back to indexNoam Brown: AI vs Humans in Poker and Games of Strategic Negotiation | Lex Fridman Podcast #344
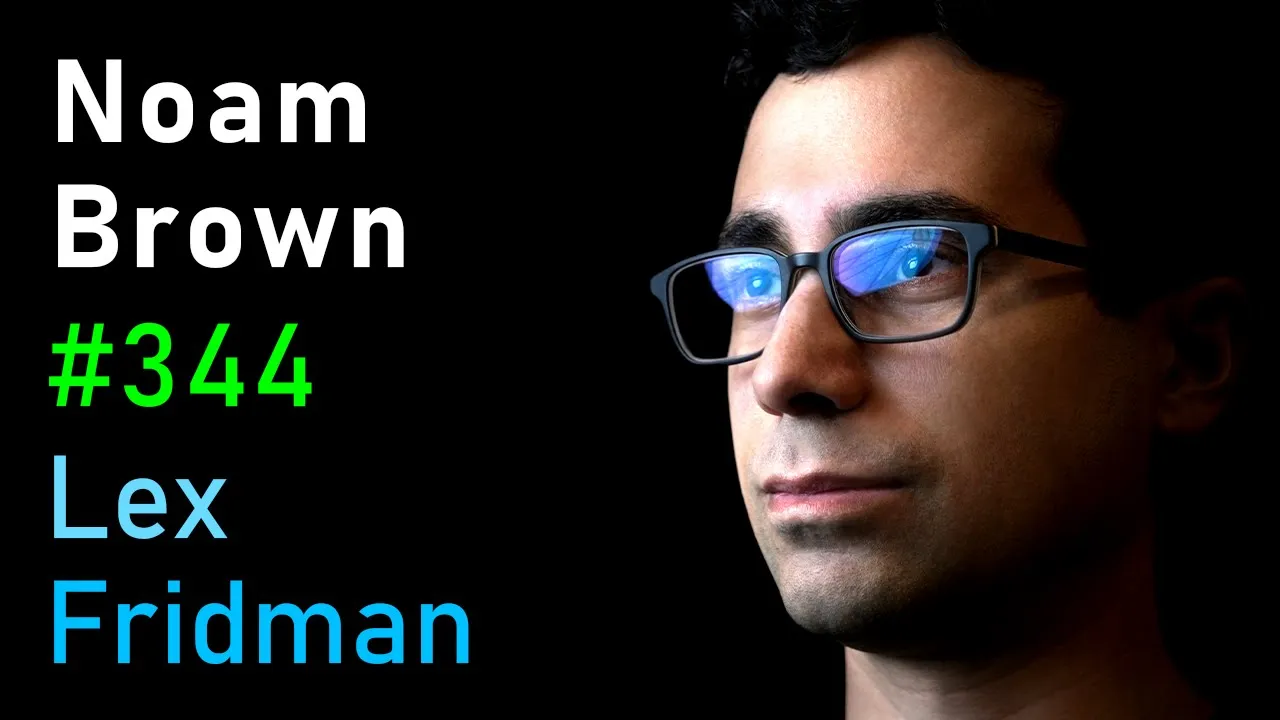
Chapters
0:0 Introduction
1:9 No Limit Texas Hold 'em
5:2 Solving poker
18:12 Poker vs Chess
24:50 AI playing poker
58:18 Heads-up vs Multi-way poker
69:8 Greatest poker player of all time
72:42 Diplomacy game
82:33 AI negotiating with humans
124:58 AI in geopolitics
129:43 Human-like AI for games
135:44 Ethics of AI
139:57 AGI
143:57 Advice to beginners
00:00:01.540 |
oh, this whole idea of game theory, it's just nonsense. 00:00:05.480 |
you got to like look into the other person's eyes 00:00:07.220 |
and read their soul and figure out what cards they have. 00:00:10.640 |
But what happened was where we played our bot 00:00:22.780 |
It was just trying to approximate the Nash equilibrium 00:00:28.660 |
The following is a conversation with Noah Brown, 00:00:40.600 |
in no limit Texas hold them, both heads up and multiplayer. 00:00:55.340 |
which is a war game that emphasizes negotiation. 00:01:02.460 |
Please check out our sponsors in the description. 00:01:08.440 |
You've been a lead on three amazing AI projects. 00:01:22.140 |
You got Pleribus that solved no limit Texas hold them poker 00:01:42.140 |
It was loved by JFK, John F. Kennedy and Henry Kissinger 00:01:46.980 |
and many other big famous people in the decades since. 00:01:52.100 |
So let's talk about poker and diplomacy today. 00:01:54.780 |
First poker, what is the game of no limit Texas hold them? 00:02:02.300 |
is the most popular variant of poker in the world. 00:02:08.420 |
The game that you're playing is no limit Texas hold them. 00:02:20.940 |
in that you can bet any amount of chips that you want. 00:02:26.060 |
You start out with like one or $2 in the pot. 00:02:32.460 |
- So the option to increase the number very aggressively 00:02:36.340 |
- Right, the no limit aspect is there's no limits 00:02:47.900 |
you're always welcome to put $10,000 into the pot. 00:02:50.500 |
- So I've got a chance to hang out with Phil Helmuth 00:02:52.780 |
who plays all these different variants of poker. 00:03:10.420 |
is strategy also rewarded in no limit Texas hold them? 00:03:17.220 |
But I think what's different about no limit hold them 00:03:23.220 |
You know, you go in there thinking you're gonna play 00:03:28.900 |
And suddenly there's like, you know, $1,000 in the pot. 00:03:37.300 |
that's going to maximize your expected value. 00:03:44.820 |
is going to have a material impact on your life, 00:03:49.240 |
then you're gonna play in a more risk averse style. 00:03:53.780 |
you're gonna, if you're playing no limit hold them 00:04:26.380 |
in terms of their ability to reason optimally. 00:04:34.500 |
is to put the other person into an uncomfortable position. 00:04:38.020 |
And if you're doing that, then you're playing poker well. 00:04:40.860 |
And there's a lot of opportunities to do that 00:04:47.860 |
and you know, that's sometimes if you do it right, 00:04:51.020 |
it puts the other person in a really tough spot. 00:04:53.200 |
Now it's also possible that you make huge mistakes that way. 00:04:56.380 |
And so it's really easy to lose a lot of money 00:04:57.980 |
in no limit hold them if you don't know what you're doing. 00:05:04.700 |
that play these games, we'll talk about poker, 00:05:08.620 |
Are you drawn in in part by the beauty of the game itself, 00:05:12.700 |
AI aside, or is it to you primarily a fascinating 00:05:21.840 |
When I started playing poker when I was in high school, 00:05:34.280 |
then you're making unlimited money basically. 00:05:38.220 |
That's like a really fascinating concept to me. 00:05:41.160 |
And so I was fascinated by the strategy of poker, 00:05:49.000 |
- So there was a sense that you can solve poker, 00:05:51.580 |
like in the way you can solve chess, for example, 00:05:54.440 |
or checkers, I believe checkers got solved, right? 00:06:05.800 |
- You could solve chess, you could solve poker. 00:06:08.800 |
- So this gets into the concept of a Nash equilibrium. 00:06:14.200 |
- Okay, so in any finite two-player zero-sum game, 00:06:19.080 |
there is an optimal strategy that if you play it, 00:06:21.960 |
you are guaranteed to not lose an expectation 00:06:26.600 |
And this is kind of a radical concept to a lot of people, 00:06:31.880 |
it's true in any finite two-player zero-sum game. 00:06:39.020 |
In rock, paper, scissors, if you randomly choose 00:06:48.440 |
You're not going to lose an expectation in the long run. 00:06:57.600 |
you are guaranteed to not lose money in the long run. 00:07:00.440 |
And I should say, this is for two-player poker. 00:07:08.240 |
you're guaranteed not to lose in expectation. 00:07:22.480 |
then you are guaranteed to at least break even, 00:07:51.800 |
So I mean, most games that you play are finite in size. 00:08:20.960 |
- Okay, so that's, and then the zero-sum aspect. 00:08:31.760 |
by two-player zero-sum, I mean there's two players, 00:08:34.520 |
and whatever one player wins, the other player loses. 00:09:02.720 |
there's no guarantee that you're going to win 00:09:11.320 |
which is like the pleasure you draw from playing the game? 00:09:15.360 |
And then if you're a professional poker player, 00:09:20.840 |
the money you would get from the attention you get 00:09:27.320 |
is that, that would be a fun thing to model in. 00:09:33.440 |
to include the human factor in its full complexity? 00:09:36.920 |
- I think you bring up a couple of good points there. 00:09:38.480 |
So I think a lot of professional poker players, 00:09:44.640 |
but from the sponsorships and having a personality 00:09:49.520 |
That's a big way to make a name for yourself in poker. 00:09:55.040 |
if you create, and we'll talk about this more, 00:10:02.960 |
that that becomes part of the function to maximize. 00:10:15.480 |
And maybe sometimes you want to be overly aggressive 00:10:24.200 |
is that there's a difference between making an AI 00:10:25.880 |
that wins a game and an AI that's fun to play with. 00:10:33.200 |
- Yeah, and I think, I've heard talks from game designers 00:10:39.880 |
for actual recreational games that people play. 00:10:44.440 |
between trying to make an AI that actually wins. 00:10:49.280 |
the way that the AIs play is not optimal for trying to win. 00:11:03.960 |
who is the creator of "Fallout" and the "Elder Scrolls" series 00:11:10.560 |
And the creator of what I think is the greatest game 00:11:13.160 |
of all time, which is "Skyrim" and the NPCs there. 00:11:15.640 |
The AI that governs that whole game is very interesting, 00:11:20.780 |
And considering what language models might do to NPCs 00:11:33.440 |
of the first applications where we're going to see 00:11:35.420 |
real consumer interaction with large language models. 00:11:38.640 |
I guess "Elder Scrolls VI" is in development now. 00:11:42.720 |
They're probably pretty close to finishing it, 00:11:44.680 |
but I would not be surprised at all if "Elder Scrolls VII" 00:11:48.160 |
was using large language models for their NPCs. 00:11:55.880 |
- No, but they're just releasing the "Starfield" game. 00:12:01.360 |
- And so whatever it is, whenever the date is, 00:12:07.000 |
but it would be, I don't know, like 2024, '25, '26. 00:12:14.240 |
- I was listening to this talk by a gaming executive 00:12:20.840 |
And one of the questions that a person in the audience asked 00:12:27.780 |
And the person responded that it's just so much harder 00:12:30.880 |
to make an AI that can talk with you and cooperate with you 00:12:36.600 |
And I think once this technology develops further 00:12:41.380 |
not every single line of dialogue has to be scripted, 00:12:44.160 |
it unlocks a lot of potential for new kinds of games, 00:12:57.720 |
you'll just be hanging out and like arguing with an AI 00:13:03.720 |
And then you won't be able to sleep that night. 00:13:10.520 |
I mean, yeah, I think that's actually an exciting world. 00:13:15.040 |
Whatever is the drama, the chaos that we love, 00:13:19.480 |
I think it's possible to do that in the video game world. 00:13:24.280 |
and make more mistakes in a video game world, 00:13:35.640 |
it's kind of understood that you're in a not a real world. 00:13:43.880 |
Just like with a game of diplomacy, it's a game. 00:13:49.880 |
So you can have a little bit of fun, a little bit of chaos. 00:14:15.640 |
So it will start playing the game totally randomly, 00:14:21.240 |
it'll eventually get to the end of the game and make $50. 00:14:38.460 |
And because it's playing against a copy of itself, 00:14:40.220 |
it's able to do that counterfactual reasoning. 00:14:42.560 |
So it can say, okay, well, if I took this action 00:14:51.440 |
And so it updates the regret value for that action. 00:14:56.160 |
Regret is basically like how much does it regret 00:15:00.540 |
And when it encounters that same situation again, 00:15:03.740 |
it's going to pick actions that have higher regret 00:15:07.680 |
Now, it'll just keep simulating the games this way. 00:15:10.920 |
It'll keep accumulating regrets for different situations. 00:15:20.760 |
it's proven to converge to a Nash equilibrium. 00:15:36.400 |
- This is counterfactual regret minimization. 00:15:41.680 |
if you follow this kind of process, self-play or not, 00:15:44.520 |
you will be able to arrive at an optimal set of actions. 00:15:53.120 |
that's proven to converge to Nash equilibria, 00:16:04.800 |
the algorithm doesn't have to be as theoretically sound 00:16:14.920 |
the word self-play has mapped to neural networks, 00:16:21.320 |
The self-play mechanism is just the mechanism 00:16:26.960 |
Self-play is not tied specifically to neural nets, 00:16:28.840 |
it's a kind of reinforcement learning, basically. 00:16:32.040 |
And I would also say this process of trying to reason, 00:16:46.120 |
oh, what do you have called me if I raise there? 00:16:49.400 |
That's a person trying to do the same kind of learning 00:16:56.360 |
you're gonna be able to learn an optimal policy. 00:17:01.800 |
I said, okay, if it's in that situation again, 00:17:04.720 |
then it will choose the action that has high regret. 00:17:07.520 |
Now, the problem is that poker is such a huge game. 00:17:21.600 |
- Yeah, I mean, it depends on the number of chips 00:17:24.240 |
but the version that we were playing was 10 to the 161. 00:17:27.080 |
- Which I assume would be a somewhat simplified version 00:17:29.360 |
anyway, 'cause I bet there's some step function 00:17:36.160 |
- Oh, no, no, no, I'm saying we played the full game. 00:17:45.800 |
- Yeah, I mean, 161 plus or minus 10 doesn't matter. 00:17:54.960 |
you know, you don't have to run into the same exact situation 00:17:58.280 |
The odds of you running into the same exact situation 00:18:00.160 |
are pretty slim, but if you run into a similar situation, 00:18:05.120 |
that you've been in that kind of look like that one, 00:18:07.000 |
and you can say like, well, these other situations, 00:18:10.400 |
and so maybe I should play that action here as well. 00:18:22.780 |
- It's like somebody's screaming on Reddit right now. 00:18:43.760 |
but like once you introduce imperfect information, 00:18:50.920 |
maybe you can describe what is seen to the players, 00:18:54.360 |
what is not seen in the game of Texas Hold 'Em. 00:18:59.040 |
you get two cards face down that only you see. 00:19:02.640 |
And so that's the hidden information of the game. 00:19:04.560 |
The other players also all get two cards face down 00:19:08.000 |
And so you have to kind of, as you're playing, 00:19:10.000 |
reason about like, okay, what do they think I have? 00:19:16.320 |
And that's kind of where bluffing comes into play, right? 00:19:22.640 |
the fact that you can bet with a bad hand and still win, 00:19:25.740 |
is because they don't know what your cards are. 00:19:29.680 |
between a perfect information game like poker, 00:19:56.240 |
to estimate the range of hands that they have? 00:20:08.320 |
about why imperfect information makes things difficult, 00:20:14.720 |
but the probability that you're gonna play those actions. 00:20:17.400 |
So you think about rock, paper, scissors, for example, 00:20:21.280 |
rock, paper, scissors is an imperfect information game. 00:20:25.200 |
- Because you don't know what I'm about to throw. 00:20:30.360 |
I'm just gonna throw a rock every single time, 00:20:32.240 |
because the other person's gonna figure that out 00:20:39.640 |
you have to figure out the probability that you play it. 00:20:42.020 |
And really importantly, the value of an action 00:20:45.320 |
depends on the probability that you're gonna play it. 00:21:00.820 |
the value of that action is gonna be really high. 00:21:04.580 |
what that means is the value of bluffing, for example, 00:21:09.100 |
if you're the kind of person that never bluffs 00:21:10.600 |
and you have this reputation as somebody that never bluffs, 00:21:14.480 |
there's a really good chance that that bluff is gonna work 00:21:19.880 |
like if they seen you play for a long time and they see, 00:21:21.720 |
oh, you're the kind of person that's bluffing all the time, 00:21:37.240 |
And you contrast that with a game like chess, 00:21:40.220 |
it doesn't matter if you're opening with the queen's gambit 00:21:48.240 |
So that's why we need these algorithms that understand, 00:21:54.560 |
not just we have to figure out what actions are good, 00:21:57.720 |
we need to get the exact probabilities correct. 00:21:59.600 |
And that's actually when we created the bot Libratus, 00:22:10.920 |
- The balance of how often in the key sort of branching 00:22:23.880 |
but it's not just how often to bluff or not to bluff, 00:22:27.720 |
it's like, how often should you bet in general? 00:22:29.740 |
How often should you, what kind of bet should you make? 00:22:37.720 |
And so this is where the idea of a range comes from, 00:22:40.680 |
because when you're bluffing with a particular hand 00:22:53.560 |
okay, would I also bet with a good hand in this spot? 00:23:04.280 |
- Is there explicit estimation of like a theory of mind 00:23:09.960 |
or is that just a emergent thing that happens? 00:23:34.360 |
So maybe that's jumping ahead to six players, 00:23:53.320 |
Is there a continuation in terms of estimating 00:24:04.500 |
they don't, and the way that humans approach it also, 00:24:07.940 |
the way they approach it is to basically assume 00:24:16.460 |
where even if I were to play it for 10,000 hands 00:24:18.380 |
and you could figure out exactly what it was, 00:24:30.700 |
like I said, I'm still unbeatable in expectation. 00:24:50.660 |
So who's the greatest poker player of all time 00:25:06.100 |
So maybe can you speak from an AI perspective 00:25:32.880 |
Now, I think when somebody like Phil Hellmuth 00:25:54.140 |
They're going to deviate from a Nash equilibrium style 00:26:00.860 |
So you kind of get into the mind games there. 00:26:12.900 |
in the poker community and the academic community. 00:26:19.140 |
game theory optimal poker or exploitative play. 00:26:27.140 |
I think actually exploitative play had the advantage. 00:26:31.140 |
oh, this whole idea of game theory, it's just nonsense. 00:26:35.080 |
you got to like look into the other person's eyes 00:26:36.820 |
and read their soul and figure out what cards they have. 00:26:40.260 |
But what happened was people started adopting 00:26:46.860 |
And they weren't trying to adapt so much to the other player. 00:26:50.140 |
They were just trying to play the Nash equilibrium. 00:26:56.940 |
where we played our bot against four top heads up, 00:27:07.380 |
It was just trying to approximate the Nash equilibrium 00:27:11.540 |
I think, you know, we were playing for $50, $100 blinds. 00:27:39.180 |
from our complex psychology as a human civilization. 00:27:43.340 |
It's emerging from the collective intelligence 00:28:02.180 |
where every year all the different research labs 00:28:04.620 |
that were working on AI for poker would get together. 00:28:09.700 |
And we made a bot that actually won the 2014 competition, 00:28:16.220 |
And so we decided we're gonna take this bot, build on it, 00:28:22.460 |
heads up no limit Texas Hold'em poker players. 00:28:25.020 |
So we invited four of the world's best players 00:28:30.620 |
And we challenged them to 120,000 hands of poker 00:28:40.220 |
where it would basically be divided among them, 00:28:42.060 |
depending on how well they did relative to each other. 00:28:56.660 |
where we also played against professional poker players 00:28:58.940 |
and the bot lost by a pretty sizable margin actually. 00:29:02.080 |
Now there were some big improvements from 2015 to 2017. 00:29:13.900 |
So 2015, it was much more focused on trying to come up 00:29:20.780 |
like trying to solve the entire game of poker, 00:29:25.420 |
where you're saying like, "Oh, I'm in this situation. 00:29:32.820 |
It was trying to say, "Okay, well, let me in real time 00:29:40.940 |
"by playing against myself during self-play." 00:29:50.700 |
There's different actions like raising, calling. 00:29:59.620 |
- So in a game like chess, the search is like, 00:30:09.860 |
is the actions that you can take for your hand, 00:30:12.980 |
the probabilities that you take those actions, 00:30:14.960 |
and then also the probabilities that you take other actions 00:30:19.140 |
And that's kind of like hard to wrap your head around. 00:30:22.980 |
Like, why are you searching over these other hands 00:30:30.740 |
And the idea is, again, you wanna always be balanced 00:30:36.840 |
And so if you're a search algorithm that's saying like, 00:30:41.300 |
Well, in order to know whether that's a good action, 00:30:44.780 |
Let's say you have a bad hand and you're saying like, 00:31:02.060 |
so that action could be mapped by your opponent 00:31:04.220 |
to a lot of different hands, then that's a good action. 00:31:07.220 |
- Basically what you wanna do is put your opponent 00:31:15.900 |
And if you are raising in the appropriate balance 00:31:20.440 |
then you're putting them into that tough spot. 00:31:24.760 |
that would put the opponent into a difficult position. 00:31:26.840 |
- Can you give a metric that you're trying to maximize 00:31:32.120 |
that we're talking about in terms of putting your opponent 00:31:37.380 |
- Yeah, ultimately what you're trying to maximize 00:31:39.200 |
is your expected winnings, your expected value, 00:31:41.740 |
the amount of money that you're gonna walk away from, 00:31:43.660 |
assuming that your opponent was playing optimally 00:32:12.420 |
- So there's not an explicit, like objective function 00:32:15.300 |
that maximizes the toughness of the spot they're put in. 00:32:34.520 |
Now in practice, what that ends up looking like 00:32:36.700 |
is it's putting the opponent into difficult situations 00:32:39.640 |
where there's no obvious decision to be made. 00:32:49.260 |
whenever I was making the other, the opponent sweat. 00:32:51.700 |
Okay, so you're, in 2015, you didn't do as well. 00:33:04.460 |
and we actually learned a lot from that competition. 00:33:09.580 |
is that the way the humans were approaching the game 00:33:11.620 |
was very different from how the bot was approaching the game. 00:33:21.140 |
It would just be playing against itself for months, 00:33:23.180 |
but then when it's actually playing the game, 00:33:26.020 |
And the humans, when they're in a tough spot, 00:33:31.540 |
even like five minutes about whether they're gonna call 00:33:39.720 |
there's a good chance that that's what's missing 00:33:45.020 |
to try to figure out how much of a difference 00:33:57.380 |
It wouldn't try to come up with a better strategy 00:33:59.780 |
in real time over what it had pre-computed during training. 00:34:04.420 |
Whereas the human, like they have all this intuition 00:34:06.580 |
about how to play, but they're also in real time 00:34:22.300 |
You have an intuition and search on top of that, 00:34:39.100 |
But if you can leverage extra computational resources, 00:34:53.660 |
if you do a little bit of search, even just a little bit, 00:35:03.600 |
Like you can kind of think of it as your neural net, 00:35:08.520 |
And it just like blew away all of the research 00:35:11.800 |
and trying to like scale up this like pre-computed solution. 00:35:15.820 |
It was dwarfed by the benefit that we got from search. 00:35:19.740 |
- Can you just linger on what you mean by search here? 00:35:29.020 |
How are you selecting the other hands to search over? 00:35:34.040 |
- No, it's all the other hands that you could have. 00:35:35.780 |
So when you're playing no limit Texas hold 'em, 00:35:39.100 |
And so that's 52 choose two, 1,326 different combinations. 00:35:51.980 |
And so when we're doing, when the bot's doing search, 00:35:56.120 |
there are these thousand different hands that I could have. 00:35:58.300 |
There are these thousand different hands that you could have. 00:36:00.660 |
Let me try to figure out what would be a better strategy 00:36:03.440 |
than what I've pre-computed for these hands and your hands. 00:36:22.040 |
where the train system comes in is the value at the end. 00:36:34.360 |
And at that point you can use the pre-computed solution 00:36:38.800 |
to figure out what's the value here of this strategy. 00:36:42.900 |
- Is it of a single action, essentially in that spot? 00:36:57.860 |
but in the process, in order to maximize the value 00:37:14.200 |
And then we searched all the way to the end of the game. 00:37:23.600 |
So there's the pre-flop, the flop, the turn and the river. 00:37:26.800 |
And so we would start doing search halfway through the game. 00:37:30.920 |
Now the first half of the game, that was all pre-computed. 00:37:37.080 |
then it would always search to the end of the game. 00:37:42.120 |
It would actually search just a few moves ahead. 00:37:45.000 |
But that came later and that drastically reduced 00:37:48.400 |
the amount of computational resources that we needed. 00:37:55.080 |
So like that's where you don't just get one bet 00:37:59.920 |
You can have multiple arbitrary number of bets, right? 00:38:04.600 |
I'm gonna bet and then what are you gonna do in response? 00:38:06.640 |
Are you gonna raise me or are you gonna call? 00:38:12.720 |
up until the end of the game in the case of Libratus. 00:38:15.560 |
- So for Libratus, what's the most number of re-raises 00:38:19.560 |
- You probably cap out at like five or something 00:38:23.760 |
because at that point you're basically all in. 00:38:26.680 |
- I mean, is there like interesting patterns like that 00:38:31.840 |
Like you'll have like alpha zero doing way more sacrifices 00:38:36.920 |
Is there something like Libratus was constantly re-raising 00:38:55.200 |
maybe you bet like $75 or somewhere around there, 00:39:05.120 |
It was actually really easy for us to say like, 00:39:07.560 |
oh, if you want, you can bet like 10 times the pot. 00:39:09.600 |
And we didn't think it would actually do that. 00:39:11.080 |
It was just like, why not give it the option? 00:39:16.680 |
And by the way, this was like a very last minute decision 00:39:19.760 |
And so we did not think the bot would do this. 00:39:25.640 |
when it did start to do this, like, oh, is this a problem? 00:39:29.880 |
But it would put the humans into really difficult spots 00:39:34.720 |
Because you could imagine like you have the second best hand 00:39:42.240 |
And suddenly the bot bets $20,000 into a $1,000 pot. 00:39:53.920 |
like now you get a really tough choice to make. 00:39:56.440 |
And so the humans would sometimes think like five 00:40:03.120 |
And when I saw the humans like really struggling 00:40:06.000 |
with that decision, like that's when I realized like, 00:40:07.400 |
oh, actually this is maybe a good thing to do after all. 00:40:09.760 |
- And of course the system is a no that it's making, 00:40:13.440 |
again, like we said, that it's putting them in a tough spot. 00:40:28.200 |
in a difficult spot, like that's just, you know, 00:40:39.320 |
that the humans walked away from the competition saying like, 00:40:43.800 |
And now these overbets, what are called overbets, 00:40:46.200 |
have become really common in high-level poker play. 00:40:48.760 |
- Have you ever talked to like somebody like Daniel 00:41:04.680 |
when we had dinner together with some other people. 00:41:17.200 |
And he honestly, he wanted to play against the bot. 00:41:20.000 |
He thought he had a decent chance of beating it. 00:41:23.400 |
I think, you know, this was like several years ago 00:41:26.920 |
when I think it was like not as clear to everybody 00:41:36.440 |
there's like no chance that you have in a game like poker. 00:41:41.120 |
The bots have heads up and in other variants too. 00:41:54.320 |
is it true for every single variant of poker? 00:42:00.920 |
they can make an AI that would beat all humans at it. 00:42:08.720 |
And then we followed that up with six player poker as well, 00:42:18.720 |
it's pretty clear that humans don't stand a chance. 00:42:26.560 |
like actually tries to optimize the toughness of the spot 00:42:31.320 |
And I would love to see how different is that 00:42:35.520 |
So you try to maximize the heart rate of the human player, 00:42:39.120 |
like the freaking out over a long period of time. 00:42:42.720 |
I wonder if there's going to be different strategies 00:42:46.480 |
that emerge that are close in terms of effectiveness. 00:43:03.840 |
that it's like a decent proxy for score, right? 00:43:06.760 |
And this is actually like the common poker wisdom 00:43:09.760 |
where they're teaching players, before there were bots, 00:43:12.880 |
and they were trying to teach people how to play poker. 00:43:16.560 |
is to put your opponent into difficult spots. 00:43:27.240 |
And maybe if you can also relate it to chess and go 00:43:31.420 |
what's the role of search to solving these games? 00:43:42.500 |
A lot of people underestimate the importance of search 00:43:48.300 |
An example of this is TD Gammon that came out in 1992. 00:43:52.720 |
This was the first real instance of a neural net 00:43:58.240 |
It was actually the inspiration for AlphaZero 00:44:02.000 |
It used two-ply search to figure out its next move. 00:44:06.320 |
There, it was very heavily focused on search, 00:44:09.960 |
looking many, many moves ahead farther than any human could. 00:44:21.060 |
as a landmark achievement for neural nets, and it is, 00:44:24.780 |
but there's also this huge component of search, 00:44:33.420 |
I think a good example of this is you look at 00:44:55.460 |
AlphaZero, the strongest version, is around 5,200 Elo. 00:44:59.940 |
But if you take out the search that's being done 00:45:02.980 |
at test time, and by the way, what I mean by search 00:45:12.980 |
and you see like what the board state looks like, 00:45:17.220 |
If you take out the search that's done during the game, 00:45:21.860 |
So even today, what, seven years after AlphaGo, 00:45:29.080 |
that's being done at when playing against the human, 00:45:34.040 |
Nobody has made a raw neural net that is superhuman in Go. 00:45:38.300 |
- That's worth lingering on, that's quite profound. 00:45:49.840 |
So having a function that estimates accurately 00:45:58.380 |
what's called a policy network, where it will tell you, 00:46:00.700 |
this is what the neural net thinks is the next best move. 00:46:03.540 |
And it's kind of like the intuition that a human has. 00:46:09.980 |
and any Go or chess master will be able to tell you like, 00:46:14.580 |
oh, instantly, here's what I think the right move is. 00:46:22.620 |
can make a better decision if they have more time to think, 00:46:25.180 |
when you add on this Monte Carlo Tree Search, 00:46:30.460 |
- Yeah, I mean, of course a human is doing something 00:46:41.700 |
It's more like sequential language model generation. 00:46:50.820 |
I wonder what the human brain is doing in terms of searching 00:46:58.980 |
they have a really strong ability to estimate, 00:47:08.300 |
But they're still doing search in their head, 00:47:22.020 |
and I think it's a really important question. 00:47:38.860 |
but it isn't actually like full on neural net. 00:47:46.180 |
in these kinds of like perfect information board games 00:47:50.060 |
But if you take it to a game like poker, for example, 00:47:52.900 |
It can't understand the concept of hidden information. 00:47:56.660 |
It doesn't understand the balance that you have to strike 00:48:08.700 |
for all these different games in a very general way. 00:48:14.380 |
And I think it's a really important missing piece. 00:48:16.260 |
The ability to plan and reason more generally 00:48:26.380 |
makes you better at each one of the games, not worse. 00:48:32.820 |
they'll give you like Transformers, for example, 00:48:37.620 |
it'll output an answer in like 100 milliseconds. 00:48:41.380 |
"Oh, you've got five minutes to give me a decision, 00:48:43.420 |
feel free to take more time to make a better decision." 00:48:47.300 |
But a human, if you're playing a game like chess, 00:48:50.700 |
they're gonna give you a very different answer 00:49:00.020 |
Transformers language models in an iterative way 00:49:12.460 |
- Yeah, and I think it's a good step in the right direction. 00:49:17.740 |
to Monte Carlo rollouts in a game like chess. 00:49:23.060 |
"I'm gonna roll out my intuition and see like, 00:49:30.740 |
What would I do if I just acted according to intuition 00:49:36.980 |
but I think that there's much richer kinds of planning 00:49:42.140 |
- So when Labradors actually beat the poker players, 00:49:51.700 |
I mean, poker was one of the games that you thought 00:50:24.420 |
Like what programming languages is it written in? 00:50:26.860 |
What's some interesting implementation details 00:50:33.340 |
- Yeah, so one of the interesting things about Labradors 00:50:41.500 |
and that kind of gives us some sense of like, 00:50:45.900 |
But we had no idea like what the bar actually was. 00:50:50.900 |
at trying to make the strongest bot possible. 00:51:06.500 |
- Well, it's still a lot for even any grad student today. 00:51:15.180 |
in terms of scale at CMU, at MIT, anything like that. 00:51:18.900 |
- Yeah, and talking about terabytes of memory. 00:51:25.620 |
because the more games that you could simulate, 00:51:40.180 |
- There were all sorts of optimizations that I had to make 00:51:42.460 |
to try to get this thing to run as fast as possible. 00:51:44.500 |
They were like, how do you minimize the latency? 00:51:46.620 |
How do you like, you know, package things together 00:51:48.860 |
so that like you minimize the amount of communication 00:52:17.900 |
- It's the community, basically converged on. 00:52:25.340 |
and then you show up to the day of competition. 00:52:42.820 |
in a totally normal style, I think we'll squeak out a win. 00:52:49.820 |
And if they do, and we're playing like for 20 days, 00:52:53.660 |
They have a lot of time to find weaknesses in the system. 00:53:03.300 |
it wasn't like they were winning from the start. 00:53:09.900 |
they were just crushing the bot, stealing money from it. 00:53:31.820 |
it wasn't able to, because it wasn't doing search, 00:53:46.180 |
And in some situations that really matters a lot. 00:53:48.180 |
And so they could put the bot into those situations 00:53:54.100 |
Okay, so I didn't realize it was over 20 days. 00:53:57.500 |
So what were the humans like over those 20 days? 00:54:04.460 |
- So we had set up the competition, you know, 00:54:06.700 |
like I said, there was $200,000 in prize money 00:54:12.100 |
depending on how well they did relative to each other. 00:54:14.380 |
So I was kind of hoping that they wouldn't work together 00:54:20.220 |
with their like number one objective being to beat the bot. 00:54:22.700 |
And they didn't care about like individual glory. 00:54:24.900 |
They were like, we're all gonna work as a team 00:54:28.100 |
And so they immediately started comparing notes. 00:54:50.380 |
and I'm not sure why we did that in retrospect, 00:54:58.900 |
I mean, to know, usually when you play poker, 00:55:01.220 |
you see about a third of the hands to show down 00:55:14.700 |
could they find patterns in the bot, weaknesses? 00:55:32.540 |
- I mean, I'm sure you didn't think of it that way, 00:55:46.580 |
When we actually, when we announced the competition, 00:55:49.620 |
the poker community decided to gamble on who would win. 00:55:52.820 |
And their initial odds against us were like four to one. 00:55:58.460 |
The bot ended up winning for three days straight. 00:56:06.660 |
And then at that point, it started to look like 00:56:19.500 |
they thought that they spotted some weaknesses 00:56:27.300 |
And from that point, I mean, for a while there, 00:56:35.460 |
and now we're just gonna lose the whole thing. 00:56:37.340 |
But no, it ended up going in the other direction 00:56:39.620 |
and the bot ended up like crushing them in the long run. 00:56:51.580 |
and as a person who appreciates the beauty of AI, 00:56:55.500 |
is there, did you feel a certain kind of way about it? 00:57:03.660 |
I had spent five years working on this project 00:57:09.580 |
I mean, to spend five years working on something 00:57:12.780 |
Yeah, I wouldn't trade that for anything in the world. 00:57:18.020 |
It's not like getting some percent accuracy on a data set. 00:57:30.700 |
And this is humans doing their best to beat the machine. 00:57:33.420 |
So this is a real benchmark, unlike anything else. 00:57:36.460 |
- Yeah, and I mean, this is what I had been dreaming about 00:57:48.060 |
be able to beat all the poker players in the world with it. 00:57:51.420 |
So to actually see that come to fruition and be realized, 00:58:05.780 |
that's why you want to look at betting markets 00:58:08.100 |
if you want to actually understand what people really think. 00:58:11.500 |
And in the same sense, poker, it's really high stakes 00:58:15.580 |
And to solve that game, that's an amazing accomplishment. 00:58:18.820 |
So the leap from that to multi-way six player poker, 00:58:34.100 |
Nash equilibrium in two player zero-sum games. 00:58:38.180 |
you are guaranteed to not lose an expectation 00:58:43.340 |
you're no longer playing a two player zero-sum game. 00:58:46.580 |
among the academic community and among the poker community 00:59:08.820 |
in practice, it still gives you a really strong strategy. 00:59:17.860 |
I mean, for one, the game is just exponentially larger. 00:59:29.260 |
So I said before, like, you know, we would do search, 00:59:39.380 |
extending all the way to the end of the game. 00:59:41.340 |
So it would have to start from the turn onwards, 01:00:03.060 |
and then stopping there and substituting a value estimate 01:00:06.140 |
of like how good is that strategy at that point, 01:00:08.700 |
then we're able to do a much more scalable form of search. 01:00:17.060 |
is there something cool in the paper in terms of graphics? 01:00:24.020 |
- Figure one, an example of equilibrium selection problem. 01:00:34.780 |
- So when you go outside of two players, you're a sum. 01:00:38.120 |
So a Nash equilibrium is a set of strategies, 01:00:48.300 |
imagine you have a game where there's a ring. 01:00:55.320 |
is to be as far away from the other players as possible. 01:00:58.780 |
There's a Nash equilibrium is for all the players 01:01:04.580 |
But there's infinitely many different Nash equilibria. 01:01:16.220 |
then there's no guarantee that the joint strategy 01:01:18.900 |
that they're all playing is going to be a Nash equilibrium. 01:01:32.940 |
to do the selection of the equilibria you're chasing? 01:01:37.620 |
So is there like a meta problem to be solved here? 01:01:51.420 |
there's no guarantee that you're going to win. 01:01:58.140 |
and all the other players decide to team up against you, 01:02:06.420 |
whether Nash equilibrium and all these techniques 01:02:10.460 |
once you go outside of two player zero-sum games. 01:02:30.520 |
because six player poker is such an adversarial game, 01:02:38.160 |
the techniques that were used in two player poker 01:02:45.340 |
- There's some deep way in which six player poker 01:02:49.200 |
is just a bunch of heads up poker, like games in one. 01:02:55.540 |
So the competitiveness is more fundamental to poker 01:03:05.280 |
In fact, you're not even allowed to cooperate in poker. 01:03:28.760 |
where approximating an equilibrium through self-play 01:03:40.900 |
So there are these kinds of games called potential games, 01:03:49.480 |
where this approach to approximating an equilibrium 01:03:57.920 |
but it is possible that there is some classic games 01:04:04.200 |
- So what are some interesting things about Pleribus 01:04:08.180 |
that was able to achieve human level performance 01:04:16.180 |
- Personally, I think the most interesting thing 01:04:18.300 |
about Pleribus is that it was so much cheaper than Libratus. 01:04:22.780 |
I mean, Libratus, if you had to put a price tag 01:04:25.540 |
on the computational resources that went into it, 01:04:27.740 |
I would say the final training run took about $100,000. 01:04:37.940 |
- Is this normalized to computational inflation? 01:04:41.540 |
So meaning, does this just have to do with the fact 01:04:51.920 |
computing resources are getting cheaper every day, 01:04:55.000 |
but you're not gonna see a thousand fold decrease 01:04:57.680 |
in the computational resources over two years 01:05:02.060 |
The real improvement was algorithmic improvements 01:05:04.720 |
and in particular, the ability to do depth limited search. 01:05:08.440 |
- So does depth limited search also work for Libratus? 01:05:13.260 |
So where this depth limited search came from is, 01:05:21.080 |
and that reduced the computational resources needed 01:05:31.600 |
- What do you learn from that, from that discovery? 01:05:38.080 |
is that algorithmic improvements really do matter. 01:05:40.200 |
- How would you describe the more general case 01:05:45.200 |
So it's basically constraining the scale, temporal, 01:05:48.120 |
or in some other way of the computation you're doing, 01:05:53.280 |
So like with, like how else can you significantly 01:05:59.640 |
- Well, I think the idea is that we want to be able 01:06:04.160 |
And the way that we were doing it in Libratus 01:06:05.960 |
required us to search all the way to the end of the game. 01:06:11.440 |
to the end of the game is kind of unimaginable, right? 01:06:15.360 |
where you just won't be able to use search in that case 01:06:20.960 |
And this technique allowed us to leverage search 01:06:36.600 |
And more generally, what role do neural nets have to play 01:06:44.640 |
- So we actually did not use neural nets at all 01:06:49.220 |
And a lot of people found this surprising back in 2017. 01:06:55.440 |
that we were able to do this without using any neural nets. 01:07:01.360 |
I mean, I think neural nets are incredibly powerful 01:07:06.840 |
even for poker AIs, do rely quite heavily on neural nets. 01:07:14.160 |
Like I think what neural nets are really good for, 01:07:17.320 |
if you're in a situation where finding features 01:07:30.600 |
was that nobody had a good way of looking at a board 01:07:44.680 |
of different board positions into this neural net, 01:07:49.800 |
But in poker, the features weren't the challenge. 01:07:53.400 |
The challenge was how do you design a scalable algorithm 01:07:57.640 |
that would allow you to find this balanced strategy 01:08:10.040 |
The complexity of poker that you've described? 01:08:14.860 |
- Yeah, so the way the value functions work in poker, 01:08:19.260 |
they do use neural nets for the value function. 01:08:24.380 |
from how it's done in a game like chess or Go, 01:08:26.360 |
because in poker, you have to reason about beliefs. 01:08:31.100 |
And so the value of a state depends on the beliefs 01:08:35.600 |
that players have about what the different cards are. 01:08:41.700 |
then whether that's a really, really good hand 01:08:44.460 |
or just an okay hand depends on whether you know 01:08:50.580 |
then if I bet, you're gonna fold immediately. 01:08:53.420 |
But if you think that I have a really bad hand, 01:08:55.720 |
then I could bet with pocket aces and make a ton of money. 01:09:05.100 |
which is very different from how chess and Go AIs work. 01:09:13.700 |
who do you think is the greatest poker player of all time? 01:09:20.900 |
Can you actually analyze the quality of play? 01:09:34.060 |
is there an Elo rating type of system for poker? 01:09:37.700 |
I suppose you could, but there's just not enough. 01:09:41.220 |
You would have to play a lot of games, right? 01:09:46.380 |
The deterministic game makes it easier to estimate Elo. 01:09:55.320 |
The problem is that the game is very high variance. 01:10:05.260 |
I mean, you've got top professional poker players 01:10:12.300 |
- So for Elo, you have to have a nice clean way of saying 01:10:31.540 |
But the same way that AIs have now taken over chess 01:10:35.180 |
and all the top professional chess players train with AIs, 01:10:49.900 |
try to learn from the AIs to improve their strategy. 01:10:52.980 |
So now, yeah, so the game has been revolutionized 01:10:57.740 |
in the past five years by the development of AI 01:11:01.020 |
- The skill with which you avoided the question 01:11:05.180 |
- So my feeling is that it's a difficult question 01:11:10.620 |
where you can't really compare Magnus Carlsen today 01:11:13.220 |
to Garry Kasparov, because the game has evolved so much. 01:11:17.260 |
The poker players today are so far beyond the skills 01:11:23.180 |
of people that were playing even 10 or 20 years ago. 01:11:27.420 |
So you look at the kinds of all-stars that were on ESPN 01:11:33.260 |
pretty much all those players are actually not that good 01:11:35.540 |
at the game today, at least the strategy aspect. 01:11:39.380 |
I mean, they might still be good at reading the player 01:11:42.180 |
at the other side of the table and trying to figure out 01:11:45.620 |
But in terms of the actual computational strategy 01:11:48.340 |
of the game, a lot of them have really struggled 01:11:58.140 |
who you actually had on the podcast recently, 01:12:15.180 |
- So he is trying to, he's constantly studying 01:12:20.900 |
And I think a lot of the old school poker players 01:12:45.260 |
What is at a high level the game of diplomacy? 01:12:54.340 |
is that it's very different from these adversarial games 01:12:59.500 |
like chess, go, poker, even Starcraft and Dota. 01:13:02.820 |
Diplomacy has a much bigger cooperative element to it. 01:13:13.700 |
It's like a map of Europe with seven great powers 01:13:16.780 |
and they're all trying to form alliances with each other. 01:13:25.540 |
is on forming alliances with the other players 01:13:53.040 |
Because the only way that you can make progress 01:13:54.660 |
is by working with somebody else against the others. 01:13:58.740 |
And then after that negotiation period is done, 01:14:01.300 |
all the players simultaneously submit their moves 01:14:22.780 |
you're actually saying phrases that are structured? 01:14:25.920 |
- So there's different ways to play the game. 01:14:34.500 |
that you can make, the kinds of things that you can discuss. 01:14:41.660 |
You can play it live online or over voice chat. 01:14:46.580 |
But the focus, the important thing to understand 01:14:52.980 |
You can make any sorts of deals that you want 01:14:56.940 |
So it's not like you're all around the board together 01:15:01.620 |
You're grabbing somebody going off into a corner 01:15:07.020 |
- And there's no limit in theory to the conversation 01:15:16.300 |
"Hey, let's have a long-term alliance against this guy." 01:15:20.020 |
And in return, I'll do this other thing for you next turn." 01:15:24.720 |
just you can talk about like what you talked about 01:15:32.060 |
is that it's kind of like a mix between Risk, 01:15:37.620 |
There's like this big element of like trying to, 01:15:43.680 |
And the best way that I would describe the game 01:15:54.900 |
Poker, because there's a game theory component 01:16:04.200 |
And then Survivor, because of the social component, 01:16:21.360 |
like really imagine yourself as the leader of France 01:16:28.320 |
It's actually fun to really lean into being that leader. 01:16:34.700 |
where they just like kind of view it as a strategy game, 01:16:37.060 |
but also a role-playing game where they can like act out, 01:16:39.100 |
like, what would I be like if I was, you know, 01:16:45.500 |
And they sometimes use like the old-timey language 01:16:54.960 |
Anyway, so what are the different turns of the game? 01:17:04.200 |
So you start out controlling like just a few units 01:17:07.480 |
and the object of the game is to gain control 01:17:10.560 |
If you're able to do that, then you've won the game. 01:17:13.120 |
But like I said, the only way that you're able to do that 01:17:16.840 |
So on every turn, you can issue a move order. 01:17:26.340 |
or you can support a move or a hold of a different unit. 01:17:34.300 |
- It's kind of like risk where the map is divided up 01:17:42.460 |
if you're moving into that territory with more supports 01:17:47.000 |
or the person that's trying to move in there. 01:17:48.900 |
So if you're moving in and there's somebody already there, 01:17:51.660 |
then if neither of you have support, it's a one versus one 01:17:54.380 |
and you'll bounce back and neither of you will make progress. 01:17:58.620 |
that move into the territory, then it's a two versus one 01:18:10.060 |
this unit is supporting this other unit into this territory. 01:18:19.460 |
because you can support your own units into territory, 01:18:22.340 |
but you can also support other people's units 01:18:25.300 |
And so that's what the negotiations really revolve around. 01:18:41.020 |
- That tension is absolutely core to the game. 01:18:43.420 |
The fact that you can make all sorts of promises, 01:18:46.640 |
but you have to reason about the fact that like, 01:18:54.140 |
when they say that they're gonna support you. 01:19:01.360 |
Is it true that Henry Kissinger loved the game 01:19:05.300 |
I've heard like a bunch of different people that, 01:19:23.880 |
- It's interesting that they went with World War I 01:19:29.600 |
- So the story that I've heard for the creation of the game 01:19:32.620 |
is it was created by somebody that had looked at 01:19:39.200 |
and they saw World War I as a failure of diplomacy. 01:19:52.640 |
that would basically teach people about diplomacy. 01:19:55.140 |
And it's really fascinating that in his ideal version 01:20:00.900 |
of the game of diplomacy, nobody actually wins the game. 01:20:03.640 |
Because the whole point is that if somebody is about to win, 01:20:05.880 |
then the other players should be able to work together 01:20:13.800 |
And it's kind of has a nice like wholesome take home message 01:20:32.580 |
which is more powerful, Russia versus Germany 01:20:38.100 |
- So I think the general consensus is that France 01:20:45.860 |
So the fact that France has an inherited advantage 01:20:48.380 |
from the beginning means that the other players 01:20:59.840 |
while all the other players start with three. 01:21:02.040 |
But Russia is also in a much more vulnerable position 01:21:13.040 |
Okay, what else is important to know about the rules? 01:21:25.440 |
- Usually the game lasts, I would say about 15 or 20 turns. 01:21:34.280 |
I mean, if you're playing a house game with friends, 01:21:35.880 |
at some point you just get tired and you all agree like, 01:21:37.880 |
okay, we're gonna end the game here and call it a draw. 01:21:41.320 |
If you're playing online, there's usually like set limits 01:21:45.000 |
- And what's the end, what's the termination condition? 01:21:47.600 |
Like, does one country have to conquer everything else? 01:21:52.600 |
- So if somebody is able to actually gain control 01:21:55.200 |
of a majority of the map, then they've won the game. 01:22:01.480 |
especially with strong players, because like I said, 01:22:03.320 |
the game is designed to incentivize the other players 01:22:09.640 |
Usually what ends up happening is that, you know, 01:22:12.800 |
all the players agree to a draw and then the score, 01:22:16.640 |
the win is divided among the remaining players. 01:22:21.760 |
The one that we used in our research basically gives 01:22:26.400 |
a score relative to how much control you have of the map. 01:22:29.800 |
So the more that you control, the higher your score. 01:22:39.720 |
- Yeah, so people have been working on AI for diplomacy 01:22:44.360 |
There was some really exciting research back then, 01:22:47.640 |
but the approach that was taken was very different 01:22:51.600 |
I mean, the research in the '80s was a very rule-based 01:22:56.160 |
It was very in line with the kind of research 01:22:59.520 |
You know, basically trying to encode human knowledge 01:23:08.600 |
and so much more complicated than the kinds of games 01:23:12.080 |
that people were working on like chess and go and poker 01:23:33.640 |
First of all, you have the natural language components. 01:23:49.760 |
Your action space is basically all the different sentences 01:23:53.520 |
that you could communicate to somebody else in this game. 01:23:59.400 |
So is part of it like the ambiguity in the language? 01:24:07.920 |
if you narrowed the set of possible sentences 01:24:12.720 |
- The real difficulty is the breadth of things 01:24:26.920 |
are basically like, am I trading you two sheep for a wood 01:24:33.560 |
the breadth of conversations that you're going to have 01:24:45.240 |
They're lying because they told this other person 01:25:12.640 |
But ultimately we thought the most impactful way 01:25:21.360 |
and just try to go for the full game upfront. 01:25:27.880 |
Greetings England, this should prove to be a fun game 01:25:41.840 |
- Yeah, that's just kind of like the generic greetings 01:25:44.400 |
I think that the meat comes a little bit later 01:25:58.880 |
So that kind of stuff, making friends, making enemies. 01:26:02.480 |
- Yeah, or like if you look at the next line, 01:26:05.040 |
I've heard bits about a Lepanto and an octopus opening 01:26:25.200 |
It's hard for us humans, but for AI it's even harder 01:26:27.920 |
'cause you have to understand like at every level 01:26:31.800 |
- Right, I mean, there's the complexity in understanding 01:26:34.240 |
when somebody is saying this to me, what does that mean? 01:26:36.640 |
And then there's also the complexity of like, 01:26:43.520 |
hey, you might be getting attacked by this other power? 01:26:46.720 |
- Okay, so how are we supposed to think about? 01:26:54.160 |
How do you even begin trying to solve this game? 01:27:00.120 |
- Yeah, and I mean, there's the natural language aspect. 01:27:02.280 |
And then even besides the natural language aspect, 01:27:04.320 |
you also have the cooperative elements of the game. 01:27:11.160 |
If you look at all the previous game AI breakthroughs, 01:27:15.240 |
they've all happened in these purely adversarial games 01:27:27.680 |
Starcraft, Dota 2, like in some of those cases, 01:27:31.280 |
they leveraged human data, but they never needed to. 01:27:33.840 |
They were always just trying to have a scalable algorithm 01:27:38.520 |
that then they could throw a lot of computational resources 01:27:41.720 |
at a lot of memory at, and then eventually it would converge 01:27:47.760 |
This perfect strategy that in a two player zero-sum game 01:27:55.840 |
- So you can't leverage self-play to solve this game. 01:27:59.840 |
but it's no longer sufficient to beat humans. 01:28:02.760 |
- So how do you integrate the human into the loop of this? 01:28:05.240 |
- So what you have to do is incorporate human data. 01:28:12.000 |
like imagine you're playing a negotiation game, 01:28:13.800 |
like diplomacy, but you're training completely from scratch 01:28:19.920 |
The AI is not going to suddenly like figure out 01:28:24.360 |
It's going to figure out some weird robot language 01:28:31.960 |
they're going to think this person's talking gibberish 01:28:34.320 |
and they're just going to ally with each other 01:28:39.880 |
And so in order to be able to play this game with humans, 01:28:43.640 |
it has to understand the human way of playing the game, 01:28:58.440 |
doesn't need to play like a human to beat a human. 01:29:07.000 |
so that you can understand how to work with them. 01:29:13.160 |
What does it mean to have like a reciprocal relationship 01:29:20.880 |
they're just going to work with somebody else instead. 01:29:26.360 |
in some deep sense of the spirit of the Turing test 01:29:32.460 |
this is what the Turing test actually looks like? 01:29:35.060 |
- So, because of open-ended natural language conversation 01:29:47.760 |
that seems like how you actually perform the Turing test. 01:29:51.960 |
- I think it's different from the Turing test. 01:29:53.720 |
Like the way that the Turing test is formulated, 01:29:55.880 |
it's about trying to distinguish a human from a machine 01:29:59.120 |
and seeing, oh, could the machine successfully pass 01:30:08.960 |
Whereas in diplomacy, it's not about trying to figure out 01:30:14.400 |
It's ultimately about whether I can work with this player 01:30:17.560 |
regardless of whether they are a human or a machine. 01:30:19.880 |
And can the machine do that better than a human can? 01:30:26.100 |
but that just feels like the implied requirement for that 01:30:35.880 |
that if you're going to play in this human game, 01:30:39.080 |
you have to somehow adapt to the human surroundings 01:31:10.560 |
I was wrapping up the work on six-player poker on Pluribus 01:31:15.320 |
and was trying to think about what to work on next. 01:31:17.880 |
And I had been seeing all these other breakthroughs 01:31:24.600 |
you have AlphaStar beating humans in StarCraft, 01:31:26.920 |
you've got the Dota 2 stuff happening at OpenAI, 01:31:39.400 |
And people were throwing out these other games 01:31:42.440 |
about what should be the next challenge for multi-agent AI. 01:31:50.060 |
If you look at a game like chess or a game like Go, 01:31:56.320 |
to ultimately reach superhuman performance at. 01:32:14.920 |
But we felt like that was a goal worth aiming for. 01:32:26.120 |
But I was talking to a coworker of mine, Adam Lear, 01:32:31.480 |
"We'll learn some interesting things along the way 01:32:38.880 |
considering just how much progress there was in AI 01:32:43.000 |
and that progress has continued in the years since. 01:32:45.480 |
- So winning in diplomacy, what does that really look like? 01:33:08.400 |
- Ultimately, the problem is simple to quantify, right? 01:33:14.120 |
Like you're going to play this game with humans 01:33:41.980 |
And so not like number one, but way, way higher than- 01:33:49.580 |
Are they intermediate players, advanced players? 01:33:55.940 |
how do you measure the performance in diplomacy? 01:33:58.060 |
And I would argue that when you're measuring performance 01:34:05.760 |
It's kind of like if you're developing a self-driving car, 01:34:08.580 |
you don't want to measure that car on the road 01:34:13.400 |
You want to put it on a road of like an actual American city 01:34:24.080 |
We're saying like, we're going to stick this game, 01:34:33.500 |
or expert human player would in the same situation? 01:34:47.780 |
I think they're more predictable in the quality of play. 01:34:51.020 |
The space of strategies you're operating under 01:34:57.600 |
It's really frustrating to go against beginners. 01:35:07.100 |
But yeah, the variance in strategies is greater, 01:35:24.780 |
is when they're playing with these weak players. 01:35:30.520 |
that they won't be able to pull off a stab as well, 01:35:45.080 |
that there are some weak players in the game. 01:35:47.520 |
- Okay, so if you have to incorporate human play data, 01:35:55.960 |
- Yeah, so that's really the crux of the problem. 01:36:06.180 |
while keeping the strategy as human compatible as possible? 01:36:10.800 |
And so what we did is we first trained a language model, 01:36:14.760 |
and then we made that language model controllable 01:36:21.560 |
which are basically like an action that we want to play 01:36:24.160 |
and an action that we would like the other player to play. 01:36:27.160 |
And so this gives us a way to generate dialogue 01:36:29.400 |
that's not just trying to imitate the human style, 01:36:31.880 |
whatever a human would say in this situation, 01:36:45.560 |
that we're discussing comes from a strategic reasoning model 01:36:50.640 |
that uses reinforcement learning and planning. 01:36:53.000 |
- So the computing the intents for all the players, 01:37:06.840 |
- It's a combination of reinforcement learning and planning. 01:37:11.240 |
Actually very similar to how we approached poker 01:37:14.280 |
and how people have approached chess and Go as well. 01:37:18.200 |
We're using self-play and search to try to figure out 01:37:26.920 |
that we would like this other player to play. 01:37:28.920 |
Now, the difference between the way that we approached 01:37:32.960 |
reinforcement learning and search in this game 01:37:48.160 |
in a way that maximize the chance of following the intent 01:38:03.160 |
So we're coming up with this plan for the action 01:38:07.640 |
that we're gonna play and the other person's gonna play 01:38:09.120 |
and then we feed that action into the dialogue model 01:38:11.800 |
that will then send a message according to those plans. 01:38:14.120 |
- So the language model there is mapping action to... 01:38:27.360 |
like here are the actions that you should be discussing. 01:38:43.840 |
Oh man, the number of ways it probably goes horribly, 01:38:47.480 |
I would have imagined it goes horribly wrong. 01:38:54.000 |
- I mean, there are a lot of ways that this could fail. 01:38:55.880 |
So for example, I mean, you could have a situation 01:39:09.160 |
And so like, let's say you're about to attack somebody, 01:39:17.920 |
So it doesn't really have a way of knowing like, 01:39:24.240 |
So we have to like develop a lot of other techniques 01:39:31.120 |
is we try to calculate if I'm going to send this message, 01:39:34.660 |
what would I expect the other person to do in response? 01:39:54.760 |
- So you have for particular kinds of messages, 01:39:59.520 |
that does the, estimates the value of that message. 01:40:03.200 |
- Yeah, so we have these kinds of filters that like- 01:40:06.600 |
So there's a good, is that filter in your network 01:40:15.120 |
It's a neural network, but it's also using planning. 01:40:20.040 |
what is the policy that the other players are going to play 01:40:26.120 |
And then is that better than not sending the message? 01:40:30.560 |
Like there's a language model that generates random crap 01:40:43.400 |
I'll think of something and it's hilarious to me. 01:41:02.080 |
an intent that you want your opponent to achieve, 01:41:12.880 |
- Yeah, and we're filtering for several things. 01:41:15.280 |
We're filtering like, is this a sensible message? 01:41:19.720 |
will generate messages that are just like totally nonsense. 01:41:25.140 |
We also try to filter out messages that are basically lies. 01:41:51.120 |
would make the bot perform worse in the long run. 01:41:59.660 |
And trust is a huge aspect of the game of diplomacy. 01:42:02.920 |
'cause I think this applies to life lessons too. 01:42:06.960 |
- Oh, I think it's a really, yeah, really strong- 01:42:15.000 |
- Yeah, I mean, I think when people play diplomacy 01:42:18.280 |
they approach it as a game of deception and lying. 01:42:21.120 |
And they, ultimately, if you talk to top diplomacy players, 01:42:28.200 |
and being able to build trust in an environment 01:42:38.320 |
about whether you are being honest in your communication? 01:42:41.040 |
And how can the AI persuade you that it is being honest 01:42:45.400 |
"Hey, I'm actually going to support you this turn." 01:43:03.920 |
what are the fundamental aspects of forming trust 01:43:10.000 |
I mean, that's a really, really important question 01:43:15.960 |
that's fundamental to the human-robot interaction problem. 01:43:19.520 |
How do we form trust between intelligent entities? 01:43:23.840 |
- So one of the things I'm really excited about 01:43:40.580 |
a bunch of mechanical Turkers that are being paid 01:43:43.660 |
and trying to get through the task as quickly as possible. 01:43:47.060 |
You have these people that are really invested 01:43:49.860 |
and they're really trying to do the best that they can. 01:43:52.980 |
And so I'm really excited that we're able to, 01:44:07.580 |
so that they can investigate these kinds of questions. 01:44:16.660 |
for the generation of the messages and the filtering. 01:44:38.220 |
- We should say, what is the name of the system? 01:44:43.180 |
And what's the name, like you're open sourcing, 01:44:45.580 |
what's the name of the repository and the project? 01:44:49.140 |
Is it also just called Cicero the big project? 01:44:53.300 |
- The data set comes from this website, webdiplomacy.net, 01:44:56.820 |
is this site that's been online for like 20 years now. 01:45:11.180 |
So it's a pretty massive data set that people can use to, 01:45:18.540 |
for all sorts of interesting research questions. 01:45:28.060 |
to explore this kind of human AI interaction? 01:45:37.420 |
to investigate these kinds of questions of negotiation, 01:45:44.220 |
I wouldn't say it's the best data set in the world 01:45:45.900 |
for human AI interaction, that's a very broad field. 01:45:49.660 |
But I think that it's definitely up there as like, 01:45:52.100 |
if you're really interested in language models 01:45:57.260 |
where their incentives are not fully aligned, 01:45:59.660 |
this seems like an ideal data set for investigating that. 01:46:02.900 |
- So you have a paper with some impressive results 01:46:07.900 |
and just an impressive paper that taken this problem on. 01:46:20.660 |
- Yeah, I think there's a few aspects of the results 01:46:25.460 |
So first of all, the fact that we were able to achieve 01:46:29.900 |
I was surprised by and pleasantly surprised by. 01:46:33.780 |
So we played 40 games of diplomacy with real humans 01:46:46.700 |
and the bot was ranked second out of those players. 01:46:49.200 |
And the bot was really good in two dimensions. 01:46:53.860 |
One, being able to establish strong connections 01:46:58.060 |
being able to like persuade them to work with it, 01:47:19.820 |
- What are some interesting things that the bot said? 01:47:26.260 |
like are there rules to what you're allowed to say 01:47:39.420 |
- Yeah, politely, you know, like keep it in character. 01:47:43.700 |
We actually had a researcher watching the bot 24/7, 01:47:50.580 |
and start like threatening somebody or something like that. 01:47:52.420 |
- I would just love it if the bot started like mocking, 01:47:56.460 |
like some weird quirky strategies would emerge. 01:47:59.620 |
That have you seen anything interesting that you, 01:48:19.500 |
And that, in a good way, the humans actually, 01:48:22.340 |
you know, we've talked to some expert diplomacy players 01:48:24.580 |
about these results and their takeaway is that, 01:48:27.180 |
well, maybe humans are approaching this the wrong way. 01:48:29.020 |
And this is actually like the right way to play the game. 01:48:37.860 |
or to exploit the suboptimal behavior of a player? 01:48:45.500 |
and irrational behavior that you need to estimate, 01:48:53.860 |
Is there like a weakness that you can exploit in the game 01:49:00.200 |
- Well, I think you're asking kind of two questions there. 01:49:13.420 |
you can't treat all the other players like they're machines. 01:49:17.060 |
you're going to end up playing really poorly. 01:49:35.060 |
and we trained a bot for the full seven player version 01:49:37.300 |
of the game through self-play without any human data. 01:49:44.820 |
where there's no explicit natural language communication, 01:49:49.100 |
because it just wouldn't be able to understand 01:49:50.960 |
how the other players were approaching the game 01:49:58.520 |
there's an individual personality to each player 01:50:01.940 |
But what do you mean it's not able to understand the players? 01:50:07.580 |
expect the human to support it in a certain way 01:50:13.740 |
think like, no, I'm not supposed to support you here. 01:50:22.800 |
it might learn to drive on the left side of the road. 01:50:28.260 |
that are also driving on the left side of the road. 01:50:30.120 |
But if you put it in an American city, it's gonna crash. 01:50:33.020 |
- But I guess the intuition I'm trying to build up 01:50:34.700 |
is why does it then crush a human player heads up 01:50:52.600 |
where you don't have to worry about the other player 01:50:59.400 |
the only way that deviating from a Nash equilibrium 01:51:11.280 |
Do you always have to have one friend in the game? 01:51:23.040 |
And boy, and the lying comes into play there. 01:51:32.280 |
- Yeah, I mean, I guess you have to attack somebody 01:51:35.320 |
- Right, so that's the tension, but this is too real. 01:51:53.800 |
of how this suboptimality and irrationality comes into play, 01:51:57.320 |
there's a really common situation in the game of diplomacy 01:52:04.200 |
and they're at the point where they're controlling 01:52:09.000 |
who have all been fighting each other the whole game 01:52:19.960 |
where you got the others coming from the north 01:52:22.040 |
and all the people have to work out their differences 01:52:39.720 |
it will also at the same time attack the other players 01:52:47.540 |
it will use those to grab as many centers as possible 01:52:55.020 |
the other players should just live with that. 01:52:57.760 |
"Hey, a score of one is better than a score of zero. 01:53:15.160 |
because you did something that's not fair to me. 01:53:20.720 |
Is the bot supposed to model that kind of human frustration? 01:53:25.660 |
So that is something that seems almost impossible to model 01:53:32.760 |
And so you need human data to be able to understand that, 01:53:40.100 |
It might be suboptimal, it might be irrational, 01:53:42.300 |
but that's an aspect of humanity that you have to deal with. 01:53:47.220 |
- So how difficult is it to train on human data 01:53:51.340 |
versus what a purely self-play mechanism can generate? 01:53:55.380 |
- That's actually one of the major challenges 01:54:01.380 |
What we try to do is leverage as much self-play as possible 01:54:11.660 |
very similar to how it's been done in poker and Go, 01:54:28.100 |
that are very unlikely under the human data set. 01:54:38.260 |
- Yeah, so we train a bot through supervised learning 01:54:44.140 |
So we basically train a neural net on those 50,000 games, 01:54:50.080 |
that gives us a policy that resembles to some extent 01:54:54.300 |
Now, this isn't a perfect model of human play 01:55:07.620 |
So on the language side of things, is there some, 01:55:22.780 |
we didn't use the language model during self-play training, 01:55:29.020 |
on tons of internet data as much as possible. 01:55:35.700 |
So we are able to leverage the wider data set 01:55:44.340 |
besides just specifically in these diplomacy games. 01:55:47.920 |
What are some interesting things that came to life 01:56:01.200 |
and cooperation, deep cooperation is involved? 01:56:06.040 |
So first of all, the fact that you can't rely purely 01:56:12.920 |
that you really have to have an understanding 01:56:17.480 |
I think that that's one of the major conclusions 01:56:20.640 |
And that is, I think, applicable more broadly 01:56:25.000 |
So we've actually already taken the approaches 01:56:31.840 |
And we've had a lot of success in that game as well. 01:56:39.200 |
that we were able to control the language model 01:56:43.080 |
through this intense approach was very effective. 01:56:49.880 |
how humans would communicate, we're able to go beyond that 01:56:52.720 |
and able to feed into its superhuman strategies 01:56:57.720 |
that it can then generate messages corresponding to. 01:57:02.600 |
- Is there something you could say about detecting 01:57:07.900 |
- The bot doesn't explicitly try to calculate 01:57:30.800 |
Based on your messages, if I think you're going 01:57:34.620 |
to attack me this turn, even though your messages say 01:57:47.260 |
- But you could probably reformulate with all the same data 01:57:56.620 |
That was not something that we were focused on, 01:58:00.240 |
if you came up with some measurements of like, 01:58:06.000 |
Like if you're withholding some information, is that a lie? 01:58:11.860 |
but you forgot to mention this one action out of 10, 01:58:16.500 |
It's hard to draw the line, but if you're willing to do that 01:58:22.580 |
- This feels like an argument inside a relationship now. 01:58:27.860 |
Depends what you mean by the definition of the word is. 01:58:32.260 |
Okay, still it's fascinating because trust and lying 01:58:37.260 |
is all intermixed into this and it's language models 01:58:41.700 |
that are becoming more and more sophisticated. 01:58:52.340 |
that is inspired by the breakthrough performance 01:59:04.180 |
I think really what it's showing us is the potential 01:59:12.220 |
that this kind of result was possible even today, 01:59:14.980 |
despite all the progress that's been made in language models. 01:59:17.540 |
And so it shows us how we can leverage the power 01:59:21.420 |
of things like self-play on top of language models 01:59:43.100 |
a dance between entities that are trying to cooperate 01:59:46.780 |
and at the same time, a little bit adversarial, 01:59:53.880 |
the entire process of Reddit or like internet communication. 02:00:02.300 |
you're having debates, you're having a camaraderie, 02:00:06.780 |
- I think one of the things that's really useful 02:00:08.700 |
about diplomacy is that we have a well-defined 02:00:16.660 |
And in a setting like a general chatbot setting, 02:00:27.460 |
- What about like what we talked about earlier 02:00:44.740 |
all kinds of violence with a sword and fighting dragons, 02:00:51.580 |
- The way that we've approached AI in diplomacy 02:01:06.700 |
where there's some intent or there's some objective 02:01:12.200 |
And then the language can correspond to that intent. 02:01:17.460 |
Now, I'm not saying that this is happening imminently, 02:01:19.820 |
but I'm saying that this is like a future application 02:01:25.020 |
- So what's the more general formulation of this? 02:01:27.820 |
Making self-play be able to scale the way self-play does 02:01:33.740 |
- The way that we've approached self-play in diplomacy 02:01:47.980 |
Now, there is the potential to have a broader set of intents, 02:01:51.420 |
things like long-term cooperation or long-term objectives 02:01:56.420 |
or gossip about what another player was saying. 02:02:01.080 |
These are things that we're currently not conditioning 02:02:03.140 |
the language model on, and so we're not able to control it 02:02:09.800 |
But it's quite possible that you could expand 02:02:17.820 |
the self-play would become much more complicated. 02:02:23.820 |
- Okay, the increase in the number of intents. 02:02:25.580 |
I still am not quite clear how you keep the self-play 02:02:35.800 |
- I'm a little bit loose on understanding how you do that. 02:02:39.380 |
- So we train in neural nets to imitate the human data 02:02:56.520 |
because we don't have unlimited neural network capacity, 02:03:00.220 |
it's actually a relatively suboptimal approximation 02:03:10.220 |
And so what we do is we get a better approximation, 02:03:19.080 |
we say you can deviate from this human anchor policy 02:03:29.240 |
But it would have to be a really high expected value 02:03:32.260 |
in order to deviate from this human-like policy. 02:03:40.920 |
stay as close as possible to the human policy. 02:03:46.580 |
the relative weighting of those competing objectives. 02:03:52.620 |
is how sophisticated can the anchor policy get? 02:03:56.500 |
So I have a policy that approximates human behavior, right? 02:04:03.440 |
as you generalize the space in which this is applicable, 02:04:27.600 |
- I think the more human data you have, the better. 02:04:30.040 |
And I think that that's going to be the major bottleneck 02:04:40.800 |
where we leveraged tons of data on the internet 02:04:47.900 |
that you can leverage huge amounts of data across the board 02:05:00.440 |
to the general, the real world diplomacy, the geopolitics? 02:05:06.400 |
You know, there's a game theory has a history 02:05:13.120 |
and to give us hope about nuclear weapons, for example. 02:05:17.820 |
is a game theoretic concept that you can formulate. 02:05:27.320 |
Do you see a future where this kind of system 02:05:40.800 |
for the game of diplomacy was the failures of World War I, 02:05:46.680 |
And the real take-home message of diplomacy is that, 02:05:53.000 |
the right way, then war is ultimately unsuccessful. 02:06:06.020 |
And my hope is that, you know, as AI progresses, 02:06:12.480 |
to help people make better decisions across the board 02:06:21.360 |
- Yeah, I mean, I just came back from Ukraine. 02:06:36.520 |
leaders getting together and having conversations 02:06:53.520 |
If I'm nice, what are the possible consequences? 02:06:56.720 |
My guess is that if the president of the United States 02:07:01.200 |
got together with Vladimir Zelensky and Vladimir Putin, 02:07:10.240 |
to the president of the United States not having the ego 02:07:14.880 |
of kind of playing down, of giving away a lot of chips 02:07:22.200 |
So giving a lot of power to the two presidents 02:07:29.120 |
but it'd be nice to run a bunch of simulations. 02:07:33.280 |
You really, 'cause it's like the game of diplomacy 02:07:39.040 |
You need like, I guess that's the question I have. 02:07:44.160 |
like I don't know, any kind of negotiation, right? 02:07:47.600 |
Like to any kind of, some local, I don't know, 02:08:01.440 |
I mean, I think you look at RL breakthroughs, 02:08:09.200 |
You haven't really seen it deployed in the real world 02:08:16.600 |
and you don't have a well-defined action space. 02:08:21.280 |
You don't have a well-defined reward function. 02:08:29.440 |
Now, there are some domains where you do have that. 02:08:35.360 |
Theorem proving mathematics, that's another example 02:08:47.120 |
But yeah, I think that those are the barriers 02:08:51.120 |
to deploying this at scale in the real world. 02:09:09.520 |
And it also feels like you could get data on that 02:09:14.760 |
- Yeah, and that's why I do think that diplomacy 02:09:17.400 |
is taking a big step closer to the real world 02:09:23.200 |
The fact that we're communicating in natural language, 02:09:30.640 |
like general data set of dialogue and communication 02:09:39.940 |
We're not 100% there, but we're getting closer at least. 02:09:44.020 |
- So if we actually return back to poker and chess, 02:09:47.320 |
are some of the ideas that you're learning here 02:09:48.920 |
with diplomacy, could you construct AI systems 02:09:55.080 |
Like make for a fun opponent in a game of chess? 02:10:01.240 |
We've already started looking into this direction a bit. 02:10:03.080 |
So we tried to use the techniques that we've developed 02:10:08.840 |
And what we found is that it led to much more human-like 02:10:32.400 |
and we end up with a bot that's both strong and human-like. 02:10:43.000 |
AI for chess is to collect a bunch of human games, 02:10:49.560 |
and just to supervise learning on those games. 02:10:53.880 |
what you end up with is an AI that's substantially weaker 02:10:57.200 |
than the human grandmasters that you trained on. 02:10:59.760 |
Because the neural net is not able to approximate 02:11:06.240 |
This goes back to the planning thing that I mentioned, 02:11:10.520 |
that these human grandmasters, when they're playing, 02:11:12.640 |
they're using search and they're using planning. 02:11:22.080 |
it's not able to approximate those details very effectively. 02:11:28.560 |
you can leverage search and planning very heavily, 02:11:40.020 |
by setting the regularization parameters correctly 02:11:44.440 |
but try to keep it close to the human policy, 02:11:49.800 |
in both a very human-like style and a very strong style. 02:11:58.320 |
So you can say, play in the style of like a 2800 ELO human. 02:12:01.480 |
- I wonder if you could do specific type of humans 02:12:04.920 |
or categories of humans, not just skill, but style. 02:12:10.400 |
And so this is where the research gets interesting. 02:12:13.720 |
Like, one of the things that I was thinking about is, 02:12:18.280 |
there's a researcher at the University of Toronto 02:12:26.960 |
you can make an AI that plays like Magnus Carlsen. 02:12:29.680 |
And then where I think this gets interesting is like, 02:12:42.600 |
that he might struggle with and try to figure out like, 02:12:48.880 |
On the other hand, you can also have Magnus Carlsen 02:12:51.040 |
working with this bot to try to figure out where he's weak 02:13:03.600 |
becomes extremely valuable because you can use that data 02:13:10.040 |
- So increasingly human-like behavior in bots, however, 02:13:20.040 |
The way that cheat detection works in a game like poker 02:13:23.540 |
and a game like chess and Go, from what I understand, 02:13:26.100 |
is trying to see like, is this person making moves 02:13:30.180 |
that are very common among chess AIs or AIs in general? 02:13:48.280 |
then that poses serious challenges for cheat detection. 02:13:51.280 |
- And it makes you now ask yourself a hard question 02:13:56.720 |
as they become more and more integrated in our society? 02:14:03.620 |
has some deep ethical issues that we should be aware of. 02:14:08.620 |
And also it's a kind of cybersecurity challenge, right? 02:14:14.260 |
one of the assumptions we have when we play games 02:14:17.100 |
is that there's a trust that it's only humans involved. 02:14:26.140 |
human-like AI systems with different styles of humans 02:14:36.880 |
human versus human game in a deeply fair way. 02:14:44.320 |
- Yeah, I think there's a lot of like negative potential 02:15:05.360 |
oh, I'm a 2000 Elo human, how do I get to 2200? 02:15:08.240 |
Now you can have an AI that plays in the style 02:15:10.760 |
of a 2200 Elo human, and that will help you get better. 02:15:14.160 |
Or, you know, you mentioned this problem of like, 02:15:16.840 |
how do you know that you're actually playing with humans 02:15:19.440 |
when you're playing like online and in video games? 02:15:22.000 |
Well, now we have the potential of populating 02:15:28.560 |
like AI agents that are actually fun to play with, 02:15:30.720 |
and you don't have to always be playing with other humans 02:15:38.560 |
And I think, you know, with any sort of tool, 02:15:44.520 |
- So in the paper that I got a chance to look at, 02:15:53.560 |
Is it some of the stuff we already talked about? 02:15:55.760 |
- There's some things that we've already talked about. 02:16:04.960 |
you know, there is a deception aspect to the game. 02:16:11.360 |
that are capable of deception is I think a dicey issue 02:16:16.160 |
makes research on diplomacy particularly challenging. 02:16:18.800 |
And, you know, so those kinds of issues of like, 02:16:32.680 |
in order to figure out where the ethical lines are. 02:16:53.360 |
I sure as hell want that AI system to lie to me. 02:16:56.880 |
So there's a trade off between lying and being nice. 02:17:04.920 |
And we're back to discussions inside relationships. 02:17:09.840 |
that's kind of going to the question of like, 02:17:20.520 |
to deep human questions as we design AI systems. 02:17:36.920 |
there's an inherent anti-AI bias in these kinds of games. 02:17:45.000 |
where, you know, we told the participants like, 02:17:47.000 |
hey, in every single game, there's going to be an AI. 02:17:55.040 |
And then as soon as they thought they figured it out, 02:17:58.480 |
And, you know, overcoming that inherent anti-AI bias 02:18:05.520 |
- On the flip side, I think when robots become the enemy, 02:18:10.520 |
that's when we get to heal our human divisions, 02:18:18.000 |
it's that Reagan thing when the aliens show up, 02:18:33.120 |
something like a civil rights movement for robots. 02:18:35.440 |
I think that's the fascinating thing about AI systems, 02:18:38.200 |
and that is they ask, they force us to ask about 02:18:50.400 |
And how do we design products that show emotion and not? 02:19:04.640 |
I mean, these are all fascinating human questions, 02:19:22.280 |
because you'll have transformational impact on human society 02:19:26.960 |
depending on what you design inside those systems. 02:19:33.480 |
is a step towards the direction of the real world, 02:19:35.960 |
applying these RL methods towards the real world. 02:19:50.720 |
This feels like it can give us some deep insights 02:19:53.320 |
about human behavior at the large geopolitical scale. 02:20:08.960 |
towards solving intelligence, towards creating AGI systems? 02:20:15.600 |
by the way, we should say a part of great teams 02:20:43.360 |
And I should say, the amount of progress that's been made, 02:20:46.400 |
especially in the past few years is truly phenomenal. 02:20:49.360 |
I mean, you look at where AI was 10 years ago, 02:20:53.840 |
that can generate language and generate images 02:21:04.560 |
Now, there are aspects of AI that I think are still lacking. 02:21:19.920 |
It requires a huge number of samples of training examples 02:21:32.000 |
Whereas a human can pick it up in like, you know, 02:21:34.160 |
I don't know, how many games does a human Go player, 02:21:47.080 |
- Overcoming this challenge of data efficiency. 02:21:50.120 |
if we want to deploy AI systems in real world settings 02:21:56.480 |
because, you know, for example, with robotics, 02:21:58.440 |
it's really hard to generate a huge number of samples. 02:22:01.360 |
It's a different story when you're working in these, 02:22:06.100 |
where you can play a million games and it's no big deal. 02:22:08.520 |
- I was planning on just launching like a thousand 02:22:23.800 |
- Like I actually tried to see if there's a law 02:22:26.280 |
against robots, like legged robots just operating 02:22:30.080 |
in the streets of a major city and there isn't, 02:22:35.160 |
So I'll take it all the way to the Supreme Court. 02:22:48.040 |
- I mean, that's the trillion dollar question in AI today. 02:22:50.840 |
I mean, if you can figure out how to make AI systems 02:22:52.840 |
more data efficient, then that's a huge breakthrough. 02:22:58.960 |
- It could be just a gigantic background model, 02:23:03.780 |
the training becomes like prompting that model 02:23:11.960 |
a search into the space of the things it's learned 02:23:14.580 |
to customize that to whatever problem you're trying to solve. 02:23:17.480 |
So maybe if you form a large enough language model, 02:23:26.840 |
a game like poker, they're not coming at it from scratch. 02:23:31.400 |
of background knowledge about how humans work, 02:23:38.040 |
So they're able to leverage that kind of information 02:23:49.640 |
this sample complexity problem is by allowing AIs 02:23:56.740 |
- So, like I said, you did a lot of incredible work 02:24:01.600 |
in the space of research and actually building systems. 02:24:04.720 |
What advice would you give to, let's start with beginners. 02:24:11.840 |
Just they're at the very start of their journey, 02:24:15.280 |
thinking like this seems like a fascinating world. 02:24:24.800 |
working on similar aspects of machine learning 02:24:27.640 |
and to not be afraid to try something a bit different. 02:24:39.920 |
and then shifting more towards reinforcement learning 02:24:44.880 |
And that actually had a lot of benefits, I think, 02:24:46.600 |
because it allowed me to look at these problems 02:24:49.920 |
from the way a lot of machine learning researchers view it. 02:24:54.120 |
And that comes with drawbacks in some respects. 02:24:58.360 |
Like I think there's definitely aspects of machine learning 02:25:00.560 |
where I'm weaker than most of the researchers out there, 02:25:10.160 |
there's something that I'm bringing to the table 02:25:11.320 |
and there's something that they're bringing to the table. 02:25:20.960 |
there could be problems like that still out there 02:25:26.440 |
- I think that there's a lot of challenges left. 02:25:42.360 |
I would say that's more for like a grad student 02:25:46.880 |
like a complete beginner, what's a good journey. 02:25:51.360 |
in the math side of things, doing game theory, 02:25:53.880 |
all that, so it's basically build up a foundation 02:25:59.440 |
it could even be physics, but build that foundation. 02:26:03.400 |
- Yeah, I would say build a strong foundation 02:26:08.280 |
but don't be afraid to try something that's different 02:26:17.680 |
There's value in having a different background 02:26:22.040 |
Yeah, so, but certainly having a strong math background, 02:26:30.560 |
are incredibly helpful today for learning about 02:26:37.000 |
since you're taking steps from poker to diplomacy, 02:26:45.280 |
- Well, what is it, like in poker and diplomacy, 02:26:49.360 |
you need a value function, you need to have a reward system. 02:26:52.120 |
And so what does it mean to live a life that's optimal? 02:26:55.080 |
- So, okay, so then you can exactly like lay down 02:26:59.200 |
a reward function being like, I wanna be rich 02:27:10.920 |
- There's a lot of talk today about in AI safety circles 02:27:15.760 |
about like misspecification of reward function. 02:27:19.160 |
So you say like, okay, my objective is to be rich 02:27:28.280 |
- And so you wanna, is that really what you want? 02:27:30.560 |
Is your objective really to be rich at all costs 02:27:50.760 |
the actual policy that gets you to the reward function. 02:28:01.080 |
is figuring out exactly what that reward function is. 02:28:06.360 |
The same way that trying to handcraft the optimal policy 02:28:12.280 |
It's not so clear cut what the reward function is for life. 02:28:28.960 |
into a more and more real-world-like problem space 02:28:49.400 |
- Thanks for listening to this conversation with Noah Brown. 02:28:52.400 |
To support this podcast, please check out our sponsors 02:28:56.120 |
And now, let me leave you with some words from Sun Tzu 02:29:01.720 |
"The whole secret lies in confusing the enemy 02:29:08.120 |
Thank you for listening and hope to see you next time.