back to indexJeff Hawkins: Thousand Brains Theory of Intelligence | Lex Fridman Podcast #25
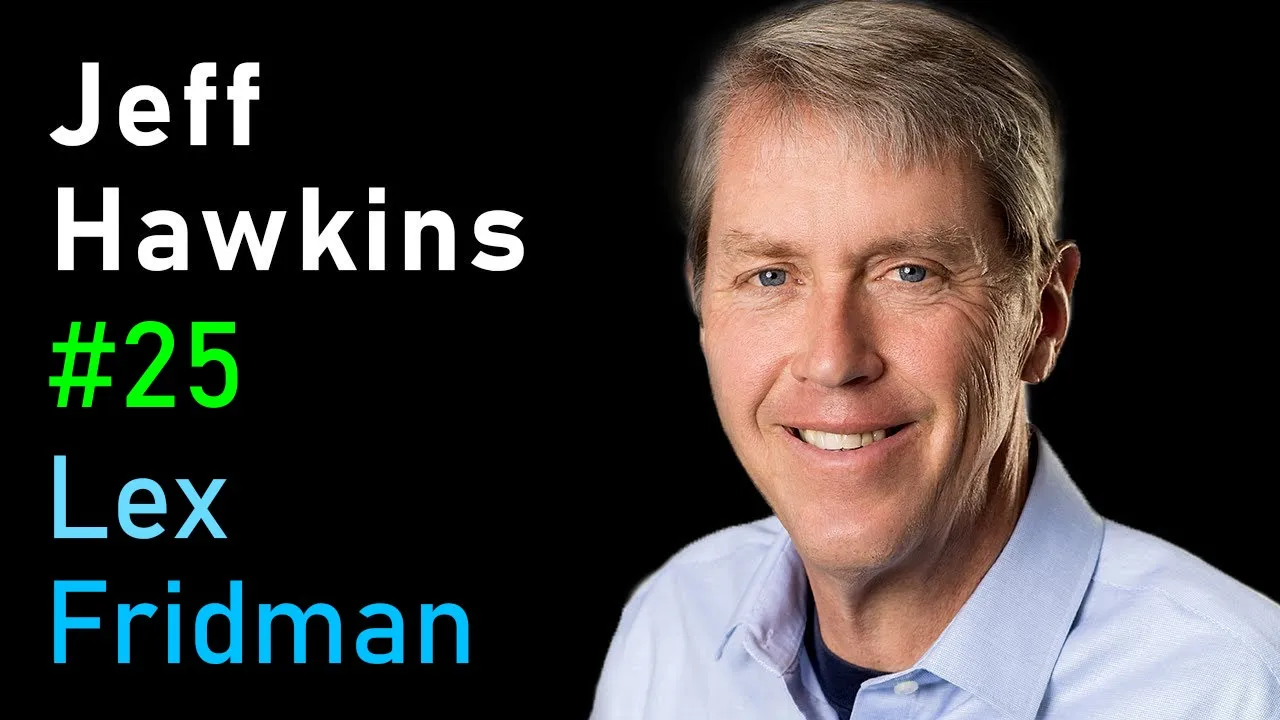
Chapters
0:0
6:0 Neocortex
10:42 The Common Cortical Algorithm
14:22 The Hierarchical Temporal Memory Theory
28:54 Evolutionary Timeline
34:35 Thousand Brain Theory
39:1 Somatosensory Cortex
39:50 Thousand Brains Theory of Intelligence
40:52 The Sensor Fusion Problem
43:2 Associative Memory Mechanism
54:33 Bats
58:15 Head Direction Cells
64:18 Deep Learning
71:34 Properties of Sparse Representations
72:26 Deep Neural Networks
76:47 The Entrepreneurs Dilemma
77:22 The Sparse Representations
90:10 Fast Learning
92:59 Silent Synapses
95:42 How Far Are We from Solving Intelligence
101:24 Embodiment
00:00:00.000 |
The following is a conversation with Jeff Hawkins. 00:00:04.160 |
for Theoretical Neuroscience in 2002 and NuMenta in 2005. 00:00:13.880 |
he and his team have worked to reverse engineer 00:00:16.200 |
the neurocortex and propose artificial intelligence 00:00:23.660 |
These ideas include hierarchical temporal memory, HTM, 00:00:26.640 |
from 2004, and new work, "The Thousands Brains Theory 00:00:38.200 |
beyond the current machine learning approaches, 00:00:48.080 |
more than small incremental steps forward in AI. 00:00:51.440 |
Jeff is a brilliant mind, and many of the ideas 00:00:54.120 |
he has developed and aggregated from neuroscience 00:01:02.960 |
Forward progress in AI is shrouded in mystery. 00:01:07.760 |
can help provide an inspiring spark for new ideas. 00:01:14.040 |
If you enjoy it, subscribe on YouTube, iTunes, 00:01:21.520 |
And now, here's my conversation with Jeff Hawkins. 00:01:26.320 |
Are you more interested in understanding the human brain 00:01:37.640 |
actually understand the underpinning workings of our mind? 00:01:41.480 |
- So, there's a clear answer to that question. 00:01:43.960 |
My primary interest is understanding the human brain. 00:01:47.680 |
But, I also firmly believe that we will not be able 00:01:55.040 |
until we understand how the human brain works. 00:02:01.720 |
with machine intelligence if you don't understand 00:02:05.680 |
And so, I actually believe that studying the brain 00:02:07.920 |
is actually the fastest way to get to machine intelligence. 00:02:12.000 |
- And within that, let me ask the impossible question. 00:02:14.640 |
How do you, not define, but at least think about 00:02:19.400 |
- So, I didn't try to answer that question first. 00:02:22.240 |
We said, let's just talk about how the brain works 00:02:24.480 |
and let's figure out how certain parts of the brain, 00:02:26.680 |
mostly the neocortex, but some other parts too, 00:02:29.880 |
the parts of the brain most associated with intelligence, 00:02:32.320 |
and let's discover the principles by how they work. 00:02:35.800 |
'Cause intelligence isn't just like some mechanism 00:02:44.040 |
And so, now that we've made a lot of progress on this, 00:02:50.440 |
on how the neocortex works and we can talk about that, 00:02:53.160 |
I now have a very good idea what's gonna be required 00:03:04.560 |
We'll get to the neocortex and some of the theories 00:03:09.160 |
And you're saying, as we understand more and more 00:03:11.720 |
about the neocortex, about our own human mind, 00:03:14.740 |
we'll be able to start to more specifically define 00:03:18.640 |
It's not useful to really talk about that until-- 00:03:23.560 |
Look, there's a long history of AI, as you know. 00:03:26.160 |
And there's been different approaches taken to it. 00:03:31.280 |
So, the good old-fashioned AI, the expert systems, 00:03:43.760 |
But I would think almost everyone would agree 00:03:49.880 |
And so, it's just the question of how do you get 00:03:56.440 |
to where a lot of people think we're gonna go. 00:03:59.240 |
And there's a big, big gap there, a huge gap. 00:04:02.320 |
And I think the quickest way of bridging that gap 00:04:10.200 |
oh, which of these principles that the brain works on 00:04:16.600 |
and intelligent machines aren't gonna be built 00:04:22.760 |
But there's a lot of stuff that goes on in the brain 00:04:25.940 |
- So, let me ask maybe, before we get into the fun details, 00:04:30.320 |
let me ask maybe a depressing or difficult question. 00:04:33.280 |
Do you think it's possible that we will never 00:04:38.080 |
That maybe there's aspects to the human mind, 00:04:41.880 |
like we ourselves cannot introspectively get to the core, 00:05:04.480 |
There's like, what's the difference between living matter 00:05:14.720 |
And I just never even consider that's a possibility. 00:05:17.620 |
I would also say, today, we understand so much 00:05:22.820 |
We've made tremendous progress in the last few years 00:05:25.480 |
that I no longer think of it as an open question. 00:05:31.880 |
and the pieces we don't know are clear to me, 00:05:36.120 |
and it's like, oh, okay, we're gonna be able to do this. 00:05:44.040 |
- So, then let's get into it for people like myself 00:05:47.780 |
who are not very well versed in the human brain, 00:05:53.820 |
Can you describe to me, at the highest level, 00:05:57.300 |
what are the different parts of the human brain, 00:06:02.060 |
the parts of the neocortex, and so on, a quick overview? 00:06:06.620 |
The human brain, we can divide it roughly into two parts. 00:06:18.040 |
It's new because it didn't exist before mammals. 00:06:36.000 |
There's the spinal cord, and there's the brainstem, 00:06:46.280 |
You have basic behaviors, so like walking and running 00:06:49.460 |
are controlled by the old parts of the brain. 00:07:03.340 |
as sort of high-level perception and cognitive functions, 00:07:08.100 |
anything from seeing and hearing and touching things 00:07:21.740 |
Our abilities in those regards are correlated 00:07:30.500 |
and you obviously can't understand the neocortex 00:07:33.420 |
completely isolated, but you can understand a lot of it 00:07:37.020 |
with just a few interfaces to the old parts of the brain. 00:07:44.980 |
The other remarkable thing about the neocortex 00:07:58.780 |
I always like to say it's like the size of a dinner napkin, 00:08:05.980 |
Everywhere you look in that two and a half millimeters 00:08:15.420 |
Where if you look at the old parts of the brain, 00:08:17.100 |
there's lots of little pieces that do specific things. 00:08:19.660 |
So it's like the old parts of our brain evolved, 00:08:22.100 |
like this is the part that controls heart rate, 00:08:27.220 |
And these evolved for eons, a long, long time, 00:08:33.260 |
and they got this thing called the neocortex, 00:08:35.220 |
and it got large by just replicating the same thing 00:08:45.380 |
and this is an idea that was first articulated 00:08:52.020 |
by a guy named Vernon Malkasal in 1978, I think it was, 00:08:55.660 |
that the neocortex all works on the same principle. 00:09:01.580 |
So language, hearing, touch, vision, engineering, 00:09:06.980 |
are all built in the same computational substrate. 00:09:11.820 |
- So at the low level, the building blocks all look similar. 00:09:17.900 |
We're talking about this very complex circuit 00:09:19.940 |
that exists throughout the neocortex is remarkably similar. 00:09:23.500 |
It's like, yes, you see variations of it here and there, 00:09:35.580 |
why is one a visual area and one is a auditory area? 00:09:38.620 |
And his answer was, it's because one is connected to eyes 00:09:47.820 |
in terms of number of connections to the sensor. 00:09:52.900 |
and attached it to a different part of the neocortex, 00:09:57.940 |
This experiment was actually done by Morganka Sur 00:10:18.260 |
because of some congenital defect or something. 00:10:31.980 |
It's not like, oh, they're all built on neurons. 00:10:33.700 |
No, they're all built in this very complex circuit 00:10:36.420 |
and somehow that circuit underlies everything. 00:10:42.140 |
it's called the common cortical algorithm, if you will. 00:10:47.940 |
and they just say, I can't believe that's true, 00:10:50.020 |
but the evidence is overwhelming in this case. 00:10:54.300 |
to figure out how the brain creates intelligence 00:11:02.020 |
If you can figure out what that circuit does, 00:11:08.140 |
what all these other cognitive functions are. 00:11:19.780 |
and you look maybe a couple centuries from now, 00:11:25.480 |
would still be accurate two centuries from now? 00:11:27.720 |
So how close are we in terms of understanding? 00:11:30.840 |
- I have to speak from my own particular experience here. 00:11:43.840 |
We study the neocortex and we publish our results and so on. 00:11:53.320 |
We've now published, I think, three papers on it. 00:12:02.320 |
I would say that almost all the empirical data 00:12:06.240 |
we've collected about the brain, which is enormous. 00:12:08.520 |
If you don't know the neuroscience literature, 00:12:27.840 |
It's data without, in the language of Thomas Kuhn, 00:12:32.240 |
the historian, it would be sort of a pre-paradigm science. 00:12:35.280 |
Lots of data, but no way to fit it in together. 00:12:47.240 |
It's not like we have two or three competing good theories, 00:12:50.040 |
which ones are right and which ones are wrong. 00:12:51.520 |
It's like, nah, people just like scratching their heads, 00:12:54.760 |
Some people have given up on trying to like figure out 00:12:57.600 |
In fact, there's very, very few labs that we do 00:13:03.320 |
and all this unassimilated data and trying to explain it. 00:13:11.160 |
- So it's really, I would say, pretty early days 00:13:15.080 |
in terms of understanding the fundamental theories, 00:13:21.120 |
I would have said that's true five years ago. 00:13:27.040 |
we had some really big breakthroughs on this recently 00:13:39.480 |
but from where I sit today, from what I know, 00:13:45.080 |
The way these things go is it's not a linear path, right? 00:13:50.840 |
No, you've got all this stuff you've collected. 00:13:53.800 |
All these different things are just sort of around. 00:13:55.680 |
And then you're gonna have some breaking points 00:13:57.160 |
where all of a sudden, oh my God, now we got it right. 00:14:01.160 |
And I personally feel like we passed that little thing 00:14:12.680 |
That's why I'm willing to say it on tape like this. 00:14:27.560 |
and went through a few different generations. 00:14:35.440 |
- Yeah, so one of the things that neuroscientists 00:14:43.000 |
and especially people who are thinking about theory, 00:14:51.680 |
The information coming into the brain is constantly changing. 00:15:01.480 |
about every 10 milliseconds or so, you'd have it change. 00:15:11.360 |
If I were to touch something like a coffee cup, 00:15:15.160 |
So this idea that the brain works on time-changing patterns 00:15:19.480 |
is almost completely, or was almost completely missing 00:15:26.840 |
in front of you and flash it and say, what is it? 00:15:29.560 |
Convolutional neural networks work that way today, right? 00:15:35.960 |
Vision is this sort of crazy time-based pattern 00:15:41.840 |
So the first part of hierarchical temporal memory 00:15:50.040 |
unless you're dealing with time-based patterns. 00:15:52.480 |
The second thing was, the memory component of it was, 00:15:55.440 |
is to say that we aren't just processing input. 00:16:06.360 |
the part of the neocortex, it learns a model of the world. 00:16:19.920 |
And many people just, they were thinking about 00:16:22.080 |
like certain processes without memory at all. 00:16:34.440 |
different parts of it project to other parts, 00:16:39.360 |
And there is a sort of a rough hierarchy in terms of that. 00:16:43.080 |
So the hierarchical temporal memory is just saying, 00:16:54.000 |
And that was a placeholder for a bunch of components 00:17:00.840 |
We still believe all those things I just said, 00:17:02.640 |
but we now know so much more that I'm stopping to use 00:17:08.200 |
because it's insufficient to capture the stuff we know. 00:17:11.320 |
So again, it's not incorrect, but it's, I now know more 00:17:14.320 |
and I would rather describe it more accurately. 00:17:16.840 |
- Yeah, so you're basically, we can think of HTM 00:17:20.360 |
as emphasizing that there's three aspects of intelligence 00:17:28.880 |
So in terms of time, how do you think of nature of time 00:17:36.800 |
sensory inputs changing every 10, 20 minutes. 00:17:39.200 |
What about every few minutes, every few months and years? 00:17:42.160 |
- Well, if you think about a neuroscience problem, 00:17:44.880 |
the brain problem, neurons themselves can stay active 00:17:54.280 |
So you could hold a certain perception or an activity 00:18:14.400 |
the neurons haven't been active throughout that time. 00:18:17.800 |
So if I ask you, what did you have for breakfast today? 00:18:22.000 |
That is, you've built it into your model of the world now. 00:18:41.840 |
That's the timescales of activities of neurons. 00:18:49.560 |
oh yes, well now I can remember what I had for breakfast 00:18:54.240 |
I may forget it tomorrow, but I'd store it for now. 00:19:06.240 |
is not just about concepts, it's also about time? 00:19:15.560 |
If I ask you, how does the brain learn a model 00:19:21.400 |
I have a coffee cup, and I'm at the coffee cup. 00:19:28.480 |
whether it's a visual model or tactile model. 00:19:32.560 |
but the model itself doesn't really have much time. 00:19:38.960 |
My brain has learned a model of the cell phone. 00:19:43.320 |
And I said, well this has time aspects to it. 00:19:48.000 |
what's gonna happen, what or how long it's gonna take 00:19:54.000 |
And so I have, it's like melodies in the world. 00:20:08.240 |
So it's sort of infused throughout the models of the world. 00:20:13.680 |
you're learning the structure of the objects in the world, 00:20:19.720 |
- Okay, so it really is just the fourth dimension 00:20:30.040 |
So like you mentioned, the state of neuroscience 00:20:36.000 |
A lot of data collection, that's where it is. 00:20:44.520 |
- And then you're proposing a theory of intelligence, 00:20:52.880 |
But why is HTM, or what we'll talk about soon, 00:21:11.960 |
Is it kind of closer to where string theory is in physics, 00:21:15.560 |
where there's mathematical components which show that, 00:21:22.960 |
it fits together too well for it not to be true, 00:21:32.760 |
is definitely much more on the empirical side 00:21:36.040 |
The way this goes about, we're theorists, right? 00:21:40.760 |
and we're trying to come up with some sort of model 00:21:46.840 |
there's vast more amounts of empirical data here 00:21:57.560 |
and figure out what kind of constructs would explain this. 00:22:18.160 |
and we say, "Oh, did someone find this already?" 00:22:27.920 |
and we say, "Oh, either it can support the theory, 00:22:33.400 |
And then we say, "Okay, we have to start over again. 00:22:45.440 |
and it's what I call is a set of constraints. 00:22:49.880 |
We're trying to figure out how the actual brain works. 00:22:59.400 |
So we have this huge number of constraints on the problem, 00:23:03.160 |
which initially makes it very, very difficult. 00:23:09.480 |
"and how you can do this, you can do that, you can do this." 00:23:11.360 |
But if you consider all biology as a set of constraints, 00:23:15.560 |
and even if you're working on one little part 00:23:18.400 |
there are hundreds and hundreds of constraints. 00:23:24.840 |
to come up with a theoretical framework for that. 00:23:27.280 |
But when you do, and it solves all those constraints at once, 00:23:35.640 |
It's just mathematically almost impossible not to be. 00:23:39.160 |
So that's the curse and the advantage of what we have. 00:23:43.000 |
The curse is we have to meet all these constraints, 00:23:54.920 |
In addition, then we work with scientific labs. 00:23:58.040 |
So we'll say, "Oh, there's something we can't find. 00:24:01.160 |
"but we can't find it anywhere in the literature." 00:24:04.160 |
So we will then, we have people we've collaborated with, 00:24:09.580 |
"I have some collected data, which I didn't publish, 00:24:14.800 |
Which is much easier than designing a new experiment. 00:24:17.400 |
Neuroscience experiments take a long time, years. 00:24:20.360 |
So although some people are doing that now too. 00:24:31.640 |
We are blessed with the fact that we can test our theories 00:24:37.960 |
And we can also falsify our theories very easily, 00:24:45.480 |
When you figure out that the sun's at the center 00:24:54.880 |
- Yeah, I think that's the general nature of a hot moment. 00:25:04.160 |
You could say the same thing about the double helix. 00:25:09.720 |
That people have been working on a problem for so long 00:25:12.840 |
and have all this data and they can't make sense of it, 00:25:23.120 |
I asked both Jim Watson and Francis Crick about this. 00:25:28.080 |
I asked them, when you were working on trying 00:25:31.960 |
to discover the structure of the double helix, 00:25:41.000 |
but it was sort of a guess, it wasn't really verified yet. 00:25:51.880 |
And it doesn't matter if other people didn't believe it 00:25:59.080 |
And that's the kind of thing you hear a lot with scientists 00:26:15.920 |
- Yeah, in fact, Francis Crick was very interested 00:26:28.800 |
And that was when I decided I'm going to leave 00:26:35.600 |
I'm just reading that one essay from Francis Crick. 00:26:39.880 |
I spoke at the Salk Institute and he was in the audience. 00:26:58.680 |
And so we weren't really overlapping a lot there. 00:27:07.960 |
When he was director of the Cold Spring Harbor Laboratories, 00:27:16.600 |
And so he had a personal interest in this field. 00:27:22.280 |
And in fact, the last time was a little bit over a year ago. 00:27:30.360 |
about the progress we were making in our work. 00:27:46.640 |
Next to him was the director of the lab, Bruce Stillman. 00:27:50.160 |
So these guys are in the front row of this auditorium. 00:27:52.560 |
Nobody else in the auditorium wants to sit in the front row 00:27:54.600 |
because there's Jim Watson and there's the director. 00:27:57.000 |
And I gave a talk and I had dinner with Jim afterwards. 00:28:08.560 |
sort of explaining the basics of this new framework we have. 00:28:21.760 |
the first public talk he gave was at Cold Spring Harbor Labs. 00:28:25.920 |
And there's a picture, there's a famous picture 00:28:29.320 |
with an overhead thing pointing at something, 00:28:33.320 |
And it actually looks a lot like the picture of me. 00:28:37.440 |
and there's Jim Watson staring intently at it. 00:28:39.280 |
And of course, there was whatever, 60 years earlier, 00:28:41.640 |
he was standing, pointing at the double helix. 00:28:44.280 |
- It's one of the great discoveries in all of whatever, 00:28:54.520 |
Do you think in terms of evolutionary timeline in history, 00:28:58.360 |
the development of the neocortex was a big leap 00:29:06.040 |
So like if we ran the whole thing over again, 00:29:12.640 |
how likely would we develop the mechanism of the neocortex? 00:29:15.280 |
- Okay, well, those are two separate questions. 00:29:35.880 |
I don't really have any idea how likely it is. 00:29:38.400 |
If we look at evolution, we have one data point, 00:29:45.180 |
whether it was introduced here or it created here 00:29:48.080 |
or someone introduced it, we don't really know, 00:29:51.160 |
It took a long, long time to get to multicellular life. 00:29:57.160 |
it took a long, long time to get the neocortex. 00:30:02.280 |
And we've only had the neocortex for a few hundred thousand 00:30:09.560 |
Well, it certainly isn't something that happened 00:30:17.160 |
that would happen instantaneously on other planets 00:30:20.560 |
It might take several billion years on average. 00:30:25.680 |
for several billion years to find out, probably. 00:30:43.920 |
Pretty much, let's start with a little preface. 00:30:47.700 |
Many of the things that humans are able to do 00:30:50.460 |
do not have obvious survival advantages precedent. 00:31:00.200 |
Is there a really survival advantage to that? 00:31:04.840 |
Is there a real survival advantage to mathematics? 00:31:09.520 |
You can try to figure these things out, right? 00:31:14.760 |
everything had immediate survival advantages to it. 00:31:18.660 |
So I'll tell you a story, which I like, may or may not be true 00:31:43.680 |
that kind of just keeps calming on new things 00:31:48.700 |
Initially it had a very clear survival advantage 00:31:52.500 |
in that it produced better vision and better hearing 00:31:57.780 |
But what I think happens is that evolution discovered, 00:32:01.100 |
it took a mechanism and this is in our recent theory 00:32:05.060 |
but it took a mechanism that evolved a long time ago 00:32:08.140 |
for navigating in the world, for knowing where you are. 00:32:10.380 |
These are the so-called grid cells and place cells 00:32:15.140 |
And it took that mechanism for building maps of the world 00:32:37.240 |
- Concepts, yes, and not just almost, exactly. 00:32:40.280 |
And so, and it just started replicating this stuff. 00:32:50.080 |
to solve certain problems that are important to survival 00:33:06.760 |
but aren't really clearly survival characteristics. 00:33:13.860 |
And that it seems to only have happened in humans 00:33:22.900 |
we've sort of escaped the gravity of evolutionary pressure 00:33:34.320 |
which may not really help us, doesn't matter. 00:33:40.200 |
or that there might be the age of the universe 00:33:50.420 |
- Or at least not in the obvious way perhaps. 00:33:54.840 |
if you look at the entire universe in an evolutionary way, 00:33:58.500 |
it's required for us to do interplanetary travel 00:34:04.460 |
- Yeah, but evolution works at one time frame. 00:34:08.140 |
- It's survival, if you think of survival of the phenotype, 00:34:18.660 |
I'm not transferring any genetic traits to my children 00:34:23.380 |
that are gonna help them survive better on Mars. 00:34:25.860 |
- Right, totally different mechanism, that's right. 00:34:32.940 |
I don't know if you have a nice name, Thousand-- 00:34:35.580 |
- We call it the Thousand Brain Theory of Intelligence. 00:34:38.420 |
Can you talk about this idea of spatial view of concepts 00:34:51.140 |
That's a very simple, this is really what happened. 00:34:54.580 |
We were deep into problems about understanding 00:35:03.020 |
And I was holding a coffee cup just like this in my hand. 00:35:07.220 |
And my finger was touching the side, my index finger, 00:35:12.700 |
and I was gonna feel the rim at the top of the cup. 00:35:20.100 |
I know that my brain predicts what it's gonna feel 00:35:26.060 |
And so we know that the brain's making predictions 00:35:28.500 |
So the question is, what does it take to predict that? 00:35:34.600 |
the brain has to know it's touching a coffee cup, 00:35:37.980 |
and needs to know where the finger currently is 00:35:44.420 |
it needs to know where it's going to be on the cup 00:35:46.340 |
after the movement is completed relative to the cup. 00:35:56.380 |
needs to know that it's sensing it's touching a cup, 00:35:59.380 |
and it needs to know the location of my finger 00:36:01.420 |
relative to that cup in a reference frame of the cup. 00:36:04.380 |
It doesn't matter where the cup is relative to my body, 00:36:13.540 |
has a reference frame that's anchored to the cup. 00:36:17.340 |
'Cause otherwise I wouldn't be able to say the location, 00:36:19.260 |
and I wouldn't be able to predict my new location. 00:36:26.260 |
and therefore every part of my skin is making predictions, 00:36:28.100 |
and every part of my skin must have a reference frame 00:36:33.520 |
So the big idea is that throughout the neocortex, 00:36:46.720 |
You can think of them like XYZ reference frames, 00:36:50.360 |
We know a lot about the neural mechanisms for this, 00:37:01.160 |
well, I would bring it up in some CAD software, 00:37:04.760 |
and say this features at this locations and so on. 00:37:14.320 |
And then a zillion things fell into place after that, 00:37:27.680 |
and extracting features from that sensory data, 00:37:29.800 |
and then extracting features from the features, 00:37:31.800 |
very much like a deep learning network does today. 00:37:41.840 |
And there are thousands, hundreds of thousands of them 00:37:51.040 |
you understand that it explains almost every, 00:37:53.800 |
almost all the mysteries we've had about this structure. 00:38:08.640 |
If you take every little square millimeter of the cortex, 00:38:34.080 |
because I'm now filling in that three-dimensional map, 00:38:50.280 |
which is only looking at a small part of the world 00:38:55.080 |
And so if you think about the part of the brain 00:38:59.080 |
so they're spread across the top of your head here. 00:39:10.040 |
is that all of them are building models of this cup, 00:39:19.480 |
but they're all building models of something. 00:39:26.860 |
there are multiple models of the cup being invoked. 00:39:30.600 |
there are again many models of the cup being invoked 00:39:38.920 |
It's just like your fingers touching the cup. 00:39:43.160 |
And thousands and thousands of models of the cup 00:39:56.440 |
There are thousands of models of your cell phone 00:40:04.960 |
- So that's a really compelling and interesting idea. 00:40:08.840 |
So one on the ensemble part of everything coming together, 00:40:13.760 |
How do you know which one has done the best job 00:40:20.540 |
There's a problem that's known in neuroscience 00:40:32.440 |
Oh, by now it's all spread out all over the place 00:40:45.440 |
but I also have sounds or touches associated with the cup. 00:40:54.280 |
As if all these disparate parts have to be brought together 00:41:01.160 |
The right idea is that you got all these guys voting. 00:41:11.920 |
on black and white and ones focusing on color. 00:41:14.480 |
There's just thousands and thousands of models of this cup. 00:41:17.920 |
They don't actually come together in one spot. 00:41:21.920 |
Imagine you have these columns are like about the size 00:41:29.960 |
They're not physical, but you can think of them that way. 00:41:51.000 |
Or if I had to look through the world through a straw, 00:41:58.160 |
But if I open my eyes, I see the whole thing at once. 00:42:00.480 |
So what we think is going on is all these little pieces 00:42:02.800 |
of spaghetti, if you will, all these little columns 00:42:16.560 |
It's a probabilistic guess of what they might be touching. 00:42:25.800 |
and it's not really a probability distribution. 00:42:29.480 |
In the brain, it doesn't work as a probability distribution. 00:42:39.960 |
And another column says, I think it could be a coffee cup 00:42:42.320 |
or a telephone or a camera or whatever, right? 00:42:46.100 |
And all these guys are saying what they think it might be. 00:42:53.480 |
So there's some layers in some cells types in each column 00:43:01.760 |
And so there's a simple associative memory mechanism. 00:43:07.360 |
That says they can all quickly settle on the only 00:43:16.400 |
they all vote and say, yep, it's gotta be the coffee cup. 00:43:18.960 |
And at that point, they all know it's a coffee cup. 00:43:21.080 |
And at that point, everyone acts as if it's a coffee cup. 00:43:24.240 |
even though I've only seen one little piece of this world, 00:43:30.920 |
are looking at different parts in different places, 00:43:33.060 |
different sensory input, different locations. 00:43:36.220 |
But this layer that's doing the voting, it solidifies. 00:43:44.160 |
And so you don't bring these models together in one model, 00:43:46.480 |
you just vote and there's a crystallization of the vote. 00:43:49.160 |
- Great, that's at least a compelling way to think 00:44:04.720 |
that this extends to much more than coffee cups? 00:44:15.040 |
And well, the first, the primary face of evidence for that 00:44:20.720 |
that are associated with language or high level thought 00:44:31.640 |
And so one would say, well, if Vernon Mouncastle, 00:44:36.440 |
who proposed that all the parts of the neocortex 00:44:45.760 |
They must be working on the principle of reference frames. 00:44:50.200 |
But of course, we had no prior idea how these things happen. 00:45:22.720 |
And the evidence would suggest it's how it's happening. 00:45:27.600 |
Like, what does it mean to do high-level thought? 00:45:30.120 |
How would that fit into a reference frame framework? 00:45:36.120 |
I don't know if you could tell me if there's a connection, 00:45:45.200 |
that helps you remember completely random concepts 00:45:47.880 |
by trying to put them in a physical space in your mind 00:45:54.760 |
For some reason, that seems to work really well. 00:45:57.680 |
Now, that's a very narrow kind of application 00:46:04.000 |
So this seems like you're describing a mechanism 00:46:09.680 |
- So basically, the way, what we think is going on 00:46:11.880 |
is all things you know, all concepts, all ideas, 00:46:15.120 |
words, everything you know are stored in reference frames. 00:46:24.320 |
you have to basically navigate through a reference frame 00:46:28.640 |
and the same way my finger navigates to this coffee cup. 00:46:39.360 |
you already know very well to see your house, right? 00:46:42.120 |
And the idea of the method of loci is you can say, 00:46:43.640 |
"Okay, in my lobby, I'm gonna put this thing. 00:46:51.760 |
You just walk mentally, you walk through your house. 00:46:54.200 |
You're mentally moving through a reference frame 00:46:59.360 |
there's two things that are really important about it. 00:47:00.680 |
It tells us the brain prefers to store things 00:47:08.320 |
is to move mentally through those reference frames. 00:47:11.600 |
You could move physically through some reference frames. 00:47:13.600 |
Like I could physically move through the reference frame 00:47:16.360 |
I can also mentally move through the reference frame 00:47:26.800 |
There was some recent research using human subjects 00:47:31.440 |
in fMRI, and I'm gonna apologize for not knowing the name 00:47:36.720 |
But what they did is they put humans in this fMRI machine, 00:47:42.840 |
And they gave the humans tasks to think about birds. 00:47:49.680 |
and long necks and long legs, things like that. 00:47:57.600 |
you get to tell when humans were thinking about the birds, 00:48:10.360 |
and there are grid cell-like patterns of activity 00:48:20.160 |
that even when you're thinking about something abstract, 00:48:28.800 |
these grid cells are the basic same neural mechanisms 00:48:33.560 |
in the old part of the brain, the entorhinal cortex, 00:48:40.080 |
It's the same nature to preserve this interesting way 00:48:46.960 |
that when you think about concepts like birds, 00:49:01.120 |
And when you think about something, you go through that, 00:49:03.520 |
you can make the same example, let's take a mathematics. 00:49:10.040 |
Conjecture is a statement you believe to be true, 00:49:21.120 |
you say, well, I know this is true, and I know this is true. 00:49:23.240 |
And I think that maybe to get to the final proof, 00:49:25.880 |
I need to go through some intermediate results. 00:49:28.640 |
What I believe is happening is literally these equations 00:49:33.120 |
or these points are assigned to a reference frame, 00:49:41.680 |
but you might be able to plus transform or something else. 00:49:44.080 |
That is like a movement in the reference frame of the math. 00:49:47.520 |
And so you're literally trying to discover a path 00:49:56.160 |
And if you can get to these intermediate results, 00:50:00.440 |
and you know you're using the right operations. 00:50:02.960 |
Much of what we think about is solving hard problems 00:50:05.960 |
is designing the correct reference frame for that problem, 00:50:15.240 |
- Yeah, so if you dig in an idea of this reference frame, 00:50:19.320 |
whether it's the math, you start a set of axioms 00:50:24.320 |
Can you try to describe, maybe take a step back, 00:50:28.160 |
how you think of the reference frame in that context? 00:50:30.680 |
Is it the reference frame that the axioms are happy in? 00:50:35.680 |
Is it the reference frame that might contain everything? 00:50:44.560 |
the thousand brain theory of intelligence says 00:50:50.840 |
And we can talk about this, the mathematics work out, 00:50:55.960 |
- But how many reference frames does a coffee cup have? 00:51:00.080 |
- Let's say you ask how many reference frames 00:51:07.440 |
Because there are many, many models of the coffee cup. 00:51:15.200 |
how many different things can my finger learn? 00:51:21.760 |
everything you've ever know about that you can say, 00:51:23.840 |
I know that thing has a reference frame associated with it. 00:51:28.240 |
And what we do when we build composite objects, 00:51:33.960 |
So my coffee cup has multiple components to it. 00:51:37.040 |
It's got a rim, it's got a cylinder, it's got a handle. 00:51:39.800 |
And those things have their own reference frames 00:51:42.800 |
and they're assigned to a master reference frame, 00:51:48.160 |
Well, that's something that exists elsewhere in the world. 00:51:53.120 |
well, how can I assign the Numenta logo reference frame 00:51:59.240 |
So it's all, we talked about this in the paper 00:52:05.780 |
The idea of how you can assign reference frames 00:52:08.800 |
to reference frames, how neurons could do this. 00:52:12.680 |
even though you mentioned reference frames a lot, 00:52:20.160 |
I mean, it was already helpful for me to understand 00:52:26.360 |
- Okay, so let's just say that we're gonna have 00:52:28.800 |
some neurons in the brain, not many actually, 00:52:35.400 |
Right, what is a reference frame in this case? 00:52:37.320 |
First of all, these reference frames are different 00:52:43.440 |
For example, we know the Cartesian coordinates, XYZ, 00:53:03.560 |
The interesting thing about the reference frames 00:53:07.880 |
because these have been established through neuroscience 00:53:13.560 |
This is known neuroscience in an old part of the brain. 00:53:29.060 |
you can then tell what the next point should be. 00:53:31.400 |
And you can then tell what the next point would be, 00:53:43.200 |
Or how do I get my finger from the side of my cup 00:53:46.720 |
How do I get from the axioms to the conjecture? 00:53:54.440 |
And I can, if you want, I can describe in more detail, 00:54:02.960 |
- But is it helpful to think of it as spatial in some sense? 00:54:17.480 |
In the old part of the brain, the entorhinal cortex, 00:54:24.280 |
It's like the rat is in some box in a maze or whatever, 00:54:35.560 |
That's a mammal and they fly in three dimensional space. 00:54:41.760 |
So this is a current area of active research. 00:54:46.440 |
in the entorhinal cortex can learn three dimensional space. 00:54:59.560 |
It's on bioRxiv, where they show that you can, 00:55:08.120 |
but grid cells can represent any n-dimensional space. 00:55:21.920 |
There's a whole bunch of two dimensional models. 00:55:24.280 |
And you can just, you can slice up any n-dimensional space 00:55:29.320 |
So, and you could have one dimensional model. 00:55:31.640 |
So there's nothing inherent about the mathematics 00:55:35.800 |
which constrain the dimensionality of the space, 00:55:41.460 |
So obviously I have a three dimensional map of this cup. 00:55:44.080 |
Maybe it's even more than that, I don't know. 00:55:46.360 |
But it's clearly a three dimensional map of the cup. 00:56:00.120 |
building up more and more information over time, 00:56:04.060 |
do you think that mechanism is well understood? 00:56:06.440 |
In your mind, you've proposed a lot of architectures there. 00:56:11.860 |
is the big piece, the thousand brain theory of intelligence, 00:56:30.720 |
And with that as a framework for how the brain works, 00:56:40.560 |
Well, it turns out, if you think about grid cells 00:56:42.540 |
and place cells in the old parts of the brain, 00:56:47.060 |
There's a lot of debate about exactly the details, 00:56:50.720 |
And we have that still, that same level of detail, 00:57:05.240 |
It's not like, oh, this thing seems to work, we're done. 00:57:08.800 |
but these are other things we know it has to do 00:57:20.000 |
but the basic principles about how different layers 00:57:23.140 |
in the neocortex are doing much of this, we understand, 00:57:29.980 |
- So what would you say is one of the harder open problems 00:57:34.060 |
or one of the ones that have been bothering you, 00:57:38.420 |
- Oh, well, right now, this is a detailed thing 00:57:46.200 |
We've talked about as if, oh, to predict what you're going 00:57:51.500 |
where my finger is gonna be on the coffee cup. 00:57:55.420 |
Think about my finger touches the edge of the coffee cup. 00:57:58.500 |
My finger can touch it at different orientations. 00:58:10.100 |
there's an orientation component of this as well. 00:58:13.300 |
This is known in the old parts of the brain too, 00:58:20.440 |
So if my finger were a rat, in three dimensions, 00:58:28.620 |
you might think of it as a two-dimensional location, 00:58:30.620 |
a two-dimensional, a one-dimensional orientation, 00:58:43.940 |
as well as the location, is a tricky problem. 00:58:55.140 |
so perspective's super interesting, but super specific. 00:59:06.940 |
the fact that we are in a building in North America, 00:59:12.620 |
that we, in the day and age where we have mugs, 00:59:27.340 |
- Yeah, and that is another really interesting question. 00:59:37.980 |
- And also the question, does it matter, because-- 00:59:56.500 |
I think the way to think about it is the following, 01:00:01.740 |
is we have all these models of the world, okay? 01:00:06.180 |
And as I said earlier, I kind of snuck it in there, 01:00:08.900 |
our models are actually, we build composite structure. 01:00:12.500 |
So every object is composed of other objects, 01:00:21.580 |
Now we can just arrange these things in a certain way, 01:00:24.300 |
and go, oh, that's in the Nementa Conference Room. 01:00:26.600 |
And what we do is when we go around the world, 01:00:36.420 |
the first thing I do is say, oh, I'm in this room. 01:00:39.340 |
Then I can say, oh, look, there's a table here. 01:00:44.120 |
I'm then assigning this table in a context of the room. 01:00:46.260 |
Then I say, oh, on the table, there's a coffee cup. 01:00:51.860 |
Oh, and look, in the logo, there's the letter E. 01:00:56.360 |
And it doesn't actually, but pretend it does. 01:00:58.620 |
So the point is, your attention is kind of drilling 01:01:07.460 |
And I can pop back up, and I can pop back down. 01:01:13.220 |
I haven't lost the context of everything else, 01:01:15.660 |
but it's sort of, there's this sort of nested structure. 01:01:18.900 |
- So the attention filters the reference frame formation 01:01:36.660 |
But until, but most people don't even think about this. 01:01:41.660 |
oh, I looked at the chair, and I looked at the board, 01:01:47.300 |
- So what percent of your day are you deeply aware of this, 01:01:49.980 |
and what part can you actually relax and just be Jeff? 01:01:55.180 |
- Unfortunately, I'm afflicted with too much of the former. 01:02:01.580 |
- Well, unfortunately or unfortunately, okay. 01:02:06.780 |
I think about this stuff almost all the time. 01:02:15.820 |
And then I stay awake for at least an hour with my eyes shut 01:02:19.340 |
in sort of a half-sleep state thinking about these things. 01:02:21.620 |
I come up with answers to problems very often 01:02:37.820 |
- Are you, when you're thinking about this stuff, 01:02:41.180 |
like almost taking a step outside of yourself 01:02:43.700 |
and trying to figure out what is your mind doing right now? 01:02:45.700 |
- I do that all the time, but that's not all I do. 01:02:50.780 |
So as soon as I started thinking about grid cells, 01:02:58.140 |
You know, that's where you know where you are. 01:03:00.700 |
we always have a sense of where we are, unless we're lost. 01:03:19.420 |
in the middle of the night to go to the bathroom, 01:03:23.500 |
I mean, the coffee cup is an example of that too. 01:03:25.620 |
So I think, I find that these sort of everyday 01:03:41.740 |
but you have to sort of build up those constraints 01:03:44.140 |
and the knowledge about the field and who's doing what 01:03:46.860 |
and what exactly they think is happening here. 01:03:59.260 |
I come in with these introspective ideas and say, 01:04:14.780 |
of the neuroscience, then it's really helpful. 01:04:17.780 |
- So let's talk a little bit about deep learning 01:04:20.180 |
and the successes in the applied space of neural networks. 01:04:37.460 |
of being able to generalize from the training set 01:04:41.700 |
onto data that's similar to that training set. 01:04:52.180 |
of constructing a theory of human intelligence? 01:04:55.140 |
- Yeah, well, I'm not an expert in this field. 01:04:57.840 |
I'm somewhat knowledgeable, so I, but I'm not-- 01:05:02.540 |
- Well, I have a little bit more than intuition, 01:05:07.740 |
do I spend all my time thinking about neuroscience? 01:05:09.260 |
I do, that's to the exclusion of thinking about things 01:05:15.300 |
So look, I think it's great, the progress they've made. 01:05:21.860 |
The models that we have today are actually derived 01:05:33.300 |
They use things that we might call them neurons, 01:05:45.500 |
from neuroscience, and so there's a lot of things, 01:05:48.060 |
the learning rules, basically, not back prop, 01:05:50.520 |
but other, you know, sort of heavy entitlement. 01:05:52.140 |
- I'd be curious to say they're not neurons at all. 01:06:00.380 |
in which you think are the biggest differences. 01:06:11.420 |
A real neuron in the brain is a complex thing. 01:06:27.240 |
the ones that are close to the soma, the cell body, 01:07:04.480 |
meaning they're all receiving an input at the same time, 01:07:09.080 |
within a very short distance on the dendrite, 01:07:14.560 |
right next to each other at some distant place, 01:07:21.260 |
And dendritic spike travels through the dendrites 01:07:26.860 |
Now, when it gets there, it changes the voltage, 01:07:31.220 |
which is sort of like gonna make the cell fire, 01:07:36.020 |
It's sort of what we call it says we depolarize the cell, 01:07:58.420 |
They can write about 10, 20 synapses at a time, 01:08:04.420 |
So the neuron actually is a predictive engine on its own. 01:08:21.220 |
is that when it actually does produce a spike 01:08:24.740 |
it does so slightly sooner than it would have otherwise. 01:08:31.780 |
there's all the neurons in the excitatory neurons 01:08:34.040 |
in the brain are surrounded by these inhibitory neurons. 01:08:36.660 |
And they're very fast, the inhibitory neurons, 01:08:40.440 |
And if I get my spike out a little bit sooner 01:08:43.300 |
than someone else, I inhibit all my neighbors around me. 01:08:47.380 |
So what you end up with is a different representation. 01:08:57.880 |
And so we showed how networks of these neurons 01:09:00.300 |
can do very sophisticated temporal prediction, basically. 01:09:07.020 |
real neurons in the brain are time-based prediction engines. 01:09:18.100 |
I don't think you can build a brain without them. 01:09:20.060 |
I don't think you can build intelligence without them, 01:09:21.340 |
because it's where a large part of the time comes from. 01:09:27.080 |
and the time is, there's a prior and a prediction 01:09:49.900 |
So even one digit of precision is not possible. 01:09:52.480 |
So the way real neurons work is they don't add these, 01:10:02.540 |
is detect the presence of some 10 to 20 active synapses 01:10:16.260 |
and I think that's actually another essential component, 01:10:20.980 |
and all that mechanism is based on sparse patterns, 01:10:24.220 |
and I don't actually think you could build real brains 01:10:30.740 |
- It's hard to even think about the complexity 01:10:41.020 |
and everything you just mentioned in the past couple minutes. 01:10:47.980 |
It's not like, it's no longer a mystery to me. 01:10:49.900 |
- No, but sorry, as a function, in a mathematical way, 01:11:10.820 |
Oh, I have four neurons and they're doing this. 01:11:16.340 |
Even the complexity of convolutional neural networks today, 01:11:24.820 |
My colleague Subitain Ahmad, he did a nice paper on this. 01:11:34.140 |
Talking about sort of the mathematical properties 01:11:37.700 |
And so we can't, what we can do is we can show mathematically 01:11:40.660 |
for example, why 10 to 20 synapses to recognize a pattern 01:11:50.020 |
We can show mathematically some of these concepts 01:12:12.020 |
But there is mathematical benefits and intuitions 01:12:20.620 |
Most of our papers have a section about that. 01:12:23.340 |
- So I think it's refreshing and useful for me 01:12:25.940 |
to be talking to you about deep neural networks. 01:12:30.940 |
that we can't achieve anything like intelligence 01:12:37.820 |
- I'm sure we can do it in the ultimate form. 01:12:41.300 |
and see what your thoughts are there a little bit. 01:12:43.340 |
So I'm not sure if you read this little blog post 01:12:48.260 |
Recently he's a reinforcement learning pioneer. 01:12:53.260 |
His basic idea is that all the stuff we've done in AI 01:12:56.780 |
in the past 70 years, he's one of the old school guys. 01:13:12.100 |
is a simple general method that just relies on Moore's Law. 01:13:40.620 |
That you just try to go as general as possible 01:13:44.220 |
and wait years or decades for the computation 01:14:02.380 |
you guys are trying to do, we are trying to do, 01:14:07.380 |
He's saying it's only going to be short-term gains. 01:14:19.060 |
is do you think if we just scale things up significantly, 01:14:53.940 |
But I don't think you can get to the kind of things 01:15:00.740 |
and that we think of as intelligence by just scaling it up. 01:15:07.700 |
what's happened recently with the reemergence 01:15:25.920 |
on the whole cortical theory in the last few years 01:15:28.940 |
that last year we decided to start actively pursuing 01:15:33.940 |
how do we get these ideas embedded into machine learning? 01:15:39.180 |
Well, that's again, being led by my colleague, 01:15:41.860 |
Subrata Iman, and he's more of a machine learning guy 01:15:46.740 |
So this is now our new, this is, I wouldn't say our focus, 01:15:54.180 |
because we need to proselytize what we've learned 01:16:03.740 |
So we're putting, we have a plan in place right now. 01:16:05.580 |
In fact, we just did our first paper on this. 01:16:17.120 |
and maybe we should just think about this a bit more 01:16:20.860 |
and what are those team at Numenta, what have they done? 01:17:01.100 |
and you say, I can see that long-term vision, 01:17:25.380 |
So just in case you don't know what that means 01:17:28.420 |
or some of the listeners don't know what that means. 01:17:34.100 |
what you would see is maybe 2% of them active at a time. 01:17:56.220 |
They're sitting there in a little block together. 01:17:57.940 |
It's a teeny little block of neurons, 10,000 neurons. 01:18:02.500 |
they're representing the input from my sensors. 01:18:11.900 |
So which 200 cells are active tells me what's going on. 01:18:14.980 |
It's not, individual cells aren't that important at all. 01:18:23.140 |
then all kinds of beautiful properties come out of them. 01:18:28.060 |
And we've written and described these benefits 01:18:32.420 |
So they give this tremendous robustness to the systems. 01:18:56.820 |
Now other people are thinking along these lines, 01:18:58.100 |
but we're going about it in a more principled way, I think. 01:19:00.940 |
And we're showing that if you enforce sparseness 01:19:04.060 |
throughout these convolutional neural networks, 01:19:06.380 |
in both the, which sort of which neurons are active 01:19:15.640 |
So one of the current hot topics in deep learning right now 01:19:23.500 |
and I can give you a picture that looks perfect 01:19:34.120 |
They're trying to have some contest for this. 01:19:36.580 |
But if you enforce sparse representations here, 01:19:44.940 |
So we've already shown some of those results. 01:19:52.780 |
And you can, I think it's on bioRxiv right now or on iCry, 01:20:02.460 |
Okay, that's a take something from the brain. 01:20:09.500 |
- What's your intuition why sparsity leads to robustness? 01:20:12.380 |
'Cause it feels like it would be less robust. 01:20:19.060 |
- So it just feels like if the fewer neurons are involved, 01:20:36.380 |
So for engineers, machine learning people, this would be easy 01:20:45.700 |
you're trying to divide some very high dimensional space 01:20:50.340 |
And you're trying to create some point where you say, 01:20:52.780 |
all these points in this high dimensional space are A 01:20:54.740 |
and all these points in this high dimensional space are B. 01:20:57.540 |
And if you have points that are close to that line, 01:21:12.680 |
imagine I pick, I'm gonna pick 200 cells active 01:21:20.320 |
Now let's say I pick randomly a different representation, 200. 01:21:24.380 |
The overlap between those is gonna be very small, 01:21:28.040 |
I can pick millions of samples randomly of 200 neurons 01:21:32.800 |
and not one of them will overlap more than just a few. 01:21:41.440 |
to look like one of those other representations, 01:21:52.640 |
- In terms of further, so you mentioned sparsity. 01:22:02.360 |
So again, we're trying to come up incremental ways 01:22:07.840 |
to machine learning, current machine learning world 01:22:19.060 |
that you have many, many models that are voting. 01:22:27.120 |
But the way the brain does it is a little different. 01:22:33.600 |
And the kind of way it represents uncertainty is different. 01:22:39.940 |
but we're gonna try to see if we can sort of incorporate 01:22:45.920 |
like lots of simple models that talk to each other 01:23:10.080 |
the machine learning, computer vision community 01:23:18.160 |
And I would argue, especially from your perspective, 01:23:24.680 |
for testing the aspects that the brain is good at 01:23:31.280 |
It's a very fine, and it's been extremely useful 01:23:41.680 |
So you think you also have a role in proposing better tests? 01:24:06.600 |
you have to have huge data sets and huge computing power. 01:24:21.120 |
We come at it, our approach to this is all based on, 01:24:26.120 |
We're coming at it from like a theoretical base 01:24:27.760 |
that we think, oh my God, this is so clearly elegant. 01:24:29.980 |
This is how brains work, this is what intelligence is. 01:24:31.880 |
But the machine learning world has gotten in this phase 01:24:36.600 |
as long as you do 0.1% better on this benchmark, 01:24:42.740 |
We have to figure out how to get around that. 01:24:47.320 |
That's one of the challenges that we have to deal with. 01:24:55.920 |
But part of the reasons I'm talking to you here today 01:24:59.960 |
is I hope I'm going to get some machine learning people 01:25:05.200 |
I'm tired of doing this 0.1% improvement stuff. 01:25:10.440 |
because I think machine learning now as a community 01:25:15.520 |
needs to be orthogonal to what has received success 01:25:31.040 |
maybe we should look at the brain, things like that. 01:25:33.440 |
So hopefully that thinking will occur organically. 01:25:38.080 |
And then we're in a nice position for people to come 01:25:43.160 |
- Yeah, MIT is launching a billion dollar computing college 01:25:51.000 |
- Well, the idea that the humanities, psychology, 01:25:58.920 |
- Yeah, I mean, Stanford just did this human centered AI. 01:26:02.560 |
I'm a little disappointed in these initiatives because, 01:26:05.360 |
you know, they're focusing on sort of the human side of it 01:26:12.200 |
how humans interact with intelligent machines, 01:26:17.640 |
but that is orthogonal to what we're trying to do. 01:26:23.720 |
In fact, I want to build intelligent machines 01:26:25.480 |
that aren't emotional, that don't smile at you, 01:26:31.800 |
- Yeah, there is that pattern that when you talk about 01:26:38.360 |
that you start slipping into topics of ethics or, 01:26:41.860 |
yeah, like you said, the interactive elements 01:26:57.720 |
But until you have that theory about what the brain does, 01:27:00.680 |
You know, it's just, you're going to be wasting time. 01:27:03.200 |
- Just to break it down on the artificial neural network side, 01:27:05.560 |
maybe you can speak to this on the biological neural network 01:27:19.840 |
there's a difference between the learning stage 01:27:25.000 |
One of the big distinctions that people often say, 01:27:30.640 |
is artificial neural networks need a lot of data. 01:27:42.000 |
or is that just something we deceive ourselves with? 01:27:52.320 |
So remember I talked early about the constraints. 01:27:54.840 |
We always feel, well, one of those constraints 01:27:57.280 |
is the fact that brains are continually learning. 01:28:00.960 |
That's not something we said, oh, we can add that later. 01:28:14.440 |
on sequence memory, we showed how that happens. 01:28:16.760 |
How do brains infer and learn at the same time? 01:28:37.160 |
There are some parts of the neocortex in the brain 01:28:44.240 |
they're like cycles of activity in the brain. 01:28:49.240 |
that you're doing more of inference on one part of the phase 01:28:52.280 |
and more of learning on the other part of the phase. 01:28:58.320 |
But in general, I would say that's an important problem. 01:29:01.560 |
We have all of our networks that we've come up with do both. 01:29:05.600 |
And they're learning, continuous learning networks. 01:29:13.640 |
- So we have to like, we get in our little soapbox 01:29:23.920 |
in some commercial system or something, it's a little harder. 01:29:26.680 |
- So yeah, one of the things I had to linger on that is, 01:29:30.080 |
in some ways to learn the concept of a coffee cup, 01:29:41.760 |
and I'm reaching, I'm trying to touch something. 01:29:43.640 |
I don't know up front if it's something I already know 01:29:47.800 |
And I have to, I'm doing both at the same time. 01:29:50.440 |
I don't say, oh, let's see if it's a new thing. 01:29:55.520 |
As I go, my brain says, oh, it's new or it's not new. 01:29:59.360 |
And if it's new, I start learning what it is. 01:30:02.240 |
So, and by the way, it starts learning from the get go, 01:30:10.080 |
The other thing you mentioned was the fast learning. 01:30:12.640 |
So I was just talking about continuous learning, 01:30:34.280 |
I mentioned this earlier, but I'll mention it again. 01:30:36.880 |
imagine I am a section of a dendrite of a neuron. 01:30:39.280 |
And I wanna learn, I'm gonna learn something new. 01:30:49.000 |
So what I'm gonna do is I'm gonna form new synapses. 01:31:06.480 |
They're not all being added together like a point neuron. 01:31:09.640 |
So if I learn something new on this segment here, 01:31:14.920 |
So I can add something without affecting previous learning. 01:31:19.840 |
Now let's talk, we can talk about the quickness, 01:31:26.800 |
Yes, it can take maybe an hour to form a new synapse. 01:31:32.600 |
And I can explain that in how it's tuned if you want. 01:31:38.360 |
- That's great, but is there an understanding 01:32:06.360 |
the psychologist who proposed, Heb, Donald Heb, 01:32:09.640 |
he proposed that learning was the modification 01:32:12.040 |
of the strength of a connection between two neurons. 01:32:22.360 |
between the effect of one neuron and another. 01:32:33.920 |
It's literally, you can see this little thing going, 01:32:39.760 |
Now, there's another thing that we've speculated 01:32:49.200 |
how do I form a memory really, really quickly? 01:32:56.840 |
So there are types of synapses called silent synapses. 01:33:01.720 |
They look like a synapse, but they don't do anything. 01:33:10.120 |
Some parts of the brain have more of these than others. 01:33:12.480 |
For example, the hippocampus has a lot of them, 01:33:14.040 |
which is where we associate most short-term memory with. 01:33:17.040 |
So what we speculated, again, in that 2016 paper, 01:33:22.080 |
we proposed that the way we form very quick memories, 01:33:28.920 |
is that we convert silent synapses into active synapses. 01:33:33.920 |
It's like saying a synapse has a zero weight and a one weight 01:33:37.840 |
but the long-term memory has to be formed by synaptogenesis. 01:33:49.080 |
It's like, doesn't do anything till it releases transmitter. 01:33:58.480 |
is that most neural networks today are fully connected. 01:34:10.680 |
so that any neuron connects to some subset of the neurons 01:34:15.320 |
and it does so on a dendrite by dendrite segment basis. 01:34:21.560 |
And that then learning is not adjusting all these weights, 01:34:26.320 |
okay, connect to these 10 cells here right now. 01:34:30.120 |
- In that process, with artificial neural networks, 01:34:32.920 |
it's a very simple process of back propagation 01:34:52.000 |
People are trying to make it happen in brains, 01:34:56.760 |
What synaptogenesis is pure Hebbian learning. 01:35:05.320 |
How do I form connections between those active cells? 01:35:07.960 |
And it's literally saying, this guy became active, 01:35:19.880 |
All the networks we do, all the models we have 01:35:22.200 |
work on, almost completely on Hebbian learning, 01:35:26.400 |
but in dendritic segments and multiple synapses 01:35:37.780 |
If you look at the history of artificial intelligence, 01:35:52.080 |
to ask, 'cause no one can predict the future. 01:35:58.160 |
I used to run a different neuroscience institute 01:36:08.000 |
And I used to ask them all the same question. 01:36:10.360 |
I would say, well, how long do you think it'll be 01:36:11.680 |
before we understand how the neocortex works? 01:36:18.200 |
So I got, the typical answer was 50 to 100 years. 01:36:36.280 |
As I mentioned earlier, these are step functions. 01:36:45.600 |
So if I can do my job correctly over the next five years, 01:36:58.760 |
machine learning people should pay attention to these ideas, 01:37:01.440 |
then we're definitely in an under 20 year timeframe. 01:37:04.600 |
If I can do those things, if I'm not successful in that, 01:37:07.800 |
and this is the last time anyone talks to me, 01:37:22.960 |
how quickly are they gonna populate the world? 01:37:28.840 |
but I think if I can do what I said, we're starting it. 01:37:36.160 |
everybody gives up on your ideas for 20 years, 01:37:40.080 |
and then all of a sudden, somebody picks it up again. 01:37:43.600 |
- Yeah, so that would be a failure on my part. 01:37:49.800 |
Charles Babbage, he's the guy who invented the computer 01:37:53.360 |
And everyone forgot about it until 100 years later. 01:37:59.480 |
It's, hey, this guy figured this stuff out a long time ago. 01:38:06.480 |
I recognize this is part of any entrepreneur's challenge. 01:38:15.920 |
And this is the challenge as to how you get people 01:38:52.760 |
I haven't decided yet if that's good or bad for my mission. 01:39:15.440 |
and they don't imagine they need anything else, 01:39:24.280 |
and there was no money for anybody to do anything, 01:39:30.760 |
You want sort of the soft landing approach, right? 01:39:33.400 |
You want enough people, the senior people in AI 01:39:45.280 |
"Okay, let's start a little project on the side here, 01:39:53.960 |
and yet I don't want it to be sunny all the time either. 01:39:59.120 |
to build a system with human level intelligence 01:40:03.000 |
where once demonstrated, you would be very impressed? 01:40:08.720 |
Does it have to have the C word we used before, 01:40:12.760 |
consciousness, as an entirety, in a holistic sense? 01:40:20.480 |
is to create a machine that is human level intelligence. 01:40:24.960 |
Back to Turing, I think it was a false statement. 01:40:30.760 |
of all different scales, all different capabilities. 01:40:36.120 |
that'd be pretty good to have a dog, you know? 01:40:38.480 |
What about something that doesn't look like an animal 01:40:41.600 |
So my thinking about this is that we want to define 01:40:48.760 |
We can then say, okay, we're now gonna build systems 01:40:51.040 |
that work on those principles, or some subset of them, 01:40:54.280 |
and we can apply them to all different types of problems. 01:41:09.640 |
No, no, because we know that what the principles 01:41:11.280 |
of computing are, and I can apply those principles 01:41:14.880 |
And same, intelligence just needs to get there. 01:41:23.200 |
Now, you did bring up a very interesting question 01:41:30.640 |
It has to be able to move through these reference frames 01:41:36.360 |
if I'm gonna have an AI that understands coffee cups, 01:41:40.520 |
and touch it and look at it with its eyes and hands, 01:41:48.200 |
maybe it needs to move through mathematical spaces. 01:41:51.360 |
I could have a virtual AI that lives in the internet, 01:41:59.040 |
and digging into files, but it's got a location 01:42:05.080 |
You can't have an AI that just takes some flash thing input, 01:42:15.280 |
movement pattern, movement pattern, attention, 01:42:22.720 |
whether it's physical or not, has to be part of it. 01:42:25.760 |
- So self-awareness in the way to be able to answer 01:42:31.440 |
- No, no, the very narrow definition of self, 01:42:33.680 |
meaning knowing a sense of self enough to know 01:42:37.720 |
where am I in the space where it's interesting. 01:42:40.360 |
- Basically, the system needs to know its location, 01:42:43.520 |
or each component of the system needs to know 01:42:46.000 |
where it is in the world at that point in time. 01:42:51.680 |
Do you think, one, from the perspective of neuroscience 01:43:05.280 |
- And is it useful, or is it just a side effect of us? 01:43:12.840 |
to figure out how to build intelligent machines. 01:43:21.880 |
that are like, well, if I build a system like this, 01:43:25.320 |
or if I build it like this, it wouldn't be self-aware. 01:43:37.160 |
I can't remember his name, I apologize for that. 01:43:48.280 |
I plug myself in again in the morning, and there I am. 01:43:52.000 |
People get kind of bent out of shape about this. 01:43:56.040 |
I have very definite, very detailed understanding, 01:43:59.520 |
or opinions about what it means to be conscious, 01:44:04.320 |
I don't think it's that interesting a problem. 01:44:10.920 |
I didn't actually listen to your interview with him, 01:44:12.360 |
but I know him, and I know that's the thing he cares about. 01:44:15.800 |
- He also thinks intelligence and consciousness 01:44:33.720 |
is it means different things to different people, 01:44:55.840 |
So it can remember what you did this morning, 01:44:57.520 |
can remember what you had for breakfast, and so on. 01:45:14.160 |
And then I said to you, "Lex, were you conscious 01:45:18.480 |
I said, "Here's a video of you eating the bagel." 01:45:22.280 |
"that's not possible 'cause I must've been unconscious 01:45:25.600 |
So we can just make this one-to-one correlation 01:45:27.400 |
between memory of your body's trajectory through the world 01:45:32.000 |
a memory of it, and the ability to recall that memory 01:45:35.800 |
I was conscious of that, it's a self-awareness. 01:45:48.080 |
"I remember what I did, all right, I got it." 01:45:52.320 |
although some people think that's a hard one. 01:45:57.280 |
is one that's sometimes used, going by the word, equalia, 01:46:17.480 |
these are all just neurons, and so how is it that, 01:46:20.240 |
why does looking at you feel different than hearing you? 01:46:24.080 |
It feels different, but this is neurons in my head, 01:46:33.560 |
he wrote a book called, "Why Red Doesn't Sound Like a Bell." 01:46:37.480 |
It's a little, it's not a trade book, easy to read, 01:46:51.120 |
Property of the world that exists is light frequency, 01:46:54.200 |
and that gets turned into, we have certain cells 01:46:57.440 |
in the retina that respond to different frequencies 01:46:59.280 |
different than others, and so when they enter the brain, 01:47:07.920 |
I mean, there's no color coming in on those synapses, 01:47:24.400 |
why does it even appear to have a color at all? 01:47:37.040 |
having the subject, assigning the feeling of red 01:47:47.960 |
- Yeah, I think that's a good way of putting it. 01:47:55.360 |
to say it's useful to have a model like this. 01:48:07.040 |
And what they claim is they can have their arm is removed, 01:48:21.880 |
And if they move their non-existent arm behind their back, 01:48:33.520 |
And just like, but it's useful to have a model of something 01:48:38.560 |
that sort of correlates to things in the world 01:48:40.400 |
so you can make predictions about what would happen 01:48:44.680 |
but I think you're getting right towards the answer there. 01:48:46.520 |
It's useful for the model of to express things certain ways 01:48:51.360 |
that we can then map them into these reference frames 01:49:01.880 |
- It does feel special that we have subjective experience, 01:49:09.120 |
It's not necessary for the work we're doing here. 01:49:13.160 |
to build intelligent machines at all, not at all. 01:49:24.400 |
intelligent machines, it feels wrong to then turn them off. 01:49:29.400 |
Because if you're able to build a lot of them, 01:49:45.720 |
Well, first of all, I'll say when you're dead, 01:49:48.240 |
it doesn't matter at all, who cares, you're dead. 01:49:53.440 |
One is because we are programmed genetically to fear death. 01:49:57.720 |
That's a survival and propagating the genes thing. 01:50:06.840 |
We don't feel sad for someone we don't know dies. 01:50:09.560 |
they don't understand, I don't feel bad about them 01:50:16.840 |
genetically embedded things that we fear death. 01:50:34.460 |
you know, our whole conception of our world model 01:50:43.800 |
And that death is this terror that underlies it all. 01:51:11.920 |
You haven't, even though you said you've accepted it, 01:51:14.320 |
you haven't really accepted the notion you're gonna die 01:51:19.720 |
it sounds like you disagree with that notion. 01:51:23.680 |
Like, literally, every night I go to bed, it's like dying. 01:51:29.760 |
And if I didn't wake up, it wouldn't matter to me. 01:51:33.000 |
Only if I knew that was gonna happen would it be bothering me. 01:51:35.240 |
If I didn't know it was gonna happen, how would I know? 01:51:39.560 |
So imagine I was a loner and I lived in Alaska. 01:51:43.080 |
And I lived out there and there was no animals, 01:51:57.080 |
- Well, so most people that think about this problem 01:51:59.840 |
would say that you're just deeply enlightened 01:52:05.960 |
But I would say that's a very enlightened way 01:52:17.920 |
I mean, we really don't have an understanding 01:52:22.440 |
of why the heck it is we're born and why we die 01:52:26.120 |
- Well, maybe there isn't a reason, maybe there is. 01:52:27.960 |
So I'm interested in those big problems too, right? 01:52:32.360 |
you know, and there's people like that, right? 01:52:33.680 |
I'm interested in those big problems as well. 01:52:38.360 |
I made a list of the biggest problems I could think of. 01:52:43.480 |
Second, why did we have the laws of physics that we have? 01:52:46.720 |
Third, is life inevitable and why is it here? 01:52:50.200 |
Fourth, is intelligence inevitable and why is it here? 01:53:04.520 |
And so I said, my mission, you know, you asked me earlier, 01:53:13.720 |
because even if it doesn't occur in my lifetime, 01:53:21.260 |
And, but that will be the quickest way for us to, 01:53:29.560 |
we can make super physicists brains that do these things. 01:53:34.280 |
And that can run experiments that we can't run, 01:53:37.520 |
we don't have the abilities to manipulate things and so on, 01:53:41.680 |
that do all those things and with the ultimate goal 01:53:44.880 |
of finding out the answers to the other questions. 01:53:47.700 |
- Let me ask you another depressing and difficult question, 01:53:55.200 |
do you, of creating, no, of understanding intelligence, 01:54:05.800 |
or understanding the answers to the big questions? 01:54:15.720 |
I mean, you know, just put aside this, you know, 01:54:22.800 |
you can think about, we can talk about the risk of AI 01:54:26.600 |
- I'd love to, so let's talk about the Terminator. 01:54:28.280 |
- But I think the world would be far better knowing things. 01:54:32.120 |
Do you think it's better, is it a better place to live in 01:54:40.000 |
I think it's a more, I dread, I sometimes think like, 01:54:43.400 |
God, what it would be like to live 300 years ago? 01:54:45.360 |
I'd be looking up at the sky, I can't understand anything. 01:54:47.440 |
Oh my God, I'd be like going to bed every night going, 01:54:50.160 |
- Well, I mean, in some sense I agree with you, 01:54:54.760 |
So I'm also a scientist, so I share your views, 01:54:57.220 |
but I'm not, we're like rolling down the hill together. 01:55:10.200 |
- We're climbing, we're getting pulled up a hill 01:55:13.840 |
- Our poliocity is, we're pulling ourselves up the hill 01:55:16.960 |
- Yeah, assistive is doing the same thing with the rock. 01:55:29.040 |
of existential threats of intelligence systems? 01:55:31.920 |
- No, I'm not worried about existential threats at all. 01:55:33.760 |
There are some things we really do need to worry about. 01:55:36.380 |
Even today's AI, we have things we have to worry about. 01:55:39.520 |
and about how it impacts false beliefs in the world. 01:55:51.440 |
There's no question, you know, the whole issue about, 01:55:53.840 |
you know, making intelligent armament and weapons 01:55:57.060 |
is something that really we have to think about 01:55:59.920 |
I don't think of those as existential threats. 01:56:01.880 |
I think those are the kind of threats we always face, 01:56:19.140 |
they're based on people who really have no idea 01:56:44.960 |
- Then, so there's a lot of ways in which it could be. 01:56:49.120 |
One way is us humans are actually irrational, inefficient, 01:56:57.320 |
of not happiness, but whatever the objective function is 01:57:05.760 |
- The paperclip problem and things like that. 01:57:10.480 |
So we already faced this threat in some sense. 01:57:18.960 |
that would like to turn everything into bacteria. 01:57:23.040 |
they're constantly changing to evade our protections. 01:57:26.360 |
And in the past, they have killed huge swaths 01:57:52.160 |
actually seems to make it less existential to me. 01:57:57.680 |
It limits the number of things it can do in many ways. 01:58:05.040 |
So the other one, just in your intuition about intelligence, 01:58:09.520 |
when you think about the intelligence of us humans, 01:58:24.680 |
- I wanna make another point here, Lex, before I get there. 01:58:42.840 |
If I wanna have a system that wants to reproduce 01:58:44.520 |
and have sex, make bacteria, make computer viruses. 01:58:53.520 |
But if I just say I wanna have an intelligence system, 01:58:56.080 |
why does it have to have any of the human-like emotions? 01:59:03.800 |
It's just in a trance thinking about mathematics 01:59:27.920 |
as engineers of systems, humans as builders of systems 01:59:34.720 |
- So this is why I mentioned the bacteria one. 01:59:37.560 |
Because you might say, well, some person's gonna do that. 01:59:40.680 |
Well, some person today could create a bacteria 01:59:42.840 |
that's resistant to all the known antibacterial agents. 01:59:52.680 |
So just accept that and then we have to deal with it. 01:59:56.160 |
Yeah, so my point has nothing to do with intelligence. 02:00:03.480 |
that wants to reproduce and do stupid things. 02:00:10.440 |
My dad is a physicist, believes that the reason, 02:00:14.800 |
for example, nuclear weapons haven't proliferated 02:00:21.680 |
there's not that many evil people in the world 02:00:24.520 |
that would use, whether it's bacteria or nuclear weapons, 02:00:36.200 |
And the second is that it's actually really hard, 02:00:39.880 |
So the intersection between evil and competent is small 02:00:45.080 |
- And by the way, to really annihilate humanity, 02:00:46.960 |
you'd have to have sort of the nuclear winter phenomenon, 02:00:50.760 |
which is not one person shooting or even 10 bombs. 02:01:00.400 |
- So it's extreme evil combined with extreme competence. 02:01:10.400 |
I mean, look, we could have some nuclear bomb go off 02:01:14.480 |
I think that's actually quite likely, even in my lifetime. 02:01:23.120 |
And it's the same as, you know, the virus of 1917, 02:01:28.920 |
These bad things can happen, and the plague, and so on. 02:01:38.240 |
until we combine all those crazy things together 02:01:41.200 |
- So on the spectrum of intelligence from zero to human, 02:01:51.560 |
or at least double that of human intelligence? 02:02:01.600 |
Can I make something that's a million times faster 02:02:06.280 |
Could I make something that has a lot more storage 02:02:13.600 |
Can I make something that attaches to different sensors 02:02:24.280 |
Like, you know, they could be all around the place. 02:02:26.760 |
Those are the levers I have, but is it more intelligent? 02:02:47.920 |
we're talking about reference frames and context. 02:02:50.160 |
- Could I have something that's a super physicist 02:02:53.760 |
- And the question is, once you have a super physicist, 02:02:58.780 |
Do you have a sense that it will be orders of magnitude, 02:03:13.280 |
I mean, you know, you can paint it in a fuzzy picture, 02:03:19.920 |
and the deep intuitions are really, really hard. 02:03:28.800 |
but general relativity, man, that's too much. 02:03:31.000 |
And so we already live with this to some extent. 02:03:34.960 |
The vast majority of people can't understand, 02:03:36.880 |
actually, what the vast majority of other people 02:03:39.480 |
We're just, either we don't have the effort to, 02:03:48.560 |
Einstein has spoken in a way that I can understand. 02:03:56.880 |
and think about, you know, concepts that are similar. 02:04:02.200 |
It's not like he's existed in some other plane 02:04:04.040 |
and there's no connection to my plane in the world here. 02:04:18.720 |
that it'll be so far, we can't make the connections. 02:04:26.700 |
when you actually figure it out, it's not that hard. 02:04:29.840 |
almost everyone can understand the multiverses. 02:04:32.160 |
Almost everyone can understand quantum physics. 02:04:34.040 |
Almost everyone can understand these basic things, 02:04:36.120 |
even though hardly any people could figure those things out. 02:04:43.840 |
- You need to only understand the projections, 02:04:47.920 |
the sprinkles of the useful insights from that. 02:04:51.800 |
His general theory of relativity is one thing 02:04:56.240 |
And what if we just said those other few people 02:05:07.600 |
And so did the other people who worked with him. 02:05:18.440 |
- So do you think we are the only intelligent life 02:05:30.620 |
There is a question about contemporaneous intelligence life, 02:05:34.040 |
which is hard to even answer when we think about relativity 02:05:59.280 |
You know, what, 200 years, something like that. 02:06:02.680 |
And we don't have any data, a good data point 02:06:04.920 |
on whether it's likely that we'll survive or not. 02:06:07.420 |
So do I think that there have been intelligent life 02:06:18.840 |
This is another reason I'm excited about our work, 02:06:24.200 |
I think we can build intelligent machines that outlast us. 02:06:32.080 |
You know, they don't have to be tied to earth. 02:06:35.840 |
I'm not saying they're recreating, you know, aliens. 02:06:41.960 |
and this might be a good point to end on here. 02:06:47.280 |
We're not particularly interesting physically. 02:06:58.800 |
that extends beyond what we can actually sense. 02:07:01.200 |
We're the only people who know about the far side of the moon 02:07:09.520 |
There's no, that knowledge doesn't exist anywhere else. 02:07:17.140 |
And that is what we've created that's unique. 02:07:20.400 |
And if I asked me, what is the legacy of humanity? 02:07:33.040 |
is it has to go along with intelligent machines 02:07:43.760 |
We should be thinking about what we wanna leave behind 02:07:52.440 |
- And understanding intelligence and creating intelligence 02:07:58.360 |
- It does give us a better chance to prolong life, yes. 02:08:01.100 |
It gives us a chance to live on other planets. 02:08:04.980 |
I mean, our solar system will disappear one day, 02:08:08.700 |
So I don't know, I doubt we'll ever be able to travel 02:08:15.500 |
but we could send intelligent machines to do that. 02:08:21.820 |
of our knowledge of the echoes of human civilization 02:08:26.100 |
living through the intelligence systems we create? 02:08:30.120 |
Well, I think the intelligence systems are great, 02:08:31.440 |
or in some sense, the vessel for bringing them beyond Earth, 02:08:35.120 |
or making them last beyond humans themselves. 02:08:48.680 |
Human today is not the same as human just 50 years ago. 02:08:56.180 |
As I point out, our genetics are no more interesting 02:08:59.500 |
no more interesting than a monkey's genetics. 02:09:01.780 |
What we have, what's unique and what's valuable 02:09:04.620 |
is our knowledge, what we've learned about the world.