back to indexMIT AGI: Artificial General Intelligence
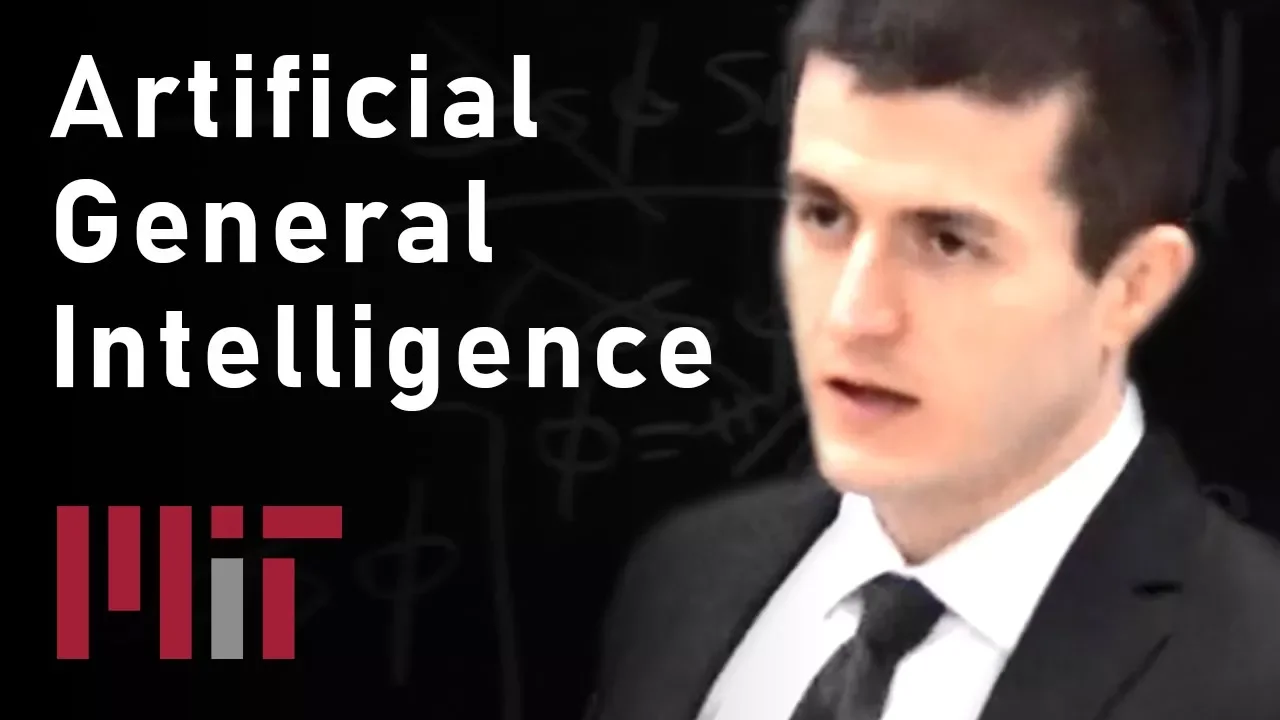
Chapters
0:0 Intro
0:23 MIT AGI Mission: Engineer Intelligence
3:41 Balance Between Paralyzing Technophobia and Blindness to Big Picture Consequences
11:2 Human Drive to explore and Uncover the Mysteries of the Universe
17:23 ANGEL: Artificial Neural Generator of Emotion and Language
18:56 EthicalCar: Machine Learning Approach
21:53 Josh Tenenbaum, MIT Computational Cognitive Science
24:16 Lisa Feldman Barrett, NEU Emotion Creation
25:40 Re-Enacting Intelligence
26:41 Sophia: Embodied Re-Enactment
29:32 Nate Derbinsky, NEU Cognitive Modeling
30:37 Deep Learning is Representation Learning Talca Feature Learning
33:9 Neuron: Biological Inspiration for Computation
35:49 Deep Learning from Human and Machine
37:28 Past and Future of Deep Learning Breakthroughs
38:45 Current Challenges
40:17 Stephen Wolfram Knowledge-Based Programming
41:38 "Artificial Life Simulation": Cellular Automata and Emerging Complexity
43:13 Richard Moyes, Article36 Al Safety and Autonomous Weapon Systems
43:46 Marc Raibert, CEO, Boston Dynamics Robots in the Real World
00:00:00.000 |
Welcome to course 6S099 Artificial General Intelligence 00:00:10.500 |
From as much as possible an engineering perspective 00:00:31.500 |
What that means is we want to explore the fundamental science 00:00:42.500 |
The core concepts behind our understanding of what is intelligence 00:00:48.500 |
But we always want to ground it in the creation of intelligent systems 00:00:59.500 |
In understanding how today we can build artificial intelligence systems 00:01:09.500 |
First and foremost, we're scientists and engineers 00:01:16.500 |
We want to provide with this approach a balance to 00:01:23.500 |
the very important but over-represented view of artificial general intelligence 00:01:34.500 |
Where the idea is once we know how to create a human level intelligence system 00:01:50.500 |
Will we achieve a utopia that will remove the need to do any of the messy jobs 00:01:58.500 |
Those kinds of beautiful philosophical concepts are interesting to explore 00:02:03.500 |
But that's not what we're interested in doing 00:02:05.500 |
I believe that from an engineering perspective 00:02:13.500 |
Start to build insights and intuitions about how we create systems 00:02:30.500 |
However, the dimension of the metric behind the word far 00:02:53.500 |
Our current methods as we will explore from the various ideas and approaches 00:02:57.500 |
and the guest speakers coming here over the next two weeks and beyond 00:03:02.500 |
Our best understanding, our best intuition and insights 00:03:14.500 |
and paradigm shift towards human level intelligence 00:03:17.500 |
So it's not constructive to consider the impact of artificial intelligence 00:03:32.500 |
It's not constructive to consider those questions 00:03:36.500 |
without also deeply considering the black box 00:03:40.500 |
of the actual methods of artificial intelligence 00:03:46.500 |
And that's what I see, what I hope this course can be 00:03:50.500 |
Its first iteration, its first exploratory attempt 00:03:56.500 |
to try to look at different approaches of how we can engineer intelligence 00:04:07.500 |
The future impact of society 10, 20, 30, 40 years out 00:04:11.500 |
But fundamentally grounded in what kind of methods do we have today 00:04:16.500 |
And what are their limitations and possibilities of achieving that 00:04:32.500 |
that get, become increasingly more intelligent 00:04:35.500 |
The fundamental disagreement lies in the fact 00:04:43.500 |
Which is how hard is it to build an AGI system 00:04:48.500 |
How hard is it to create a human level artificial intelligence system 00:05:04.500 |
To brilliant leaders in various fields of artificial intelligence 00:05:11.500 |
There's been a lot of incredibly impressive results 00:05:15.500 |
In deep learning, in neuroscience, in computational cognitive science 00:05:28.500 |
That's the fundamental question that we need to explore 00:05:31.500 |
Before we consider the questions, the future impact on society 00:05:38.500 |
And the goal for this class is to build intuition 00:05:48.500 |
About what the limitations of current approaches are 00:05:54.500 |
A nice meme that I caught on Twitter recently 00:05:59.500 |
Of the difference between the engineering approach 00:06:08.500 |
Typing a for loop that just does a grid search 00:06:14.500 |
And on the right is the way media would report this for loop 00:06:25.500 |
I think it's easy for us to go one way or the other 00:06:33.500 |
Our first goal is to avoid the pitfalls of black box thinking 00:06:38.500 |
Of the futurism thinking that results in hype 00:06:41.500 |
That's detached from scientific engineering understanding 00:06:49.500 |
That's what some of our speakers will explore in a rigorous way 00:06:59.500 |
Ray Kurzweil on Wednesday will explore this topic 00:07:03.500 |
Next week, talking about AI safety and autonomous weapon systems 00:07:11.500 |
How do we design systems today that would lead to safe systems tomorrow? 00:07:17.500 |
But the reality is a lot of us need to put a lot more emphasis 00:07:21.500 |
On the left, on the for loops, on creating these systems 00:07:25.500 |
At the same time, the second goal of what we're trying to do here 00:07:29.500 |
Is not emphasize the silliness, the simplicity 00:07:37.500 |
In the same way as was the process in creating nuclear weapons 00:07:50.500 |
That I'm just a scientist is also a flawed way of thinking 00:07:58.500 |
The near term, negative consequences that are preventable 00:08:02.500 |
The low hanging fruit that can be prevented through that very engineering process 00:08:24.500 |
Our best understanding of the capabilities of modern systems 00:08:30.500 |
Seem limited, seem far from human level intelligence 00:08:33.500 |
Our ability to learn and represent common sense reasoning 00:08:48.500 |
Means that just around the corner is a singularity 00:08:52.500 |
Is a breakthrough idea that will change everything 00:08:58.500 |
Moreover, we have to be cautious of the fact that 00:09:07.500 |
Our adoption of new technologies has gotten faster and faster 00:09:36.500 |
Is fundamentally cynical on artificial general intelligence 00:09:42.500 |
We have to always remember that overnight everything can change 00:09:46.500 |
Through this question of beginning to approach 00:09:53.500 |
From brain simulation, computational cognitive science 00:10:14.500 |
How far away are we from creating intelligent systems 00:10:32.500 |
By the certain analogy that we're in this dark room 00:10:39.500 |
With no knowledge of where the light switch is 00:10:48.500 |
It's anywhere we'll be able to find it in any time 00:12:19.500 |
Travel for the sake of discovery and adventure 00:13:01.500 |
Paved the way to colonization of the Americas 00:17:05.500 |
Who produces the most beautiful visualization 00:20:22.500 |
Autonomous vehicles that function in this world 00:20:27.500 |
From point A to point B as quickly as possible 00:21:04.500 |
On the topic of artificial general intelligence 00:21:39.500 |
And Nate Dzerbinski from Northeastern University 00:21:56.500 |
I'd like to go through each of these speakers 00:22:11.500 |
In the discussion of the future impact on society 00:22:41.500 |
And our own possibilities to act and interact with others 00:22:49.500 |
More than just the deep learning memorization engines 00:23:15.500 |
Often only need one example to learn a concept 00:24:06.500 |
And how that transfers to the increasing exponential growth 00:24:11.500 |
Of development of artificial general intelligence 00:24:20.500 |
Is Lisa Feldman Barrett coming here on Thursday 00:24:36.500 |
There is a detachment between what we feel in our bodies 00:24:52.500 |
Now why is there a person who is a psychology person 00:24:56.500 |
In a fundamental engineering computer science topic like AGI 00:25:00.500 |
Because if emotions are created in the way she argues 00:25:10.500 |
As human beings we're learning societal norms 00:25:14.500 |
The idea of emotional intelligence is learned 00:25:16.500 |
Which means we can have machines learn this idea 00:25:33.500 |
So it's going to be a little bit challenging and fun 00:25:46.500 |
Through video, through audio, through the project 00:25:52.500 |
So there's been work in reenacting intelligence 00:25:59.500 |
Mapping different emotions on video that was previously recorded 00:26:05.500 |
That means we can take emotions that we've created 00:26:09.500 |
The kind of emotion creation we've been discussing 00:26:18.500 |
Is taking raw human data that we already have 00:26:30.500 |
The representation of emotion visual or auditory 00:26:39.500 |
It could be in the embodied form like with Sophia 00:27:06.500 |
It might be possible to make them more effective 00:27:27.500 |
She's not a strong natural language processing system 00:27:44.500 |
That there's intelligence underlying something 00:28:16.500 |
Where underneath it is really trivial technology 00:31:59.500 |
And the ability to automatically learn features 00:34:33.500 |
Obviously our human brains are always learning 00:35:00.500 |
The underlying computational unit of a neuron 00:35:09.500 |
To represent both spatial information with images 00:49:41.500 |
How do we create artificial intelligence systems 00:50:20.500 |
We don't have a computational neuroscience speaker 00:50:37.500 |
To get the perspective of how our brain works 00:51:08.500 |
With that, I'd like to thank you for coming today