 Lack of Investment in Research
53:56 What's Harder Getting an Idea
58:44 What's Next
59:34 How To Market an Early Stage Company
00:00:00.000 |
- Hi everybody and welcome to the literally just launched 00:00:12.760 |
So I actually was only wearing this for the advertising. 00:00:29.640 |
I'm originally from Australia, grew up in Melbourne 00:00:40.880 |
What I always used to think of as Silicon Valley, 00:00:43.280 |
but then I got there, was staying in San Francisco 00:00:46.600 |
when somebody said, let's meet up in Silicon Valley 00:00:48.680 |
and an hour and a half later, I still hadn't got there. 00:00:52.120 |
it's actually quite a long way, especially with the traffic. 00:00:55.920 |
So San Francisco Bay area, I was there for about a decade 00:00:59.280 |
and returned back here to Australia two months ago 00:01:04.160 |
and have made the move from Melbourne to Queensland, 00:01:15.420 |
Having said that, overwhelmingly the reaction 00:01:21.120 |
that Rachel, my wife and fast AI co-founder and I get 00:01:24.680 |
when we tell somebody, when they come up and they'll say, 00:01:27.480 |
oh, welcome to Australia, welcome to Queensland. 00:01:50.900 |
Not really, San Francisco, but what are you doing? 00:02:07.800 |
In fact, we're way down here in terms of investment in AI 00:02:18.100 |
And this data is from Andrew Lai from Boab AI. 00:02:22.360 |
Thank you very much to Andrew, who's actually given me 00:02:24.200 |
quite a lot of cool data that I'll be sharing. 00:02:34.480 |
I gotta say it's 0.29% more than when I left, 00:02:47.400 |
to start a tech startup and actually a really great place 00:03:07.640 |
through the lens of kind of describing my journey, 00:03:15.800 |
So my journey, as I said, kind of started in Australia, 00:03:20.800 |
right, that's a bit of a thick one, isn't it? 00:03:36.280 |
you know, it'd be really cool to start a startup. 00:03:38.700 |
I mean, I can only think of the startup sense, 00:03:45.720 |
Jeremy, you don't know anything about business. 00:03:55.060 |
And it's like, nah, you don't know anything about business. 00:04:04.920 |
So I thought, okay, let's go to McKinsey and company. 00:04:10.140 |
They know about business and spend a couple of years there. 00:04:15.400 |
And I went to a couple of different consulting firms 00:04:28.680 |
that people want and then selling it to them. 00:04:31.960 |
So I did certainly learn some valuable skills 00:04:35.960 |
particularly the skills around how to influence people, 00:04:42.760 |
but the actual explicit feedback I got about my ideas 00:04:54.880 |
that I had bought that contained this really cool thing. 00:05:03.560 |
And it's like, this person likes these movies 00:05:07.040 |
And through some kind of magic I didn't understand, 00:05:09.300 |
which I now know is called collaborative filtering, 00:05:13.640 |
and it would tell you other movies you might like. 00:05:16.080 |
And so I went into and I talked to one of the directors 00:05:23.400 |
Like you could even have like a website that wasn't static, 00:05:26.120 |
but you go to their homepage and it could like tell you 00:05:44.400 |
Similar reaction when somebody was talking about 00:06:05.140 |
what if we instead of having like lots of humans 00:06:09.240 |
finding websites and putting them into a hierarchy, 00:06:14.560 |
that would automatically find interesting websites 00:06:17.160 |
based on like what you typed in or something? 00:06:22.800 |
Humans need other humans to help them find things. 00:06:31.720 |
And so overall, this was kind of my experience 00:06:39.400 |
for potential people doing tech startups here 00:06:46.640 |
'Cause us old people don't know what we're talking about, 00:06:51.080 |
unless it's explicitly about the actual thing 00:06:56.280 |
And they actually have years of experience in that thing, 00:07:02.420 |
Because otherwise, all you get is these kind of 00:07:07.040 |
biases about business as usual about the status quo. 00:07:19.120 |
and I thought there's something wrong with me 00:07:23.800 |
that I didn't understand why these ideas were bad ideas. 00:07:27.160 |
So I actually ended up doing consulting for 10 years, 00:07:29.760 |
which was eight years longer than I had planned, 00:07:31.800 |
still trying to figure out what's wrong with me. 00:07:42.600 |
Now, the problem is that I'd read that statistically speaking, 00:07:57.040 |
probabilistically speaking, better chance of success. 00:08:04.440 |
And literally within like a month of each other, 00:08:09.220 |
Now, aren't you drawing Optimal Decisions Group? 00:08:17.000 |
It was basically the first one to provide synchronized email, 00:08:20.240 |
whether email you got in your phone or on your laptop 00:08:23.580 |
or in your workplace, you're to see the same email. 00:08:26.480 |
It's something that actually everybody in business 00:08:31.000 |
or they used Lotus Notes, but normal people didn't. 00:08:35.760 |
So I built this company and it's still going great. 00:08:40.760 |
And then Optimal Decisions was a insurance pricing, 00:08:48.360 |
Fastmail sold to millions of customers around the world. 00:08:52.640 |
And Optimal Decisions sold to huge insurance companies. 00:08:57.040 |
So it's basically only three or four insurance companies 00:09:01.540 |
And then, you know, a couple of dozen in America, 00:09:15.920 |
I didn't get any funding 'cause like for a consultant, 00:09:19.780 |
You just build things and sell them to people. 00:09:44.200 |
and I had them shipped to somewhere in New York 00:09:56.040 |
was about a hundred times cheaper than Australia. 00:09:59.160 |
And the number of customers I had access to in America 00:10:15.320 |
the focus, I mean, I certainly had some Australian clients 00:10:21.600 |
'cause there's a lot more big insurance companies in America. 00:10:31.240 |
I didn't quite have a sense of how far away we are 00:10:42.720 |
And but the fact that then we were just companies, 00:10:53.520 |
It just, you know, we were competing on a global stage 00:10:57.080 |
without any constraints caused by our location. 00:11:24.040 |
in Australia don't try to be an Australian company, you know? 00:11:31.880 |
but that is tiny compared to all the world out there. 00:11:41.920 |
If you create something like fast mail, right? 00:11:51.560 |
you come across this company called Octopus Deploy, 00:12:01.200 |
Created an open source software, chucked it up on GitHub, 00:12:12.520 |
It was a company that happened to be in Australia. 00:12:20.660 |
they got, I think it was $185 million of funding. 00:12:25.660 |
And none of that funding was from Australian investors. 00:12:29.720 |
So it kind of bypassed the whole Australian thing 00:12:38.100 |
"I pretty much understand quite well deployment. 00:12:47.160 |
And so it's a similar thing now for Rachel and I with FastAI. 00:12:50.960 |
We started FastAI, which we'll come back to later in the US. 00:13:04.800 |
And so, you know, we have access to the global marketplace. 00:13:19.600 |
So ODGI co-founded, and obviously the next one, 00:13:26.880 |
With Kaggle, we decided to try a different approach 00:13:42.160 |
let's not even try to get funding in Australia 00:13:45.840 |
because Australia doesn't fund tech startups. 00:14:03.180 |
is less than the amount of funding of startups 00:14:08.240 |
So when I say it's different, it's very, very different. 00:14:12.180 |
So we went to San Francisco to try and get funding. 00:14:20.720 |
And honestly, we didn't tell this to the VCs. 00:14:31.080 |
but didn't quite know how to make money out of it. 00:15:10.880 |
But this was actually a start of a theme in the Bay Area, 00:15:19.920 |
which was every time we'd say we want to do X, 00:15:23.320 |
people would say like, well, okay, that's great. 00:15:27.520 |
Or like, what if you could make an even better X? 00:15:34.880 |
came to our little co-working space in San Francisco. 00:15:47.480 |
And they all know everything about what's going on. 00:15:49.480 |
So the Node was like, oh, I heard Mark Andreessen 00:16:04.480 |
And they were like, wow, it just kept pushing. 00:16:12.400 |
'cause I found doing my little startups in Australia, 00:16:23.320 |
an email company that does synchronized email 00:16:39.440 |
There's like, honestly, is there any chance that, 00:16:42.640 |
obviously there's no chance you can beat them. 00:16:50.760 |
Is there something more targeted you could do? 00:17:31.320 |
and try to compete with you won't do as well as you 00:17:34.000 |
You have to have the arrogance to believe you can win. 00:17:44.240 |
and they actually have some better ideas than you. 00:17:46.080 |
And so sometimes you should borrow those ideas 00:17:48.080 |
or sometimes you should try and find ways to do it better. 00:17:56.120 |
And in Australia, I found people mainly noticed the arrogance. 00:18:01.600 |
But yeah, in the Bay Area, everybody was just like, 00:18:05.080 |
oh, this is really cool that you're trying to do this thing. 00:18:11.520 |
The other thing that I got a lot in Australia 00:18:22.280 |
It's almost like you're a whinger or a complainer. 00:18:29.040 |
You know, why aren't you okay with what's there? 00:18:32.560 |
Whereas the other thing is there's this nice sense 00:18:33.920 |
in the Bay Area of like, oh, it's really cool 00:18:39.800 |
And so there are some cultural things that I felt 00:18:46.880 |
to build a great tech entrepreneur ecosystem. 00:18:54.440 |
who are cheering you on and who are believing in you. 00:19:05.600 |
They hadn't done any machine learning investments before. 00:19:11.480 |
is the VCs you speak to don't do any of the tech stuff 00:19:20.520 |
we don't say to have a great ecosystem for here either, 00:19:23.200 |
is like, you don't see this strong connection 00:19:25.080 |
between investors and academics in Australia. 00:19:30.560 |
one of the professors at Stanford or Berkeley 00:19:32.960 |
and say, can you please meet with Jeremy and Anthony? 00:19:38.960 |
So with Andres and Horowitz, I mean, to their credit, 00:19:41.360 |
they, through their DD, they kind of came to the point 00:19:44.240 |
where they said, okay, we're just not convinced 00:19:45.560 |
about the size of the machine learning marketplace. 00:19:50.280 |
So we got out, we ended up getting our $5 million 00:19:55.480 |
in the VC world over there is the whole thing 00:19:58.840 |
is so driven by fear of missing out, by FOMO. 00:20:02.440 |
So then suddenly people that we hadn't heard from 00:20:12.960 |
We're really excited about what you're doing. 00:20:14.400 |
These are people who not replied to emails for weeks. 00:20:24.560 |
between Anthony and I had a promise between ourselves. 00:20:31.760 |
We're like, okay, we've said, we always say yes. 00:20:39.960 |
The people who said they were dying to see us 00:20:45.280 |
for like half an hour in their giant board room. 00:21:09.360 |
If Mark fucking Andreessen was here right now, 00:21:30.440 |
'cause I fucking hate Mark fucking Andreessen. 00:21:34.680 |
It's like, it was so much like this over there. 00:21:40.600 |
If you've ever seen Silicon Valley, the TV show, 00:21:46.880 |
but they couldn't put that in the real thing. 00:21:49.880 |
Do you guys remember the hot dog detector in that show? 00:21:53.880 |
Did you notice there was a real hot dog detector? 00:21:58.360 |
That was built by a fast AI student, by the way. 00:22:06.120 |
and he'd always ask these weird asked questions. 00:22:09.960 |
He'd be like, I can't tell you what I'm doing, 00:22:12.560 |
but let's say somebody was trying to find microphones 00:22:17.440 |
and then they got lots of pictures of microphones 00:22:29.400 |
and he's like, okay, that's what I was building. 00:22:34.000 |
That was definitely one of our star students. 00:22:49.600 |
Was I actually didn't expect us to raise any money, honestly. 00:23:00.760 |
He was always the one with gumption, you know? 00:23:08.000 |
and I'll build the deck, but don't have high expectations. 00:23:12.840 |
and yeah, the Node Coaster kind of looked at us 00:23:17.880 |
and was like, so when are you guys moving here? 00:23:26.760 |
'cause I've been in every pitch and whatever. 00:23:55.320 |
It was interesting, like I was really starstruck. 00:24:07.320 |
and I'd be like talking to a Google product manager 00:24:09.480 |
and I was definitely like, wow, this is very exciting. 00:24:14.880 |
But the other thing I really noticed was like, 00:24:20.360 |
but then I was like, they're actually really normal. 00:24:24.000 |
You know, I kind of expected them to be on another level. 00:24:36.440 |
to my mates back in Australia, they weren't all that. 00:24:42.760 |
They were smart enough, they were passionate. 00:24:49.920 |
the Australian kind of talent pool is just fantastic, 00:25:02.960 |
Like everybody I spoke to, you know, in San Francisco, 00:25:13.000 |
The Airbnb, people that ran the Airbnb, I was at like, 00:25:19.480 |
'Cause like everybody is there doing tech startup. 00:25:25.880 |
And I've got this idea that's gonna revolutionize 00:25:33.160 |
Like everybody you talk to has not just got an idea, 00:25:40.800 |
Which I don't get that, or at least at that time 00:25:50.480 |
So I think that was a really interesting difference. 00:26:13.080 |
I guess it's part of this kind of boldness, right? 00:26:16.960 |
So I felt like folks there were on the home or bold. 00:26:20.480 |
But interestingly, even though they were in the center 00:26:30.840 |
American startups through American audiences, 00:26:37.200 |
that we're gonna chuck stuff up on the internet 00:26:41.120 |
And you know, in terms of like who really needs 00:26:47.880 |
Now one of the really cool things about being at Kaggle 00:26:57.480 |
I was the chief scientist there as well as the president. 00:27:06.120 |
what are the actual best ways to do things right now. 00:27:09.240 |
And around 2012, I started noticing deep learning, 00:27:13.600 |
starting to win things or at least do pretty well. 00:27:18.080 |
And I had last used neural nets like 20 years earlier. 00:27:24.520 |
probably gonna change the world one day, but not yet. 00:27:27.280 |
And then 2012, it's like, oh, I think the day is coming. 00:27:34.200 |
And that really became very clear during 2013. 00:27:51.080 |
were like, they were like all the same person. 00:28:06.000 |
like trying to find their cats in their photos or whatever. 00:28:09.800 |
I mean, look, okay, it's nice to find your cats 00:28:11.800 |
in your photos and people make a lot of money from that. 00:28:16.240 |
with global water shortages or access to education 00:28:32.200 |
you only get a kind of a diversity of problems solved 00:28:35.360 |
if you have a diversity of people solving them. 00:28:37.880 |
So we actually started getting pretty concerned about that. 00:29:01.560 |
I wonder if I've got any slides about this thing, 00:29:28.000 |
And it's literally like electricity and steam engine 00:29:34.880 |
to generally put human or animal energy inputs in anymore 00:29:44.360 |
to doing the same thing for intellectual inputs. 00:29:54.000 |
there are people who kind of have this sense of like, 00:30:17.240 |
Like if you just look at what it can do, right? 00:30:24.400 |
You type in an illustration of a baby daikon radish 00:30:33.920 |
It's not finding these, it's drawing them from scratch 00:30:37.760 |
'cause nobody's asked for that before, right? 00:30:40.920 |
You type in an armchair in the shape of an avocado, 00:30:53.200 |
This is not something a logistic regression does. 00:31:02.720 |
DeepMind created this thing called alpha fold, 00:31:10.280 |
which blew away decades of research in protein folding 00:31:25.080 |
really close example of this from kind of what I've seen 00:31:29.920 |
is early in the days of my medical startup in Lytic, 00:31:41.640 |
And so we had this guy come in and tell us about his PhD 00:31:47.680 |
And he spent 45 minutes telling us about his, you know, 00:31:55.440 |
And he was getting like new state of the art results 00:31:58.960 |
on this particular kind of histopathology segmentation. 00:32:02.080 |
And we were like, oh, that sounds pretty cool. 00:32:04.600 |
He was like, yeah, I used to think that too yesterday. 00:32:09.960 |
with deep learning and I kind of got curious. 00:32:12.400 |
So I thought I'd try this with deep learning yesterday 00:32:22.240 |
And like, this is like a really common story. 00:32:40.640 |
if you haven't done any deep learning yourself, 00:32:53.880 |
that go between kind of one model and another. 00:33:11.640 |
to the computer vision object recognition models 00:33:16.720 |
It's a base of basically a bunch of residual layers 00:33:23.520 |
And it's just an extraordinarily powerful general approach. 00:33:28.520 |
And so it's really cool kind of as a researcher 00:34:00.520 |
I realized that there really was some low hanging fruit 00:34:11.300 |
No one literally was doing deep learning in medicine. 00:34:17.340 |
And it turns out that there's such a shortage globally 00:34:40.200 |
I wonder if we could help make doctors more productive 00:34:45.200 |
by adding some deep learning stuff to what they're doing. 00:34:50.320 |
Let's try and do some kind of proof of concept. 00:35:06.640 |
And again, like literally none of us knew anything 00:35:10.680 |
And we discovered much throughout kind of shock 00:35:13.240 |
that this thing we trained had much lower false negatives 00:35:22.360 |
than a panel of four top Stanford radiologists. 00:35:31.420 |
And yeah, again, for Enlidic, I went the VC route, 00:35:47.540 |
And it was kind of a lot easier 'cause I knew people. 00:36:01.620 |
It was great in the sense that I really hoped 00:36:04.380 |
that this startup would help put medical deep learning 00:36:11.500 |
And within a couple of years, particularly in radiology, 00:36:26.820 |
when there's so many great people around the world 00:36:30.980 |
solving important problems and disaster resilience 00:36:54.960 |
working on deep learning, Rachel and I actually decided 00:37:02.340 |
And so fast.ai is all about helping everybody 00:37:16.580 |
but not having a bunch of deep learning people do it, 00:37:23.100 |
by disaster resilience people and have ecology stuff 00:37:27.960 |
Because it's much easier, this is our hypothesis, 00:37:31.140 |
it'd be much easier for a domain expert in ecology 00:37:34.580 |
to become an effective deep learning practitioner 00:37:48.500 |
and make the connections in the networks, blah, blah, blah. 00:38:07.800 |
It's something that only a few people in the world 00:38:21.780 |
And it was really lots and lots of gatekeeping. 00:38:34.480 |
And every few days we get lovely, lovely emails 00:38:40.140 |
from people telling us how they've just published a paper 00:38:46.220 |
or they've bought deep learning to their startup. 00:38:49.700 |
And increasingly they're using also the software 00:39:08.320 |
which I guess is something I did learn from consulting 00:39:38.980 |
and grounded group of people, as you may have noticed. 00:39:42.220 |
And in fact, in industry, there's a lot of brilliant people 00:39:47.760 |
And so this has been one of the interesting things 00:39:49.380 |
in fast.ai is a lot of the really powerful examples 00:39:52.400 |
we hear about are actually coming from industry. 00:39:56.280 |
Unfortunately, the problem with America is, well, you know. 00:40:11.780 |
and we certainly couldn't bring up our child there, 00:40:23.120 |
and eventually the government here let us in. 00:40:25.440 |
And coming back to Australia was just amazing 00:40:35.760 |
I kind of had this vague sense that Australia 00:40:45.380 |
But then coming back here, it just really hit me 00:40:49.540 |
that like Australia is such a bloody good country. 00:40:53.980 |
Like, and the people, like there's this kind of like, 00:41:06.020 |
And it's just after spending 10 years in America, 00:41:19.500 |
You know, it was like, it felt like I'd been stifling 00:41:22.580 |
humidity for 10 years and I kind of came back to sanity. 00:41:30.140 |
I was also shocked by how little have changed here. 00:41:33.260 |
Yes, a whole lot of accelerators and incubators 00:41:43.780 |
But when it actually came to the rubber hitting the road, 00:41:56.340 |
huge global impact or venture capitalist investing 00:42:07.500 |
And actually, Michael Evans was kind enough to 00:42:11.500 |
let me share some stuff that he has been working on, 00:42:15.580 |
kind of looking at this from a data point of view. 00:42:18.180 |
And you can kind of see it in the data, right? 00:42:25.260 |
seed and angel investment in Australia is like, 00:42:29.220 |
per capita, is like an order of magnitude behind the US. 00:42:33.500 |
And this is like, this is where things get going, right? 00:42:43.740 |
that's gonna be really hard for entrepreneurs, right? 00:43:00.780 |
And here's something that Michael told me that shocked me. 00:43:05.420 |
Now you might think, oh, fair enough, COVID, guess what? 00:43:09.900 |
So on the rest of the world, investors went like, 00:43:14.100 |
In Australia, which is like not even hit that much by COVID, 00:43:33.020 |
not only are we worse, but we're getting worse, right? 00:43:43.220 |
So in general, tech, our share of the global value added, 00:43:57.340 |
It's plummeting and it's near the very bottom of the OECD. 00:44:10.140 |
that reflect something that I was already seeing. 00:44:12.980 |
So like I kind of caught up Michael and I was like, 00:44:18.260 |
I've got the data to show you what you're seeing. 00:44:21.380 |
This is actually the one that kind of resonated 00:44:32.500 |
They asked, okay, why are you interested in AI? 00:44:47.740 |
this is worse than every other country that they spoke to. 00:44:57.420 |
like if you want to sell to enterprises in Australia, 00:45:06.980 |
and become a global success story, they don't care. 00:45:14.220 |
and it's kind of absolutely true from all of my experience. 00:45:31.540 |
You know, we're smart, we're technical, you know? 00:45:58.300 |
if you can get past all this stuff pulling you down, 00:46:24.020 |
And so when, one of the things that was fascinating 00:46:27.300 |
in San Francisco was that people would say like, 00:46:38.100 |
And so we're paying, you know, I think it was like 00:46:40.620 |
on average one quarter to one fifth of the salaries 00:46:48.220 |
And in Lytic, to get people straight out of undergrad, 00:46:50.540 |
I had to pay them at least 200 grand US, right? 00:47:01.060 |
This is the technology where like people who understand it 00:47:10.380 |
So it's not a bad thing to have in your toolbox 00:47:15.420 |
So it's actually, sadly, it's kind of like this hidden gem. 00:47:22.140 |
And so I've often noticed when kind of VCs come and visit 00:47:37.180 |
even though I'm Australian, I'm looking out for it, 00:47:42.620 |
It's like, you know, even looking at like academic papers, 00:47:53.100 |
that helped me with my work in deep learning. 00:48:00.700 |
it was because they've moved to the Bay Area, you know? 00:48:03.820 |
And I think that's, yeah, I think that's such a waste. 00:48:08.820 |
You know, we have all these brilliant people. 00:48:16.020 |
We've got, you know, technically competent people, 00:48:32.220 |
where deep learning is some key component, you know, 00:48:35.180 |
why wouldn't you be like being at the start of the steam age 00:48:49.100 |
in as un-Australian a way as possible, right? 00:48:54.900 |
It's like, you don't have to have Australian investors. 00:49:00.060 |
Like just believe that you can put something up 00:49:02.060 |
on the internet that people are gonna buy, you know? 00:49:05.940 |
And, you know, don't worry about whether it's mining 00:49:13.500 |
who's never built trained a deep learning model, 00:49:25.020 |
you know, we can have some great startups here. 00:49:30.500 |
And I will say, as that happens, things will change, right? 00:49:40.780 |
So Adelaide has this fantastic AI and machine learning center 00:49:45.780 |
and they're doing something which is almost unheard of 00:49:49.180 |
in universities, which is that they're forging 00:49:51.860 |
really great partnerships with the tech community 00:49:56.860 |
to the point where Amazon is now there too, right? 00:50:02.460 |
we're gonna partner with Adelaide University of Adelaide. 00:50:06.060 |
And so there's now kind of the two centers next door, 00:50:12.340 |
I can't tell you the details, but it happened to know 00:50:14.780 |
lots more big tech companies are now planning 00:50:20.060 |
And so you can imagine what's gonna happen, right? 00:50:22.060 |
Now, lots of people are gonna like go to those 00:50:24.860 |
and then they'll leave and they'll create startups 00:50:28.100 |
and then other big companies who wanna go there. 00:50:32.060 |
what's gonna happen in all the other capitals, 00:50:41.500 |
'Cause universities like here are in many ways 00:50:46.420 |
incredibly anti-entrepreneur, anti-tech entrepreneur. 00:50:55.260 |
a lot of brilliant work gets done out of UQ and QUT. 00:50:58.180 |
They're sponsoring this AI hub, that's fantastic. 00:51:00.700 |
But if an academic there wants to start a startup, 00:51:11.880 |
And let me tell you, that's literally impossible. 00:51:17.660 |
'cause that's like no one will invest in that company 00:51:20.580 |
and the founder can't even be invested in that company. 00:51:24.980 |
this is basically every university in Australia. 00:51:29.700 |
Adelaide made a huge step of going from 70% to 49%. 00:51:39.680 |
where like every academic I know there in engineering 00:51:43.080 |
or computer science has four or five startups, 00:51:47.840 |
You know, half of their students go to those startups. 00:51:50.880 |
Then those students find interesting research directions 00:51:58.280 |
and then they fund a new group of people at the university. 00:52:01.720 |
I mean, if you look at the relationship, for example, 00:52:09.480 |
huge amounts of funding from Google to Stanford, 00:52:12.000 |
lots of job opportunities for standard people at Google. 00:52:14.840 |
The idea that the way you leverage your academic talent 00:52:19.840 |
is by forcing them to give you 70% of their company 00:52:23.800 |
is absolute insanity and it's totally not working. 00:52:28.120 |
And I personally know of many academics in Australia 00:52:34.840 |
And also because most universities will tell you, 00:52:46.600 |
you're learning about actual applied problems, 00:52:55.000 |
with how the kind of tech sector is working here 00:53:01.800 |
but the most important thing is the kind of the raw 00:53:07.080 |
which I think is one of the best in the world. 00:53:09.600 |
And so that's one of the reasons that we came here 00:53:24.520 |
to a glowing diamond that everybody around the world knows. 00:53:35.280 |
- That's awesome to get an insight into your experiences 00:53:41.200 |
over the last, well, since you started your first startup. 00:53:49.480 |
to when you went to US and now when you had your first 00:54:00.440 |
getting money or getting good data to make it all happen? 00:54:05.480 |
- I think if getting good data is the thing you find hard, 00:54:13.240 |
So the thing you're doing should be something 00:54:19.120 |
So like if you're somebody in the legal industry, 00:54:26.800 |
If you're in the HR industry, do an HR startup. 00:54:29.200 |
If you're in the medical field, do a medical startup 00:54:56.840 |
If you noticed, nothing quite works properly. 00:54:59.560 |
Everything's finicky and frustrating and has stupid bits. 00:55:04.080 |
So just particularly stuff at your workplace, 00:55:09.080 |
do you know all the stuff that takes longer than it should 00:55:12.520 |
or problems that have never been solved properly? 00:55:24.960 |
Like one thing I really noticed with fast mail 00:55:29.320 |
it was actually pretty hard to start an email company 00:55:31.400 |
'cause there was very little open source software around 00:55:35.680 |
and very few examples of how to build this kind of thing. 00:55:38.800 |
But very quickly there was kind of like all kinds 00:55:42.880 |
It became pretty easy and we got new competitors monthly 00:55:51.040 |
and then they'd disappear because it'd give up 00:56:18.360 |
When I was young it was obvious what a computer model 00:56:21.200 |
didn't understand, it couldn't recognize a car for example. 00:56:47.800 |
So yeah, I mean, it's a fascinating question. 00:56:49.360 |
I don't think there's any way to ever answer that. 00:56:55.480 |
but I don't know if you're telling the truth. 00:56:57.280 |
You know, it's just a fundamentally impossible question 00:57:03.200 |
We just need to know what can it do, what kind of do. 00:57:33.680 |
which I'm really excited about is I'm planning to do a course 00:57:36.520 |
which is a kind of full stack startup creation course 00:57:40.760 |
involving everything from like creating a Linux server 00:58:04.640 |
'cause of Coursera and it's also getting a bit dated 00:58:12.080 |
It might be 2022, but there's a couple of courses 00:58:28.320 |
I'm suddenly less interested in motorcycling, 00:58:57.360 |
I'd like this to be like a real global hub of brilliance 00:59:02.360 |
because I want people around me to be awesome. 00:59:05.120 |
So I would love it if people were flying here 00:59:12.080 |
in order to be part of this amazing community. 00:59:14.520 |
And I actually think that's totally, totally doable. 00:59:34.080 |
Okay, so how to market an early stage company? 00:59:48.920 |
so there's gotta be a pricing section, right? 00:59:56.400 |
Like, no, I'm not gonna, who does that, right? 01:00:26.960 |
So that's kind of like the first is to avoid anti-marketing, 01:00:30.280 |
where you make life difficult for your customers. 01:00:32.760 |
And then the best kind of marketing is the media, right? 01:00:37.080 |
So like you will get far, far, far more awareness 01:00:40.840 |
of what you're doing if you can get something written 01:00:43.960 |
about it in Wired or the Washington Post or BBC 01:00:51.200 |
And that is all about personal outreach from you, the CEO, 01:00:57.080 |
to journalists who you have carefully researched 01:01:04.600 |
in what you're doing and then telling them about it. 01:01:28.240 |
you'll see that we've got a shitload of media, right? 01:01:33.320 |
I wanted to like go take that to another level 01:01:40.160 |
And so I literally wanted every single person in the world 01:01:46.720 |
So I just wrote to everybody, I talked to everybody 01:01:51.160 |
and ended up on everything from Laura Ingraham on Fox News, 01:01:54.880 |
through to BBC News and wrote in the Washington Post 01:01:59.240 |
And nowadays, thank God people actually wear masks. 01:02:25.760 |
how concerned should we be with the energy usage 01:02:44.000 |
from a general resource constraint point of view, 01:02:58.320 |
Unfortunately, a lot of companies like Google, 01:03:09.040 |
that are very explicitly in center to create research 01:03:12.400 |
that shows the results of using huge amounts of energy. 01:03:15.920 |
Specifically huge amounts of Google Compute Hours. 01:03:21.720 |
because if you can, like journalists love writing 01:03:33.080 |
Now, so the thing is, this is what we focus on, 01:03:37.040 |
the vast majority of problems that we see solved in practice, 01:03:42.880 |
useful pragmatic solutions are solved on a single GPU 01:03:47.880 |
in a few hours and you can buy a GPU for a few hundred bucks. 01:03:54.000 |
And there's all kinds of resources like this, 01:03:56.840 |
as the resource of just like the amount of education 01:03:59.560 |
that you need or the resource of the amount of data 01:04:03.640 |
people dramatically overestimate the amount of resources 01:04:07.960 |
you need to get good results out of deep learning. 01:04:13.600 |
because that's what a lot of people want you to believe. 01:04:24.640 |
that you have to buy lots of their cards or whatever. 01:04:29.320 |
But yeah, overall, there's a massive over emphasis on, 01:04:35.840 |
you know, using vast amounts of stuff in deep learning. 01:04:47.920 |
if I remember correctly, 'cause I kind of skipped over it. 01:04:52.600 |
are passionate about and we went crazy when TPUs came out 01:05:03.680 |
And the media was like, okay, everybody else is screwed now 01:05:16.960 |
that had just come out just shortly after TPUs 01:05:22.160 |
which was basically who can train ImageNet the fastest. 01:05:25.200 |
And at this time, the fastest people were solving it 01:05:30.400 |
When I say solve it, that means getting it to an accuracy, 01:05:33.080 |
like I'm at a top five accuracy of something percent. 01:05:36.360 |
And yeah, not surprisingly, Google put in their pitch 01:05:41.360 |
and I think they got like three hours or something. 01:05:51.440 |
Intel competed and they of course put in an entry 01:05:54.640 |
with 1024 Intel servers operating in parallel. 01:05:58.600 |
And we thought, okay, if these guys win, we're so screwed 01:06:03.200 |
because it's gonna be like, okay, to be good at this, 01:06:07.640 |
So some of our students and me spent basically a week 01:06:23.320 |
and just like, yeah, just keeping things simple. 01:06:33.160 |
because these big tech BMS are always trying to convince you 01:06:36.200 |
that you're not smart enough, that your software 01:06:38.520 |
is not good enough, that your computers are not big enough, 01:06:41.240 |
but it's always been bullshit so far and it always will be. 01:06:59.880 |
we just wanna say thank you for sharing your time. 01:07:02.360 |
Rachel as well, we'll hopefully have you down here 01:07:05.400 |
And really looking forward to having you involved